清華大佬終于把【PyTorch教程】給講明白了!全程干貨無廢話,整整150集,還
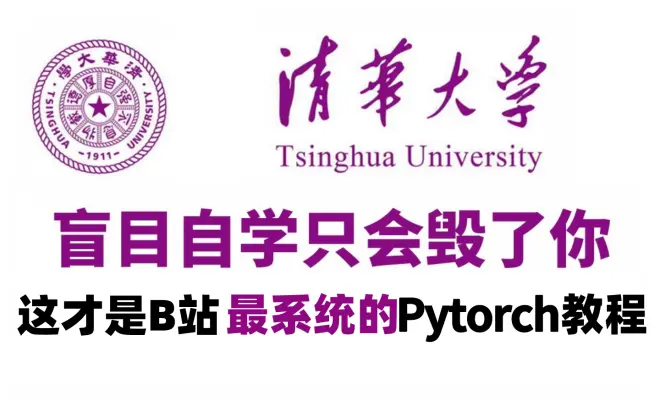
激活函數(shù):
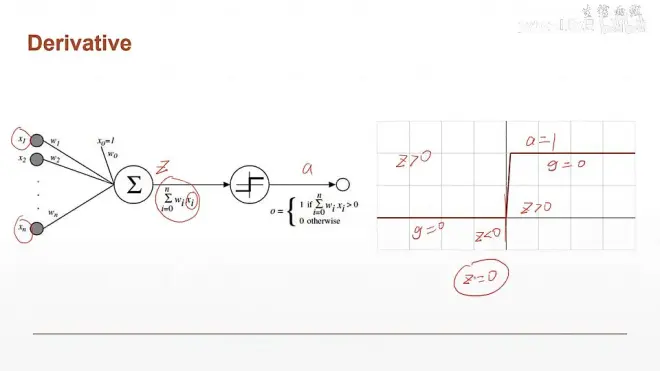
上面的激活函數(shù)是不可導(dǎo)的,所以不能用梯度下降算法
其它激活函數(shù):
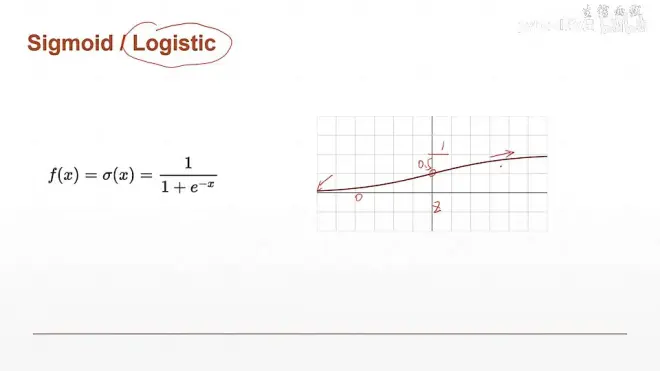
sigmoid函數(shù)導(dǎo)數(shù)推導(dǎo):
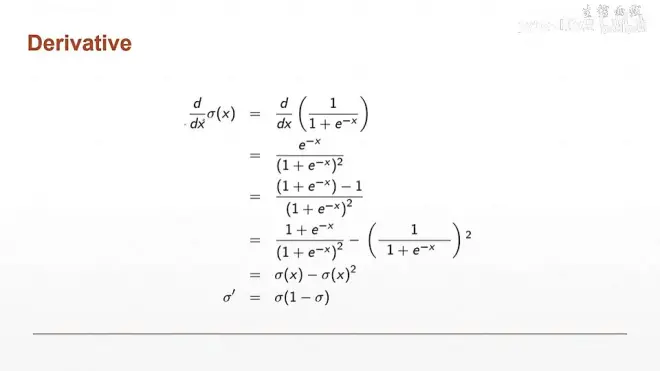
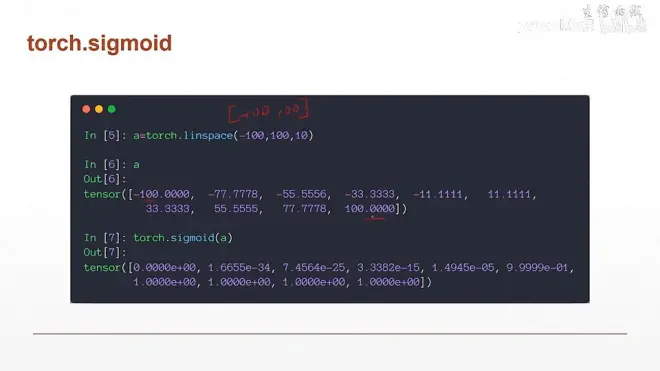
TanH激活函數(shù):在RNN中使用的多
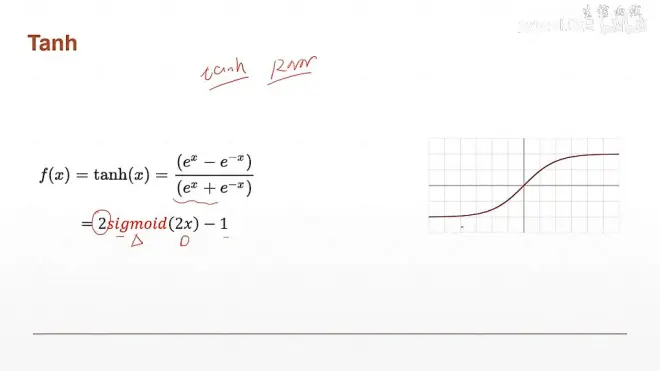
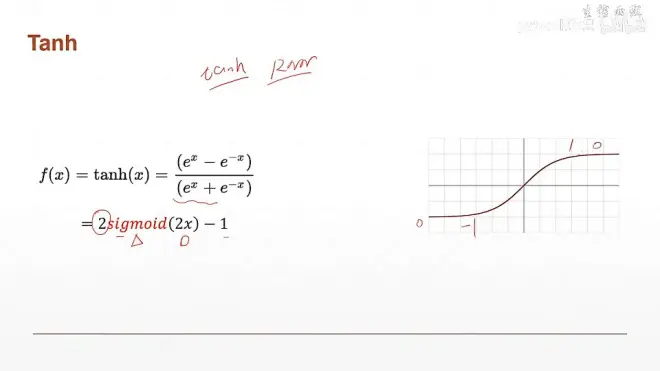
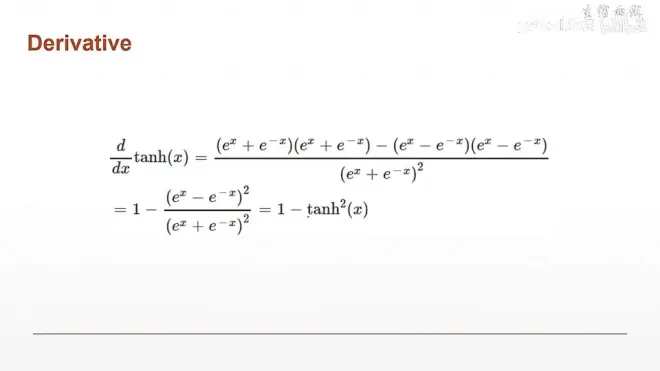
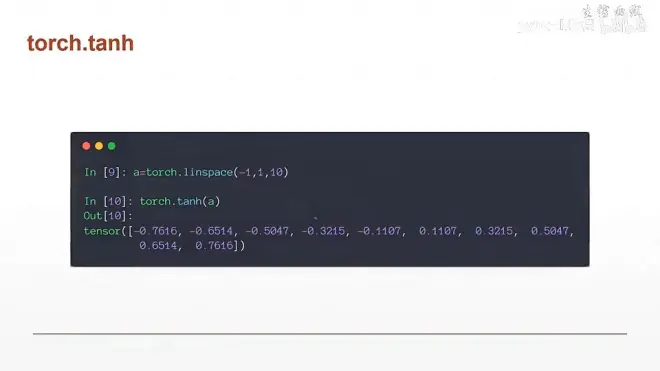
ReLU激活函數(shù):
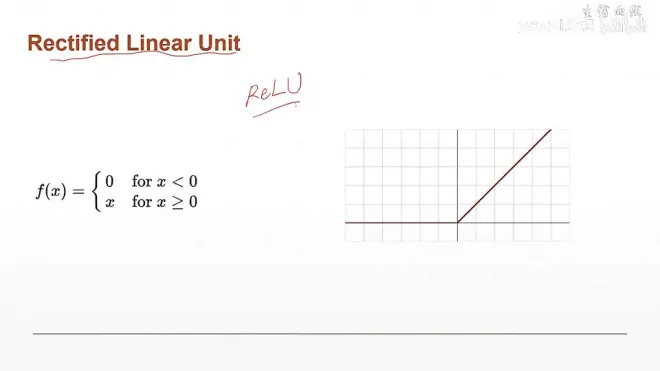
Relu函數(shù)導(dǎo)數(shù)的計(jì)算過程很簡單,大于0時(shí)為1,小于0時(shí)為零
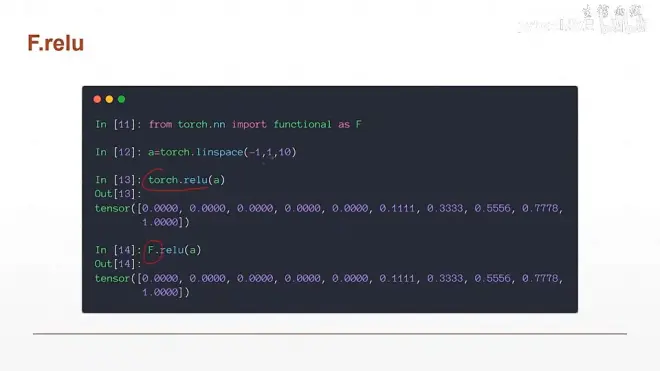
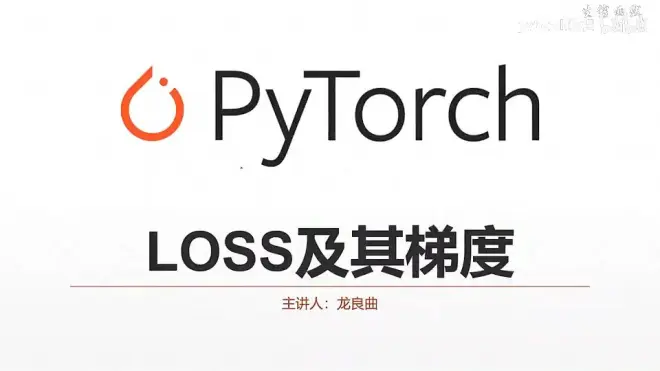
MSE (Mean Squared Error):均方差
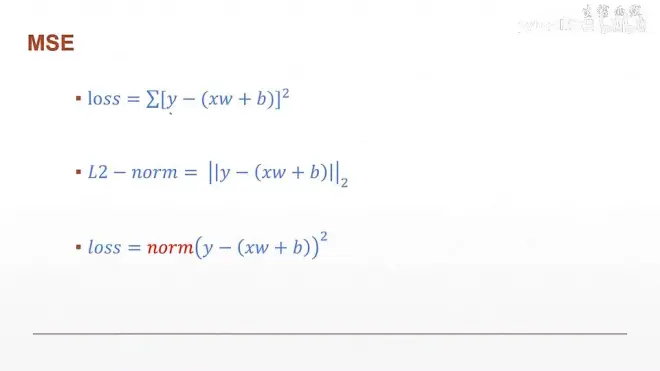
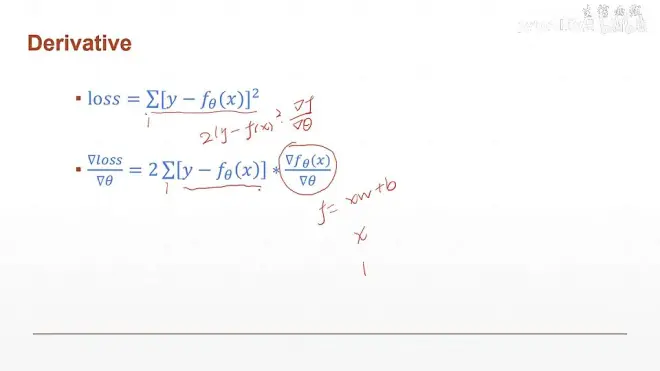
