R語言逐步多元回歸模型分析長鼻魚密度影響因素
?原文鏈接:http://tecdat.cn/?p=9564
?
目錄
如何做多元回歸
逐步回歸選擇模型
逐步程序
定義最終模型
?方差分析
預測值圖
檢查模型的假設
模型擬合標準
將模型與似然比檢驗進行比較
我從馬里蘭州生物流調查中提取了一些數據,以進行多元回歸分析。數據因變量是每75米長的水流中長鼻魚(Rhinichthys cataractae)的數量。自變量是河流流失的面積(英畝);氧濃度(毫克/升);水流段的最大深度(以厘米為單位);硝酸鹽濃度(毫克/升);硫酸鹽濃度(毫克/升);以及采樣日期的水溫(以攝氏度為單位)。
?
如何做多元回歸
?
多重相關
數據集包含多個數值變量時,最好查看這些變量之間的相關性。原因之一是,可以輕松查看哪些自變量與該因變量相關。第二個原因是,如果要構建多元回歸模型,則添加高度相關的自變量不太可能對模型有很大的改進。
?
最后,值得看一下數字變量的分布。如果分布差異很大,則使用Kendall或Spearman相關性可能更合適。同樣,如果自變量與因變量的分布不同,則可能需要對自變量進行轉換。
?
?
Data = read.table(textConnection(Input),header=TRUE)
Data.num =?
?? select(Data,
????????? Longnose,
????????? Acerage,
????????? DO2,
????????? Maxdepth,
????????? NO3,
????????? SO4,
????????? Temp)
headtail(Data.num)
?? Longnose Acerage? DO2 Maxdepth? NO3?? SO4 Temp
1??????? 13??? 2528? 9.6?????? 80 2.28 16.75 15.3
2??????? 12??? 3333? 8.5?????? 83 5.34? 7.74 19.4
3??????? 54?? 19611? 8.3?????? 96 0.99 10.92 19.5
66?????? 20??? 4106 10.0?????? 96 2.62? 5.45 15.4
67?????? 38?? 10274? 9.3?????? 90 5.45 24.76 15.0
68?????? 19???? 510? 6.7?????? 82 5.25 14.19 26.5
corr.test(Data.num,?
????????? use = "pairwise",
????????? method="pearson",
????????? adjust="none", ????# 可以調整p值
????????? alpha=.05)
Correlation matrix
???????? Longnose Acerage?? DO2 Maxdepth?? NO3?? SO4? Temp
Longnose???? 1.00??? 0.35? 0.14???? 0.30? 0.31 -0.02? 0.14
Acerage????? 0.35??? 1.00 -0.02???? 0.26 -0.10? 0.05? 0.00
DO2????????? 0.14?? -0.02? 1.00??? -0.06? 0.27 -0.07 -0.32
Maxdepth???? 0.30?? ?0.26 -0.06???? 1.00? 0.04 -0.05? 0.00
NO3????????? 0.31?? -0.10? 0.27???? 0.04? 1.00 -0.09? 0.00
SO4???????? -0.02??? 0.05 -0.07??? -0.05 -0.09? 1.00? 0.08
Temp???????? 0.14??? 0.00 -0.32???? 0.00? 0.00? 0.08? 1.00
Sample Size
Probability values (Entries above the diagonal are adjusted for multiple tests.)
???????? Longnose Acerage? DO2 Maxdepth? NO3? SO4 Temp
Longnose???? 0.00??? 0.00 0.27???? 0.01 0.01 0.89 0.26
Acerage????? 0.00??? 0.00 0.86???? 0.03 0.42 0.69 0.98
DO2????????? 0.27??? 0.86 0.00???? 0.64 0.02 0.56 0.01
Maxdepth???? 0.01??? 0.03 0.64???? 0.00 0.77 0.69 0.97
NO3????????? 0.01??? 0.42 0.02???? 0.77 0.00 0.48 0.99
SO4????????? 0.89??? 0.69 0.56???? 0.69 0.48 0.00 0.52
Temp???????? 0.26??? 0.98 0.01???? 0.97 0.99 0.52 0.00
?
?
