【中英雙語】部署AI的正確方式
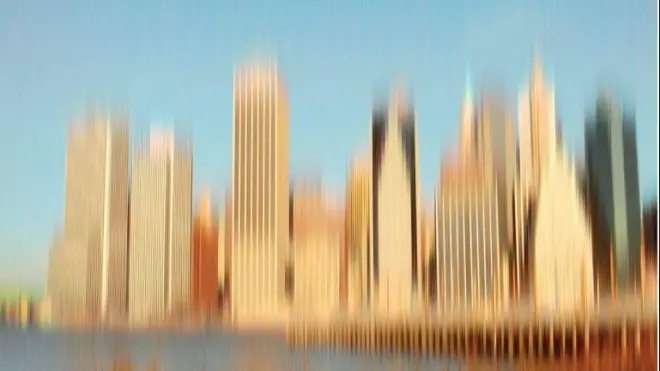
長期以來,專家一直都鼓勵人們“發(fā)揮自己的長處”。我們怎么會不想把自身最強的能力展示出來呢?但是這樣的事卻是談何容易。之所以這么說,不是因為我們很難辨識出自己擅長什么,而是因為通常情況下,我們低估了自己憑著本能就做得很好的事情。? ?
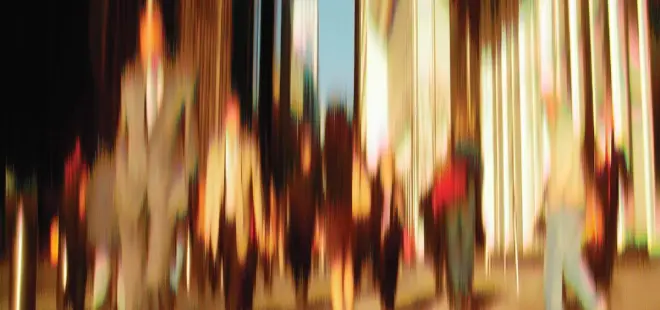
2018年勞動力研究院(Workforce Institute)針對8個工業(yè)國家的300多名管理者進行了一項調(diào)研,參與者中大多數(shù)認為人工智能是有價值的生產(chǎn)力工具。
這點不難理解:AI在處理速度、準確性和持續(xù)性(機器不會因疲倦犯錯)方面帶來了顯而易見的好處,很多職業(yè)人士都在使用AI。比如一些醫(yī)務人員利用AI輔助診斷,給出治療方案。
In a 2018 Workforce Institute?survey of?3,000 managers across eight industrialized nations, the majority of respondents described artificial intelligence as a valuable productivity tool.
It’s easy to see why: AI brings tangible benefits in processing speed, accuracy, and consistency (machines don’t make mistakes because they’re tired), which is why many professionals now rely on it. Some medical specialists, for example, use AI tools to help make diagnoses and decisions about treatment.
但參與者也表示擔心自己會被AI取代。擔心這件事的還不只是參與這項研究的管理者?!缎l(wèi)報》最近報道稱,英國600多萬員工擔心自己被機器取代。我們在各種會議和研討會上遇到的學者和高管也有同樣的擔心。AI的優(yōu)勢在一些人眼中更具負面色彩:如果機器能更好地完成工作,還要人類干嗎?
But respondents to that survey also expressed fears that AI would take their jobs. They are not alone. The?Guardian?recently reported that more than 6 million workers in the UK fear being replaced by machines. These fears are echoed by academics and executives we meet at conferences and seminars. AI’s advantages can be cast in a much darker light: Why would humans be needed when machines can do a better job?
這種恐懼感的蔓延表明,公司在為員工提供AI輔助工具時需要注意方式。2020年1月離職的埃森哲前首席信息官安德魯·威爾遜( Andrew Wilson)說,“企業(yè)如果更多地關(guān)注AI和人類如何互相幫助,可以實現(xiàn)的價值會更大。”埃森哲發(fā)現(xiàn),如果企業(yè)明確表示使用AI的目的是輔助而非取代員工,情況會比那些沒有設立這一目標或?qū)κ褂肁I的目的語焉不詳?shù)墓竞玫枚?,這種差別體現(xiàn)在多個管理生產(chǎn)率維度,特別是速度、延展性和決策有效性。
The prevalence of such fears suggests that organizations looking to reap the benefits of AI need to be careful when introducing it to the people expected to work with it. Andrew Wilson, until January 2020 Accenture’s CIO, says, “The greater the degree of organizational focus on people helping AI, and AI helping people, the greater the value achieved.” Accenture has found that when companies make it clear that they are using AI to help people rather than to replace them, they significantly outperform companies that don’t set that objective (or are unclear about their AI goals) along most dimensions of managerial productivity—notably speed, scalability, and effectiveness of decision-making.
