Numpy中的stack,軸,廣播以及CNN介紹
在神經(jīng)網(wǎng)絡(luò)學(xué)習(xí)之Ndarray對(duì)象和CNN入門?中,主要介紹了Ndarray維度的概念和CNN的大體流程圖,本文基于此介紹Ndarray中比較重要的一個(gè)函數(shù)stack函數(shù)的使用以及numpy中的廣播, 簡(jiǎn)單介紹下CNN。
Stack函數(shù)
官方API介紹,我是沒看懂,不排除有大神看一眼就懂,如果沒看懂也沒關(guān)系,可以繼續(xù)往下讀,相信一定能理解stack究竟是怎么工作的。
numpy.stack(arrays, axis=0, out=None)[source]
Join a sequence of arrays?along a new axis.
The?axis
?parameter specifies the index of the new axis in the dimensions of the result. For example, if?axis=0
?it will be the first dimension and if?axis=-1
?it will be the last dimension.
筆者查閱了大量的資料,不過總感覺少了點(diǎn)什么,就是感覺始終不能理解誒stack是怎么堆疊的。于是就只好去看源碼了,如果從一開始就看源碼,或許可以節(jié)省很多時(shí)間。
源碼
源碼:
@array_function_dispatch(_stack_dispatcher)
def stack(arrays, axis=0, out=None):
? ?if not overrides.ARRAY_FUNCTION_ENABLED:
? ? ? ?# raise warning if necessary
? ? ? ?_arrays_for_stack_dispatcher(arrays, stacklevel=2)
? ?arrays = [asanyarray(arr) for arr in arrays]
? ?if not arrays:
? ? ? ?raise ValueError('need at least one array to stack')
? ?shapes = {arr.shape for arr in arrays}
? ?if len(shapes) != 1:
? ? ? ?raise ValueError('all input arrays must have the same shape')
? ?result_ndim = arrays[0].ndim + 1
? ?axis = normalize_axis_index(axis, result_ndim)
? ?sl = (slice(None),) * axis + (_nx.newaxis,)
? ?expanded_arrays = [arr[sl] for arr in arrays]
? ?return _nx.concatenate(expanded_arrays, axis=axis, out=out)
@的作用
@在python中是函數(shù)裝飾器,和Java中的注解是不一樣的。 猜猜下面下面的代碼會(huì)出現(xiàn)什么樣子的結(jié)果(注意這里funB是多參數(shù)的)
def funA(fn):
? ?print("funA is invoked first")
? ?# 定義一個(gè)嵌套函數(shù),和JavaScript有點(diǎn)類似
? ?def innerFun(*args, **kwargs):
? ? ? ?fn(*args, **kwargs)
? ?return innerFun
@funA
def funB(name, value):
? ?print("迎關(guān)注我的微信公眾號(hào)" + name + ",", "phpMyAdmin端口配置和mysql主從復(fù)制這篇文章" + value)
funB("無情劍客", "很棒");
使用函數(shù)裝飾器 A() 去裝飾另一個(gè)函數(shù) B(),其底層執(zhí)行了如下 2 步操作:
1.將 B 作為參數(shù)傳給 A() 函數(shù);2.將 A() 函數(shù)執(zhí)行完成的返回值反饋回B。
以上代碼等價(jià)于下面的代碼:
funB = funA(funB)
funB("無情劍客", "很棒")
最終的運(yùn)行結(jié)果:
funA is invoked first 迎關(guān)注我的微信公眾號(hào)無情劍客, phpMyAdmin端口配置和mysql主從復(fù)制這篇文章很棒
知道了@的作用具體的過程可以自己動(dòng)手debug,過程比較復(fù)雜,后續(xù)抽空補(bǔ)上。
關(guān)鍵代碼解讀
asanyarray
arrays = [asanyarray(arr) for arr in arrays]
舉個(gè)例子:
import numpy as np
a = np.arange(24)
print(a.ndim)
b = a.reshape(2, 3, 4)
print(b)
c = np.stack(b, axis=2)
print(c.shape)
print(c)
當(dāng)調(diào)用stack方法步過這段代碼的時(shí)候,arrays的結(jié)果是一個(gè)list,里面的元素如下圖所示:

看下面這段代碼,就基本知道上面的list是怎么來的的,
arr = [1,2,5]
list_arr = [arr1 for arr1 in arr]
print(list_arr)
arrs = [asanyarray(arr1) for arr1 in arr]
print(arrs)
運(yùn)行結(jié)果是:
[1, 2, 5] [array(1), array(2), array(5)]
關(guān)于asanyarray: Convert the input to an ndarray, but pass?ndarray subclasses through.
