Prompt Engineering 面面觀
作者:紫氣東來(lái)
項(xiàng)目地址:https://zhuanlan.zhihu.com/p/632369186
指令:想要模型執(zhí)行的特定任務(wù)或指令。
上下文:包含外部信息或額外的上下文信息,引導(dǎo)語(yǔ)言模型更好地響應(yīng)。
輸入數(shù)據(jù):用戶輸入的內(nèi)容或問(wèn)題。
輸出指示:指定輸出的類型或格式。
從簡(jiǎn)單開始:在設(shè)計(jì)提示時(shí),需要記住這是一個(gè)迭代的過(guò)程,需要大量的實(shí)驗(yàn)來(lái)獲得最佳結(jié)果??梢詮暮?jiǎn)單的提示開始,不斷添加更多的元素和上下文,以獲得更好的結(jié)果。
指令:可以使用命令來(lái)指示模型執(zhí)行各種簡(jiǎn)單任務(wù),例如“寫入”、“分類”、“總結(jié)”、“翻譯”、“排序”等,從而為各種簡(jiǎn)單任務(wù)設(shè)計(jì)有效的提示。
具體性:對(duì)希望模型執(zhí)行的指令和任務(wù),提示越具體和詳細(xì),結(jié)果就越好。實(shí)際上,在提示中提供示例非常有效,可以以特定格式獲得所需的輸出。
避免不精確:這里的類比非常類似于有效的溝通——越直接,信息傳遞就越有效。
做還是不做:設(shè)計(jì)提示時(shí)的另一個(gè)常見(jiàn)技巧是避免說(shuō)不要做什么,而是說(shuō)要做什么。
使用迭代蒙特卡洛搜索方法通過(guò)提示(如:Generate a variation of the following instruction while keeping the semantic meaning.\n\nInput: ...\n\nOutput:...)來(lái)提出語(yǔ)義相似的變體來(lái)改進(jìn)最佳候選者。
增強(qiáng)(Augment):使用 Few-shot 或 Zero-shot CoT 提示生成給定問(wèn)題的多個(gè)偽思維鏈;
修剪(Prune):根據(jù)生成的答案是否與基本事實(shí)相匹配來(lái)修剪偽鏈。
選擇(Select):應(yīng)用減少方差的策略梯度策略來(lái)學(xué)習(xí)所選示例的概率分布,同時(shí)將示例的概率分布視為策略,將驗(yàn)證集的準(zhǔn)確性視為獎(jiǎng)勵(lì)。
問(wèn)題聚類(Question clustering):Embed 問(wèn)題使用 k-means 的方法進(jìn)行聚類。
示例選擇(Demonstration selection):從每個(gè)集群中選擇一組有代表性的問(wèn)題; 即來(lái)自一個(gè)集群的一個(gè)示例。 每個(gè)簇中的樣本按到簇質(zhì)心的距離排序,最接近質(zhì)心的樣本首先被選擇。
論據(jù)生成(Rationale generation):使用 Zero-shot CoT 為選定的問(wèn)題生成推理鏈,并構(gòu)建 Few-shot 提示以運(yùn)行推理。
OpenAI Cookbook has many in-depth examples for how to utilize LLM efficiently.
LangChain, a library for combining language models with other components to build applications.
Prompt Engineering Guide repo contains a pretty comprehensive collection of education materials on prompt engineering.