換言之,AI就像加入團隊的新人才,企業(yè)必須使之發(fā)揮積極作用,而不是有意令其失敗。明智的企業(yè)會先給新員工一些簡單的任務,創(chuàng)造包容的環(huán)境,幫助他們積累實戰(zhàn)經(jīng)驗,并安排導師為其提供幫助和建議。這樣一來,新人可以在其他人負責更高價值的工作的時候?qū)W習。新人不斷累積經(jīng)驗,證明自身工作能力,導師逐步在更關(guān)鍵的決策上信任他們的意見。學徒逐漸成為合作伙伴,為企業(yè)貢獻技能和想法。
In other words, just as when new talent joins a team, AI must be set up to succeed rather than to fail. A smart employer trains new hires by giving them simple tasks that build hands-on experience in a noncritical context and assigns them mentors to offer help and advice. This allows the newcomers to learn while others focus on higher-value tasks. As they gain experience and demonstrate that they can do the job, their mentors increasingly rely on them as sounding boards and entrust them with more-substantive decisions. Over time an apprentice becomes a partner, contributing skills and insight.
我們認為這一方式也適用于人工智能。下文我們將結(jié)合自身及其他學者針對AI和信息系統(tǒng)應用的研究和咨詢工作,以及公司創(chuàng)新及工作實踐方面的研究,提出應用AI的一種方式,分四個階段。通過這種方式,企業(yè)可以逐步培養(yǎng)員工對AI的信任(這也是接納AI的關(guān)鍵條件),致力于構(gòu)建人類和AI同時不斷進步的分布式人類-AI認知系統(tǒng)。很多企業(yè)都已嘗試過第一階段,部分企業(yè)進行到了第二、三階段;迄今為止第四階段對多數(shù)企業(yè)來說還是“未來式”,尚處在早期階段,但從技術(shù)角度來說可以實現(xiàn),能夠為利用人工智能的企業(yè)提供更多價值。
We believe this approach can work for artificial intelligence as well. In the following pages we draw on our own and others’ research and consulting on AI and information systems implementation, along with organizational studies of innovation and work practices, to present a four-phase approach to implementing AI. It allows enterprises to cultivate people’s trust—a key condition for adoption—and to work toward a distributed human-AI cognitive system in which people and AI?both?continually improve. Many organizations have experimented with phase 1, and some have progressed to phases 2 and 3. For now, phase 4 may be mostly a “future-casting” exercise of which we see some early signs, but it is feasible from a technological perspective and would provide more value to companies as they engage with artificial intelligence.
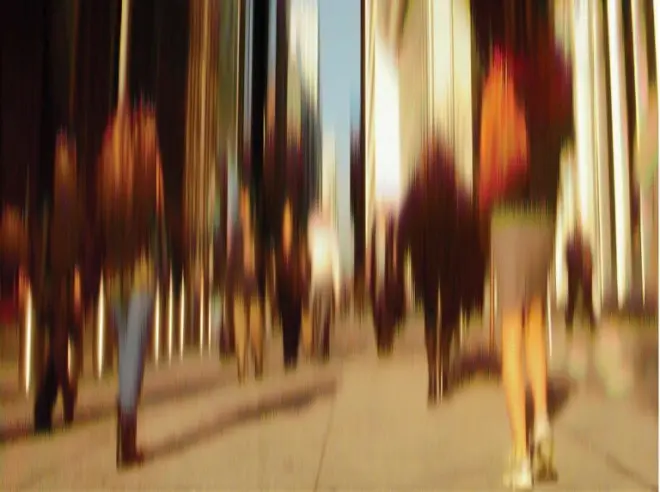
第一階段??助手
普及人工智能的第一階段和培訓助手的方式十分相似。你教給新員工一些關(guān)于AI的基本規(guī)則,將自己手頭上一些基礎但耗時的工作(如填寫網(wǎng)絡表格或者匯總文檔)分配給他,這樣你就有時間處理更重要的工作內(nèi)容。受訓者通過觀察你不斷學習,完成工作,提出問題。
Phase 1: The Assistant
This first phase of onboarding artificial intelligence is rather like the process of training an assistant. You teach the new employee a few fundamental rules and hand over some basic but time-consuming tasks you normally do (such as filing online forms or summarizing documents), which frees you to focus on more-important aspects of the job. The trainee learns by watching you, performing the tasks, and asking questions.
AI助手的常見任務之一是整理數(shù)據(jù)。20世紀90年代中期,一些提供推薦系統(tǒng)的企業(yè)幫助用戶過濾數(shù)千種產(chǎn)品,找到他們最需要的——亞馬遜和奈飛在應用這項技術(shù)方面處于領先地位。
One common task for AI assistants is sorting data. An example is the recommendation systems companies have used since the mid-1990s to help customers filter thousands of products and find the ones most relevant to them—Amazon and Netflix being among the leaders in this technology.
