使用python中pytorch庫實(shí)現(xiàn)cnn對(duì)mnist的識(shí)別
使用python中pytorch庫實(shí)現(xiàn)cnn對(duì)mnist的識(shí)別
1 環(huán)境:Anaconda3 64bit https://www.anaconda.com/download/
2 環(huán)境:pycharm 社區(qū)免費(fèi)版 https://www.jetbrains.com/pycharm/download/#section=windows 下載安裝完需要指定上面anaconda的python解釋器為pycharm的python解釋器
3 環(huán)境:pytorch,https://zhuanlan.zhihu.com/p/26871672 中離線安裝方式(下載類似于pytorch-0.1.12-py36_0.1.12cu80.tar.bz2的文件)
4 環(huán)境:Conda install torchvision
5 下載數(shù)據(jù),直接用代碼下載太慢了,用迅雷下載了mnist
6 將數(shù)據(jù)轉(zhuǎn)換成torch可用的格式
7 訓(xùn)練與測(cè)試
data.py
import os
from skimage import io
import torchvision.datasets.mnist as mnist
"""
數(shù)據(jù)集下載地址
http://yann.lecun.com/exdb/mnist/
手動(dòng)下載數(shù)據(jù)集 解壓 檢查文件名和下面幾行代碼中的文件名是否一致
然后啟動(dòng)本代碼
"""
# 下載的數(shù)據(jù)所在目錄
# F:/!BiliBili/!Py/AI/cnn_bili/mnist_data/train-images-idx3-ubyte
# F:/!BiliBili/!Py/AI/cnn_bili/mnist_data/train-labels-idx1-ubyte
# F:/!BiliBili/!Py/AI/cnn_bili/mnist_data/t10k-images-idx3-ubyte
# F:/!BiliBili/!Py/AI/cnn_bili/mnist_data/t10k-labels-idx1-ubyte
root = "F:/!BiliBili/!Py/AI/cnn_bili/mnist_data/"
# 哪個(gè)文件是訓(xùn)練特征(圖) 訓(xùn)練標(biāo)號(hào)(是幾)
train_set = (
mnist.read_image_file(os.path.join(root, 'train-images-idx3-ubyte')),
mnist.read_label_file(os.path.join(root, 'train-labels-idx1-ubyte'))
)
# 哪個(gè)文件是測(cè)試特征 測(cè)試標(biāo)號(hào)
test_set = (
mnist.read_image_file(os.path.join(root, 't10k-images-idx3-ubyte')),
mnist.read_label_file(os.path.join(root, 't10k-labels-idx1-ubyte'))
)
# 打印出有多少訓(xùn)練數(shù)據(jù) 多少測(cè)試數(shù)據(jù)
print("training set :", train_set[0].size())
print("test set :", test_set[0].size())
# 從原始數(shù)據(jù) 到 jpg圖片 順便裝到文件夾里面
def convert_to_img(train=True):
if train: # 如果是訓(xùn)練數(shù)據(jù)
# 注意這里路徑的寫法,對(duì)就是/符號(hào)
f = open(root+'train.txt', 'w')
data_path = root+'/train/' # 好像可以刪去左邊的/
# 如果不存在就新建 F:/!BiliBili/!Py/AI/cnn_bili/mnist_data//train/
if not os.path.exists(data_path):
os.makedirs(data_path)
# enumerate將可遍歷對(duì)象 組合成索引 可加參數(shù)start=2 索引從2開始
for i, (img, label) in enumerate(zip(train_set[0], train_set[1])):
img_path = data_path+str(i)+'.jpg'
# 保存圖片
io.imsave(img_path, img.numpy())
# 保存標(biāo)號(hào)文件路徑和標(biāo)號(hào)
f.write(img_path+' '+str(label)+'\n')
f.close()
else: # 如果是測(cè)試數(shù)據(jù)
f = open(root + 'test.txt', 'w')
data_path = root + '/test/'
if not os.path.exists(data_path):
os.makedirs(data_path)
for i, (img, label) in enumerate(zip(test_set[0], test_set[1])):
img_path = data_path + str(i) + '.jpg'
io.imsave(img_path, img.numpy())
f.write(img_path + ' ' + str(label) + '\n')
f.close()
print("Building training set...")
convert_to_img(True)
print("Building test set...")