learnprompting.org
PromptPerfect
Semantic Kernel
Anthropic's Red Team dataset(opens in a new tab),(論文)(opens in a new tab)
Awesome ChatGPT Prompts(opens in a new tab)
DiffusionDB(opens in a new tab)
Midjourney Prompts(opens in a new tab)
P3 - Public Pool of Prompts(opens in a new tab)
PartiPrompts(opens in a new tab)
Real Toxicity Prompts(opens in a new tab)
Stable Diffusion Dataset(opens in a new tab)
WritingPrompts(opens in a new tab)
Nature Language Reasoning, A Survey(opens in a new tab) (March 2023)
Augmented Language Models: a Survey(opens in a new tab) (Feb 2023)
A Survey for In-context Learning(opens in a new tab) (Dec 2022)
Towards Reasoning in Large Language Models: A Survey(opens in a new tab) (Dec 2022)
Reasoning with Language Model Prompting: A Survey(opens in a new tab) (Dec 2022)
Emergent Abilities of Large Language Models(opens in a new tab) (Jun 2022)
A Taxonomy of Prompt Modifiers for Text-To-Image Generation(opens in a new tab) (Apr 2022)
Pre-train, Prompt, and Predict: A Systematic Survey of Prompting Methods in Natural Language Processing(opens in a new tab) (Jul 2021)
Self-Refine: Iterative Refinement with Self-Feedback(opens in a new tab) (Mar 2023)
kNN Prompting: Beyond-Context Learning with Calibration-Free Nearest Neighbor Inference(opens in a new tab) (Mar 2023)
Visual-Language Prompt Tuning with Knowledge-guided Context Optimization(opens in a new tab) (Mar 2023)
Fairness-guided Few-shot Prompting for Large Language Models(opens in a new tab) (Mar 2023)
Context-faithful Prompting for Large Language Models(opens in a new tab) (Mar 2023)
Is Prompt All You Need? No. A Comprehensive and Broader View of Instruction Learning(opens in a new tab) (Mar 2023)
UPRISE: Universal Prompt Retrieval for Improving Zero-Shot Evaluation(opens in a new tab) (Mar 2023)
Model-tuning Via Prompts Makes NLP Models Adversarially Robust(opens in a new tab) (Mar 2023)
Structure Pretraining and Prompt Tuning for Knowledge Graph Transfer(opens in a new tab) (March 2023)
CoTEVer: Chain of Thought Prompting Annotation Toolkit for Explanation Verification(opens in a new tab) (March 2023)
Larger language models do in-context learning differently(opens in a new tab) (March 2023)
OpenICL: An Open-Source Framework for In-context Learning(opens in a new tab) (March 2023)
Dynamic Prompting: A Unified Framework for Prompt Tuning(opens in a new tab) (March 2023)
Multitask Prompt Tuning Enables Parameter-Efficient Transfer Learning(opens in a new tab) (March 2023)
Effectiveness of Data Augmentation for Prefix Tuning with Limited Data(opens in a new tab) (March 2023)
Mixture of Soft Prompts for Controllable Data Generation(opens in a new tab) (March 2023)
Prompt, Generate, then Cache: Cascade of Foundation Models makes Strong Few-shot Learners(opens in a new tab) (March 2023)
How Robust is GPT-3.5 to Predecessors? A Comprehensive Study on Language Understanding Tasks(opens in a new tab) (March 2023)