現(xiàn)在越來越多的商業(yè)決定要用到這種數(shù)據(jù)分類。例如,資產(chǎn)組合經(jīng)理在決定投資哪些股票時,要處理的信息量超出了人類的能力,而且還有源源不斷的新信息。軟件可以根據(jù)預先定義的投資標準迅速篩選股票,降低任務難度。自然語言處理技術(shù)可以搜集和某公司最相關(guān)的新聞,并通過分析師報告評估未來企業(yè)活動的輿論情緒。位于倫敦、成立于2002年的馬布爾資產(chǎn)管理公司(MBAM)較早將這項技術(shù)應用到職場。公司打造了世界一流的RAID(研究分析&信息數(shù)據(jù)庫)平臺幫助資產(chǎn)組合經(jīng)理過濾關(guān)于企業(yè)活動、新聞走勢和股票動向的海量信息。
More and more business decisions now require this type of data sorting. When, for example, portfolio managers are choosing stocks in which to invest, the information available is far more than a human can feasibly process, and new information comes out all the time, adding to the historical record. Software can make the task more manageable by immediately filtering stocks to meet predefined investment criteria. Natural-language processing, meanwhile, can identify the news most relevant to a company and even assess the general sentiment about an upcoming corporate event as reflected in analysts’ reports. Marble Bar Asset Management (MBAM), a London-based investment firm founded in 2002, is an early convert to using such technologies in the workplace. It has developed a state-of-the-art platform, called RAID (Research analysis & Information Database), to help portfolio managers filter through high volumes of information about corporate events, news developments, and stock movements.
AI還可以通過模擬人類行為提供輔助。用過谷歌搜索的人都知道,在搜索框輸入一個詞,會自動出現(xiàn)提示信息。智能手機的預測性文本也通過類似方式加快打字速度。這種用戶模擬技術(shù)出現(xiàn)在30多年前,有時叫做判斷引導,也可以應用在決策過程中。AI根據(jù)員工的決策歷史,判定員工在面對多個選擇時最有可能做出的選擇,并提出建議——幫助人類加快工作速度,而非代替人類完成工作。
Another way AI can lend assistance is to model what a human might do. As anyone who uses Google will have noticed, prompts appear as a search phrase is typed in. Predictive text on a smartphone offers a similar way to speed up the process of typing. This kind of user modeling, related to what is sometimes called?judgmental bootstrapping,?was developed more than 30 years ago; it can easily be applied to decision-making. AI would use it to identify the choice an employee is most likely to make, given that employee’s past choices, and would suggest that choice as a starting point when the employee is faced with multiple decisions—speeding up, rather than actually doing, the job.
我們來看一個具體的例子。航空公司員工在決定每架飛機的配餐數(shù)量時,會根據(jù)過往航班經(jīng)驗得出的假設進行計算,填寫餐飲訂單。計算錯誤會增加公司成本:預訂量不足可能激怒消費者不再選擇這家公司;超額預訂則代表多余的餐食將會被扔掉,而且飛機會因此儲備不必要的燃油。
Let’s look at this in a specific context. When airline employees are deciding how much food and drink to put on a given flight, they fill out catering orders, which involve a certain amount of calculation together with assumptions based on their experience of previous flights. Making the wrong choices incurs costs: Underordering risks upsetting customers who may avoid future travel on the airline. Overordering means the excess food will go to waste and the plane will have increased its fuel consumption unnecessarily.
這種情況下,人工智能可以派上用場。AI可以通過分析航空公司餐飲經(jīng)理過往的選擇,或者經(jīng)理設置的規(guī)則,預測他會如何下單。通過分析相關(guān)歷史數(shù)據(jù),包括該航線餐飲消耗量及航班乘客的歷史購物行為,每趟航線都可以定制這種“自動填寫”的“推薦訂單”。但是,就像預測性輸入一樣,人類擁有最后的決定權(quán),可以根據(jù)需要隨時覆蓋。AI僅僅通過模擬或預測他們的決策風格起到輔助作用。
An algorithm can be very helpful in this context. AI can predict what the airline’s catering manager would order by analyzing his or her past choices or using rules set by the manager. This “autocomplete” of “recommended orders” can be customized for every flight using all relevant historical data, including food and drink consumption on the route in question and even past purchasing behavior by passengers on the manifest for that flight. But as with predictive typing, human users can freely overwrite as needed; they are always in the driver’s seat. AI simply assists them by imitating or anticipating their decision style.
如果管理者通過這種方式逐步引入AI,應該不會太困難。我們已經(jīng)在生活中采用這種方式,網(wǎng)上填寫表格的時候允許AI自動補全信息。在職場,管理者可以制定AI助手在填表格時遵守的具體規(guī)則。很多企業(yè)在工作中使用的軟件(例如信用評級程序)正是人類定義的決策規(guī)則匯總。AI助手可以通過匯總管理者遵守這些規(guī)則的情境,進一步提煉規(guī)則。此類機器學習無需管理者采取任何行為,更不用“教導”AI助手。
It should not be a stretch for managers to work with AI in this way. We already do so in our personal lives, when we allow the autocomplete function to prefill forms for us online. In the workplace a manager can, for example, define specific rules for an AI assistant to follow when completing forms. In fact, many software tools currently used in the workplace (such as credit-rating programs) are already just that: collections of human-defined decision rules. The AI assistant can refine the rules by codifying the circumstances under which the manager actually follows them. This learning needn’t involve any change in the manager’s behavior, let alone any effort to “teach” the assistant.
