R語言進(jìn)行數(shù)值模擬:模擬泊松回歸模型的數(shù)據(jù)
原文鏈接:http://tecdat.cn/?p=6751
?
模擬回歸模型的數(shù)據(jù)
驗(yàn)證回歸模型的首選方法是模擬來自它們的數(shù)據(jù),并查看模擬數(shù)據(jù)是否捕獲原始數(shù)據(jù)的相關(guān)特征。感興趣的基本特征是平均值。我喜歡這種方法,因?yàn)樗梢詳U(kuò)展到廣義線性模型(logistic,Poisson,gamma,...)和其他回歸模型,比如t?-regression。
您的標(biāo)準(zhǔn)回歸模型假設(shè)存在將預(yù)測(cè)變量與結(jié)果相關(guān)聯(lián)的真實(shí)/固定參數(shù)。但是,當(dāng)我們執(zhí)行回歸時(shí),我們只估計(jì)這些參數(shù)。因此,回歸軟件返回表示系數(shù)不確定性的標(biāo)準(zhǔn)誤差。
我將用一個(gè)例子來證明我的意思。
示范
我將使用泊松回歸來證明這一點(diǎn)。我模擬了兩個(gè)預(yù)測(cè)變量,使用50的小樣本。
n <- 50
set.seed(18050518)
xc的系數(shù)為0.5?,xb的系數(shù)為1?。我對(duì)預(yù)測(cè)進(jìn)行取冪,并使用該
rpois()
函數(shù)生成泊松分布結(jié)果。
# Exponentiate prediction and pass to rpois()
summary(dat)
xc ? ? ? ? ? ? ? ? ?xb ? ? ? ? ? ? y
Min. ? :-2.903259 ? Min. ? :0.00 ? Min. ? :0.00
1st Qu.:-0.648742 ? 1st Qu.:0.00 ? 1st Qu.:1.00
Median :-0.011887 ? Median :0.00 ? Median :2.00
Mean ? : 0.006109 ? Mean ? :0.38 ? Mean ? :2.02
3rd Qu.: 0.808587 ? 3rd Qu.:1.00 ? 3rd Qu.:3.00
Max. ? : 2.513353 ? Max. ? :1.00 ? Max. ? :7.00
接下來是運(yùn)行模型。
Call:
glm(formula = y ~ xc + xb, family = poisson, data = dat)
Deviance Residuals:
Min ? ? ? 1Q ? Median ? ? ? 3Q ? ? ?Max
-1.9065 ?-0.9850 ?-0.1355 ? 0.5616 ? 2.4264
Coefficients:
Estimate Std. Error z value Pr(>|z|)
(Intercept) ?0.20839 ? ?0.15826 ? 1.317 ? ?0.188
xc ? ? ? ? ? 0.46166 ? ?0.09284 ? 4.973 6.61e-07 ***
xb ? ? ? ? ? 0.80954 ? ?0.20045 ? 4.039 5.38e-05 ***
---
Signif. codes: ?0 ‘***’ 0.001 ‘**’ 0.01 ‘*’ 0.05 ‘.’ 0.1 ‘ ’ 1
(Dispersion parameter for poisson family taken to be 1)
Null deviance: 91.087 ?on 49 ?degrees of freedom
Residual deviance: 52.552 ?on 47 ?degrees of freedom
AIC: 161.84
Number of Fisher Scoring iterations: 5
估計(jì)的系數(shù)與人口模型相距不太遠(yuǎn),.21代表截距而不是0,.46而不是.5,而0.81而不是1。
接下來模擬模型中的數(shù)據(jù),我想要10,000個(gè)模擬數(shù)據(jù)集,為了捕捉回歸系數(shù)的不確定性,我假設(shè)系數(shù)來自多元正態(tài)分布,估計(jì)系數(shù)作為均值,回歸系數(shù)的方差 - 協(xié)方差矩陣作為多元正態(tài)分布的方差 - 協(xié)方差矩陣。
coefs <- mvrnorm(n = 10000, mu = coefficients(fit.p), Sigma = vcov(fit.p))
檢查模擬系數(shù)與原始系數(shù)的匹配程度。
coefficients(fit.p)
(Intercept) ? ? ? ? ?xc ? ? ? ? ?xb
0.2083933 ? 0.4616605 ? 0.8095403
colMeans(coefs) # means of simulated coefficients
(Intercept) ? ? ? ? ?xc ? ? ? ? ?xb
0.2088947 ? 0.4624729 ? 0.8094507
標(biāo)準(zhǔn)錯(cuò)誤:
sqrt(diag(vcov(fit.p)))
(Intercept) ? ? ? ? ?xc ? ? ? ? ?xb
0.15825667 ?0.09284108 ?0.20044809
apply(coefs, 2, sd) # standard deviation of simulated coefficients
(Intercept) ? ? ? ? ?xc ? ? ? ? ?xb
0.16002806 ?0.09219235 ?0.20034148
?