Can ChatGPT Understand Too? A Comparative Study on ChatGPT and Fine-tuned BERT(opens in a new tab) (Feb 2023)
EvoPrompting: Language Models for Code-Level Neural Architecture Search(opens in a new tab) (Feb 2023)
In-Context Instruction Learning(opens in a new tab) (Feb 2023)
Chain of Hindsight Aligns Language Models with Feedback(opens in a new tab) (Feb 2023)
Language Is Not All You Need: Aligning Perception with Language Models(opens in a new tab) (Feb 2023)
Automatic Prompt Augmentation and Selection with Chain-of-Thought from Labeled Data(opens in a new tab) (Feb 2023)
Active Prompting with Chain-of-Thought for Large Language Models(opens in a new tab) (Feb 2023)
More than you've asked for: A Comprehensive Analysis of Novel Prompt Injection Threats to Application-Integrated Large Language Models(opens in a new tab) (Feb 2023)
A Prompt Pattern Catalog to Enhance Prompt Engineering with ChatGPT(opens in a new tab) (Feb 2023)
Guiding Large Language Models via Directional Stimulus Prompting(opens in a new tab) (Feb 2023)
How Does In-Context Learning Help Prompt Tuning?(opens in a new tab) (Feb 2023)
Scalable Prompt Generation for Semi-supervised Learning with Language Models(opens in a new tab) (Feb 2023)
Bounding the Capabilities of Large Language Models in Open Text Generation with Prompt Constraints(opens in a new tab) (Feb 2023)
à-la-carte Prompt Tuning (APT): Combining Distinct Data Via Composable Prompting(opens in a new tab) (Feb 2023)
GraphPrompt: Unifying Pre-Training and Downstream Tasks for Graph Neural Networks(opens in a new tab) (Feb 2023)
The Capacity for Moral Self-Correction in Large Language Models(opens in a new tab) (Feb 2023)
SwitchPrompt: Learning Domain-Specific Gated Soft Prompts for Classification in Low-Resource Domains(opens in a new tab) (Feb 2023)
Evaluating the Robustness of Discrete Prompts(opens in a new tab) (Feb 2023)
Compositional Exemplars for In-context Learning(opens in a new tab) (Feb 2023)
Hard Prompts Made Easy: Gradient-Based Discrete Optimization for Prompt Tuning and Discovery(opens in a new tab) (Feb 2023)
Multimodal Chain-of-Thought Reasoning in Language Models(opens in a new tab) (Feb 2023)
Large Language Models Can Be Easily Distracted by Irrelevant Context(opens in a new tab) (Feb 2023)
Synthetic Prompting: Generating Chain-of-Thought Demonstrations for Large Language Models(opens in a new tab) (Feb 2023)
Progressive Prompts: Continual Learning for Language Models(opens in a new tab) (Jan 2023)
Batch Prompting: Efficient Inference with LLM APIs(opens in a new tab) (Jan 2023)
Demonstrate-Search-Predict: Composing retrieval and language models for knowledge-intensive NLP(opens in a new tab) (Dec 2022)
On Second Thought, Let's Not Think Step by Step! Bias and Toxicity in Zero-Shot Reasoning(opens in a new tab) (Dec 2022)
Constitutional AI: Harmlessness from AI Feedback(opens in a new tab) (Dec 2022)