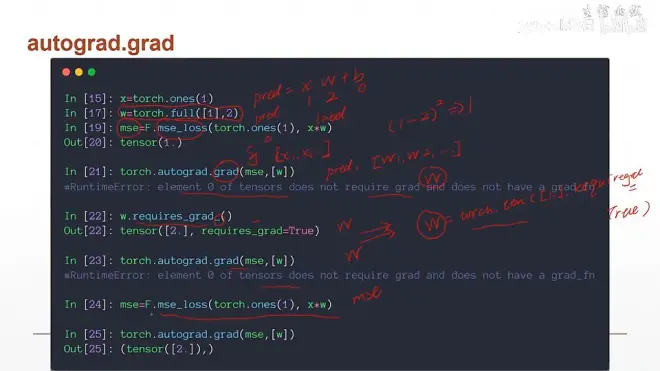
.backward()計(jì)算梯度:
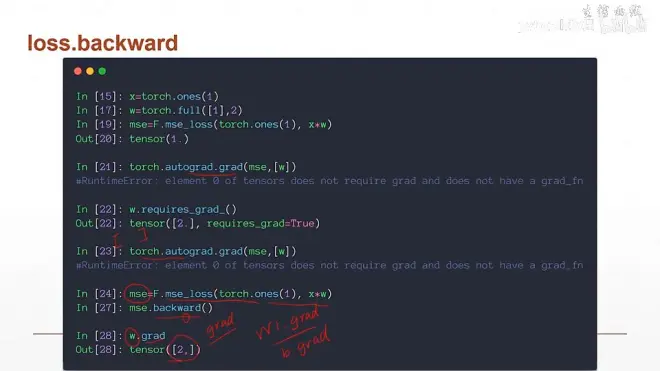
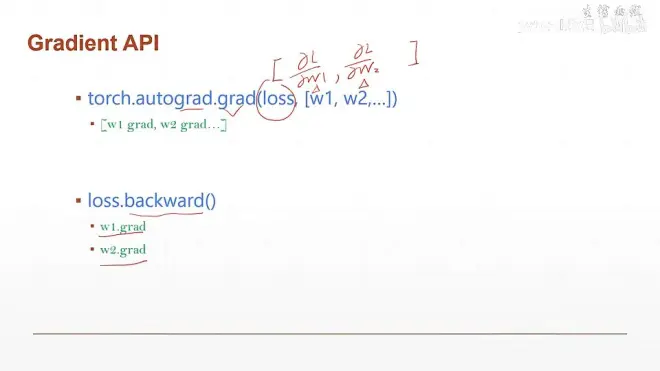
用于分類的激活函數(shù):Softmax
soft version of max 擴(kuò)大化結(jié)果差
返回值總和為1,概率
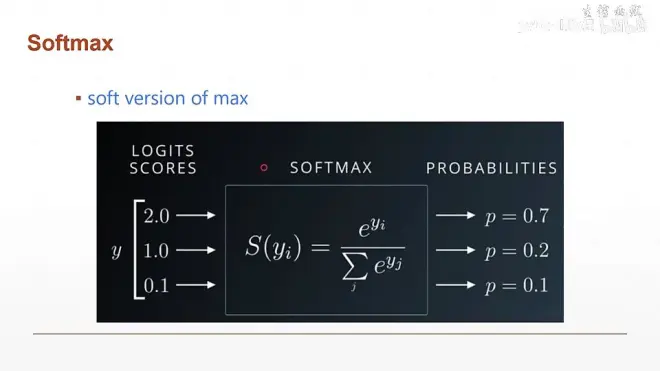
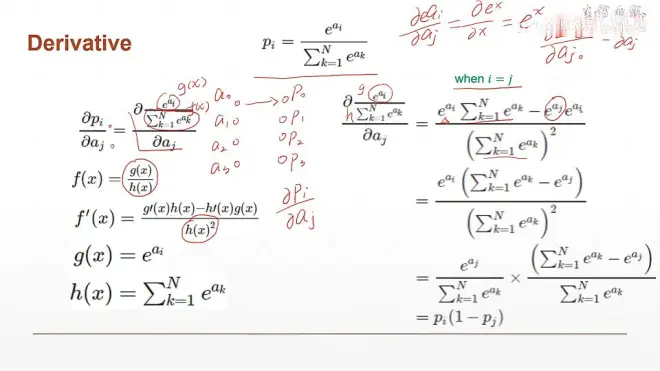
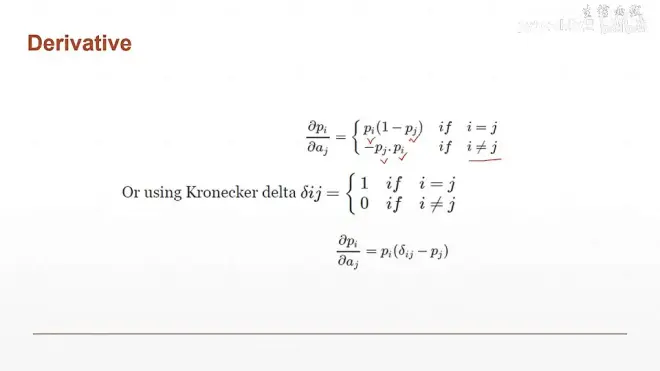
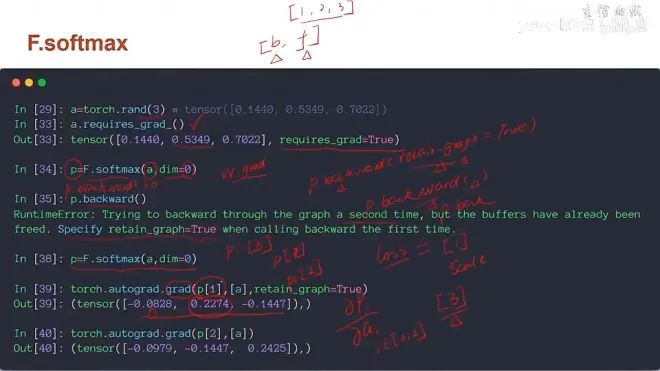
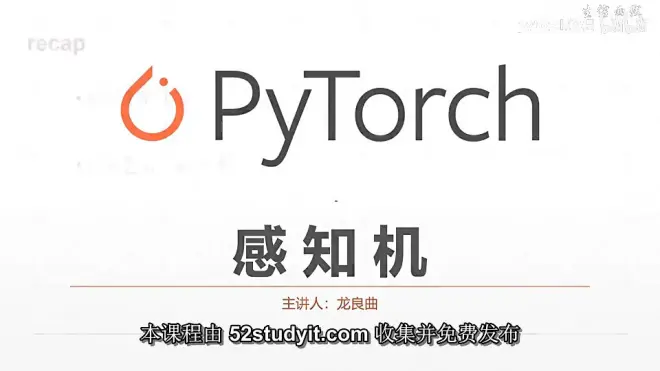
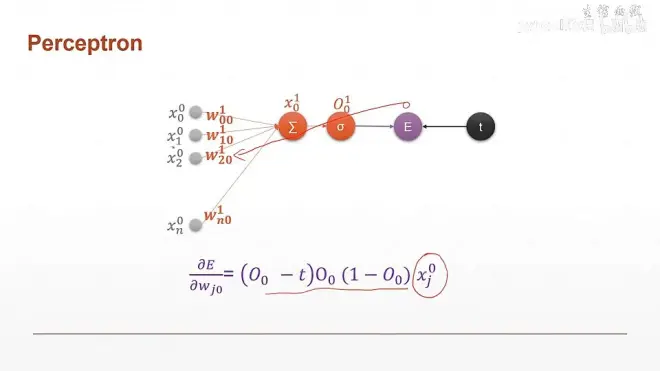
上圖中l(wèi)amda 為激活函數(shù)的值,E為mse均方差,t為真實(shí)值
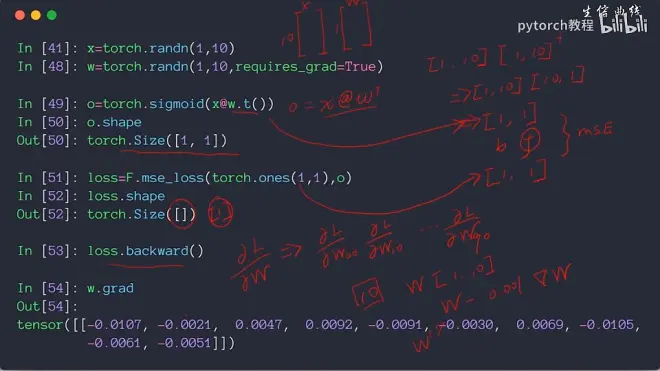
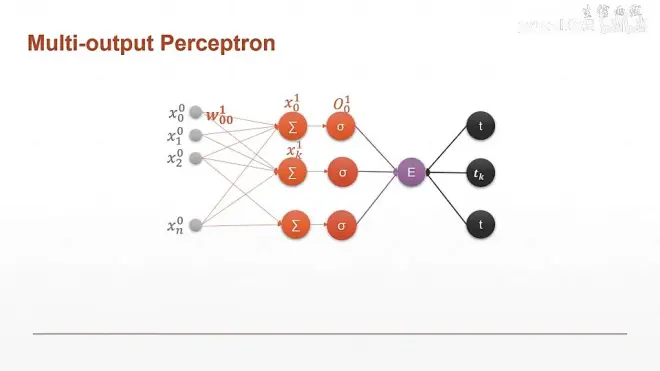
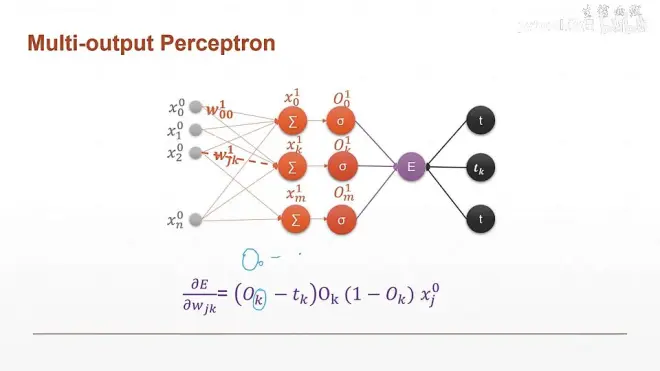
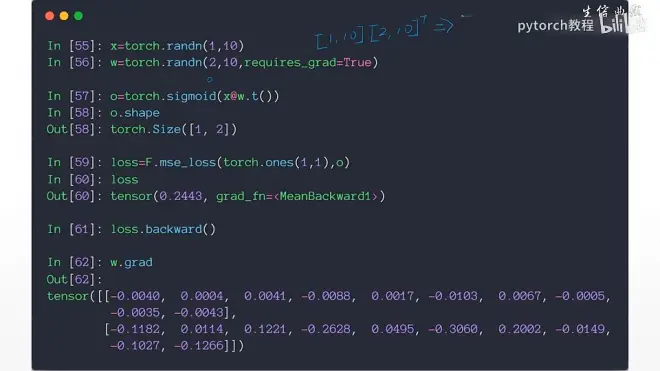
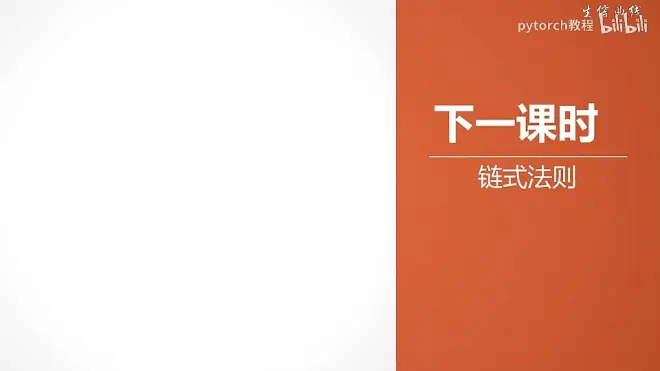
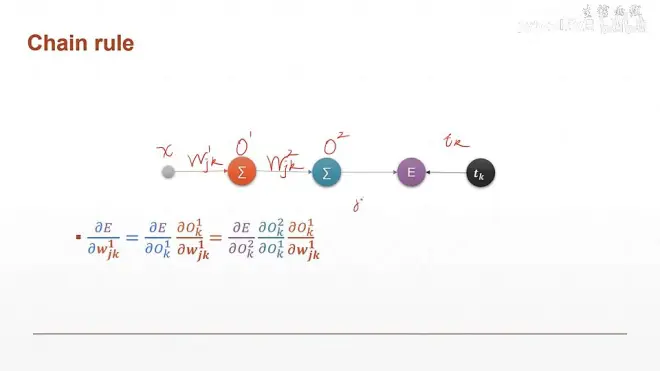
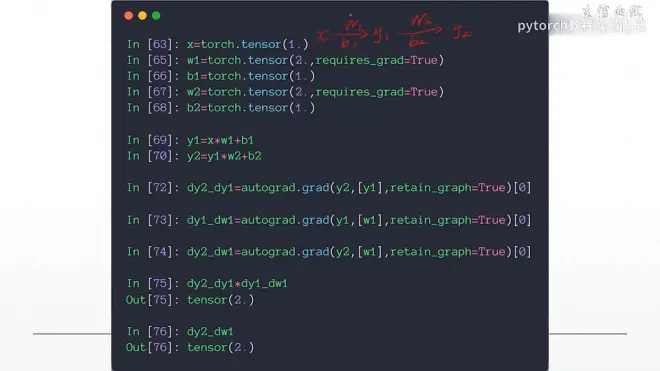
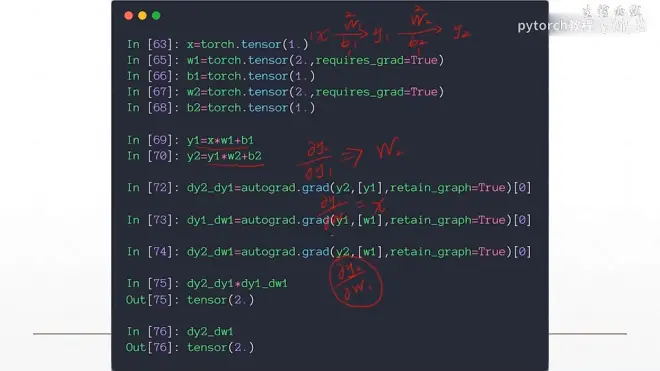