維度+1
這是和concatenate函數(shù)很重要的一個(gè)區(qū)別,也體現(xiàn)了API中的new axis.
result_ndim = arrays[0].ndim + 1
axis = normalize_axis_index(axis, result_ndim)
expanded_arrays
如何實(shí)現(xiàn)維度+1的那,下面這段代碼是關(guān)鍵:
sl = (slice(None),) * axis + (_nx.newaxis,)
expanded_arrays = [arr[sl] for arr in arrays]
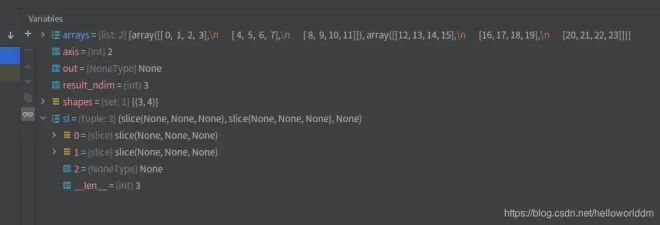
可知s1的值是個(gè)tuple。 這個(gè)sl是怎么計(jì)算出來的,舉個(gè)例子:
sl = (slice(None),) *2 + (None,)
sl
運(yùn)行結(jié)果如下: (slice(None, None, None), slice(None, None, None), None)
e = slice(None)
e
運(yùn)行結(jié)果是: slice(None, None, None)
那么arr[sl]是怎么計(jì)算出來的那?
numpy.newaxis The newaxis object can be used in all slicing operations to create an axis of length one.?newaxis is an alias for ‘None’, and ‘None’ can be used in place of this with the same result
(1)slice基本語法
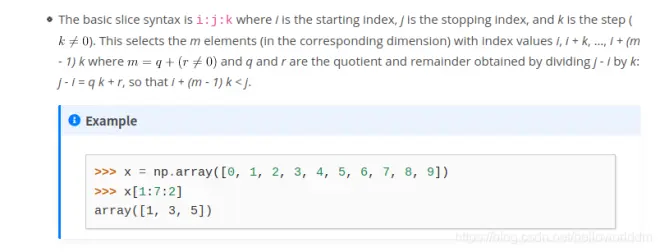
按照公式計(jì)算一下: i = 1, j =7, k=2 1, 3, 1+(m-1)*2 m = q+r q = (7-1)/2 = 3 r = 0 m = 3 因此最終結(jié)果是[1, 3, 5] (1)slice default處理
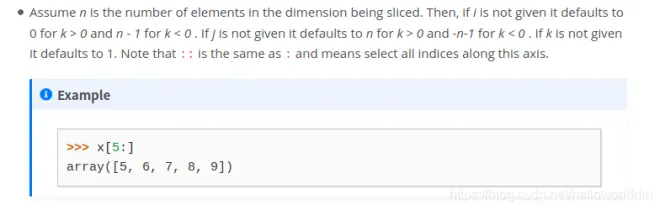
等價(jià)于x[5:4:1]
(3) 高維數(shù)組處理
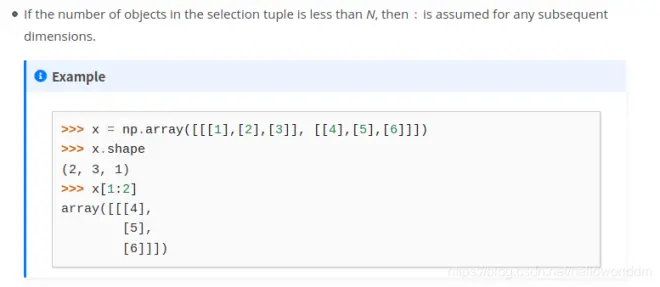
通過下面的note可知,x[1:2]等價(jià)于x[(1:2), ],很明顯,它的緯度是小于N(=2)的。因此這里面的1代表的是取索引是1的二維數(shù)組?。