convert_to_img(False)
read_cnn.py
import torch
from torch.autograd import Variable
from torchvision import transforms
from torch.utils.data import Dataset, DataLoader
from PIL import Image
root = "F:/!BiliBili/!Py/AI/cnn_bili/mnist_data/"
# -----------------準(zhǔn)備數(shù)據(jù)--------------------------
def default_loader(path):
return Image.open(path).convert('RGB')
class MyDataset(Dataset):
# txt是路徑和文件名
def __init__(self, txt, transform=transforms.ToTensor(), target_transform=None, loader=default_loader):
fh = open(txt, 'r') # 只讀打開
imgs = []
for line in fh:
line = line.strip('\n') # 刪除 回車
line = line.rstrip() # 刪除 右側(cè) 空格
words = line.split() # 分割:就兩列,0列是路徑 1列是標(biāo)號(hào)
imgs.append((words[0], int(words[1])))
self.imgs = imgs
self.transform = transform
self.target_transform = target_transform
self.loader = loader # 是個(gè)函數(shù)
# train_loader里面的
def __getitem__(self, index):
fn, label = self.imgs[index] # fn是完整路徑 label是標(biāo)號(hào)
img = self.loader(fn) # 調(diào)用上面的default_loader(path) 按照路徑讀取圖片
if self.transform is not None:
img = self.transform(img) # 將圖片轉(zhuǎn)換成FloatTensor類型
return img, label
def __len__(self):
return len(self.imgs)
# -----------------創(chuàng)建網(wǎng)絡(luò)并訓(xùn)練------------------------
class Net(torch.nn.Module):
def __init__(self):
super(Net, self).__init__()
self.conv1 = torch.nn.Sequential(
# 3通道進(jìn) 32出 卷積核大小為3*3 卷積核步長(zhǎng)1*1 (其實(shí)輸入是黑白圖)
# (self, in_channels, out_channels, kernel_size, stride=1, padding=0, dilation=1, groups=1, bias=True)
torch.nn.Conv2d(3, 32, kernel_size=(3, 3), stride=(1, 1)),
# 激活函數(shù)
# (self, inplace=False)
torch.nn.ReLU(),
# 2*2方形最大值 降(下)采樣(池化) 步長(zhǎng)2*2 膨脹1*1(不膨脹)
# (self, kernel_size, stride=None, padding=0, dilation=1, return_indices=False, ceil_mode=False)
torch.nn.MaxPool2d(kernel_size=(2, 2), stride=(2, 2), dilation=(1, 1)) # dilation膨脹
)
self.conv2 = torch.nn.Sequential(
torch.nn.Conv2d(32, 64, 3, 1, 1),
torch.nn.ReLU(),
torch.nn.MaxPool2d(2)
)
self.conv3 = torch.nn.Sequential(
torch.nn.Conv2d(64, 64, 3, 1, 1),
torch.nn.ReLU(),
torch.nn.MaxPool2d(2)
)
self.dense = torch.nn.Sequential(
# 線性分類器 64*3*3的輸入 128的輸出
torch.nn.Linear(64 * 3 * 3, 128),
torch.nn.ReLU(),
# 線性分類器 128的輸入 10的輸出
torch.nn.Linear(128, 10)
)
# 前向計(jì)算 輸入x
def forward(self, x):
# 第一層的輸出是x經(jīng)過conv1的結(jié)果
conv1_out = self.conv1(x)
# 第二層的輸出是 第一層的輸出經(jīng)過conv2的結(jié)果
conv2_out = self.conv2(conv1_out)
# 第三層的輸出是 第二層的輸出經(jīng)過conv3的結(jié)果
conv3_out = self.conv3(conv2_out)
res = conv3_out.view(conv3_out.size(0), -1)
return self.dense(res) # dense稠密
def read_cnn():
print("Reading train_data...")
train_data = MyDataset(txt=root + 'train.txt', transform=transforms.ToTensor())
# from torch.utils.data import Dataset, DataLoader 下面的函數(shù)在這里
train_loader = DataLoader(dataset=train_data, batch_size=50, shuffle=True)
print("Reading test_data...")
test_data = MyDataset(txt=root + 'test.txt', transform=transforms.ToTensor())
test_loader = DataLoader(dataset=test_data, batch_size=50)
# GPU or CPU
if torch.cuda.is_available():
is_cuda = True
print("work on GPU")
else:
is_cuda = False
print("work on CPU")
print("Setup Net...")