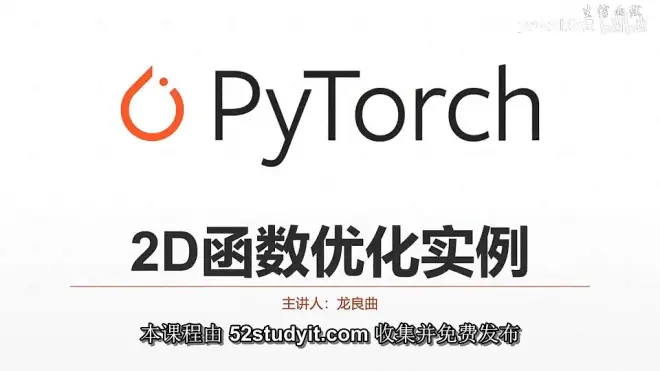
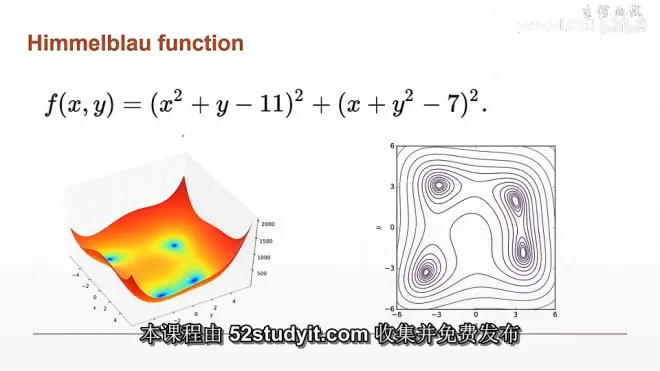
上面的函數(shù)一般用來判斷優(yōu)化器的效果,函數(shù)有四個(gè)局部最優(yōu)解,結(jié)果都是0
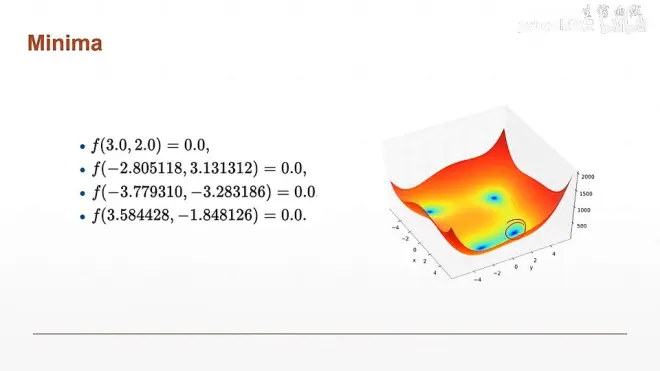
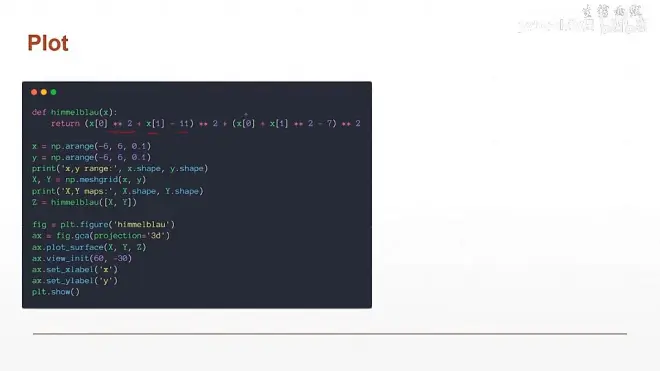
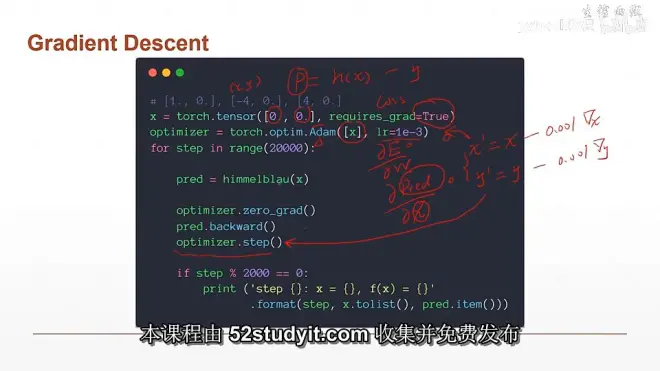
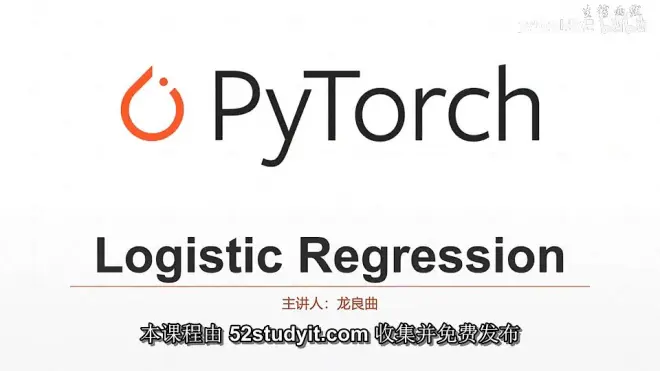
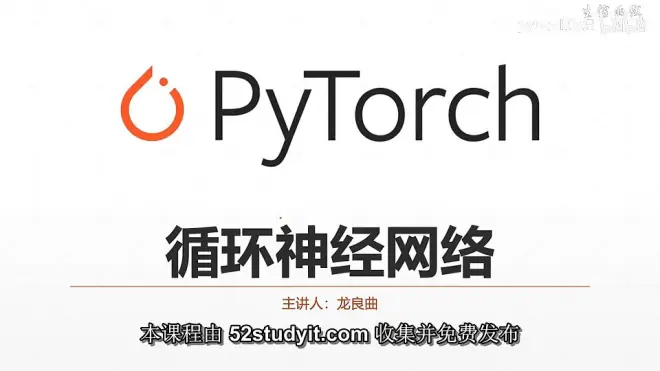
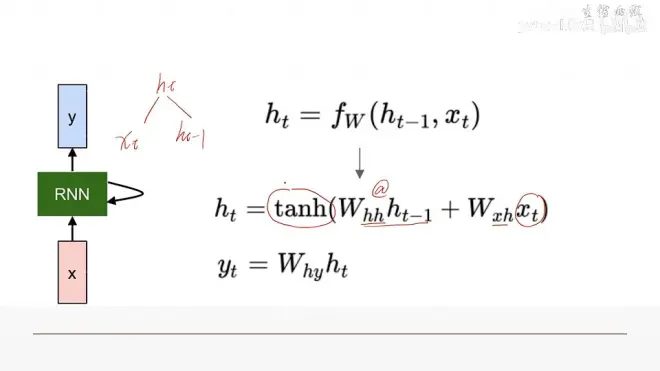
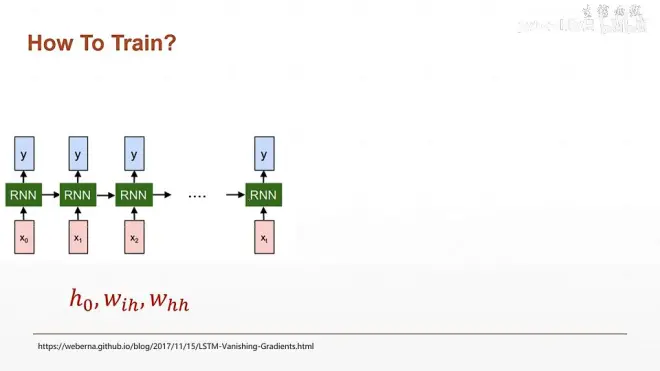
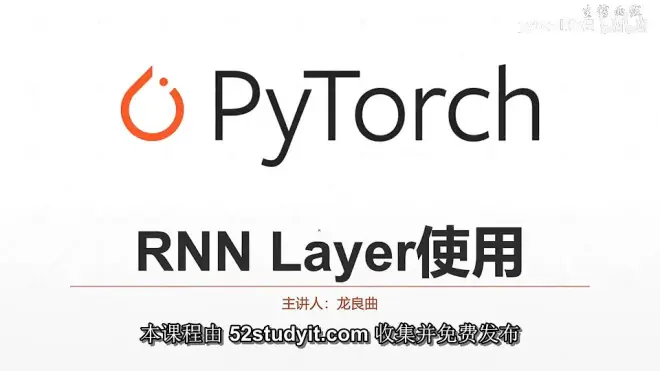
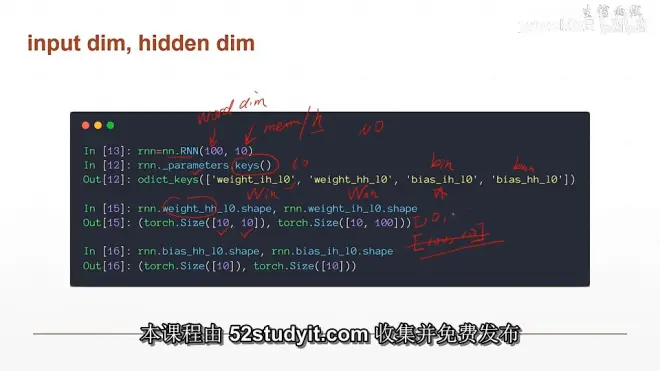
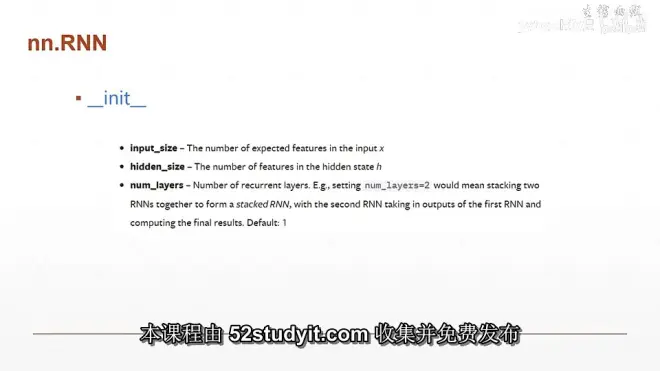
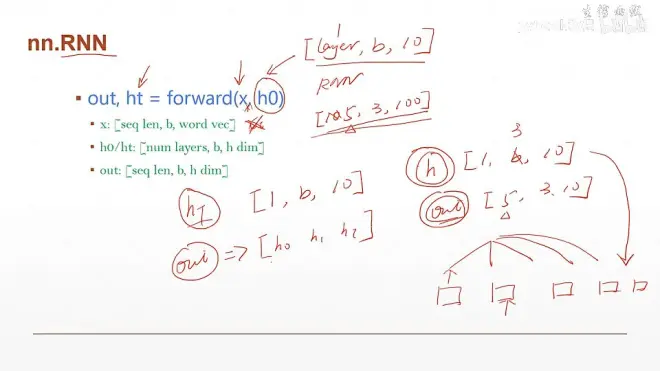
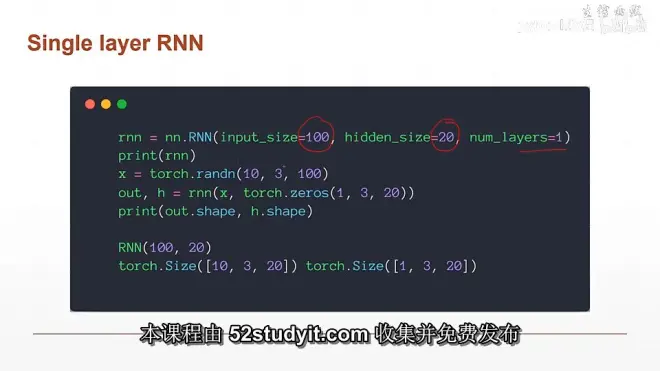
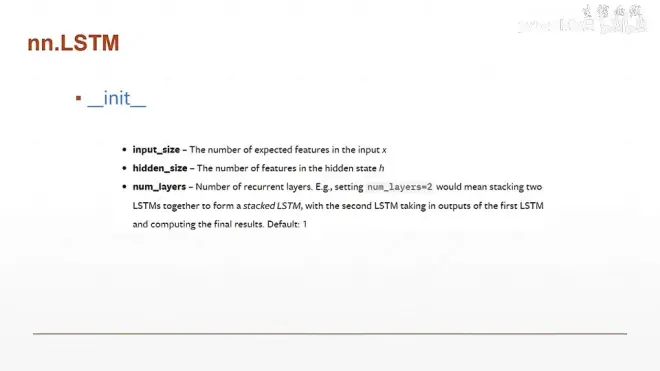
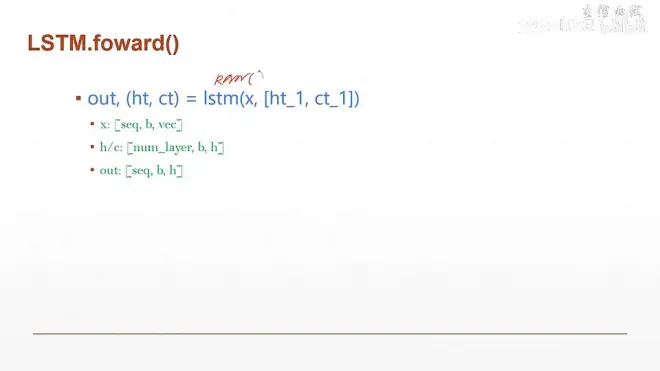
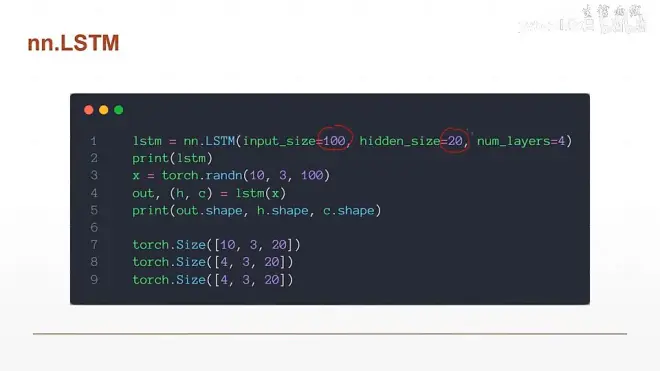
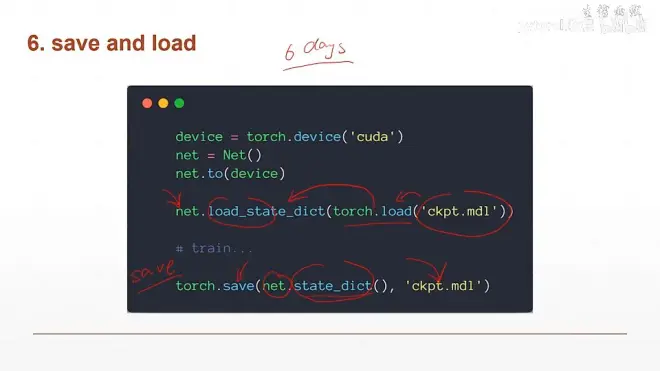
熵: entorpy