Successive Prompting for Decomposing Complex Questions(opens in a new tab) (Dec 2022)
Large Language Models are reasoners with Self-Verification(opens in a new tab) (Dec 2022)
Discovering Language Model Behaviors with Model-Written Evaluations(opens in a new tab) (Dec 2022)
Structured Prompting: Scaling In-Context Learning to 1,000 Examples(opens in a new tab) (Dec 2022)
PAL: Program-aided Language Models(opens in a new tab) (Nov 2022)
Large Language Models Are Human-Level Prompt Engineers(opens in a new tab) (Nov 2022)
Ignore Previous Prompt: Attack Techniques For Language Models(opens in a new tab) (Nov 2022)
Machine Generated Text: A Comprehensive Survey of Threat Models and Detection Methods(opens in a new tab) (Nov 2022)
Teaching Algorithmic Reasoning via In-context Learning(opens in a new tab) (Nov 2022)
Enhancing Self-Consistency and Performance of Pre-Trained Language Models through Natural Language Inference(opens in a new tab) (Nov 2022)
Ask Me Anything: A simple strategy for prompting language models(opens in a new tab) (Oct 2022)
Recitation-Augmented Language Models(opens in a new tab) (Oct 2022)
ReAct: Synergizing Reasoning and Acting in Language Models(opens in a new tab) (Oct 2022)
Prompting GPT-3 To Be Reliable(opens in a new tab) (Oct 2022)
Decomposed Prompting: A Modular Approach for Solving Complex Tasks(opens in a new tab) (Oct 2022)
Language Models Are Greedy Reasoners: A Systematic Formal Analysis of Chain-of-Thought(opens in a new tab) (Oct 2022)
Evaluating the Susceptibility of Pre-Trained Language Models via Handcrafted Adversarial Examples(opens in a new tab) (Sep 2022)
Dynamic Prompt Learning via Policy Gradient for Semi-structured Mathematical Reasoning(opens in a new tab) (Sep 2022)
Promptagator: Few-shot Dense Retrieval From 8 Examples(opens in a new tab) (Sep 2022)
Atlas: Few-shot Learning with Retrieval Augmented Language Models(opens in a new tab) (Nov 2022)
DocPrompting: Generating Code by Retrieving the Docs(opens in a new tab) (July 2022)
On the Advance of Making Language Models Better Reasoners(opens in a new tab) (June 2022)
Large Language Models are Zero-Shot Reasoners(opens in a new tab) (May 2022)
Maieutic Prompting: Logically Consistent Reasoning with Recursive Explanations(opens in a new tab) (May 2022)
MRKL Systems: A modular, neuro-symbolic architecture that combines large language models, external knowledge sources and discrete reasoning(opens in a new tab) (May 2022)
PPT: Pre-trained Prompt Tuning for Few-shot Learning(opens in a new tab) (Mqy 2022)
Toxicity Detection with Generative Prompt-based Inference(opens in a new tab) (May 2022)
Learning to Transfer Prompts for Text Generation(opens in a new tab) (May 2022)
The Unreliability of Explanations in Few-shot Prompting for Textual Reasoning(opens in a new tab) (May 2022)
A Taxonomy of Prompt Modifiers for Text-To-Image Generation(opens in a new tab) (Apr 2022)