第二階段??監(jiān)測者
下一步需要設定AI程序,為人類提供實時反饋。機器學習程序使得人類可以訓練AI,準確預測某種情境下(例如由于過度自信或疲勞導致的缺乏理性)用戶的決策。假如用戶即將做出的選擇有悖于過去的選擇記錄,系統(tǒng)會標記出矛盾之處。在決策量很大的工作中,人類員工可能因為勞累或分心出錯,這種方式可以起到很大的助益。
Phase 2: The Monitor
The next step is to set up the AI system to provide real-time feedback. Thanks to machine-learning programs, AI can be trained to accurately forecast what a user’s decision would be in a given situation (absent lapses in rationality owing to, for example, overconfidence or fatigue). If a user is about to make a choice that is inconsistent with his or her choice history, the system can flag the discrepancy. This is especially helpful during high-volume decision-making, when human employees may be tired or distracted.
心理學、行為經(jīng)濟學和認知科學的研究表明,人類的推理能力有限,而且有缺陷,特別是在商業(yè)活動中無處不在的統(tǒng)計學和概率性問題上。一些針對法庭審判決定的研究(本文作者之一陳參與了研究)表明,法官在午餐前更容易通過申請政治避難的案件;如果法官支持的美國職業(yè)橄欖球聯(lián)盟球隊在開庭前一天獲勝,他們在開庭當天的判罰會更輕;如果被告當天生日,法官會對其手下留情。很明顯,如果軟件可以告訴決策者他們即將做出的決定與之前有所矛盾,或者不符合純粹從司法角度分析的預測結(jié)果,也許更能體現(xiàn)公平公正。
Research in psychology, behavioral economics, and cognitive science shows that humans have limited and imperfect reasoning capabilities, especially when it comes to statistical and probabilistic problems, which are ubiquitous in business. Several studies (of which one of us, Chen, is a coauthor) concerning legal decisions found that judges grant political asylum more frequently before lunch than after, that they give lighter prison sentences if their NFL team won the previous day than if it lost, and that they will go easier on a defendant on the latter’s birthday. Clearly justice might be better served if human decision makers were assisted by software that told them when a decision they were planning to make was inconsistent with their prior decisions or with the decision that an analysis of purely legal variables would predict.
AI可以做到這點。另外一項研究(陳參與其中)表明,加載了由基本法律變量組成的模型的AI程序,在申請避難的案件開庭當天,可以對結(jié)果做出準確率達80%的預測。作者為程序加入了機器學習功能,AI可以根據(jù)法官過去的決定模擬每位法官的決策過程。
AI can deliver that kind of input. Another study (also with Chen as a coauthor) showed that AI programs processing a model made up of basic legal variables (constructed by the study’s authors) can predict asylum decisions with roughly 80% accuracy on the date a case opens. The authors have added learning functionality to the program, which enables it to simulate the decision-making of an individual judge by drawing on that judge’s past decisions.
這一方法也適用于其他情境。例如,馬布爾資產(chǎn)管理公司的資產(chǎn)組合經(jīng)理(PM)在做出可能提升整體資產(chǎn)組合風險的投資決定時,例如提高對某特定領域或某地區(qū)的曝光,系統(tǒng)會在電腦控制的交易流中彈出對話框提醒他們,可以適當調(diào)整。PM也許會對這樣的反饋視而不見,但起碼知道了公司的風險限制,這種反饋仍然有助于PM的決策。
The approach translates well to other contexts. For example, when portfolio managers (PMs) at Marble Bar Asset Management consider buy or sell decisions that may raise the overall portfolio risk—for example, by increasing exposure to a particular sector or geography—the system alerts them through a pop-up during a computerized transaction process so that they can adjust appropriately. A PM may ignore such feedback as long as company risk limits are observed. But in any case the feedback helps the PM reflect on his or her decisions.
AI當然并不總是“正確的”。AI的建議往往不會考慮到人類決策者才掌握的可靠的私人信息,因此也許并不會糾正潛在的行為偏差,而是起到反作用。所以對AI的使用應該是互動式的,算法根據(jù)數(shù)據(jù)提醒人類,而人類教會AI為什么自己忽略了某個提醒。這樣做提高了AI的效用,也保留了人類決策者的自主權(quán)。
Of course AI is not always “right.” Often its suggestions don’t take into account some reliable private information to which the human decision maker has access, so the AI might steer an employee off course rather than simply correct for possible behavioral biases. That’s why using it should be like a dialogue, in which the algorithm provides nudges according to the data it has while the human teaches the AI by explaining why he or she overrode a particular nudge. This improves the AI’s usefulness and preserves the autonomy of the human decision maker.