# =============================cuda()=======================
if is_cuda:
model = Net().cuda()
else:
model = Net()
# 打印網(wǎng)絡(luò)結(jié)構(gòu)
print(model)
# Adam 一種隨機(jī)優(yōu)化方法
optimizer = torch.optim.Adam(model.parameters())
# 一種優(yōu)化多類分類不平衡訓(xùn)練集的方法
loss_func = torch.nn.CrossEntropyLoss()
for epoch in range(3): # 訓(xùn)練幾次停止
print('epoch {}'.format(epoch + 1))
# 訓(xùn)練-----------------------------
train_loss = 0.
train_acc = 0.
for batch_x, batch_y in train_loader: # 特征 標(biāo)號(hào)
# =============================cuda()=======================
if is_cuda:
batch_x, batch_y = Variable(batch_x).cuda(), Variable(batch_y).cuda()
else:
batch_x, batch_y = Variable(batch_x), Variable(batch_y)
out = model(batch_x) # batch_x通過網(wǎng)絡(luò)的結(jié)果是out
loss = loss_func(out, batch_y) # 網(wǎng)絡(luò)結(jié)果out和實(shí)際batch_y對(duì)比的得到損失
train_loss += loss.data[0] # 累加訓(xùn)練損失
# =============================cuda()=======================
if is_cuda:
pred = torch.max(out, 1)[1].cuda() # 取 out和1 的最大值?
else:
pred = torch.max(out, 1)[1] # 取 out和1 的最大值?
train_correct = (pred == batch_y).sum() # 多少個(gè)對(duì)的
train_acc += train_correct.data[0] # 累加訓(xùn)練正確的數(shù)量?
optimizer.zero_grad() # 清除所有優(yōu)化的grad
loss.backward() # 誤差反向傳遞
optimizer.step() # 單次優(yōu)化
# 數(shù)據(jù)量大的時(shí)候小數(shù)點(diǎn)后6位可能不夠
# print('Train Loss: {:.6f}, Acc: {:.6f}'.format(train_loss / (len(train_data)), train_acc / (len(train_data))))
print('Train Acc: {:.6f}'.format(train_acc / (len(train_data))))
# 測(cè)試評(píng)估--------------------------------
model.eval() # 將網(wǎng)絡(luò)設(shè)置到測(cè)試評(píng)估模式,會(huì)影響網(wǎng)絡(luò)的Dropout和BatchNorm
eval_loss = 0.
eval_acc = 0.
for batch_x, batch_y in test_loader: # 特征 標(biāo)號(hào)
# =============================cuda()=======================
if is_cuda:
batch_x, batch_y = Variable(batch_x, volatile=True).cuda(), Variable(batch_y, volatile=True).cuda()
else:
batch_x, batch_y = Variable(batch_x, volatile=True), Variable(batch_y, volatile=True)
out = model(batch_x)
loss = loss_func(out, batch_y)
eval_loss += loss.data[0]
# =============================cuda()=======================
if is_cuda:
pred = torch.max(out, 1)[1].cuda()
else:
pred = torch.max(out, 1)[1]
num_correct = (pred == batch_y).sum()
eval_acc += num_correct.data[0]
# print('Test Loss: {:.6f}, Acc: {:.6f}'.format(eval_loss / (len(test_data)), eval_acc / (len(test_data))))
print('Test Acc: {:.6f}'.format(eval_acc / (len(test_data))))
if __name__ == '__main__':
read_cnn()
本文說實(shí)話不適合小白來讀,應(yīng)該是了解了相關(guān)知識(shí)的人才能夠從中獲得一些幫助吧
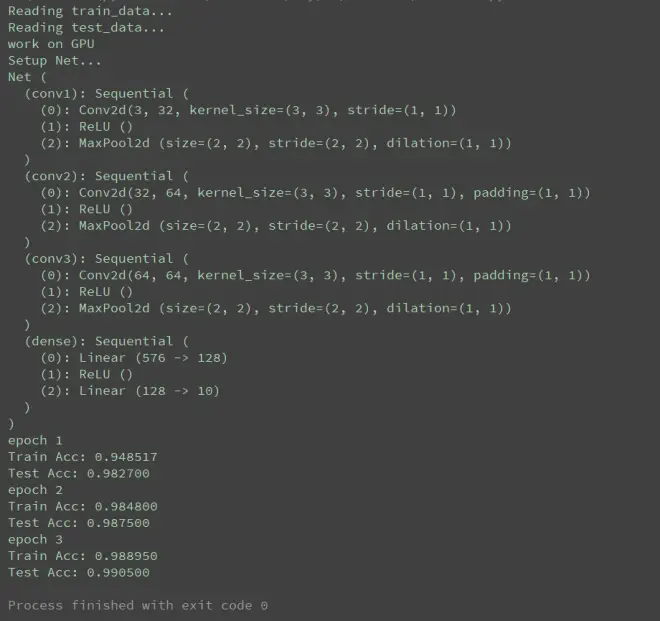