爆肝118篇!神經(jīng)輻射場(chǎng)(NeRF)近三年頂會(huì)論文分享,含2023年最新
有沒(méi)有發(fā)現(xiàn)最近神經(jīng)輻射場(chǎng)相關(guān)話題又有點(diǎn)爆了?
其實(shí)NeRF一直都挺火的,相關(guān)論文在Google Scholar上引用量已經(jīng)超過(guò)6000次,每年頂級(jí)視覺(jué)會(huì)議如CVPR、ICCV、ECCV等都有大量關(guān)于神經(jīng)輻射場(chǎng)的高質(zhì)量工作被接受。許多科研機(jī)構(gòu)和公司比如微軟、Facebook、谷歌等都在研究神經(jīng)輻射場(chǎng)的不同應(yīng)用,有的已經(jīng)將其應(yīng)用于產(chǎn)品中,如捕捉人體動(dòng)作等。
今年有關(guān)NeRF的最新研究也有不少了,上次和你們簡(jiǎn)單分享了17篇,傳送門點(diǎn)這里。
這次學(xué)姐爆肝整理了2021-2023近三年神經(jīng)輻射場(chǎng)相關(guān)的頂會(huì)論文118篇!想發(fā)paper的同學(xué)務(wù)必收藏,抓緊時(shí)間學(xué)起來(lái)了!
論文資料已經(jīng)幫大家做了分類,包含綜述、姿態(tài)估計(jì)、推理、訓(xùn)練、組合性、場(chǎng)景標(biāo)注、對(duì)象類別建模等多個(gè)細(xì)分方向,需要的同學(xué)看這里??
掃碼添加小享,回復(fù)“NeRF”
免費(fèi)獲取全部118篇論文資料合集
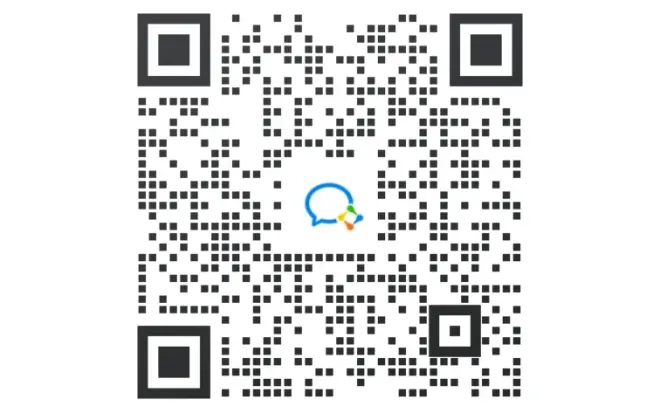
綜述
BeyondPixels: A Comprehensive Review of the Evolution of Neural Radiance Fields
神經(jīng)輻射場(chǎng)演化的全面綜述
簡(jiǎn)述:鑒于NeRF的廣泛吸引力,全面檢查NeRF的現(xiàn)有研究非常重要。盡管以前的3D渲染調(diào)研集中在傳統(tǒng)的基于計(jì)算機(jī)視覺(jué)或深度學(xué)習(xí)的方法,但只有少數(shù)討論了NeRF的潛力。這些調(diào)查主要關(guān)注NeRF的早期貢獻(xiàn),沒(méi)有探索它的全部潛力。本文回顧了NeRF的最新進(jìn)展,并根據(jù)架構(gòu)設(shè)計(jì)對(duì)其進(jìn)行分類,特別是在新視圖合成方面。

NeRF: Neural Radiance Field in 3D Vision, A Comprehensive Review
3D視覺(jué)中的神經(jīng)輻射場(chǎng),一個(gè)全面綜述
簡(jiǎn)述:神經(jīng)輻射場(chǎng)(NeRF)作為一種新穎的基于隱式場(chǎng)景表示的視圖合成方法,已在計(jì)算機(jī)視覺(jué)領(lǐng)域產(chǎn)生巨大影響。鑒于NeRF的普及和研究興趣,作者認(rèn)為有必要對(duì)過(guò)去兩年的NeRF論文進(jìn)行全面綜述。作者從架構(gòu)和應(yīng)用兩個(gè)維度對(duì)論文進(jìn)行分類,還介紹了NeRF合成新視圖的理論,以及比較了關(guān)鍵NeRF模型的性能和速度。
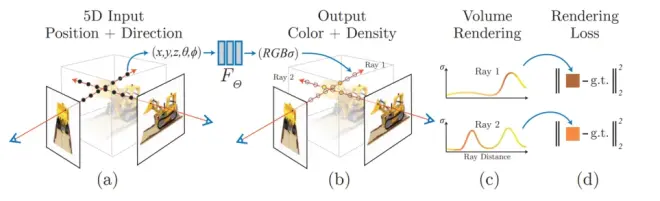
推理&訓(xùn)練&壓縮
Hardware Acceleration of Neural Graphics(推理)
神經(jīng)圖形學(xué)的硬件加速
簡(jiǎn)述:論文研究了四個(gè)代表性的神經(jīng)圖形學(xué)應(yīng)用,發(fā)現(xiàn)當(dāng)前GPU存在1.5-55倍的性能差距,難以達(dá)到理想的渲染性能。作者識(shí)別輸入編碼和多層感知器是性能瓶頸,提出了一個(gè)神經(jīng)圖形處理集群架構(gòu),通過(guò)專用引擎直接加速關(guān)鍵模塊,支持各種神經(jīng)圖形學(xué)應(yīng)用。
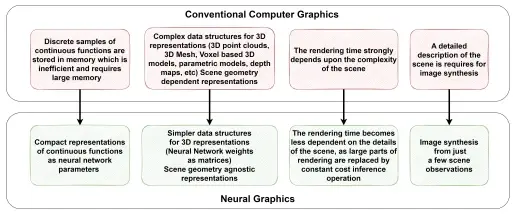
DyLiN: Making Light Field Networks Dynamic
RT-NeRF: Real-Time On-Device Neural Radiance Fields Towards Immersive AR/VR Rendering
Efficient Neural Radiance Fields for Interactive Free-viewpoint Video