可以將3維數(shù)組想象成行和列的組合,只不過這里的列是一個(gè)二維數(shù)組。
對(duì)于二維數(shù)組可以通過下圖來看,解釋一下第一個(gè),其他的同理。
arr[:2,1:]代表取到第一行(<2),從第一列到最后一列,顯然shape就是(2,2)
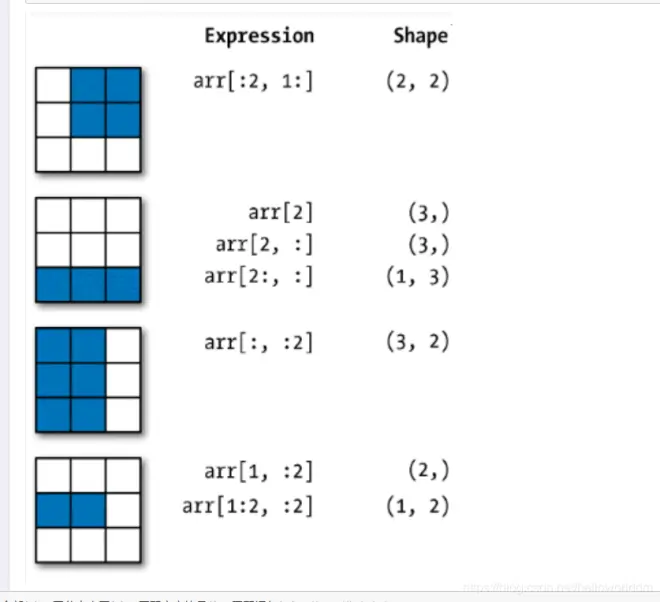
Note: In Python, x[(exp1, exp2, ..., expN)] is equivalent to x[exp1, exp2, ..., expN]; the latter is just syntactic sugar for the former.
(4) 省略號(hào)
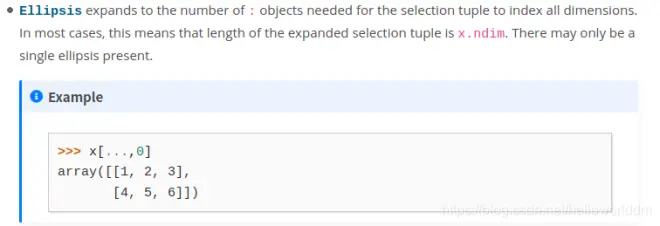
使選擇元組的長度與數(shù)組的維度相同。顯然選擇元組的長度是2,數(shù)組的維度也是2。 (5) newaxis
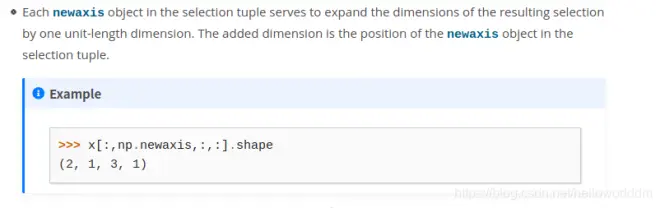
以前是(2,3,1),因?yàn)?strong>The added dimension is the position of the newaxis object in the selection tuple, 所以新的數(shù)組的shape是(2,1,3,1)。翻譯下就是2個(gè)三維數(shù)組,每個(gè)3維數(shù)組中有1個(gè)2維數(shù)組,每個(gè)2維數(shù)組中有3個(gè)1維數(shù)組,每個(gè)1維數(shù)組中有1也元素。
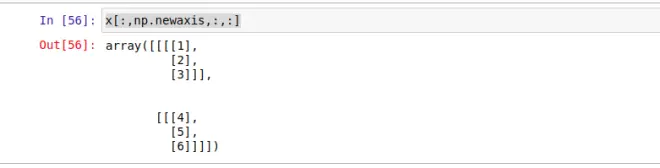
(6) slice構(gòu)造函數(shù) Remember that a slicing tuple can always be constructed as obj and used in the x[obj] notation.?Slice objects can be used in the construction in place of the [start:stop:step] notation. For example, x[1:10:5,::-1] can also be implemented as obj = (slice(1,10,5), slice(None,None,-1)); x[obj] . This can be useful for constructing generic code that works on arrays of arbitrary dimension.