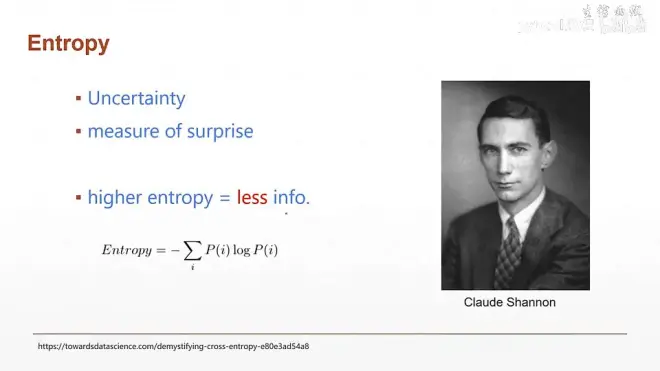
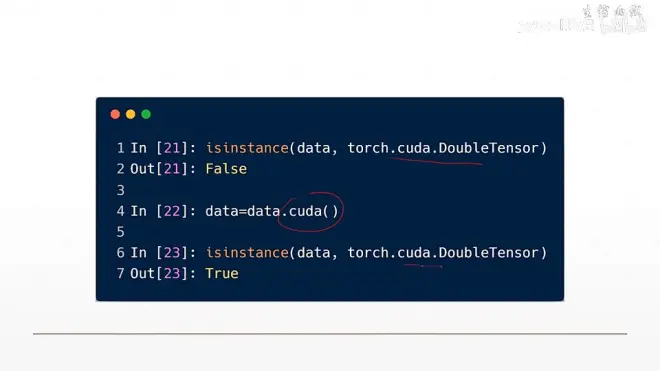
標(biāo)量:
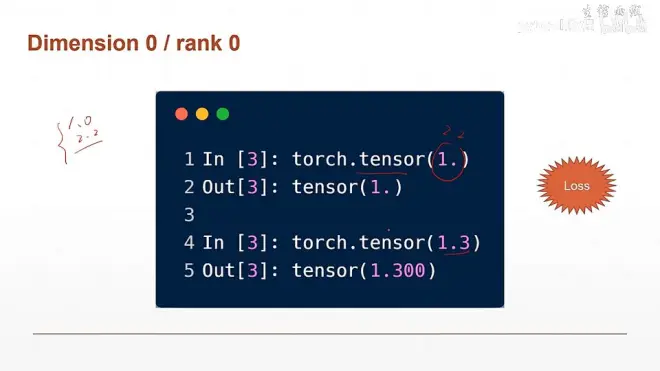
tensor:
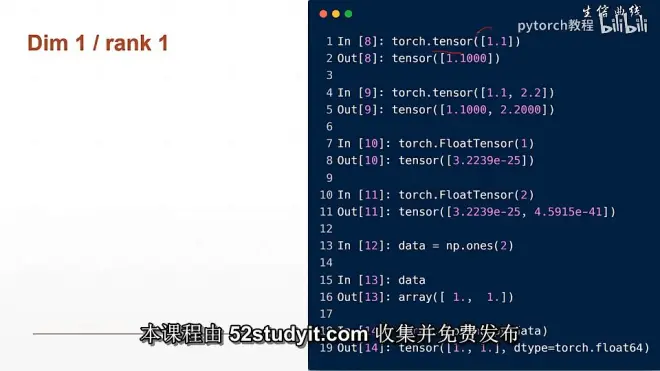
a.shape //shape 是屬性值
a.shape[0] //返回第維度為0的大小
a.size() //size() 是函數(shù)
a.size(0) //返回維度為0的大小
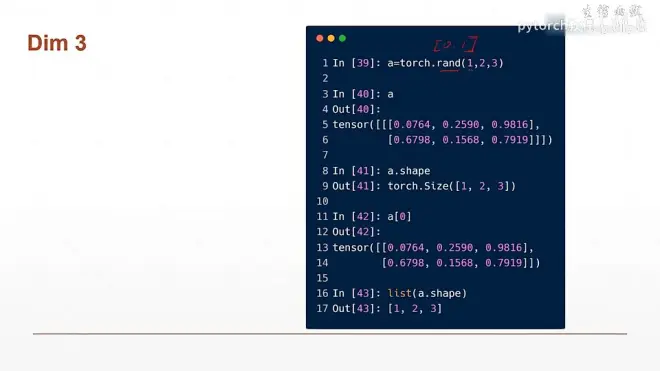
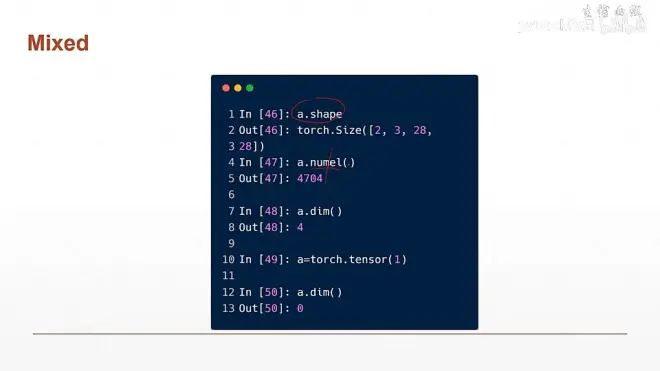
a. numel() //返回tensor的大小
a.dim() //返回維度的大小
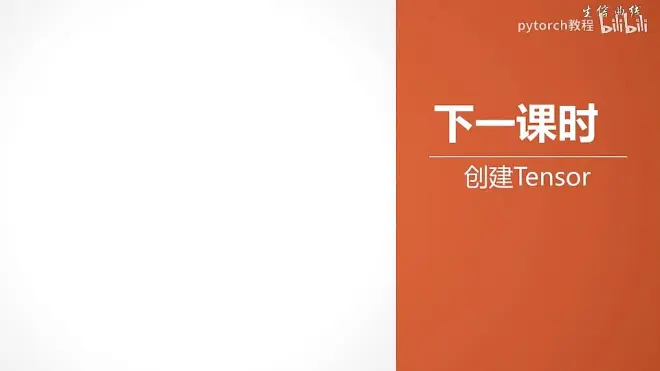
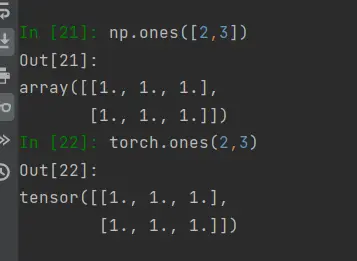
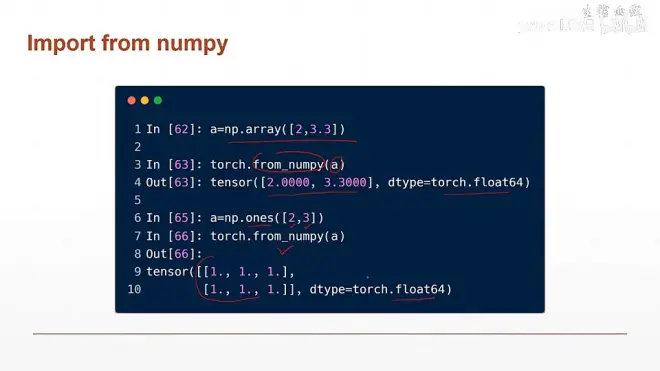
torch.tensor()
torch.Tensor()
注意:小寫tensor接收數(shù)據(jù),Tensor接收維度
torch.tensor([2,3]) //將[2,3]轉(zhuǎn)換成tensor類型
torch.Tensor(2,3) //生成一個(gè)2x3的數(shù)據(jù)類型
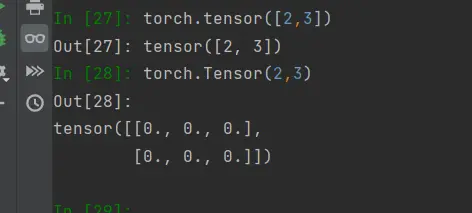
生成未初始化數(shù)據(jù):
Torch.empty(2,3)
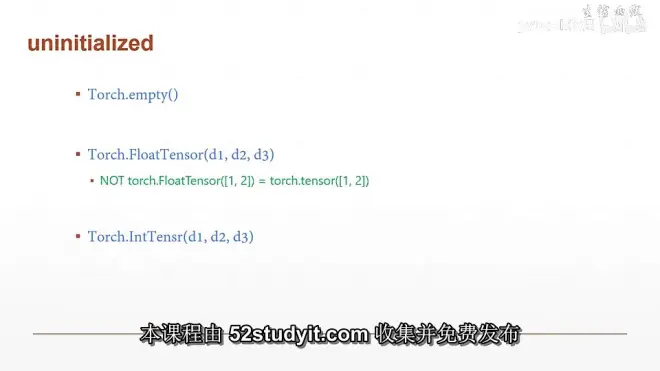
tensor()函數(shù)的 默認(rèn)類型為FloatTensor
