【信號(hào)去噪】基于融合正余弦和柯西變異麻雀算法優(yōu)化變分模態(tài)分解SCSSA-VMD實(shí)現(xiàn)信號(hào)去
?作者簡介:熱愛科研的Matlab仿真開發(fā)者,修心和技術(shù)同步精進(jìn),
代碼獲取、論文復(fù)現(xiàn)及科研仿真合作可私信。
??個(gè)人主頁:Matlab科研工作室
??個(gè)人信條:格物致知。
更多Matlab完整代碼及仿真定制內(nèi)容點(diǎn)擊??
智能優(yōu)化算法?? ? ??神經(jīng)網(wǎng)絡(luò)預(yù)測?? ? ??雷達(dá)通信?? ? ?無線傳感器?? ? ? ?電力系統(tǒng)
信號(hào)處理?? ? ? ? ? ? ?圖像處理?? ? ? ? ? ? ??路徑規(guī)劃?? ? ??元胞自動(dòng)機(jī)?? ? ? ?無人機(jī)
?? 內(nèi)容介紹
信號(hào)去噪一直是數(shù)字信號(hào)處理領(lǐng)域的一個(gè)重要研究方向。在實(shí)際應(yīng)用中,由于信號(hào)受到各種干擾和噪聲的影響,需要對信號(hào)進(jìn)行去噪處理,以提高信號(hào)的質(zhì)量和可靠性。本文將介紹一種基于融合正余弦和柯西變異麻雀算法優(yōu)化變分模態(tài)分解SCSSA-VMD實(shí)現(xiàn)信號(hào)去噪的算法流程。
首先,介紹一下SCSSA-VMD算法。SCSSA-VMD是一種基于變分模態(tài)分解(VMD)的信號(hào)去噪算法,它可以將信號(hào)分解成一系列固有模態(tài)函數(shù)(IMF)。這些IMF是不同頻率的信號(hào)成分,可以通過對這些IMF進(jìn)行加權(quán)和來重建原始信號(hào)。SCSSA-VMD算法在VMD分解過程中加入了正余弦函數(shù)的約束,以增強(qiáng)分解的穩(wěn)定性和準(zhǔn)確性。
然后,介紹一下柯西變異麻雀算法??挛髯儺惵槿杆惴ㄊ且环N優(yōu)化算法,它基于柯西分布和變異策略,通過對候選解進(jìn)行隨機(jī)變異和選擇,來搜索最優(yōu)解??挛鞣植季哂虚L尾特性,可以避免算法陷入局部最優(yōu)解。變異策略可以保證算法的全局搜索能力。
接下來,介紹一下融合正余弦和柯西變異麻雀算法優(yōu)化SCSSA-VMD的算法流程。首先,將待處理的信號(hào)進(jìn)行SCSSA-VMD分解,得到一系列IMF。然后,將正余弦函數(shù)加入到VMD分解過程中,得到正余弦約束下的IMF。接著,將柯西變異麻雀算法應(yīng)用于IMF的加權(quán)和,以優(yōu)化重建信號(hào)的質(zhì)量和可靠性。最后,通過對優(yōu)化后的IMF進(jìn)行加權(quán)和,得到去噪后的信號(hào)。
總的來說,融合正余弦和柯西變異麻雀算法優(yōu)化SCSSA-VMD是一種有效的信號(hào)去噪算法。它不僅可以提高信號(hào)的質(zhì)量和可靠性,還可以保證算法的全局搜索能力和穩(wěn)定性。在實(shí)際應(yīng)用中,可以根據(jù)具體情況選擇不同的參數(shù)和算法流程,以達(dá)到最佳效果。
?? 部分代碼
function [u, u_hat, omega] = VMD(signal, alpha, tau, K, DC, init, tol)
%
%
% Input and Parameters:
% ---------------------
% signal ?- the time domain signal (1D) to be decomposed
% alpha ? - the balancing parameter of the data-fidelity constraint
% tau ? ? - time-step of the dual ascent ( pick 0 for noise-slack )
% K ? ? ? - the number of modes to be recovered
% DC ? ? ?- true if the first mode is put and kept at DC (0-freq)
% init ? ?- 0 = all omegas start at 0
% ? ? ? ? ? ? ? ? ? ?1 = all omegas start uniformly distributed
% ? ? ? ? ? ? ? ? ? ?2 = all omegas initialized randomly
% tol ? ? - tolerance of convergence criterion; typically around 1e-6
%
% Output:
% -------
% u ? ? ? - the collection of decomposed modes
% u_hat ? - spectra of the modes
% omega ? - estimated mode center-frequencies
%---------- Preparations
% Period and sampling frequency of input signal
save_T = length(signal);
fs = 1/save_T;