下一步是模擬模型中的數(shù)據(jù)。我們通過將模擬系數(shù)的每一行乘以原始預(yù)測(cè)變量來實(shí)現(xiàn)。然后我們傳遞預(yù)測(cè):
# One row per case, one column per simulated set of coefficients
?# Cross product of model matrix by coefficients, exponentiate result,
# then use to simulate Poisson-distributed outcome
for (i in 1:nrow(coefs)) {
sim.dat[, i] <- rpois(n, exp(fit.p.mat %*% coefs[i ]))
}
rm(i, fit.p.mat) # Clean house
現(xiàn)在一個(gè)是完成模擬,將模擬數(shù)據(jù)集與原始數(shù)據(jù)集至少比較結(jié)果的均值和方差:
c(mean(dat$y), var(dat$y)) # Mean and variance of original outcome
[1] 2.020000 3.366939
c(mean(colMeans(sim.dat)), mean(apply(sim.dat, 2, var))) # average of mean and var of 10,000 simulated outcomes
[1] 2.050724 4.167751
模擬結(jié)果的平均值略高于原始數(shù)據(jù),平均方差更高。平均而言,可以預(yù)期方差比平均值更偏離目標(biāo)。方差也將與一些極高的值正偏差,同時(shí),它的界限為零,因此中位數(shù)可能更好地反映了數(shù)據(jù)的中心:
[1] 3.907143
中位數(shù)方差更接近原始結(jié)果的方差。
這是模擬均值和方差的分布:
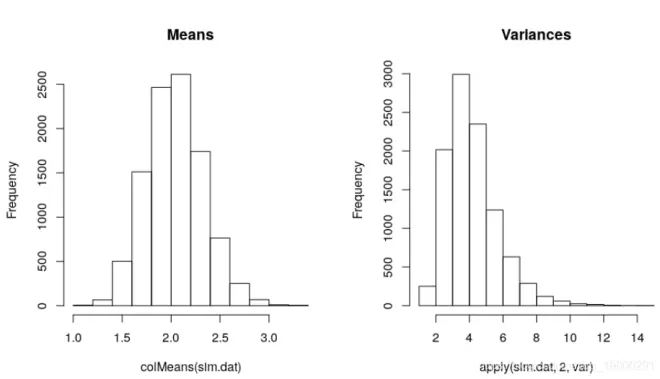
?
?繪制10,000個(gè)模擬數(shù)據(jù)集中的一些數(shù)據(jù)集的直方圖并將其與原始結(jié)果的直方圖進(jìn)行比較也是有用的。還可以測(cè)試原始數(shù)據(jù)和模擬數(shù)據(jù)集中xb = 1和xb = 0之間結(jié)果的平均差異。?
回到基礎(chǔ)R,它具有simulate()
執(zhí)行相同操作的功能:
sim.default <- simulate(fit.p, 10000)
此代碼相當(dāng)于:
sim.default <- replicate(10000, rpois(n, fitted(fit.p)))
fitted(fit.p)
是響應(yīng)尺度的預(yù)測(cè),或指數(shù)線性預(yù)測(cè)器,因?yàn)檫@是泊松回歸。因此,我們將使用模型中的單組預(yù)測(cè)值來重復(fù)創(chuàng)建模擬結(jié)果。
c(mean(colMeans(sim.default)), mean(apply(sim.default, 2, var)),
[1] 2.020036 3.931580 3.810612
與忽略系數(shù)不確定性時(shí)相比,均值和方差更接近原始結(jié)果的均值和方差。與考慮回歸系數(shù)的不確定性時(shí)相比,這種方法總是會(huì)導(dǎo)致方差較小。它要快得多,并且需要零編程來實(shí)現(xiàn),但我不習(xí)慣忽略回歸系數(shù)的不確定性,使模型看起來比它更充分。
?
非常感謝您閱讀本文,有任何問題請(qǐng)?jiān)谙旅媪粞裕?/h1>
?