可惜很多AI系統(tǒng)的應用方式侵占了人類的自主權(quán)。例如,算法一旦將某銀行交易標記為潛在詐騙,職員必須請主管甚至外部審計員確認后,才能批準這一交易。有時,人類幾乎不可能撤銷機器做出的決定,客戶和客服人員一直對此感到挫敗。很多情況下AI的決策邏輯很模糊,即便犯錯員工也沒有資格表示質(zhì)疑。
Unfortunately, many AI systems are set up to usurp that autonomy. Once an algorithm has flagged a bank transaction as possibly fraudulent, for example, employees are often unable to approve the transaction without clearing it with a supervisor or even an outside auditor. Sometimes undoing a machine’s choice is next to impossible—a persistent source of frustration for both customers and customer service professionals. In many cases the rationale for an AI choice is opaque, and employees are in no position to question that choice even when mistakes have been made.
機器搜集人類決策數(shù)據(jù)時,還有一大問題是隱私權(quán)。除了在人類和AI的互動中給予人類控制權(quán),我們還要確保機器搜集的數(shù)據(jù)都是保密的。工程師團隊和管理團隊間應該互不干涉,否則員工也許會擔心自己和系統(tǒng)不設防的交互如果犯了錯,之后會受到懲罰。
Privacy is another big issue when machines collect data on the decisions people make. In addition to giving humans control in their exchanges with AI, we need to guarantee that any data it collects on them is kept confidential. A wall ought to separate the engineering team from management; otherwise employees may worry that if they freely interact with the system and make mistakes, they might later suffer for them.
此外,企業(yè)應該在AI設計和互動方面制定規(guī)則,確保公司規(guī)范和實踐的一致性。這類規(guī)則要詳細描述在預測準確性達到何種程度的情況下需要做出提醒,何時需要給出提醒原因,確定提醒的標準,以及員工在何時應當聽從AI指令、何時該請主管決定如何處理。
Also, companies should set rules about designing and interacting with AI to ensure organizational consistency in norms and practices. These rules might specify the level of predictive accuracy required to show a nudge or to offer a reason for one; criteria for the necessity of a nudge; and the conditions under which an employee should either follow the AI’s instruction or refer it to a superior rather than accept or reject it.
為了讓員工在第二階段保有控制感,我們建議管理者和系統(tǒng)設計人員在設計時請員工參與:請他們作為專家,定義將要使用的數(shù)據(jù),并決定基本的事實;讓員工在研發(fā)過程中熟悉模型;應用模型后為員工提供培訓和互動機會。這一過程中,員工會了解建模過程、數(shù)據(jù)管理方式和機器推薦的依據(jù)。
To help employees retain their sense of control in phase 2, we advise managers and systems designers to involve them in design: Engage them as experts to define the data that will be used and to determine ground truth; familiarize them with models during development; and provide training and interaction as those models are deployed. In the process, employees will see how the models are built, how the data is managed, and why the machines make the recommendations they do.
第三階段??教練
普華永道最近一項調(diào)研表明,參與者中60%稱希望獲得每日或每周一次的工作表現(xiàn)反饋。原因并不復雜。彼得·德魯克(Peter Drucker)2005年在著名的《哈佛商業(yè)評論》文章《管理自己》(“Managing Oneself”)中指出,人們一般都不知道自己擅長什么。當他們覺得自己知道時,往往是錯誤的。
Phase 3: The Coach
In a recent PwC survey nearly 60% of respondents said that they would like to get performance feedback on a daily or a weekly basis. It’s not hard to see why. As Peter Drucker asserted in his famous 2005?Harvard Business Review?article?“Managing Oneself,”?people generally don’t know what they are good at. And when they think they do know, they are usually wrong.
問題在于,發(fā)現(xiàn)自身優(yōu)勢、獲得改進機會的唯一方式是通過關(guān)鍵決策和行為的縝密分析。而這需要記錄自己對結(jié)果的預期,9到12個月后再將現(xiàn)實和預期進行比較。因此,員工獲得的反饋往往來自上級主管在工作總結(jié)時的評價,無法自己選擇時間和形式。這個事實很可惜,因為紐約大學的特莎·韋斯特(Tessa West)在近期神經(jīng)科學方面的研究中發(fā)現(xiàn),如果員工感到自主權(quán)受保護,可以自行掌控對話(例如能選擇收到反饋的時間),就能更好地對反饋做出反應。
The trouble is that the only way to discover strengths and opportunities for improvement is through a careful analysis of key decisions and actions. That requires documenting expectations about outcomes and then, nine months to a year later, comparing those expectations with what actually happened. Thus the feedback employees get usually comes from hierarchical superiors during a review—not at a time or in a format of the recipient’s choosing. That is unfortunate, because, as Tessa West of New York University found in a recent neuroscience?study,?the more people feel that their autonomy is protected and that they are in control of the conversation—able to choose, for example, when feedback is given—the better they respond to it.
AI可以解決這一問題。前文描述的程序可以給員工提供反饋,讓他們自查績效,反省自己的錯誤。每月一次根據(jù)員工歷史表現(xiàn)提取的分析數(shù)據(jù),也許可以幫助他們更好地理解決策模式和實踐。幾家金融公司正在采用這一措施。例如MBAM的資產(chǎn)組合經(jīng)理接受來自數(shù)據(jù)分析系統(tǒng)的反饋,該系統(tǒng)會統(tǒng)計每個人的投資決定。
AI could address this problem. The capabilities we’ve already mentioned could easily generate feedback for employees, enabling them to look at their own performance and reflect on variations and errors. A monthly summary analyzing data drawn from their past behavior might help them better understand their decision patterns and practices. A few companies, notably in the financial sector, are taking this approach. Portfolio managers at MBAM, for example, receive feedback from a data analytics system that captures investment decisions at the individual level.