PromptChainer: Chaining Large Language Model Prompts through Visual Programming(opens in a new tab) (Mar 2022)
Self-Consistency Improves Chain of Thought Reasoning in Language Models(opens in a new tab) (March 2022)
Training language models to follow instructions with human feedback(opens in a new tab)
Rethinking the Role of Demonstrations: What Makes In-Context Learning Work?(opens in a new tab) (Feb 2022)
Chain of Thought Prompting Elicits Reasoning in Large Language Models(opens in a new tab) (Jan 2022)
Show Your Work: Scratchpads for Intermediate Computation with Language Models(opens in a new tab) (Nov 2021)
AI Chains: Transparent and Controllable Human-AI Interaction by Chaining Large Language Model Prompts(opens in a new tab) (Oct 2021)
Generated Knowledge Prompting for Commonsense Reasoning(opens in a new tab) (Oct 2021)
Multitask Prompted Training Enables Zero-Shot Task Generalization(opens in a new tab) (Oct 2021)
Reframing Instructional Prompts to GPTk's Language(opens in a new tab) (Sep 2021)
Design Guidelines for Prompt Engineering Text-to-Image Generative Models(opens in a new tab) (Sep 2021)
Making Pre-trained Language Models Better Few-shot Learners(opens in a new tab) (Aug 2021)
Fantastically Ordered Prompts and Where to Find Them: Overcoming Few-Shot Prompt Order Sensitivity(opens in a new tab) (April 2021)
BERTese: Learning to Speak to BERT(opens in a new tab) (April 2021)
The Power of Scale for Parameter-Efficient Prompt Tuning(opens in a new tab) (April 2021)
Prompt Programming for Large Language Models: Beyond the Few-Shot Paradigm(opens in a new tab) (Feb 2021)
Calibrate Before Use: Improving Few-Shot Performance of Language Models(opens in a new tab) (Feb 2021)
Prefix-Tuning: Optimizing Continuous Prompts for Generation(opens in a new tab) (Jan 2021)
Learning to Generate Task-Specific Adapters from Task Description(opens in a new tab) (Jan 2021)
Making Pre-trained Language Models Better Few-shot Learners(opens in a new tab) (Dec 2020)
Learning from Task Descriptions(opens in a new tab) (Nov 2020)
AutoPrompt: Eliciting Knowledge from Language Models with Automatically Generated Prompts(opens in a new tab) (Oct 2020)
Language Models are Few-Shot Learners(opens in a new tab) (May 2020)
How Can We Know What Language Models Know?(opens in a new tab) (July 2020)
Scaling Laws for Neural Language Models(opens in a new tab) (Jan 2020)
PaLM 2 Technical Report(opens in a new tab) (May 2023)
BloombergGPT: A Large Language Model for Finance(opens in a new tab) (March 2023)
Medical Intervention Duration Estimation Using Language-enhanced Transformer Encoder with Medical Prompts(opens in a new tab) (March 2023)
Soft-prompt tuning to predict lung cancer using primary care free-text Dutch medical notes(opens in a new tab) (March 2023)
TaskMatrix.AI: Completing Tasks by Connecting Foundation Models with Millions of APIs(opens in a new tab) (March 2023)