% extend the signal by mirroring
T = save_T;
f_mirror(1:T/2) = signal(T/2:-1:1);
f_mirror(T/2+1:3*T/2) = signal;
f_mirror(3*T/2+1:2*T) = signal(T:-1:T/2+1);
f = f_mirror;
% Time Domain 0 to T (of mirrored signal)
T = length(f);
t = (1:T)/T;
% Spectral Domain discretization
freqs = t-0.5-1/T;
% Maximum number of iterations (if not converged yet, then it won't anyway)
N = 500;
% For future generalizations: individual alpha for each mode
Alpha = alpha*ones(1,K);
% Construct and center f_hat
f_hat = fftshift((fft(f)));
f_hat_plus = f_hat;
f_hat_plus(1:T/2) = 0;
% matrix keeping track of every iterant // could be discarded for mem
u_hat_plus = zeros(N, length(freqs), K);
% Initialization of omega_k
omega_plus = zeros(N, K);
switch init
? ?case 1
? ? ? ?for i = 1:K
? ? ? ? ? ?omega_plus(1,i) = (0.5/K)*(i-1);
? ? ? ?end
? ?case 2
? ? ? ?omega_plus(1,:) = sort(exp(log(fs) + (log(0.5)-log(fs))*rand(1,K)));
? ?otherwise
? ? ? ?omega_plus(1,:) = 0;
end
% if DC mode imposed, set its omega to 0
if DC
? ?omega_plus(1,1) = 0;
end
% start with empty dual variables
lambda_hat = zeros(N, length(freqs));
% other inits
uDiff = tol+eps; % update step
n = 1; % loop counter
sum_uk = 0; % accumulator
% ----------- Main loop for iterative updates
while ( uDiff > tol && ?n < N ) % not converged and below iterations limit
? ?% update first mode accumulator
? ?k = 1;
? ?sum_uk = u_hat_plus(n,:,K) + sum_uk - u_hat_plus(n,:,1);
? ?% update spectrum of first mode through Wiener filter of residuals
? ?u_hat_plus(n+1,:,k) = (f_hat_plus - sum_uk - lambda_hat(n,:)/2)./(1+Alpha(1,k)*(freqs - omega_plus(n,k)).^2);
? ?% update first omega if not held at 0
? ?if ~DC
? ? ? ?omega_plus(n+1,k) = (freqs(T/2+1:T)*(abs(u_hat_plus(n+1, T/2+1:T, k)).^2)')/sum(abs(u_hat_plus(n+1,T/2+1:T,k)).^2);
? ?end
? ?% update of any other mode
? ?for k=2:K
? ? ? ?% accumulator
? ? ? ?sum_uk = u_hat_plus(n+1,:,k-1) + sum_uk - u_hat_plus(n,:,k);
? ? ? ?% mode spectrum
? ? ? ?u_hat_plus(n+1,:,k) = (f_hat_plus - sum_uk - lambda_hat(n,:)/2)./(1+Alpha(1,k)*(freqs - omega_plus(n,k)).^2);
? ? ? ?% center frequencies
? ? ? ?omega_plus(n+1,k) = (freqs(T/2+1:T)*(abs(u_hat_plus(n+1, T/2+1:T, k)).^2)')/sum(abs(u_hat_plus(n+1,T/2+1:T,k)).^2);
? ?end
? ?% Dual ascent
? ?lambda_hat(n+1,:) = lambda_hat(n,:) + tau*(sum(u_hat_plus(n+1,:,:),3) - f_hat_plus);
? ?% loop counter
? ?n = n+1;
? ?% converged yet?
