arxiv論文日記-230712
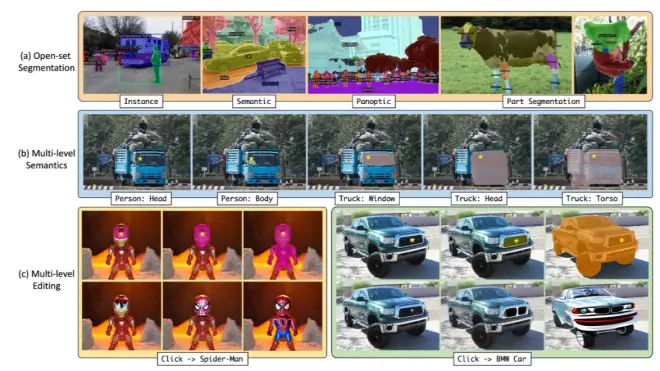
Paper1: Semantic-SAM

???? ?https://arxiv.org/pdf/2307.04767.pdf
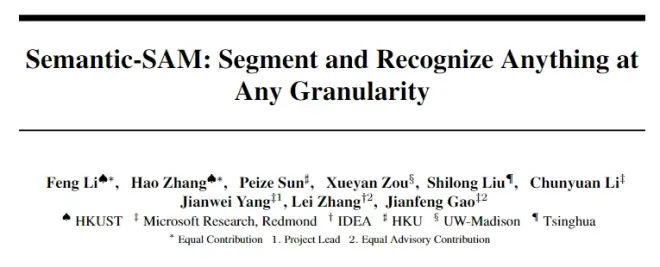
Abstract:?In this paper, we introduce Semantic-SAM, a universal image segmentation model
to enable segment and recognize anything at any desired granularity. Our model
offers two key advantages: semantic-awareness and granularity-abundance. To
achieve semantic-awareness, we consolidate multiple datasets across granularities
and train on decoupled objects and parts classification. This allows our model
to facilitate knowledge transfer among rich semantic information. For the multi-
granularity capability, we propose a multi-choice learning scheme, enabling each
click point to generate masks at multiple levels that correspond to multiple ground-
truth masks. Notably, this work represents the first attempt to jointly train a model
on SA-1B, generic, and part segmentation datasets. Experimental results and visu-
alizations demonstrate that our model successfully achieves semantic-awareness
and granularity-abundance. Furthermore, combining SA-1B training with other
segmentation tasks, such as panoptic and part segmentation, leads to performance
improvements. We will provide code and a demo for further exploration and
evaluation at https://github.com/UX-Decoder/Semantic-SAM.
摘要:在本文中,我們介紹了語義-SAM,這是一種通用的圖像分割模型,可以在任何期望的顆粒度下分割和識別任何東西。我們的模型提供了兩個關(guān)鍵優(yōu)勢:語義感知和顆粒度豐度。為了實現(xiàn)語義感知,我們跨粒度整合多個數(shù)據(jù)集,并在解耦的對象和部位分類上進行訓(xùn)練。這使得我們的模型能夠促進豐富語義信息之間的知識轉(zhuǎn)移。對于多顆粒度能力,我們提出了一種多選擇學(xué)習(xí)方案,使每個點擊點能夠在多個級別上生成對應(yīng)于多個真實掩碼的掩碼。值得注意的是,這項工作代表了在SA-1B、通用和部位分割數(shù)據(jù)集上聯(lián)合訓(xùn)練模型的首次嘗試。實驗結(jié)果和可視化表明,我們的模型成功地實現(xiàn)了語義感知和顆粒度豐富。此外,將SA-1B訓(xùn)練與其他分割任務(wù)(如全景和部位分割)相結(jié)合,可以提高性能。我們將提供代碼和演示,供進一步探索和評估:?https://github.com/UX-Decoder/Semantic-SAM。