數(shù)據(jù)展現(xiàn)了資產(chǎn)組合經(jīng)理有趣且多變的偏見。一些經(jīng)理更厭惡損失,對表現(xiàn)不佳的投資遲遲不肯止損。另一些則過度自信,可能對某項投資持倉過重。AI分析會發(fā)現(xiàn)這些行為,像教練一樣為其提供定制化反饋,標記行為隨時間的變化,給出改進決策建議。但最終由PM決定如何處理這些反饋。MBAM的領導團隊認為,這種“交易優(yōu)化”正逐漸成為公司核心的差異化因素,幫助資產(chǎn)組合經(jīng)理的發(fā)展,也讓公司變得更有吸引力。
The data can reveal interesting and varying biases among PMs. Some may be more loss-averse than others, holding on to underperforming investments longer than they should. Others may be overconfident, possibly taking on too large a position in a given investment. The analysis identifies these behaviors and—like a coach—provides personalized feedback that highlights behavioral changes over time, suggesting how to improve decisions. But it is up to the PMs to decide how to incorporate the feedback. MBAM’s leadership believes this “trading enhancement” is becoming a core differentiator that both helps develop portfolio managers and makes the organization more attractive.
更重要的是,好導師可以從被指導者身上學到東西,機器學習的“教練程序”也可以從有自主權(quán)的人類員工的決策中學習。上述關(guān)系中,人類可以反對“教練程序”,由此產(chǎn)生的新數(shù)據(jù)會改變AI的隱含模型。例如,如果由于近期公司事件,資產(chǎn)組合經(jīng)理決定不對某個標記股票進行交易,他可以給系統(tǒng)做出解釋。有了這種反饋,系統(tǒng)可以持續(xù)搜集分析數(shù)據(jù)并得出洞見。
What’s more, just as a good mentor learns from the insights of the people who are being mentored, a machine-learning “coachbot” learns from the decisions of an empowered human employee. In the relationship we’ve described, a human can disagree with the coachbot—and that creates new data that will change the AI’s implicit model. For example, if a portfolio manager decides not to trade a highlighted stock because of recent company events, he or she can provide an explanation to the system. With feedback, the system continually captures data that can be analyzed to provide insights.
如果員工能理解并控制和AI的互動,就更能將其視為獲得反饋的安全渠道,目標是幫助人類提升績效而不是評估績效。想要實現(xiàn)這點,要選擇正確的界面。例如MBAM的交易提升工具(如視覺界面)是根據(jù)PM的偏好定制的。
If employees can relate to and control exchanges with artificial intelligence, they are more likely to see it as a safe channel for feedback that aims to help rather than to assess performance. Choosing the right interface is useful to this end. At MBAM, for example, trading enhancement tools—visuals, for instance—are personalized to reflect a PM’s preferences.
第二階段中,讓員工參與設計系統(tǒng)很關(guān)鍵。AI做教練時,人們會更害怕權(quán)力被奪走。有人將AI視為合作伙伴就有人將其視為競爭對手——誰愿意被機器比下去呢?自主權(quán)和隱私的擔憂也許會更強烈。和教練共事需要誠實,但人們也許并不愿意對一個之后會把自己表現(xiàn)不佳的數(shù)據(jù)分享給HR的“教練”敞開心扉。
As in phase 2, involving employees in designing the system is essential. When AI is a coach, people will be even more fearful of disempowerment. It can easily seem like a competitor as well as a partner—and who wants to feel less intelligent than a machine? Concerns about autonomy and privacy may be even stronger. Working with a coach requires honesty, and people may hesitate to be open with one that might share unflattering data with the folks in HR.
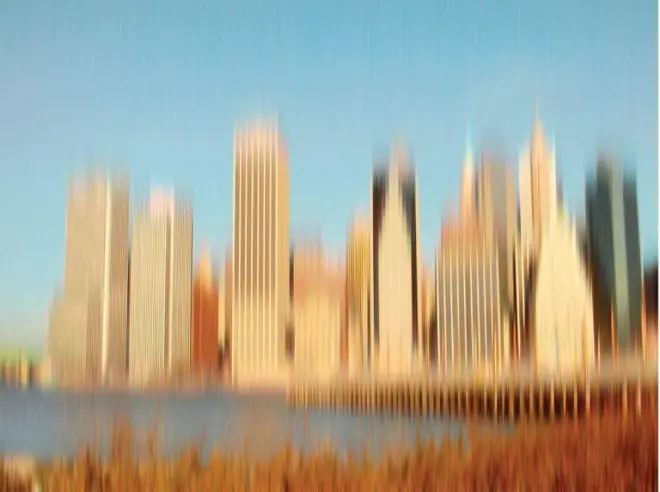
前三階段部署AI的方式當然有不足之處。長遠來看,新技術(shù)創(chuàng)造出的工作比毀掉的多,但就業(yè)市場的顛覆過程可能會很痛苦。馬特·比恩(Matt Beane)在《人機共生:組織新生態(tài)》(“Learning to Work with Intelligent Machines” ,2019年《哈佛商業(yè)評論》9月刊)一文中稱,部署AI的企業(yè)給員工親身實踐以及導師指導的機會更少。
Deploying AI in the ways described in the first three phases does of course have some downsides. Over the long term new technologies create more jobs than they destroy, but meanwhile labor markets may be painfully disrupted. What’s more, as Matt Beane argues in?“Learning to Work with Intelligent Machines”?(HBR, September–October 2019), companies that deploy AI can leave employees with fewer opportunities for hands-on learning and mentorship.