Larger Probes Tell a Different Story: Extending Psycholinguistic Datasets Via In-Context Learning(opens in a new tab) (March 2023)
Linguistically Informed ChatGPT Prompts to Enhance Japanese-Chinese Machine Translation: A Case Study on Attributive Clauses(opens in a new tab) (March 2023)
Knowledge-augmented Frame Semantic Parsing with Hybrid Prompt-tuning(opens in a new tab) (March 2023)
Debiasing Scores and Prompts of 2D Diffusion for Robust Text-to-3D Generation(opens in a new tab) (March 2023)
Zero-shot Model Diagnosis(opens in a new tab) (March 2023)
Prompting Large Language Models to Generate Code-Mixed Texts: The Case of South East Asian Languages(opens in a new tab) (March 2023)
SPeC: A Soft Prompt-Based Calibration on Mitigating Performance Variability in Clinical Notes Summarization(opens in a new tab) (March 2023)
Large Language Models and Simple, Stupid Bugs(opens in a new tab) (March 2023)
Can Generative Pre-trained Transformers (GPT) Pass Assessments in Higher Education Programming Courses?(opens in a new tab) (Mar 2023)
SelfCheckGPT: Zero-Resource Black-Box Hallucination Detection for Generative Large Language Models(opens in a new tab) (Mar 2023)
ICL-D3IE: In-Context Learning with Diverse Demonstrations Updating for Document Information Extraction(opens in a new tab) (March 2023)
MathPrompter: Mathematical Reasoning using Large Language Models(opens in a new tab) (March 2023)
Prompt-Based Learning for Thread Structure Prediction in Cybersecurity Forums(opens in a new tab) (March 2023)
Choice Over Control: How Users Write with Large Language Models using Diegetic and Non-Diegetic Prompting(opens in a new tab) (March 2023)
Prompting Large Language Models with Answer Heuristics for Knowledge-based Visual Question Answering(opens in a new tab) (March 2023)
Soft Prompt Guided Joint Learning for Cross-Domain Sentiment Analysis(opens in a new tab) (March 2023)
SpeechPrompt v2: Prompt Tuning for Speech Classification Tasks(opens in a new tab) (March 2023)
Goal Driven Discovery of Distributional Differences via Language Descriptions(opens in a new tab) (Feb 2023)
Navigating the Grey Area: Expressions of Overconfidence and Uncertainty in Language Models(opens in a new tab) (Feb 2023)
TabGenie: A Toolkit for Table-to-Text Generation(opens in a new tab) (Feb 2023)
SGL-PT: A Strong Graph Learner with Graph Prompt Tuning(opens in a new tab) (Feb 2023)
Few-Shot Table-to-Text Generation with Prompt-based Adapter(opens in a new tab) (Feb 2023)
Language Models Are Few-shot Learners for Prognostic Prediction(opens in a new tab) (Feb 2023)
STA: Self-controlled Text Augmentation for Improving Text Classifications(opens in a new tab) (Feb 2023)
Check Your Facts and Try Again: Improving Large Language Models with External Knowledge and Automated Feedback(opens in a new tab) (Feb 2023)
How Generative AI models such as ChatGPT can be (Mis)Used in SPC Practice, Education, and Research? An Exploratory Study(opens in a new tab) (Feb 2023)