R2L: Distilling Neural Radiance Field to Neural Light Field for Efficient Novel View Synthesis
Real-Time Neural Light Field on Mobile Devices
Learning Neural Transmittance for Efficient Rendering of Reflectance Fields
AUTOMATIC INTEGRATION
DONeRF: Towards Real-Time Rendering of Compact Neural Radiance Fields using Depth Oracle Networks
FastNeRF: High-Fidelity Neural Rendering at 200FPS
KiloNeRF: Speeding up Neural Radiance Fields with Thousands of Tiny MLPs
For Real-time Rendering of Neural Radiance Fields
Mixture of Volumetric Primitives for Efficient Neural Rendering
Light Field Networks: Neural Scene Representations with Single-Evaluation Rendering
BakedSDF: Meshing Neural SDFs for Real-Time View Synthesis(訓(xùn)練)
為實(shí)時(shí)視圖合成構(gòu)建神經(jīng)SDF網(wǎng)格
簡(jiǎn)述:論文提出了一種方法,用于重建大尺度無(wú)界實(shí)景的高質(zhì)量網(wǎng)格,適用于逼真的新視角合成。作者首先優(yōu)化了一個(gè)混合的神經(jīng)體積-表面場(chǎng)景表示,設(shè)計(jì)為具有良好的等值面對(duì)應(yīng)場(chǎng)景中的表面。然后,將這個(gè)表示“烘焙”成高質(zhì)量的三角網(wǎng)格,并配備基于球形高斯的簡(jiǎn)單快速的視點(diǎn)依賴外觀模型。最后,優(yōu)化這個(gè)“烘焙”表示以最好地重現(xiàn)捕捉的視點(diǎn),得到一個(gè)模型,可以利用多邊形光柵化管線進(jìn)行實(shí)時(shí)視圖合成。
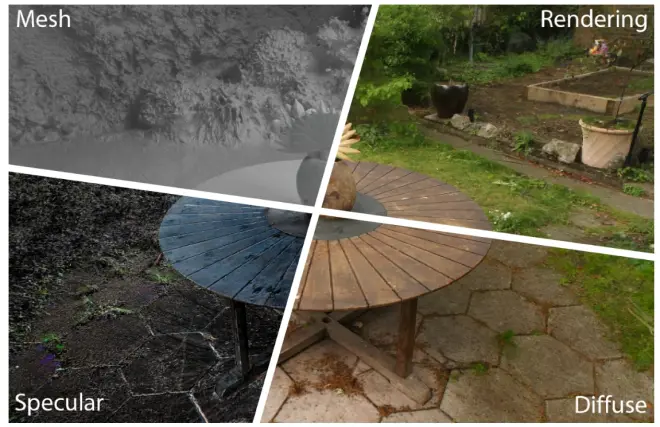
Direct Voxel Grid Optimization: Super-fast Convergence for Radiance Fields Reconstruction
Instant Neural Graphics Primitives with a Multiresolution Hash Encoding
Radiance Fields without Neural Networks
TensoRF: Tensorial Radiance Fields
Depth-supervised NeRF: Fewer Views and Faster Training for Free
Variable Bitrate Neural Fields(壓縮)
可變比特率神經(jīng)場(chǎng)
簡(jiǎn)述:論文提出了一種特征網(wǎng)格壓縮字典方法,將其內(nèi)存消耗降低了高達(dá)100倍,并允許多分辨率表示,這對(duì)流式處理很有用。作者將字典優(yōu)化表述為一個(gè)向量量化自動(dòng)解碼問(wèn)題,這使我們可以在一個(gè)沒(méi)有直接監(jiān)督的空間中端到端學(xué)習(xí)離散神經(jīng)表示,擁有動(dòng)態(tài)拓?fù)浣Y(jié)構(gòu)。
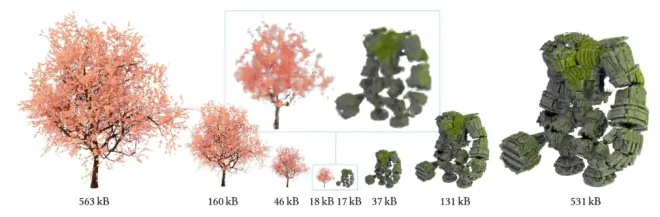
不受約束的圖像
Ha-NeRF??: Hallucinated Neural Radiance Fields in the Wild
HDR-Plenoxels: Self-Calibrating High Dynamic Range Radiance Fields
NeRF in the Wild: Neural Radiance Fields for Unconstrained Photo Collections
可變形
AligNeRF: High-Fidelity Neural Radiance Fields via Alignment-Aware Training