通過前面的分析可知arr[sl]是這樣算出來的的: arr[(slice(None, None, None), slice(None, None, None), None)] 等價(jià)與:arr[: , :, np.newaxis] 以前的arr的shape是(3,4),經(jīng)過這樣的操作之后,就變成了(3,4,1),也就是3個(gè)2維數(shù)組,每個(gè)2維度數(shù)組中有4個(gè)1維數(shù)組,每個(gè)1維數(shù)組中有1個(gè)元素。
因此expanded_arraays最終的結(jié)果就是:
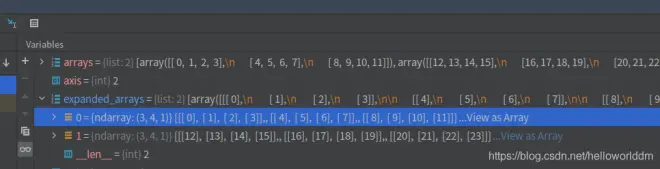
concatenate
從最內(nèi)側(cè)的軸進(jìn)行拼接。
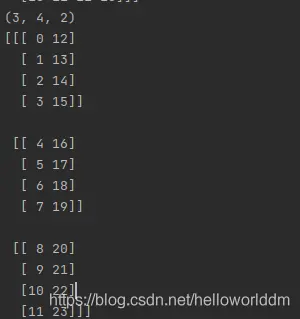
軸的概念
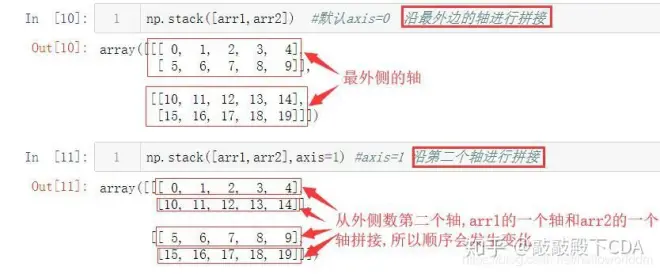
我在圖中標(biāo)注出了哪些是外邊的軸,哪些是第二個(gè)軸,哪些是最里邊的軸,有一個(gè)比較簡(jiǎn)單的方法來判斷這些軸,就是觀察一下方括號(hào),方括號(hào)數(shù)量越多的軸,越是在外層的軸,在這個(gè)例子中,最外側(cè)的軸有兩層方括號(hào),從外邊數(shù)第二個(gè)軸有一層方括號(hào),這里還好一點(diǎn),最難理解的是最里邊的軸,最后來看一下最內(nèi)側(cè)的軸。
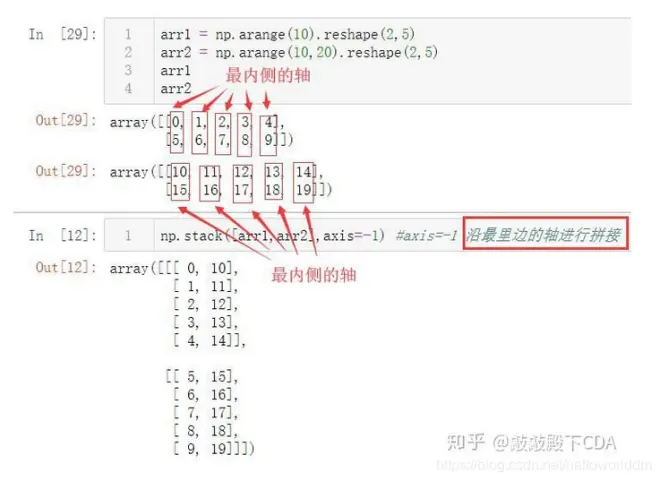
numpy中的廣播
廣播(Broadcast)是 numpy 對(duì)不同形狀(shape)的數(shù)組進(jìn)行數(shù)值計(jì)算的方式。
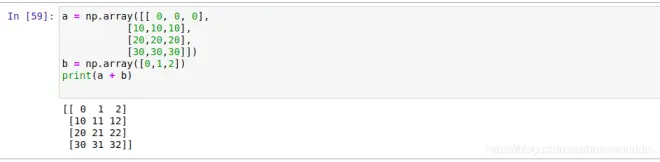
下面的圖片展示了數(shù)組 b 如何通過廣播來與數(shù)組 a 兼容。
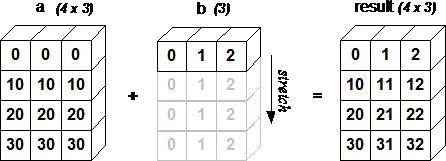
卷積神經(jīng)網(wǎng)絡(luò)入門
卷積神經(jīng)網(wǎng)絡(luò)主要用在圖像領(lǐng)域。當(dāng)然也可以用在文本分類,不過NLP領(lǐng)域,在NLP領(lǐng)域需要一些處理技巧。后續(xù)文章會(huì)詳細(xì)介紹。
簡(jiǎn)單看看CNN網(wǎng)絡(luò)能夠做什么:
輸入 -> CNN 網(wǎng)絡(luò) ->輸出
如果做圖像識(shí)別,輸入就是要識(shí)別的圖像,輸出就是可能的圖像的概率,概率越大,自然可能性越大。
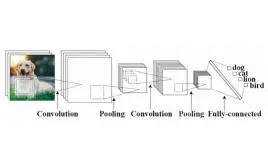
CNN 網(wǎng)絡(luò)這個(gè)黑盒要2個(gè)重要的概念: (1)卷積核,也就是特征是CNN網(wǎng)路uo自動(dòng)生成的。通過大量的訓(xùn)集來不斷調(diào)整特征和優(yōu)化參數(shù),提高準(zhǔn)確度,因此數(shù)據(jù)閱讀自然越準(zhǔn)確 (2)感受野,類比人的眼睛,看的越多,自然提取的特征就越多。橫看成嶺側(cè)成峰
對(duì)于分類人任務(wù),需要標(biāo)簽。監(jiān)督學(xué)習(xí)的類別
后續(xù)會(huì)詳細(xì)介紹CNN,這里先有一個(gè)初步的印象。
參考
?Indexing[1]?numpy數(shù)組的索引和切片[2]?NumPy 廣播(Broadcast)[3]?numpy數(shù)組的各種拼接方法:stack和vstack,hstack,concatenate[4]?numpy.stack 與 numpy.concatenate 用法[5]
公眾號(hào)
更多機(jī)器學(xué)習(xí)內(nèi)容,歡迎關(guān)注我的微信公眾號(hào): 無情劍客。
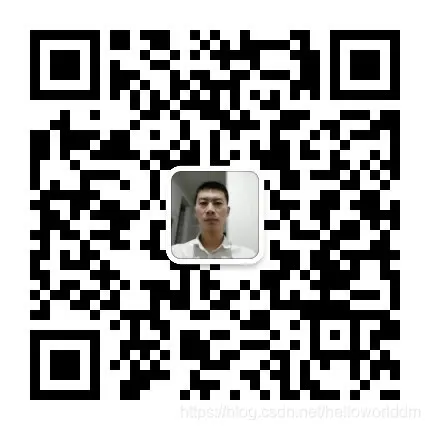
References
[1]
?Indexing:?https://numpy.org/doc/stable/reference/arrays.indexing.html[2]
?numpy數(shù)組的索引和切片:?https://www.cnblogs.com/mengxiaoleng/p/11616869.html[3]
?NumPy 廣播(Broadcast):?https://www.runoob.com/numpy/numpy-broadcast.html[4]
?numpy數(shù)組的各種拼接方法:stack和vstack,hstack,concatenate:?https://zhuanlan.zhihu.com/p/82996332[5]
?numpy.stack 與 numpy.concatenate 用法:?https://www.pianshen.com/article/1767127443/