Grimm in Wonderland: Prompt Engineering with Midjourney to Illustrate Fairytales(opens in a new tab) (Feb 2023)
LabelPrompt: Effective Prompt-based Learning for Relation Classification(opens in a new tab) (Feb 2023)
Language Model Crossover: Variation through Few-Shot Prompting(opens in a new tab) (Feb 2023)
Prompt Tuning of Deep Neural Networks for Speaker-adaptive Visual Speech Recognition(opens in a new tab) (Feb 2023)
The Capacity for Moral Self-Correction in Large Language Models(opens in a new tab) (Feb 2023)
Prompting for Multimodal Hateful Meme Classification(opens in a new tab) (Feb 2023)
PLACES: Prompting Language Models for Social Conversation Synthesis(opens in a new tab) (Feb 2023)
Commonsense-Aware Prompting for Controllable Empathetic Dialogue Generation(opens in a new tab) (Feb 2023)
Crawling the Internal Knowledge-Base of Language Models(opens in a new tab) (Jan 2023)
Legal Prompt Engineering for Multilingual Legal Judgement Prediction(opens in a new tab) (Dec 2022)
Investigating Prompt Engineering in Diffusion Models(opens in a new tab) (Nov 2022)
Learn to Explain: Multimodal Reasoning via Thought Chains for Science Question Answering(opens in a new tab) (Sep 2022)
Conversing with Copilot: Exploring Prompt Engineering for Solving CS1 Problems Using Natural Language(opens in a new tab) (Oct 2022)
Piloting Copilot and Codex: Hot Temperature, Cold Prompts, or Black Magic?(opens in a new tab) (Oct 2022)
Plot Writing From Scratch Pre-Trained Language Models(opens in a new tab) (July 2022)
Survey of Hallucination in Natural Language Generation(opens in a new tab) (Feb 2022)
Chain-of-Thought Papers(opens in a new tab)
Papers with Code(opens in a new tab)
Prompt Papers
[1] Prompt Engineering https://lilianweng.github.io/posts/2023-03-15-prompt-engineering/#chain-of-thought-cot
[2] Prompt Engineering Guide https://www.promptingguide.ai/zh
[3] https://platform.openai.com/playgroundyground
[4] [2201.11903] Chain-of-Thought Prompting Elicits Reasoning in Large Language Models (arxiv.org)
[5] [2211.01910] Large Language Models Are Human-Level Prompt Engineers (arxiv.org)
[6] Automatic Prompt Augmentation and Selection with Chain-of-Thought from Labeled Data
一、概述
提示工程(Prompt Engineering),也稱為 In-Context Prompting,是指在不更新模型權(quán)重的情況下如何與 LLM 交互以引導(dǎo)其行為以獲得所需結(jié)果的方法。 在提示工程中,任務(wù)的描述會(huì)被嵌入到輸入中。例如,不是隱含地給予模型一定的參數(shù),而是以問(wèn)題的形式直接輸入。 提示工程的典型工作方式是將一個(gè)或多個(gè)任務(wù)轉(zhuǎn)換為基于提示的數(shù)據(jù)集,并通過(guò)所謂的“基于提示的學(xué)習(xí)(prompt-based learning)”來(lái)訓(xùn)練語(yǔ)言模型。
提示工程不僅僅是關(guān)于設(shè)計(jì)和研發(fā)提示詞。它包含了與大語(yǔ)言模型交互和研發(fā)的各種技能和技術(shù)。提示工程在實(shí)現(xiàn)和大語(yǔ)言模型交互、對(duì)接,以及理解大語(yǔ)言模型能力方面都起著重要作用。用戶可以通過(guò)提示工程來(lái)提高大語(yǔ)言模型的安全性,也可以賦能大語(yǔ)言模型,比如借助專業(yè)領(lǐng)域知識(shí)和外部工具來(lái)增強(qiáng)大語(yǔ)言模型能力。
提示詞可以包含以下任意要素:
以下是設(shè)計(jì)提示的通用技巧:
二、提示技術(shù)
時(shí)至今日,改進(jìn)提示顯然有助于在不同任務(wù)上獲得更好的結(jié)果。這就是提示工程背后的整個(gè)理念。在本節(jié)中,我們將介紹更高級(jí)的提示工程技術(shù),使我們能夠完成更復(fù)雜和有趣的任務(wù),所有測(cè)試案例均通過(guò)text-davinci-003 得到。