通過(guò)對(duì)齊感知訓(xùn)練的高保真神經(jīng)輻射場(chǎng)
簡(jiǎn)述:在本文中,作者進(jìn)行了首個(gè)在高分辨率數(shù)據(jù)上訓(xùn)練NeRF的先導(dǎo)研究,并提出相應(yīng)的解決方案:1)將多層感知機(jī)與卷積層結(jié)合,可編碼更多鄰域信息同時(shí)減少參數(shù)總數(shù),2)一種新穎的訓(xùn)練策略來(lái)解決由移動(dòng)物體或小的相機(jī)標(biāo)定誤差引起的不對(duì)齊,3)一個(gè)注意高頻信息的損失函數(shù)。該方法幾乎不引入額外的訓(xùn)練/測(cè)試成本,實(shí)驗(yàn)結(jié)果表明,與當(dāng)前最先進(jìn)的NeRF模型相比,它可以恢復(fù)更多高頻細(xì)節(jié)。
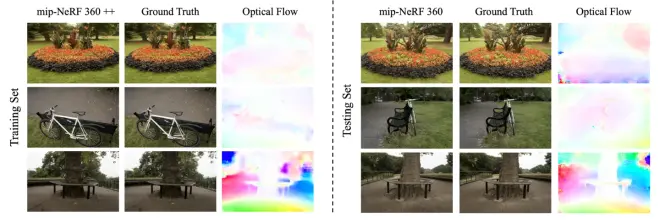
DynIBaR: Neural Dynamic Image-Based Rendering
D-NeRF: Neural Radiance Fields for Dynamic Scenes
Dynamic Neural Radiance Fields for Monocular 4D Facial Avatar Reconstruction
PVA: Pixel-aligned Volumetric Avatars
Neural Articulated Radiance Field
CLA-NeRF: Category-Level Articulated Neural Radiance Field
Animatable Neural Radiance Fields for Modeling Dynamic Human Bodies
A Higher-Dimensional Representation for Topologically Varying Neural Radiance Fields
IBRNet: Learning Multi-View Image-Based Rendering
Neural Scene Flow Fields for Space-Time View Synthesis of Dynamic Scenes
Animatable Neural Radiance Fields from Monocular RGB Videos
Neural Actor: Neural Free-view Synthesis of Human Actors with Pose Control
TiNeuVox: Fast Dynamic Radiance Fields with Time-Aware Neural Voxels
HumanNeRF: Free-viewpoint Rendering of Moving People from Monocular Video
視頻&概括
UV Volumes for Real-time Rendering of Editable Free-view Human Performance(視頻)
用于可編輯自由視角人類表演實(shí)時(shí)渲染的UV體數(shù)據(jù)集
簡(jiǎn)述:論文提出了一種新的方法,可以對(duì)人類表演者進(jìn)行實(shí)時(shí)的自由視角視頻渲染。該方法將高頻人體外觀信息從3D體數(shù)據(jù)集中分離出來(lái),編碼為2D神經(jīng)紋理?xiàng)?。使用參?shù)化映射建立人體模型與光滑紋理坐標(biāo)之間的聯(lián)系,以實(shí)現(xiàn)良好的泛化能力。該方法允許使用更小更淺的網(wǎng)絡(luò)獲得3D信息,同時(shí)在2D中捕捉細(xì)節(jié)。
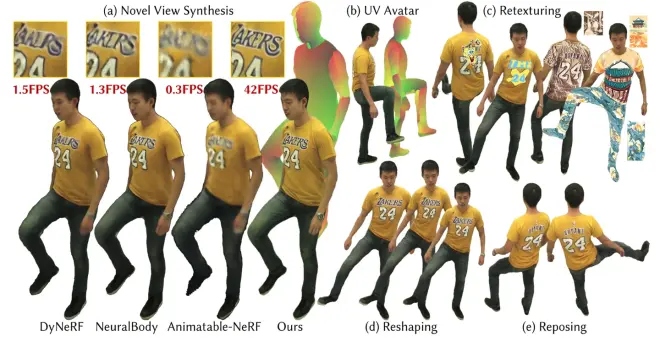
掃碼添加小享,回復(fù)“NeRF”
免費(fèi)獲取全部118篇論文資料合集
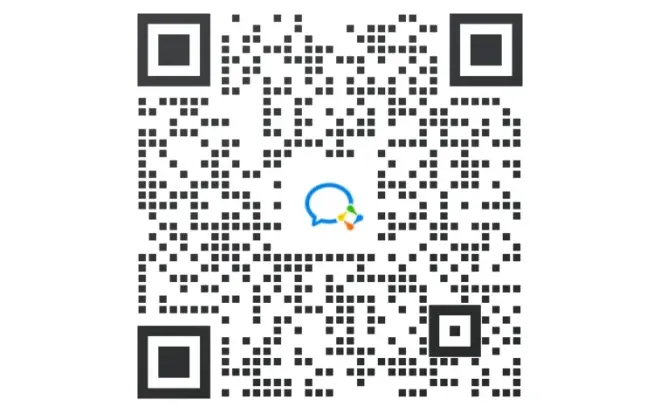
NeO 360: Neural Fields for Sparse View Synthesis of Outdoor Scenes(概括)
用于戶外場(chǎng)景稀疏視圖合成的神經(jīng)場(chǎng)
簡(jiǎn)述:論文研究了一種新的隱式神經(jīng)表示方法,稱為NeO 360,它可以從單個(gè)或很少的RGB圖像合成360°全方位視角的戶外場(chǎng)景。該方法的核心創(chuàng)新是提出一種混合的基于體素和俯視圖的場(chǎng)景表示,它結(jié)合了兩者的優(yōu)點(diǎn),既可以高效表示復(fù)雜室外場(chǎng)景,也可以從任意視點(diǎn)進(jìn)行查詢。