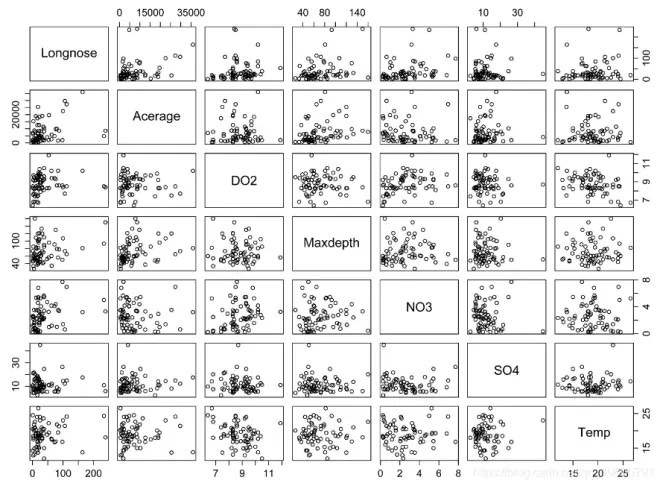
?
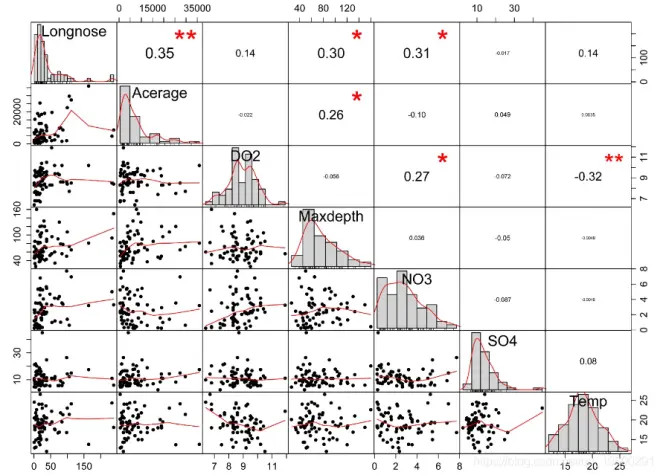
?
?
逐步回歸選擇模型
使用AIC(赤池信息標準)作為選擇標準??梢允褂眠x項k = log(n)代替BIC。?
?
逐步程序
Longnose ~ 1
?????????? Df Sum of Sq??? RSS??? AIC
+ Acerage?? 1?? 17989.6 131841 518.75
+ NO3?????? 1?? 14327.5 135503 520.61
+ Maxdepth? 1?? 13936.1 135894 520.81
<none>????????????????? 149831 525.45
+ Temp????? 1??? 2931.0 146899 526.10
+ DO2?????? 1??? 2777.7 147053 526.17
+ SO4?????? 1????? 45.3 149785 527.43
.
.
< snip... more steps >
.
.
Longnose ~ Acerage + NO3 + Maxdepth
?????????? Df Sum of Sq??? RSS??? AIC
<none>????????????????? 107904 509.13
+ Temp????? 1??? 2948.0 104956 509.24
+ DO2?????? 1???? 669.6 107234 510.70
- Maxdepth? 1??? 6058.4 113962 510.84
+ SO4?????? 1?????? 5.9 107898 511.12
- Acerage?? 1?? 14652.0 122556 515.78
- NO3?????? 1?? 16489.3 124393 516.80
Call:
lm(formula = Longnose ~ Acerage + NO3 + Maxdepth, data = Data)
Coefficients:
(Intercept)????? Acerage????????? NO3???? Maxdepth?
?-23.829067???? 0.001988???? 8.673044???? 0.336605?
?
?
定義最終模型
?
summary(model.final)??????# 顯示系數,R平方和總體p值
????????????? Estimate Std. Error t value Pr(>|t|)??
(Intercept) -2.383e+01? 1.527e+01? -1.560? 0.12367??
Acerage????? 1.988e-03? 6.742e-04?? 2.948? 0.00446 **
Maxdepth???? 3.366e-01? 1.776e-01?? 1.896? 0.06253 .
NO3???? ?????8.673e+00? 2.773e+00?? 3.127? 0.00265 **
Multiple R-squared:? 0.2798,? Adjusted R-squared:? 0.2461
F-statistic: 8.289 on 3 and 64 DF,? p-value: 9.717e-05
?
?方差分析
?
Anova Table (Type II tests)
Response: Longnose
????????? Sum Sq Df F value?? Pr(>F)??
Acerage??? 14652? 1? 8.6904 0.004461 **
Maxdepth??? 6058? 1? 3.5933 0.062529 .
NO3??????? 16489? 1? 9.7802 0.002654 **
Residuals 107904 64
?
預測值圖
?
?
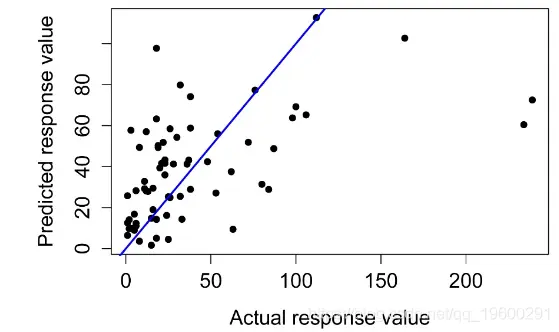
?