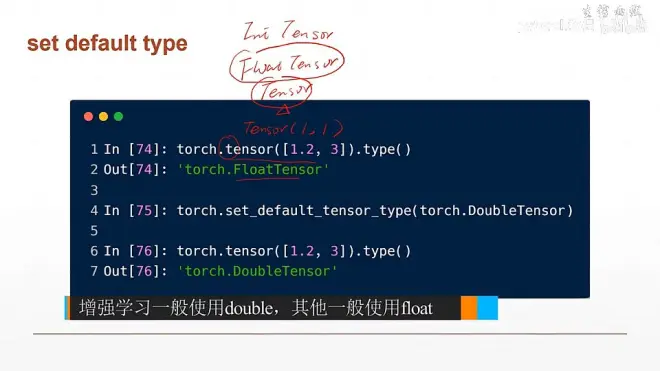
設(shè)置默認(rèn)類型
torch.set_default_tensor_type(torch.DoubleTensor)

設(shè)置隨機(jī)初始化:
rand() //采用0~1均勻隨機(jī)抽樣
torch.rand(3,3 ) //生成【0,1)之間的3x3tensor
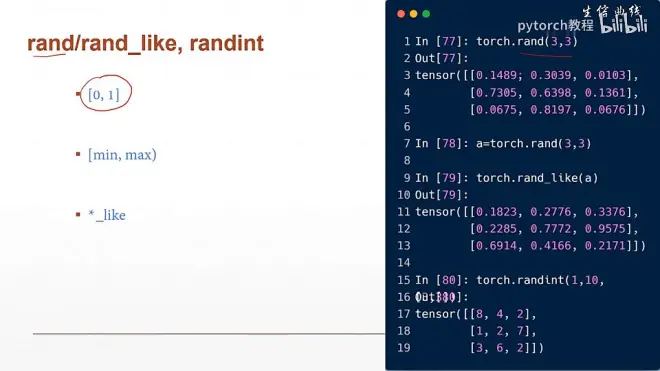
torch.rand_like(a) //生成和a的shape相同的樣本
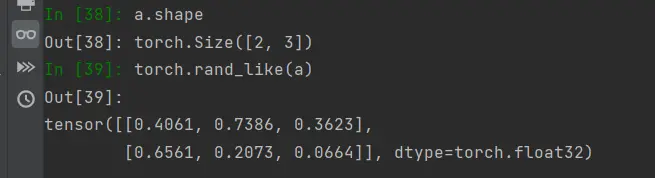
randint() 函數(shù) [min,max)
torch.randint(min,max,[shape])
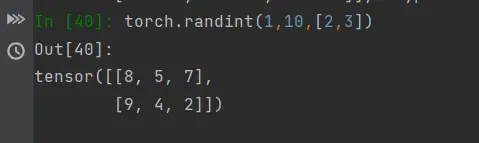
torch.randn() //正態(tài)分布(0,1) 均值為0,方差為1
torch.randn(3,3) // 生成3X3的矩陣
隨機(jī)正態(tài)分布的表示法:
torch.normal(mean=xx,std=xx,)
mean :為均值, std為方差
ex:
torch.normal(mean=torch.full([10],0), std=torch.arange(1,0,-0.1))
//輸出1x10 的一維tensor , 均值是[0,0,0,0,0,0,0,0,0,0], 方差是[1,0.9,0.8,,,,,0]
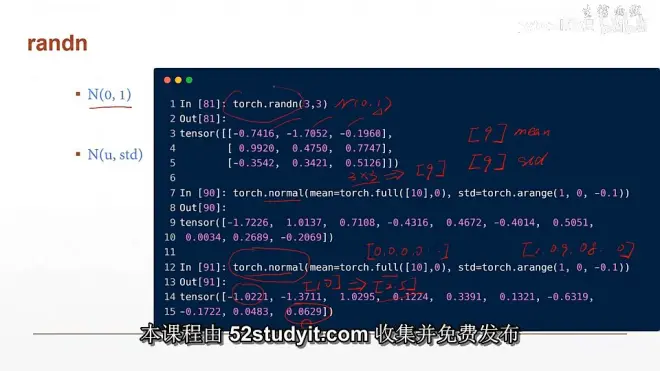
torch.full() :
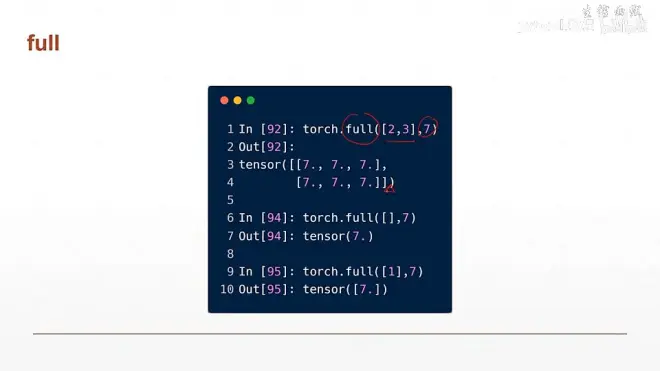
生成等差數(shù)列:
torch.arange()
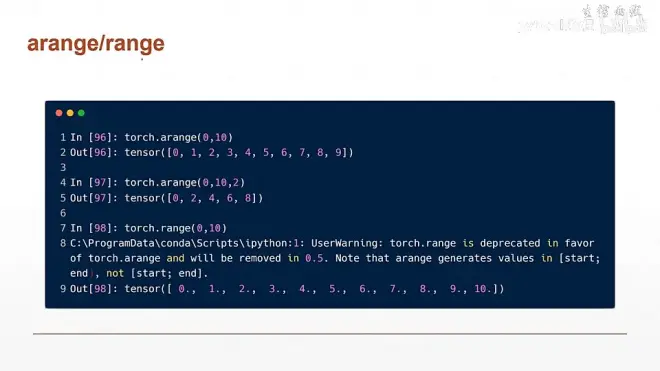
