Survey Paper 多元訪問邊緣計(jì)算的分散型深度學(xué)習(xí): 通信效率與可信度
Decentralized Deep Learning for Multi-Access Edge Computing: A Survey on Communication Efficiency and Trustworthiness (The University of Tokyo)
IEEE Transactions on Artificial Intelligence https://ieeexplore.ieee.org/document/9645169
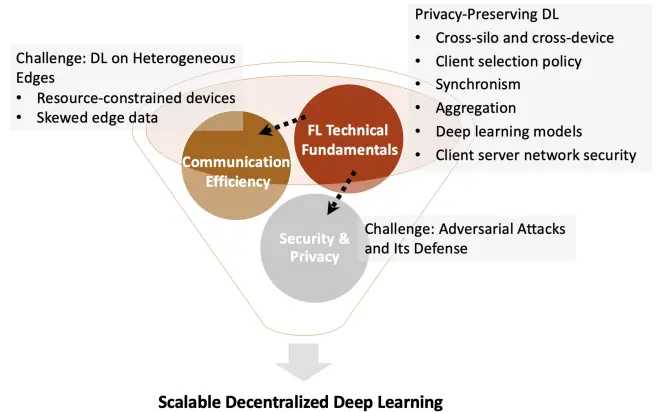
Impact Statement:
The proliferation of smart devices and edge applications based on deep learning is reshaping the contours of future high-performance edge computing, such as intelligent environment sensors, autonomous vehicles, smart grids, and so forth. Decentralized deep learning (DDL) as a key enabler of the multiaccess edge computing benefits society through distributed model training and globally shared training knowledge. However, crucial fundamental challenges have to be overcome in the first place to make DDL feasible and scalable, which are decentralization techniques, communication efficiency, and trustworthiness. This survey offers a comprehensive overview from the above perspectives, suggesting that DDL is being intensively studied, especially in terms of privacy protection, edge heterogeneity, and adversarial attacks and defenses. Moreover, the future trends of DDL put weight on topics such as efficient resource allocation, asynchronous communication, and fully decentralized frameworks.