?
檢查模型的假設
?
?
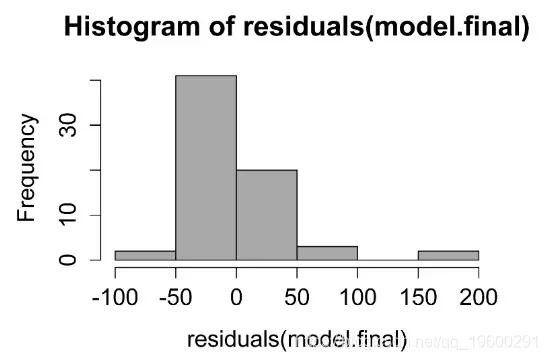
線性模型中殘差的直方圖。這些殘差的分布應近似正態(tài)。
?
?
?
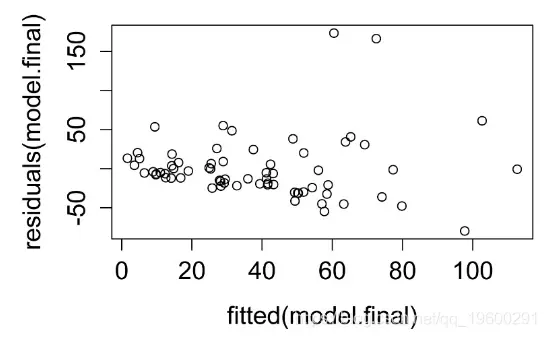
?
?
殘差與預測值的關系圖。殘差應無偏且均等。?
?
?
?????????????
?
模型擬合標準
模型擬合標準可用于確定最合適的模型。使用AIC或可選的BIC。AICc是對AIC的一種調整,它更適合于觀測值相對較少的數據集。AIC,AICc和BIC越小越好。
?
在下面的例子中,我們只討論了顯著相關的種植面積,MAXDEPTH和NO3?。?
$Models
?? Formula????????????????????????????????????
1? "Longnose ~ Acerage"???????????????????????
2? "Longnose ~ Maxdepth"??????????????????????
3? "Longnose ~ NO3"???????????????????????????
4? "Longnose ~ Acerage + Maxdepth"????????????
5? "Longnose ~ Acerage + NO3"?????????????????
6? "Longnose ~ Maxdepth + NO3"????????????????
7? "Longnose ~ Acerage + Maxdepth + NO3"??????
8? "Longnose ~ Acerage + Maxdepth + NO3 + DO2"
9? "Longnose ~ Acerage + Maxdepth + NO3 + SO4"
10 "Longnose ~ Acerage + Maxdepth + NO3 + Temp"??
$Fit.criteria
?? Rank Df.res?? AIC? AICc?? BIC R.squared Adj.R.sq?? p.value Shapiro.W Shapiro.p
1???? 2???? 66 713.7 714.1 720.4?? 0.12010? 0.10670 3.796e-03??? 0.7278 6.460e-10
2???? 2???? 66 715.8 716.2 722.4?? 0.09301? 0.07927 1.144e-02??? 0.7923 2.115e-08
3???? 2???? 66 715.6 716.0 722.2?? 0.09562? 0.08192 1.029e-02??? 0.7361 9.803e-10
4???? 3???? 65 711.8 712.4 720.6?? 0.16980? 0.14420 2.365e-03??? 0.7934 2.250e-08
5???? 3???? 65 705.8 706.5 714.7?? 0.23940? 0.21600 1.373e-04??? 0.7505 2.055e-09
6???? 3???? 65 710.8 711.4 719.6?? 0.18200? 0.15690 1.458e-03??? 0.8149 8.405e-08
7???? 4???? 64 704.1 705.1 715.2?? 0.27980? 0.24610 9.717e-05??? 0.8108 6.511e-08
8???? 5???? 63 705.7 707.1 719.0?? 0.28430? 0.23890 2.643e-04??? 0.8041 4.283e-08
9???? 5???? 63 706.1 707.5 719.4?? 0.27990? 0.23410 3.166e-04??? 0.8104 6.345e-08
10??? 5???? 63 704.2 705.6 717.5?? 0.29950? 0.25500 1.409e-04??? 0.8225 1.371e-07
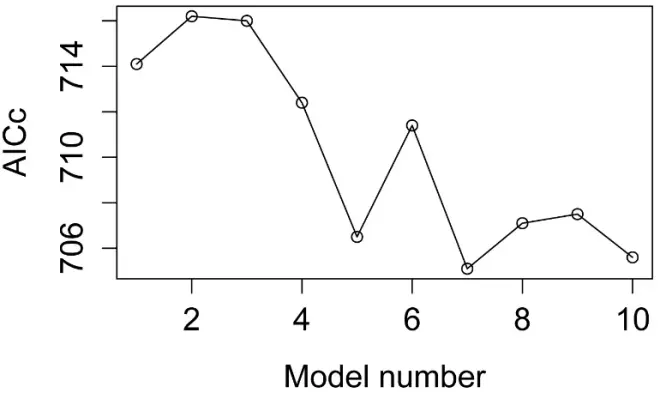
?