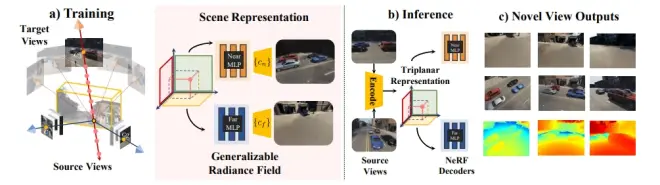
Neural Scene Flow Fields for Space-Time View Synthesis of Dynamic Scenes
Space-time Neural Irradiance Fields for Free-Viewpoint Video
Neural Body: Implicit Neural Representations with Structured Latent Codes for Novel View Synthesis of Dynamic Humans
Neural 3D Video Synthesis from Multi-view Video
Dynamic View Synthesis from Dynamic Monocular Video
Streaming Radiance Fields for 3D Video Synthesis
pixelNeRF: Neural Radiance Fields from One or Few Images
Learned Initializations for Optimizing Coordinate-Based Neural Representations
pi-GAN: Periodic Implicit Generative Adversarial Networks for 3D-Aware Image Synthesis
ShaRF: Shape-conditioned Radiance Fields from a Single View
IBRNet: Learning Multi-View Image-Based Rendering
CAMPARI: Camera-Aware Decomposed Generative Neural Radiance Fields
NeRF-VAE: A Geometry Aware 3D Scene Generative Model
Unconstrained Scene Generation with Locally Conditioned Radiance Fields
MVSNeRF: Fast Generalizable Radiance Field Reconstruction from Multi-View Stereo
Stereo Radiance Fields (SRF): Learning View Synthesis from Sparse Views of Novel Scenes
Neural Rays for Occlusion-aware Image-based Rendering
Putting NeRF on a Diet: Semantically Consistent Few-Shot View Synthesis
MINE: Towards Continuous Depth MPI with NeRF for Novel View Synthesis
T?RF: Time-of-Flight Radiance Fields for Dynamic Scene View Synthesis
CodeNeRF: Disentangled Neural Radiance Fields for Object Categories
StyleNeRF: A Style-based 3D-Aware Generator for High-resolution Image Synthesis
Generative Occupancy Fields for 3D Surface-Aware Image Synthesis
NeRF in the Dark: High Dynamic Range View Synthesis from Noisy Raw Images
Point-NeRF: Point-based Neural Radiance Fields
SinNeRF: Training Neural Radiance Fields on Complex Scenes from a Single Image
Rodin: A Generative Model for Sculpting 3D Digital Avatars Using Diffusion
SurfelNeRF: Neural Surfel Radiance Fields for Online Photorealistic Reconstruction of Indoor Scenes