因此,風險的確存在,人類不僅失去了初級職位(由于數(shù)字助手可以有效取代人類),還可能犧牲未來決策者自主決策的能力。但這并非不可避免,比恩在文章中指出,企業(yè)可以在利用人工智能為員工創(chuàng)造不同和更好的學習機會的同時提升系統(tǒng)透明度,并給員工更多控制權(quán)。未來的職場新人都將成長于人力加機器的工作環(huán)境,肯定比“前AI時代”的同事更能快速發(fā)現(xiàn)創(chuàng)新、增加價值和創(chuàng)造工作的機會。這把我們帶到了最后一個階段。
There is some risk, therefore, not only of losing entry-level jobs (because digital assistants can effectively replace human ones) but also of compromising the ability of future decision makers to think for themselves. That’s not inevitable, however. As Beane suggests, companies could use their artificial intelligence to create different and better learning opportunities for their employees while improving the system by making it more transparent and giving employees more control. Because future entrants to the workforce will have grown up in a human-plus-machine workplace, they will almost certainly be faster than their pre-AI colleagues at spotting opportunities to innovate and introduce activities that add value and create jobs—which brings us to the final phase.
第四階段??隊友
認知人類學家埃德溫·赫欽斯(Edwin Hutchins)研發(fā)出分布式認知理論。該理論基于他對艦船導航的研究,結(jié)合了水手、路線圖、標尺、指南針和繪圖工具。該理論總體上和意識延伸的概念相關(guān),假定認知過程、信仰和動機等頭腦活動并不一定僅限于大腦甚至身體。外部工具和儀器在正確的條件下,可以對認知過程起到重要作用,創(chuàng)造出所謂的耦合系統(tǒng)。
Phase 4: The Teammate
Edwin Hutchins, a cognitive anthropologist, developed what is known as the theory of distributed cognition. It is based on his study of ship navigation, which, he showed, involved a combination of sailors, charts, rulers, compasses, and a plotting tool. The theory broadly relates to the concept of extended mind, which posits that cognitive processing, and associated mental acts such as belief and intention, are not necessarily limited to the brain, or even the body. External tools and instruments can, under the right conditions, play a role in cognitive processing and create what is known as a?coupled system.
和這一思路一致,AI應用的最后一個階段(就我們所知尚未有企業(yè)達到這個水平),企業(yè)應該打造一個人類和機器同時貢獻專長的耦合網(wǎng)絡。我們認為,隨著AI和人類用戶不斷交互,搜集專家歷史決策及行為數(shù)據(jù),分析并建模,不斷完善,在完全整合了AI教練程序的企業(yè)中自然會出現(xiàn)一個專家社群。舉例來說,采購經(jīng)理在決策時只需輕輕一點,就能看到其他人可能的報價——定制化的專家團體可能會對采購經(jīng)理有所幫助。
In line with this thinking, in the final phase of the AI implementation journey (which to our knowledge no organization has yet adopted) companies would develop a coupled network of humans and machines in which both contribute expertise. We believe that as AI improves through its interactions with individual users, analyzing and even modeling expert users by drawing on data about their past decisions and behaviors, a community of experts (humans and machines) will naturally emerge in organizations that have fully integrated AI coachbots. For example, a purchasing manager who—with one click at the moment of decision—could see what price someone else would give could benefit from a customized collective of experts.
盡管技術(shù)已經(jīng)能夠?qū)崿F(xiàn)這樣的集體智慧,但這一階段仍然充滿挑戰(zhàn)。例如,任何此類AI整合都要避免建立在偏見(舊的或者新的)基礎上,必須尊重人類隱私,人類才能像信任同類一樣信任AI,這本身已經(jīng)充滿挑戰(zhàn),因為無數(shù)研究證明人類信任彼此都很難。
Although the technology to create this kind of collective intelligence now exists, this phase is fraught with challenges. For example, any such integration of AI must avoid building in old or new biases and must respect human privacy concerns so that people can trust the AI as much as they would a human partner. That in itself is a pretty big challenge, given the volume of research demonstrating how hard it is to build trust among humans.
