邏輯回歸-逐步回歸(stepwise regression)的一些思考
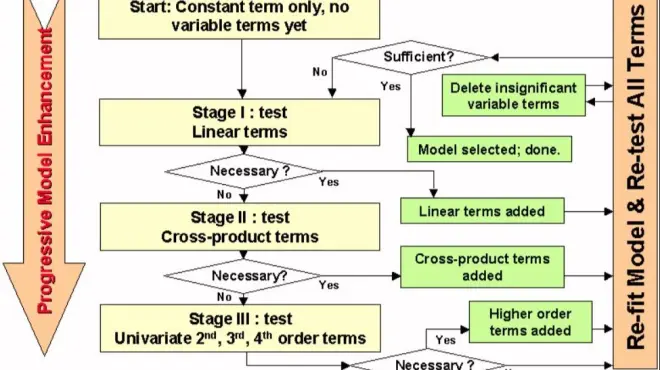
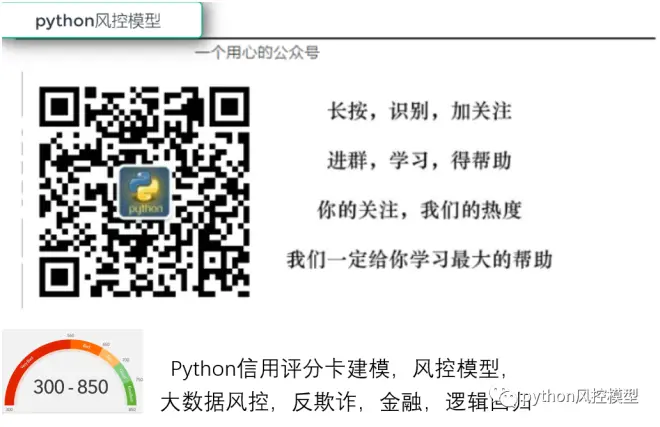
在數(shù)據(jù)挖掘中,我們經(jīng)常用到邏輯回歸算法。逐步回歸又是篩選變量的一個自動化算法,被諸多大學(xué)教授講述。我在機器學(xué)習(xí)項目中累計經(jīng)驗說明逐步回歸有時是有用的,特別是存在較多相關(guān)性高的變量時,逐步回歸可以很好降低模型維度,降低邏輯回歸模型多重共線性。當然逐步回歸不是完全消除模型多重共線性,而是很好改善情況,多重共線性是很難完全消除的。
下圖是對乳腺癌數(shù)據(jù)集的逐步回歸項目,模型維度降低一半,模型性能反而略有提高。這說明逐步回歸是有效的。
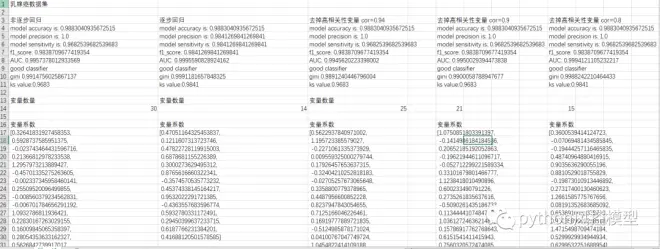
當變量相關(guān)性不高情況下,我認為可以不用逐步回歸,用了后模型性能反而下降。下圖是give me some credit數(shù)據(jù)集測試,逐步回歸后模型性能反而略有下降。
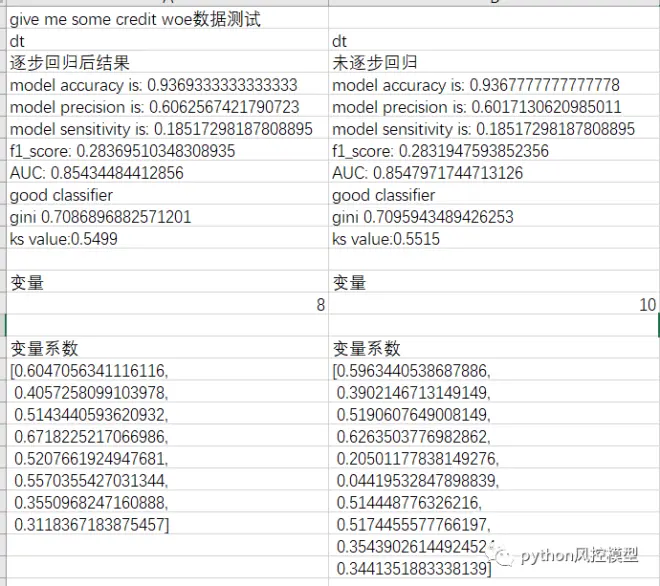
我今天看了國內(nèi)某知名大學(xué)教授講述逐步回歸視頻,案例是青島市財政收入分析。他把很多自己觀念強行和逐步回歸結(jié)果聯(lián)系起來。此教授過于強調(diào)GDP在經(jīng)濟中作用,我認為是不可取的。他認可制造業(yè)和工業(yè)這點我是贊同的。經(jīng)濟是一個非常復(fù)雜模型,變量之間存在復(fù)雜交互關(guān)系,我認為他光用逐步回歸來解釋是不全面的。
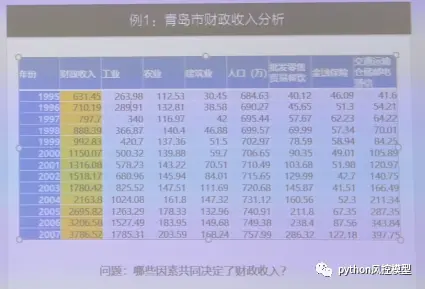
我認為逐步回歸是一種變量篩選方法,但不能神話逐步回歸。逐步回歸還是有爭議的。變量自動化篩選過程始終用的是同樣數(shù)據(jù)集,這容易過渡擬合。逐步回歸容易導(dǎo)致排除有價值變量,造成模型過于簡單。其它爭議還有很多,不一一闡述。
還是那句話,逐步回歸是一種方法,只要能降低模型維度,得到滿意模型性能,變量能夠被業(yè)務(wù)方所解釋,就是可以用的,但不能神話它和夸大它的作用。
機器學(xué)習(xí)是一門嚴謹學(xué)科,希望各位同學(xué)今后使用時要謹慎對待,要全面了解一種算法的利和弊以及什么時候可以使用。
最后附上一些逐步回歸英文的解釋
Criticism
Stepwise regression procedures are used in data mining, but are controversial. Several points of criticism have been made.
The tests themselves are biased, since they are based on the same data.Wilkinson and Dallal (1981)computed percentage points of the multiple correlation coefficient by simulation and showed that a final regression obtained by forward selection, said by the F-procedure to be significant at 0.1%, was in fact only significant at 5%.
When estimating the degrees of freedom, the number of the candidate independent variables from the best fit selected may be smaller than the total number of final model variables, causing the fit to appear better than it is when adjusting the r2 value for the number of degrees of freedom. It is important to consider how many degrees of freedom have been used in the entire model, not just count the number of independent variables in the resulting fit.
Models that are created may be over-simplifications of the real models of the data.
Such criticisms, based upon limitations of the relationship between a model and procedure and data set used to fit it, are usually addressed by verifying the model on an independent data set, as in the PRESS procedure.
Critics regard the procedure as a paradigmatic example of data dredging, intense computation often being an inadequate substitute for subject area expertise. Additionally, the results of stepwise regression are often used incorrectly without adjusting them for the occurrence of model selection. Especially the practice of fitting the final selected model as if no model selection had taken place and reporting of estimates and confidence intervals as if least-squares theory were valid for them, has been described as a scandal.Widespread incorrect usage and the availability of alternatives such as ensemble learning, leaving all variables in the model, or using expert judgement to identify relevant variables have led to calls to totally avoid stepwise model selection.
參考資料
1.《python機器學(xué)習(xí)-乳腺癌細胞挖掘》
2.《python信用評分卡建模(附代碼)》