姿態(tài)估計(jì)
L2G-NeRF: Local-to-Global Registration for Bundle-Adjusting Neural Radiance Fields
用于捆綁調(diào)整神經(jīng)輻射場(chǎng)的本地到全局注冊(cè)
簡(jiǎn)述:論文提出了L2G-NeRF,一種用于捆綁調(diào)整神經(jīng)輻射場(chǎng)的局部到全局注冊(cè)方法:首先進(jìn)行基于像素的靈活對(duì)齊,然后進(jìn)行基于幀的受限參數(shù)對(duì)齊。像素級(jí)局部對(duì)齊通過(guò)深度網(wǎng)絡(luò)無(wú)監(jiān)督學(xué)習(xí),優(yōu)化光度重建誤差。幀級(jí)全局對(duì)齊使用可微參數(shù)估計(jì)求解器在像素級(jí)對(duì)應(yīng)上找到全局變換。在合成和真實(shí)數(shù)據(jù)集上的實(shí)驗(yàn)表明,該的方法在高保真重建和解決大的相機(jī)位姿誤差方面優(yōu)于當(dāng)前最先進(jìn)的方法。
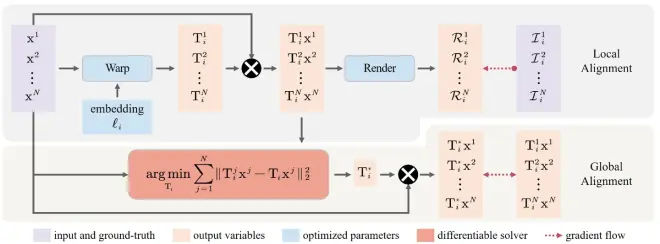
Loc-NeRF: Monte Carlo Localization using Neural Radiance Fields
Robust Camera Pose Refinement for Multi-Resolution Hash Encoding
iNeRF: Inverting Neural Radiance Fields for Pose Estimation
A-NeRF: Surface-free Human 3D Pose Refinement via Neural Rendering
NeRF--: Neural Radiance Fields Without Known Camera Parameters
iMAP: Implicit Mapping and Positioning in Real-Time
NICE-SLAM: Neural Implicit Scalable Encoding for SLAM
GNeRF: GAN-based Neural Radiance Field without Posed Camera
BARF: Bundle-Adjusting Neural Radiance Fields
Self-Calibrating Neural Radiance Fields
組合性
Unsupervised Discovery and Composition of Object Light Fields
無(wú)監(jiān)督發(fā)現(xiàn)和組合對(duì)象光場(chǎng)
簡(jiǎn)述:本文提出了一種組成式對(duì)象光場(chǎng)表示,可以高效表示和重建物體層面的光信息。該表示將物體建模為光場(chǎng),并設(shè)計(jì)了一個(gè)組合模塊,可以從對(duì)象光場(chǎng)重構(gòu)全局光場(chǎng)。這樣既實(shí)現(xiàn)了以對(duì)象為中心的表示,也大大提升了效率。實(shí)驗(yàn)表明,該表示可以實(shí)現(xiàn)無(wú)監(jiān)督學(xué)習(xí)物體表示,同時(shí)重建和渲染速度比其他3D方法提升了多個(gè)數(shù)量級(jí)。
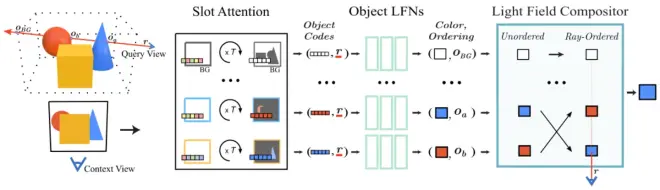
Learning Object-centric Neural Scattering Functions for Free-viewpoint Relighting and Scene Composition
GIRAFFE: Representing Scenes as Compositional Generative Neural Feature Fields
Neural Scene Graphs for Dynamic Scenes
Unsupervised Discovery of Object Radiance Fields
Learning Object-Compositional Neural Radiance Field for Editable Scene Rendering
MoFaNeRF: Morphable Facial Neural Radiance Field
照明
KiloNeuS: Implicit Neural Representations with Real-Time Global Illumination