在職場建立信任的最佳方式是增進理解。卡內(nèi)基梅隆大學戴維·丹克斯(David Danks)和同事就這一主題進行了研究,根據(jù)其模型,一個人信任某人的原因是理解對方的價值觀、欲望和目的,對方也表明始終關(guān)心我的利益。理解一直是人類彼此信任的基礎,也很適合人類和AI發(fā)展關(guān)系,因為人類對人工智能的恐懼通常也是由于對AI運作方式的不理解。
The best approaches to building trust in the workplace rely on the relationship between trust and understanding—a subject of study by David Danks and colleagues at Carnegie Mellon. According to this model, I trust someone because I understand that person’s values, desires, and intentions, and they demonstrate that he or she has my best interests at heart. Although understanding has historically been a basis for building trust in human relationships, it is potentially well suited to cultivating human–AI partnerships as well, because employees’ fear of artificial intelligence is usually grounded in a lack of understanding of how AI works.
建立信任時,一個很困難的問題是如何定義“解釋”,更不用說“好的解釋”。很多研究都在關(guān)注這個難題。例如,本文作者之一伊維紐正嘗試通過所謂“反事實解釋”的方式揭示機器學習的“黑匣子”。“反事實解釋”通過找出決定決策方向的交易特征列表,闡明AI系統(tǒng)做出某個決定(例如批準某個交易)的原因。如果交易不符合某項特征(或者和事實相反),系統(tǒng)就會做出不同決定(拒絕交易)。
In building understanding, a particular challenge is defining what “explanation” means—let alone “good explanation.” This challenge is the focus of a lot of research. For example, one of us (Evgeniou) is working to open up machine-learning “black boxes” by means of so-called counterfactual explanations. A counterfactual explanation illuminates a particular decision of an AI system (for example, to approve credit for a given transaction) by identifying a short list of transaction characteristics that drove the decision one way or another. Had any of the characteristics been different (or counter to the fact), the system would have made a different decision (credit would have been denied).
伊維紐還希望了解人們覺得什么樣的解釋是對AI決策的優(yōu)秀解釋。例如,人們是會覺得按邏輯列出特征(“因為具備X、Y、Z三個特征,所以這一交易獲批”)更好,還是說明該決定和其他決定的相關(guān)性(這一交易獲批是因為和其他獲批交易相似,你可以比較一下)更好。隨著針對AI解釋的研究繼續(xù)深入,AI系統(tǒng)會變得更透明,有助于贏得更多信任。
Evgeniou is also exploring what people perceive as good explanations for AI decisions. For example, do they see an explanation as better when it’s presented in terms of a logical combination of features (“The transaction was approved because it had X,Y,Z characteristics”) or when it’s presented relative to other decisions (“The transaction was approved because it looks like other approved transactions, and here they are for you to see”)? As research into what makes AI explainable continues, AI systems should become more transparent, thus facilitating trust.
新技術(shù)應用一直是重大挑戰(zhàn)。一項技術(shù)的影響力越大,挑戰(zhàn)就越大。人工智能技術(shù)的潛在影響讓人們感到很難將其付諸實踐。但如果我們謹慎行事,這一過程可以相對順利。這也是為什么企業(yè)必須有責任地設計和發(fā)展AI,特別注意透明度、決策自主權(quán)和隱私,而且要讓使用AI技術(shù)的人參與進來,否則,不清楚機器做決策的方式,人們害怕被機器限制甚至取代也是理所當然的。
Adopting new technologies has always been a major challenge—and the more impact a technology has, the bigger the challenge is. Because of its potential impact, artificial intelligence may be perceived as particularly difficult to implement. Yet if done mindfully, adoption can be fairly smooth. That is precisely why companies must ensure that AI’s design and development are responsible—especially with regard to transparency, decision autonomy, and privacy—and that it engages the people who will be working with it. Otherwise they will quite reasonably fear being constrained—or even replaced—by machines that are making all sorts of decisions in ways they don’t understand.
關(guān)鍵在于克服恐懼,建立信任。本文描述的四個階段都是由人類制定基本規(guī)則。通過負責任的設計,AI可以成為人類工作中真正的合作伙伴——一以貫之地快速處理大量各式數(shù)據(jù),提升人類的直覺和創(chuàng)造力,讓人類反過來指導機器。
Getting past these fears to create a trusting relationship with AI is key. In all four phases described in these pages, humans determine the ground rules. With a responsible design, AI may become a true partner in the workplace—rapidly processing large volumes of varied data in a consistent manner to enhance the intuition and creativity of humans, who in turn teach the machine.
鮑里斯·巴比克是歐洲工商管理學院決策科學助理教授。丹尼爾·陳是圖盧茲經(jīng)濟學院高級研究所教授,世界銀行司法改革計劃數(shù)據(jù)和證據(jù)首席研究員。賽奧佐羅斯·伊維紐是歐洲工商管理學院決策科學和技術(shù)管理教授,馬布爾資產(chǎn)管理公司顧問。安妮-勞倫·法雅德是紐約大學坦登工程學院創(chuàng)新、設計和企業(yè)研究副教授。
牛文靜 | 譯? ? 蔣薈蓉 | 校? ? 時青靖 | 編輯
?