幾個模型的AICc(修改后的Akaike信息標準)圖。模型7最小化了AICc,因此被選為該模型中的最佳模型。
?
?
將模型與似然比檢驗進行比較
將模型與 平方和檢驗或似然比檢驗進行比較,以查看是否有其他項顯著減少平方誤差和 。
Analysis of Variance Table
Model 1: Longnose ~ Acerage + Maxdepth + NO3
Model 2: Longnose ~ Acerage + Maxdepth
? Res.Df??? RSS Df Sum of Sq????? F?? Pr(>F)??
1???? 64 107904???????????????????????????????
2???? 65 124393 -1??? -16489 9.7802 0.002654 **
Likelihood ratio test
Model 1: Longnose ~ Acerage + Maxdepth + NO3
Model 2: Longnose ~ Acerage + Maxdepth
? #Df? LogLik Df? Chisq Pr(>Chisq)??
1?? 5 -347.05???????????????????????
2?? 4 -351.89 -1 9.6701?? 0.001873 **
Model 1: Longnose ~ Acerage + Maxdepth + NO3 + DO2
Model 2: Longnose ~ Acerage + Maxdepth + NO3 + SO4
Model 3: Longnose ~ Acerage + Maxdepth + NO3 + Temp
Model A: Longnose ~ Acerage + Maxdepth + NO3
??? DfO????? RSSO DfA????? RSSA Df??????? SS????? F Pr(>F)
1vA? 63 107234.38? 64 107903.97 -1?? -669.59 0.3934 0.5328
2vA? 63 107898.06? 64 107903.97 -1???? -5.91 0.0035 0.9533
3vA? 63 104955.97? 64 107903.97 -1? -2948.00 1.7695 0.1882
Model 1: Longnose ~ Acerage + Maxdepth + NO3 + DO2
Model 2: Longnose ~ Acerage + Maxdepth + NO3 + SO4
Model 3: Longnose ~ Acerage + Maxdepth + NO3 + Temp
Model A: Longnose ~ Acerage + Maxdepth + NO3
??? DfO??? logLikO DfA??? logLikA Df???? logLik? Chisq Pr(>Chisq)
1vA? 63 -346.83881? 64 -347.05045 -1??? 0.21164 0.4233???? 0.5153
2vA? 63 -347.04859? 64 -347.05045 -1??? 0.00186 0.0037???? 0.9513
3vA? 63 -346.10863? 64 -347.05045 -1??? 0.94182 1.8836???? 0.1699
?
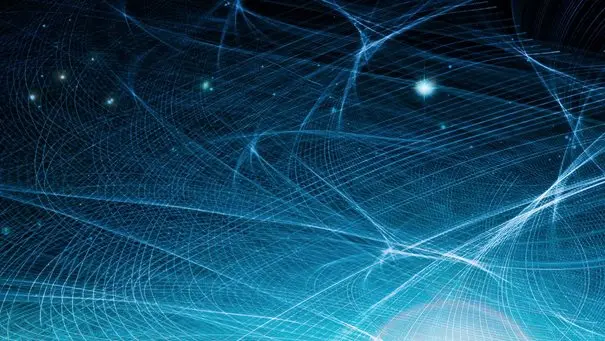
參考文獻
1.R語言多元Logistic邏輯回歸 應用案例
2.面板平滑轉移回歸(PSTR)分析案例實現
3.matlab中的偏最小二乘回歸(PLSR)和主成分回歸(PCR)
4.R語言泊松Poisson回歸模型分析案例
5.R語言回歸中的Hosmer-Lemeshow擬合優(yōu)度檢驗
6.r語言中對LASSO回歸,Ridge嶺回歸和Elastic Net模型實現
7.在R語言中實現Logistic邏輯回歸
8.python用線性回歸預測股票價格
9.R語言如何在生存分析與Cox回歸中計算IDI,NRI指標