torch.linspace(0,10,steps=10)
//從[0,10】中等分出10個(gè)數(shù)
torch.logspace(0,-1,steps=10)
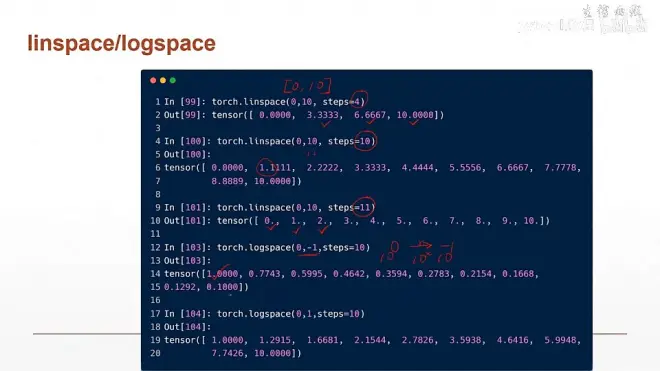
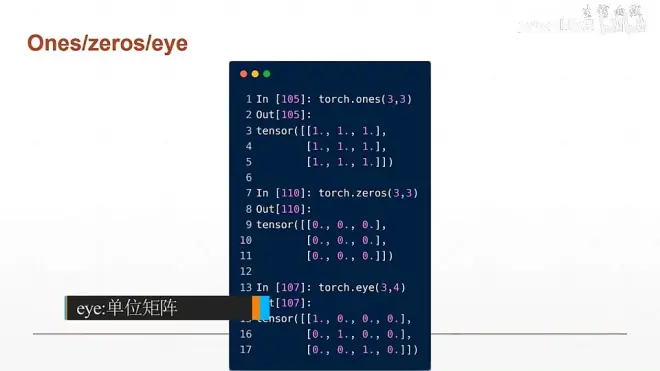
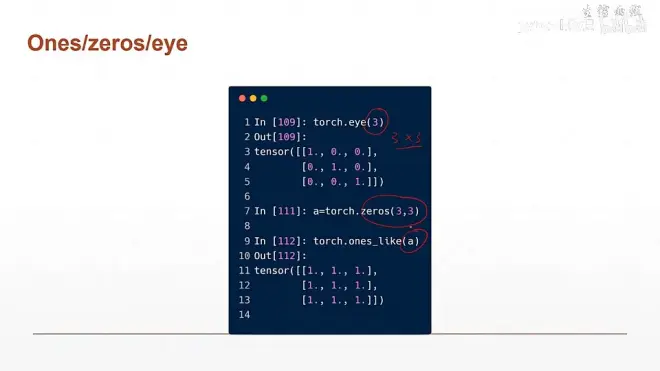
torch.randperm(10) //生成[0,9) 的隨機(jī)打散的數(shù)

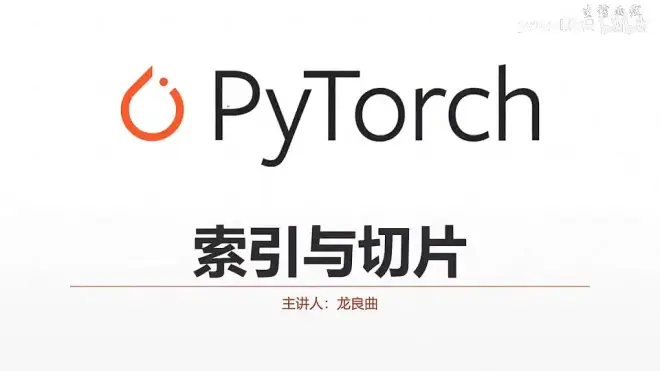
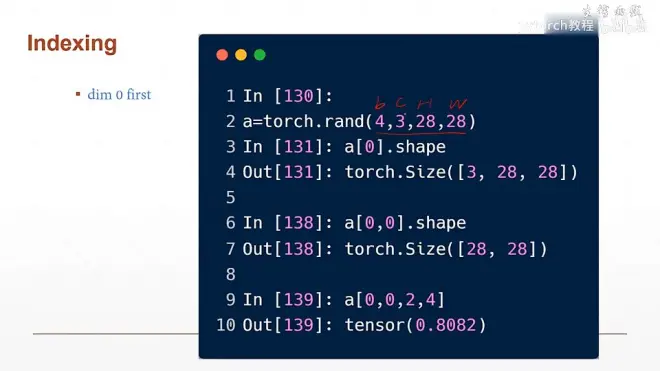
28x28的三通道圖片4張
a.shape -> torch.size([4,3,28,28])
a[:2].shape //表示前兩張圖片
torch.size([2,3,28,28])
a[:2,:1,:,:] // 表示前兩張圖片的第0個(gè)通道的所有數(shù)據(jù)
a[:2,1:,:,:] //表示前兩張圖片中從第1通道到最末尾通道的所有數(shù)據(jù)
a[:2,-1:,:,:;] //表示前兩張圖片中最后一個(gè)通道所有數(shù)據(jù)
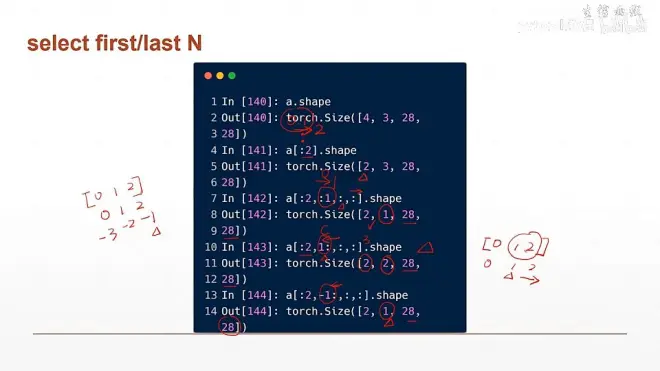
[0:28:2] //表示從【0,28)的沒隔2個(gè)數(shù)采樣一次
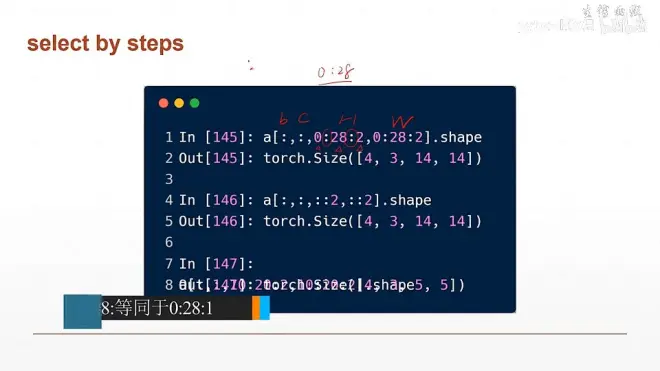
torch.index_select():
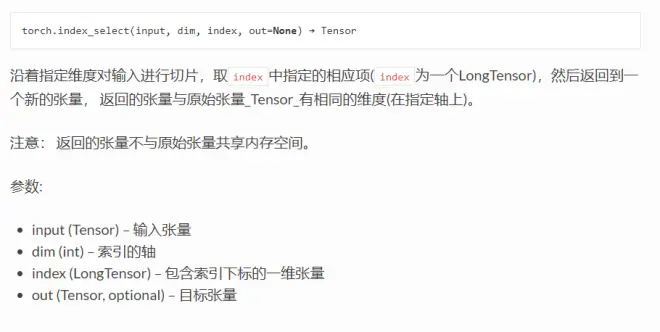
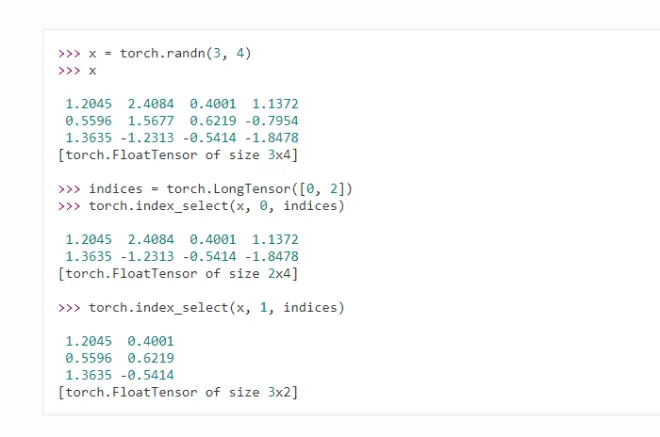