2.1 Zero-shot 與 Few-shot
Zero-shot 與 Few-shot 是最基礎(chǔ)的提示技術(shù)。經(jīng)過(guò)大量數(shù)據(jù)訓(xùn)練并調(diào)整指令的LLM能夠執(zhí)行 Zero-shot 任務(wù),即直接向模型輸入文本以獲取回答。
如,Zero-shot 輸入:
輸出:
Few-shot learning 在目標(biāo)任務(wù)上提供了一組高質(zhì)量的演示,每個(gè)演示都包含輸入和期望的輸出。 當(dāng)模型首先看到好的例子時(shí),它可以更好地理解人類的意圖和需要什么樣的答案的標(biāo)準(zhǔn)。 因此,少樣本學(xué)習(xí)通常比零樣本學(xué)習(xí)有更好的性能。 然而,它是以更多的 token 消耗為代價(jià)的,并且當(dāng)輸入和輸出文本很長(zhǎng)時(shí)可能會(huì)達(dá)到上下文長(zhǎng)度限制。
如,F(xiàn)ew-shot 輸入:
輸出:
2.2 思維鏈(Chain-of-Thought, CoT)提示
CoT 提示 生成一系列短句來(lái)逐步描述推理邏輯,稱為推理鏈或基本原理,最終得出最終答案。 CoT 的好處對(duì)于復(fù)雜的推理任務(wù)更為,同時(shí)使用大型模型(例如,參數(shù)超過(guò) 50B)時(shí)效果更加明顯。
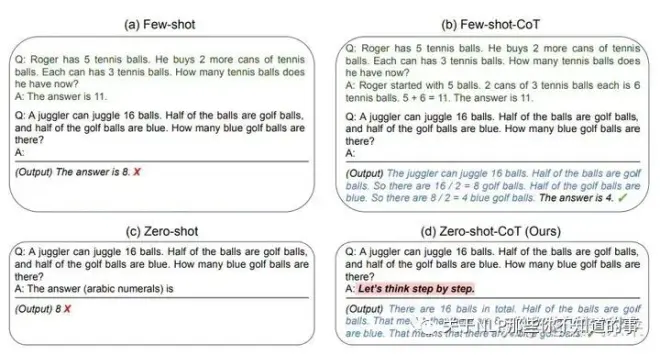
如,F(xiàn)ew-shot CoT 輸入:
輸出:
如,Zero-shot CoT 輸入:
輸出:
2.3 指令提示(Instruction Prompting)
Instructed LM(例如 InstructGPT)使用高質(zhì)量數(shù)據(jù)微調(diào)預(yù)訓(xùn)練模型,使 LM 更好地理解用戶意圖并遵循指令。 RLHF 是一種常用的方法。 instruction style fine-tuning 的好處是改進(jìn)了模型,使其更符合人類的意圖,并大大降低了溝通成本。
在與指令模型交互時(shí),我們應(yīng)該詳細(xì)描述任務(wù)要求,盡量具體和準(zhǔn)確,避免說(shuō)“不做某事”,而是具體說(shuō)明要做什么。如針對(duì)特定人群的輸入:
輸出:
In-context instruction learning 將小樣本學(xué)習(xí)與指令提示相結(jié)合。 它在提示中包含多個(gè)跨不同任務(wù)的演示示例,每個(gè)演示都由指令、任務(wù)輸入和輸出組成。 請(qǐng)注意,他們的實(shí)驗(yàn)僅針對(duì)分類任務(wù),指令提示包含所有標(biāo)簽選項(xiàng)。
如,In-context instruction learning 輸入:
輸出:
2.4 自我一致性(Self-Consistency)采樣
自我一致性旨在“替換鏈?zhǔn)剿季S提示中使用的天真貪婪解碼方法”。其想法是通過(guò)少樣本CoT采樣多個(gè)不同的推理路徑,并使用生成結(jié)果選擇最一致的答案。這有助于提高CoT提示在涉及算術(shù)和常識(shí)推理的任務(wù)中的性能。
嘗試進(jìn)行以下數(shù)學(xué)推理問(wèn)題:
When I was 6 my sister was half my age. Now I’m 70 how old is my sister?結(jié)果如下:
結(jié)果錯(cuò)誤,下面使用自我一致性來(lái)嘗試該問(wèn)題,輸入如下:
輸出1:
輸出2:
輸出3:
2.5 自動(dòng)提示工程師(Automatic Prompt Engineer, APE)
APE 是一種搜索模型生成的候選指令池,然后根據(jù)所選得分函數(shù)過(guò)濾候選集以最終選擇得分最高的最佳候選指令的方法。其過(guò)程可概括為3個(gè)階段:
提示 LLM 根據(jù)輸入輸出對(duì)形式的一小組演示生成候選指令。如:{{Given desired input-output pairs}}\n\nThe instruction is ;
為了構(gòu)造自動(dòng)戶 CoT 提示,Shum et al. (2023) 建議進(jìn)行剪枝選擇,包括以下3步:
Zhang et al. (2023) 認(rèn)為采用聚類技術(shù)對(duì)問(wèn)題進(jìn)行抽樣,然后生成鏈。 他們觀察到 LLM 傾向于犯某些類型的錯(cuò)誤。 一種類型的錯(cuò)誤在嵌入空間中可能相似,因此被組合在一起。 通過(guò)僅從頻繁錯(cuò)誤的集群中抽取一個(gè)或幾個(gè)樣本,我們可以防止對(duì)一種錯(cuò)誤類型的過(guò)多錯(cuò)誤演示,并收集一組不同的示例。
三、更多資料
3.1 實(shí)用工具
3.2 數(shù)據(jù)集
3.3 相關(guān)論文
綜述
方法
應(yīng)用
論文匯編
參考資料
關(guān)于NLP那些你不知道的事-VIP社群是高質(zhì)量圈子,無(wú)廣告營(yíng)銷及雜亂等無(wú)用內(nèi)容!只有一個(gè)人走得更快,一群人才能走得更遠(yuǎn),加入VIP社區(qū),不斷提高學(xué)習(xí)水平。
首先,因?yàn)閂IP社區(qū)(知識(shí)星球)付費(fèi)。所以我們必須對(duì)得起付費(fèi)用戶。努力編寫有價(jià)值的文章,這些文章比公眾號(hào)上的免費(fèi)材料和文件更全面、質(zhì)量更高。
AIGC的發(fā)展每日一個(gè)樣,如果不主動(dòng)學(xué)習(xí)起來(lái),不用等到35歲,估計(jì)掉隊(duì)只是分分鐘的事情,像ChatGPT、Stable diffusion等新技術(shù)是否了解,AIGC 新理念是否有接觸。
目前AIGC技術(shù)高速迭代,基本每天一個(gè) LLMs 或者新idea,因此需要我們時(shí)刻保持主動(dòng)學(xué)習(xí),帶有學(xué)習(xí)新技術(shù)新理念的心態(tài),我們星球也在持續(xù)加碼 AIGC 新理念新技術(shù)分享,像ChatGPT、Stable diffusion 等新理念/新技術(shù) 會(huì)找一些優(yōu)秀的學(xué)習(xí)資料分享在星球!讓星球的小伙伴努力跟上 AIGC 發(fā)展的腳步,不被時(shí)代所拋棄!