? ?uDiff = eps;
? ?for i=1:K
? ? ? ?uDiff = uDiff + 1/T*(u_hat_plus(n,:,i)-u_hat_plus(n-1,:,i))*conj((u_hat_plus(n,:,i)-u_hat_plus(n-1,:,i)))';
? ?end
? ?uDiff = abs(uDiff);
end
%------ Postprocessing and cleanup
% discard empty space if converged early
N = min(N,n);
omega = omega_plus(1:N,:);
% Signal reconstruction
u_hat = zeros(T, K);
u_hat((T/2+1):T,:) = squeeze(u_hat_plus(N,(T/2+1):T,:));
u_hat((T/2+1):-1:2,:) = squeeze(conj(u_hat_plus(N,(T/2+1):T,:)));
u_hat(1,:) = conj(u_hat(end,:));
u = zeros(K,length(t));
for k = 1:K
? ?u(k,:)=real(ifft(ifftshift(u_hat(:,k))));
end
% remove mirror part
u = u(:,T/4+1:3*T/4);
% recompute spectrum
clear u_hat;
for k = 1:K
? ?u_hat(:,k)=fftshift(fft(u(k,:)))';
end
end
?? 運(yùn)行結(jié)果
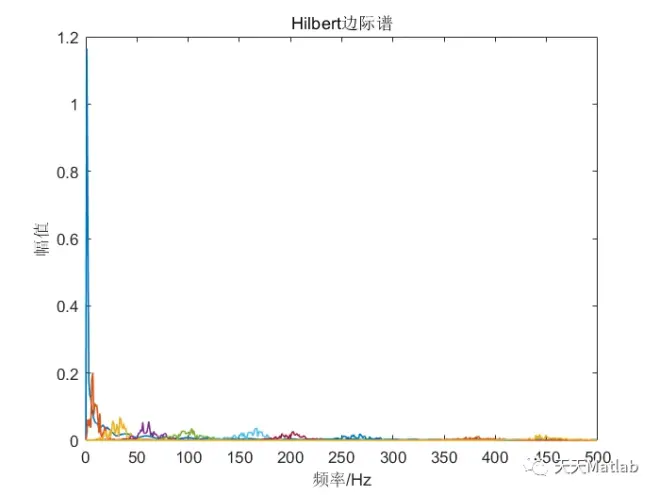
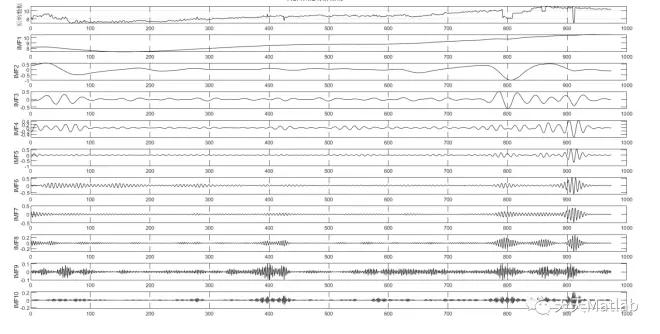
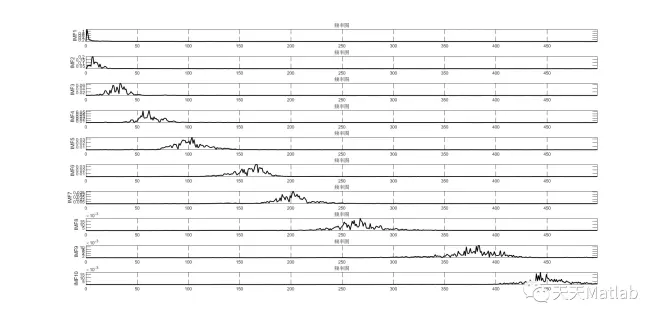
?? 參考文獻(xiàn)
[1] 魏永合,宮俊宇.基于CNN-LSTM-Attention的滾動(dòng)軸承故障診斷[J].沈陽理工大學(xué)學(xué)報(bào), 2022(004):041.
[2] 李愛蓮,全凌翔,崔桂梅,et al.融合正余弦和柯西變異的麻雀搜索算法[J].計(jì)算機(jī)工程與應(yīng)用, 2022, 58(3):9.DOI:10.3778/j.issn.1002-8331.2106-0148.
[3] 冉利民,李健偉,杜娟,等.基于變分模態(tài)分解算法的探地雷達(dá)信號(hào)去噪研究[J].世界地質(zhì), 2022(001):041.