NeRV: Neural Reflectance and Visibility Fields for Relighting and View Synthesis
NeX: Real-time View Synthesis with Neural Basis Expansion
NeRFactor: Neural Factorization of Shape and Reflectance Under an Unknown Illumination
A Shading-Guided Generative Implicit Model for Shape-Accurate 3D-Aware Image Synthesis
場(chǎng)景標(biāo)注和理解
NeRF-SOS: Any-view Self-supervised Object Segmentation on Complex Real-world Scenes
In-Place Scene Labelling and Understanding with Implicit Scene Representation
NeRF編輯
Local 3D Editing via 3D Distillation of CLIP Knowledge
SINE: Semantic-driven Image-based NeRF Editing with Prior-guided Editing Field
General Neural Gauge Fields
Editing Conditional Radiance Fields
Editable Free-viewpoint Video Using a Layered Neural Representation
NeRF-In: Free-Form NeRF Inpainting with RGB-D Priors
Unified Implicit Neural Stylization
CLIP-NeRF: Text-and-Image Driven Manipulation of Neural Radiance Fields
對(duì)象類別建模
FiG-NeRF: Figure Ground Neural Radiance Fields for 3D Object Category Modelling
NeRF-Tex: Neural Reflectance Field Textures
多尺度
Mip-NeRF 360: Unbounded Anti-Aliased Neural Radiance Fields
Mip-NeRF: A Multiscale Representation for Anti-Aliasing Neural Radiance Fields
模型重構(gòu)
NeAT: Learning Neural Implicit Surfaces with Arbitrary Topologies from Multi-view Images
UNISURF: Unifying Neural Implicit Surfaces and Radiance Fields for Multi-View Reconstruction
NeuS: Learning Neural Implicit Surfaces by Volume Rendering for Multi-view Reconstruction
Volume Rendering of Neural Implicit Surfaces
深度估計(jì)
NerfingMVS: Guided Optimization of Neural Radiance Fields for Indoor Multi-view Stereo
機(jī)器人
Differentiable Physics Simulation of Dynamics-Augmented Neural Objects
3D Neural Scene Representations for Visuomotor Control
Vision-Only Robot Navigation in a Neural Radiance World
大型場(chǎng)景
Switch-NeRF: Learning Scene Decomposition with Mixture of Experts for Large-scale Neural Radiance Fields
Block-NeRF: Scalable Large Scene Neural View Synthesis
掃碼添加小享,回復(fù)“NeRF”
免費(fèi)獲取全部118篇論文資料合集
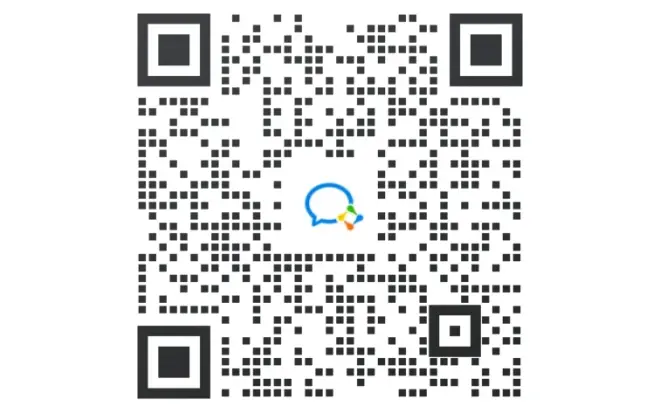