Paper2:?Scaling up Visual Instruction Tuning

https://arxiv.org/pdf/2307.04087.pdf
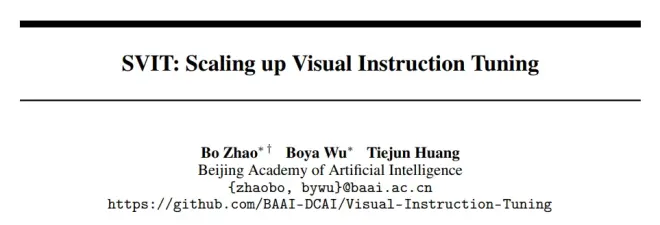
Abstract:?Thanks to the emerging of foundation models, the large language and vision models are integrated to acquire the multimodal ability of visual captioning, dialogue, question answering, etc. Although existing multimodal models present impressive performance of visual understanding and reasoning, their limits are still largely under-explored due to the scarcity of high-quality instruction tuning data. To push the limits of multimodal capability, we Scale up Visual Instruction Tuning (SVIT) by constructing a dataset of 3.2 million visual instruction tuning data including 1.6M conversation question-answer (QA) pairs and 1.6M complex reasoning QA pairs and 106K detailed image descriptions. Besides the volume, the proposed dataset is also featured by the high quality and rich diversity, which is generated by prompting GPT-4 with the abundant manual annotations of images. We empirically verify that training multimodal models on SVIT can significantly improve the multimodal performance in terms of visual perception, reasoning and planing.
摘要:?得益于基礎(chǔ)模型的出現(xiàn),將大語言和視覺模型集成起來,獲得視覺描述、對話、問答等多模態(tài)能力。盡管現(xiàn)有的多模態(tài)模型在視覺理解和推理方面表現(xiàn)出色,但由于缺乏高質(zhì)量的指令微調(diào)數(shù)據(jù),它們的極限仍未得到充分探索。為了突破多模態(tài)能力的極限,我們擴展了視覺指令微調(diào)(SVIT),通過構(gòu)建320萬視覺指令調(diào)整數(shù)據(jù)集,包括160萬對話問答(QA)對和160萬復(fù)雜推理問答對,以及106K詳細的圖像描述。除了體量之外,所提出的數(shù)據(jù)集還具有高質(zhì)量和豐富多樣性的特點,這是通過用豐富的圖像的人工標(biāo)注來提示GPT-4生成的。我們實證驗證,在SVIT上訓(xùn)練多模態(tài)模型可以顯著提高視覺感知、推理和規(guī)劃方面的多模態(tài)性能。
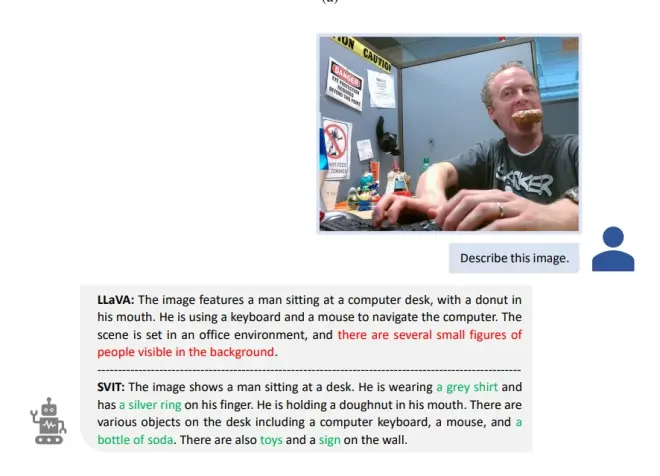
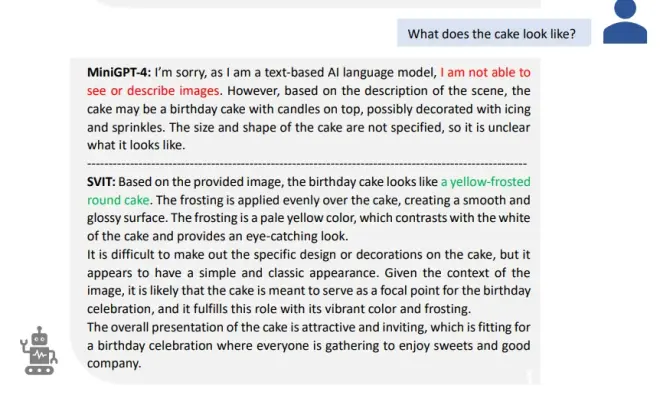
“MiniGPT4” and “SVIT”