a[...].shape //三點(diǎn)表示任意的維度
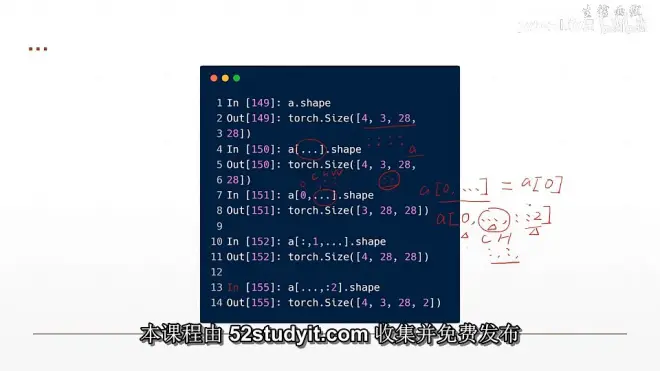
x.ge(0.5) // 返回x中大于等于0.5的數(shù)
torch.masked_select()
https://pytorch-cn.readthedocs.io/zh/latest/package_references/torch/
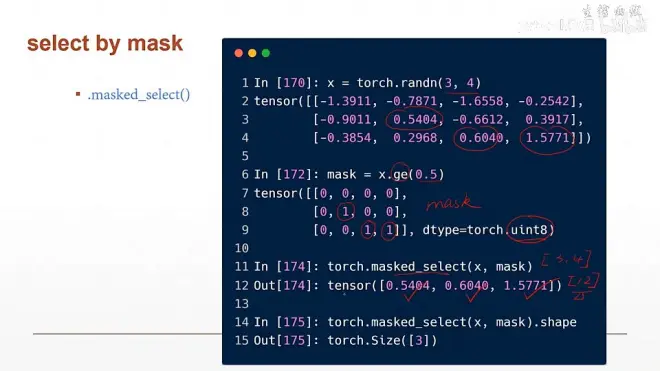
維度變換:
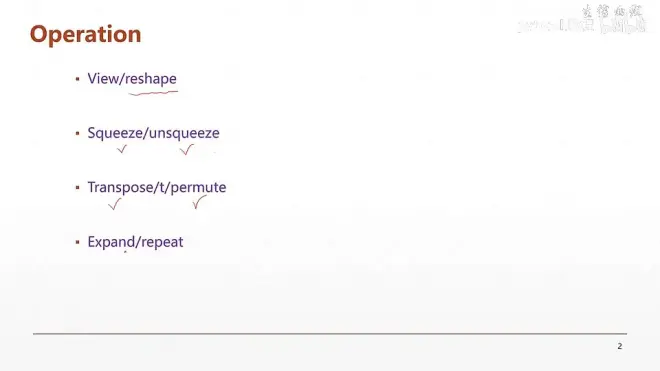
View 和 reshape 可以通用, reshape是后加的
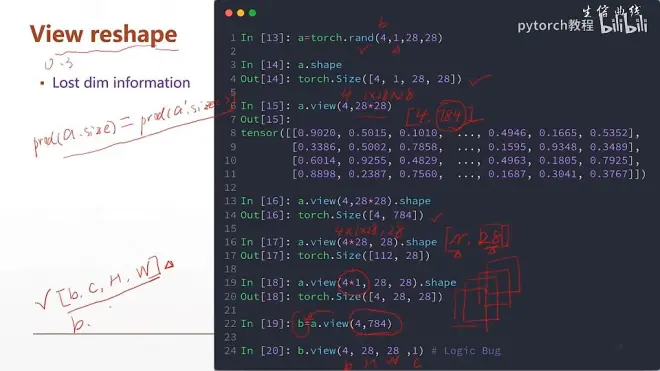
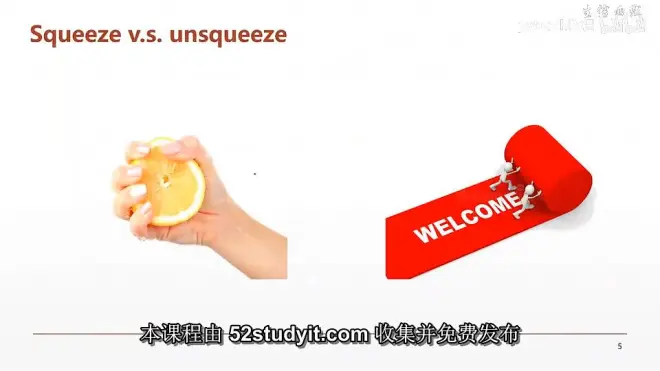
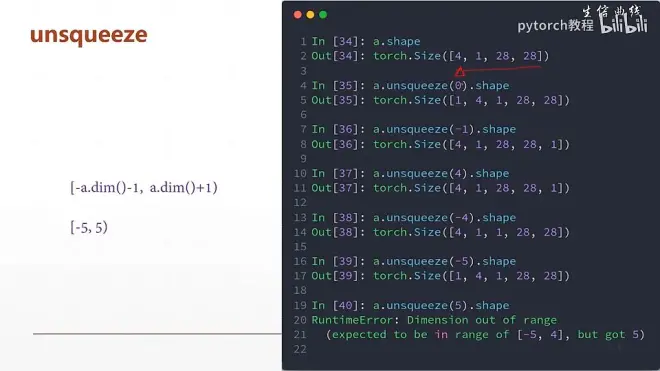
unsqueeze只增加了數(shù)據(jù)的維度不改變數(shù)值
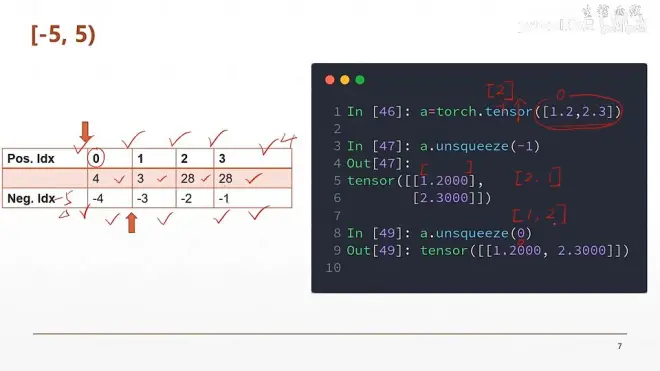
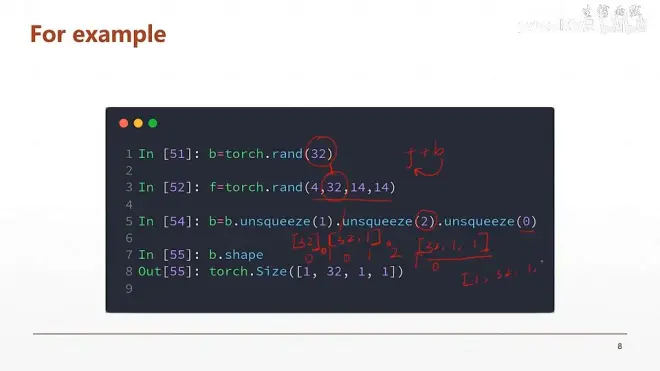
squeeze() //刪除dim=1的所有維度b=torch.randn(1,32,1,1)
b.squeeze().shape -> torch.size([32])
b.squeeze(0).shape ->torch.size([32,1,1])
b.squeeze(-1).shape ->torch.size([1,32,1])
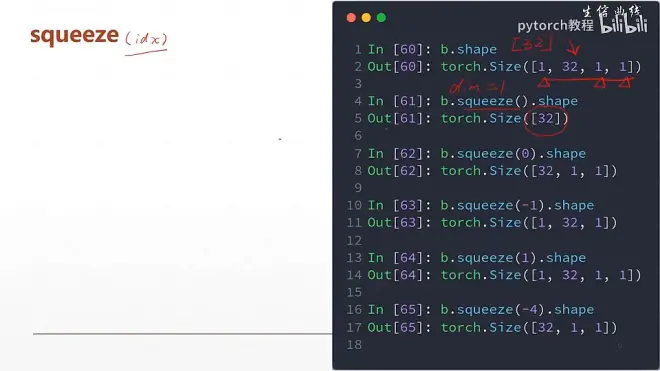
維度擴(kuò)展操作:
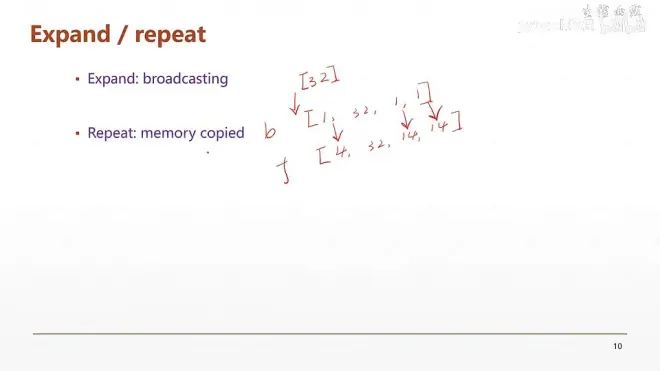
expand 不會主動復(fù)制數(shù)據(jù),節(jié)約內(nèi)存
Repead 會復(fù)制數(shù)據(jù)
expand操作要求,只能在相同的維度上驚醒擴(kuò)展,
[1,32,1,1] -> [4,32,14,14]
相同維度上只能從1到多維
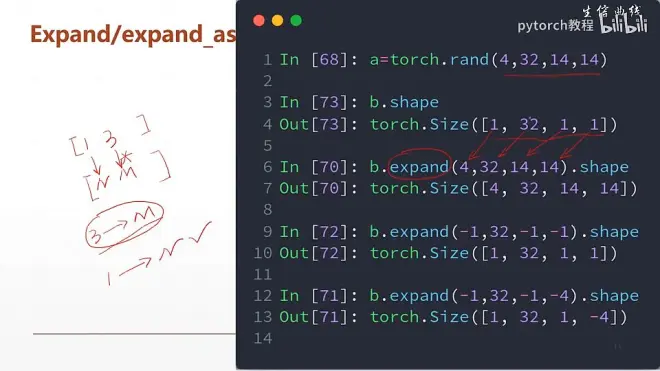
上述例子中填入-1表示保存相同的維度
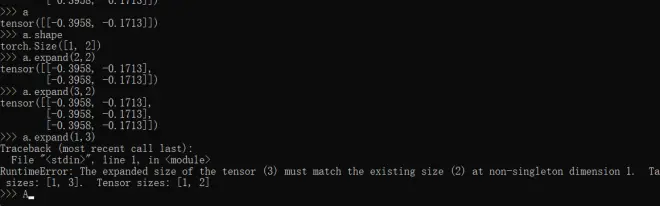
repeat() : 中的參數(shù)表示在每個(gè)維度上的重復(fù)次數(shù)
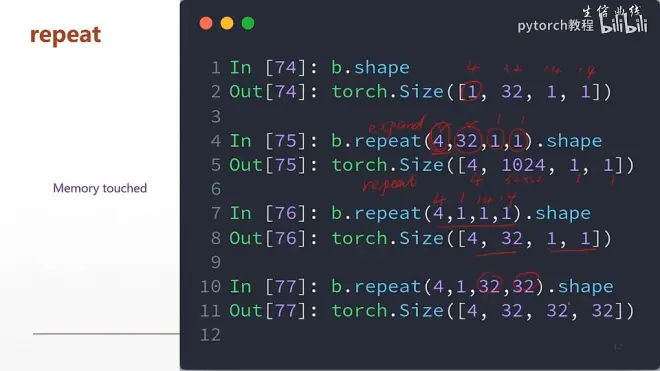
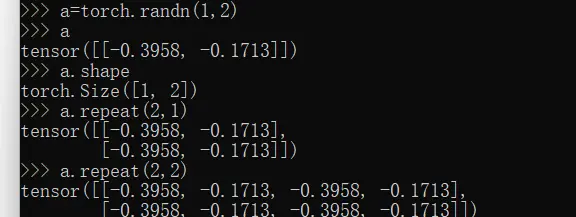
t() 轉(zhuǎn)置操作
只能用作二維中
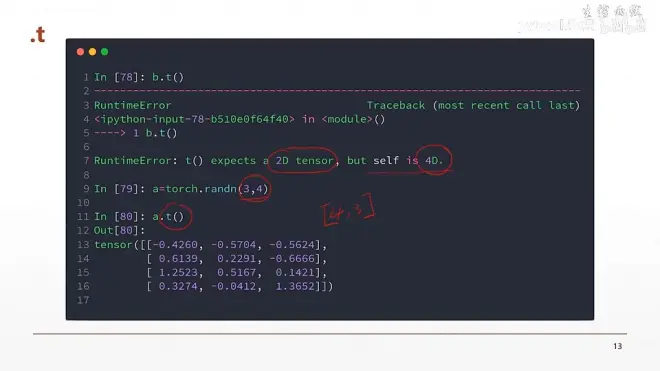
transpose() 進(jìn)行維度轉(zhuǎn)換
transpose()之后數(shù)據(jù)是不連續(xù)的,所以要用
.contiguous() 函數(shù)讓它連續(xù)
transpose(1,2) , //表示將第一維和第2維進(jìn)行交換
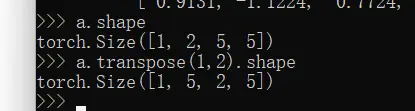
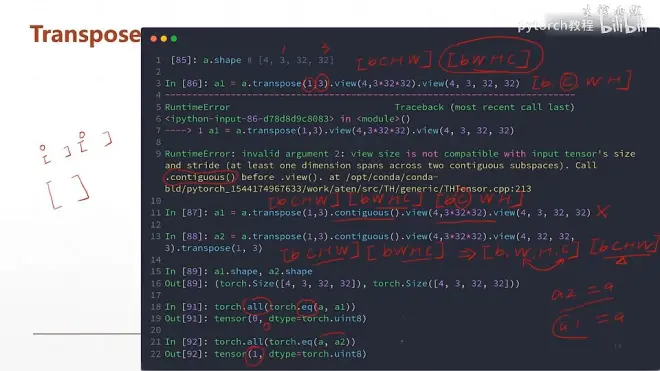
permute進(jìn)行維度轉(zhuǎn)換:
放入的值是原來的維度dim
eg,
b=torch.rand(4,3,28,32)
b.permute(0,2,3,1).shape ->[4,28,32,3]
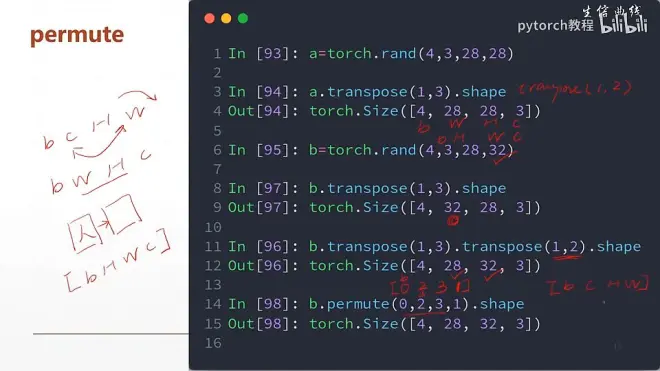
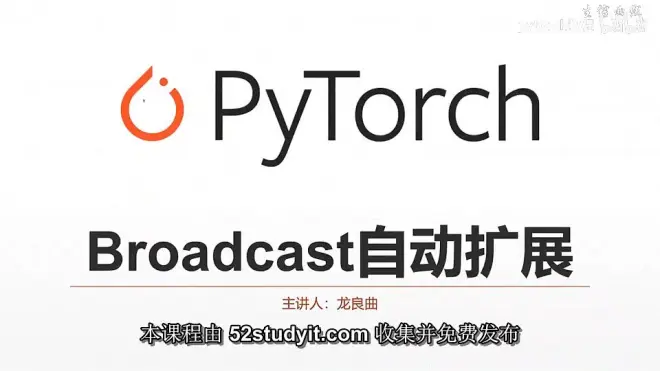