Deepmind AlphaZero - Mastering Games Wit
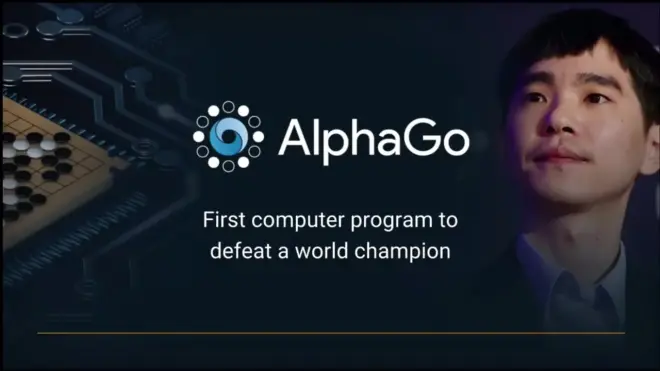
2023-5-3 16:03:03
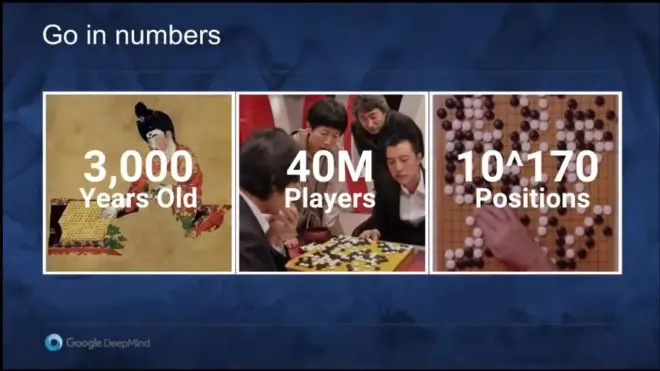
The game of go is the most
The pinnacle of human knowledge
10^170 positions
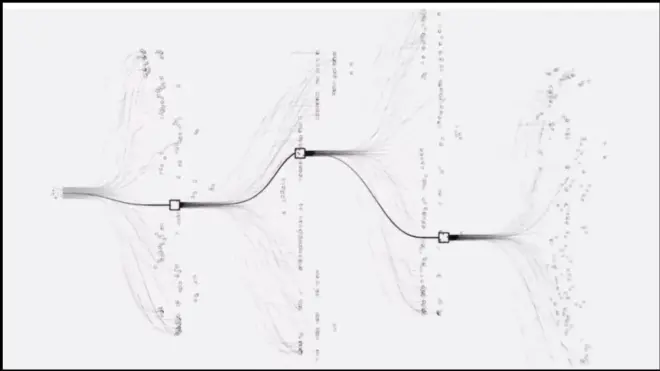
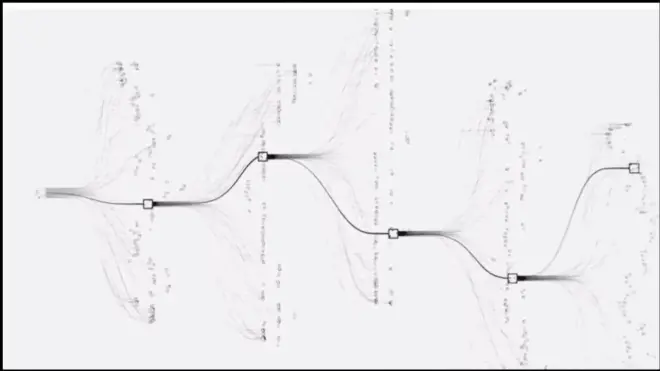
Traditional search methods cannot achieve such.
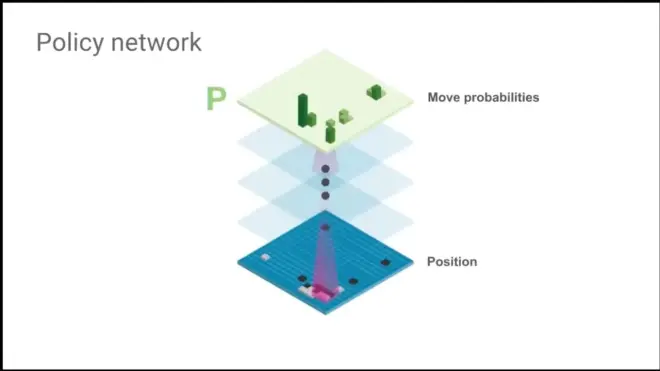
Two convolutional neural networks understanding the game of Go.
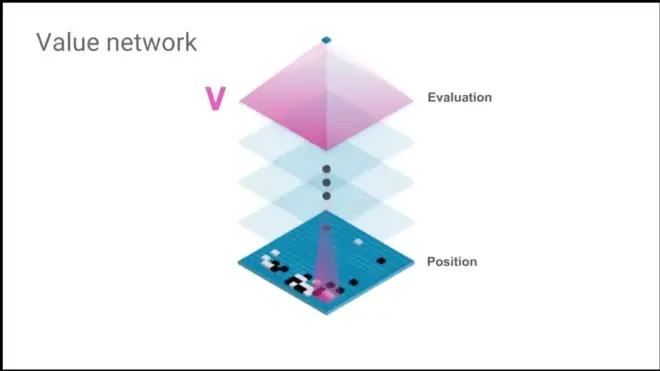
The second network.
Prerequisites: Understanding Convolutional Neural Networks, Understanding some padding knowledge. Understanding of Filtering. Computer Graphics.
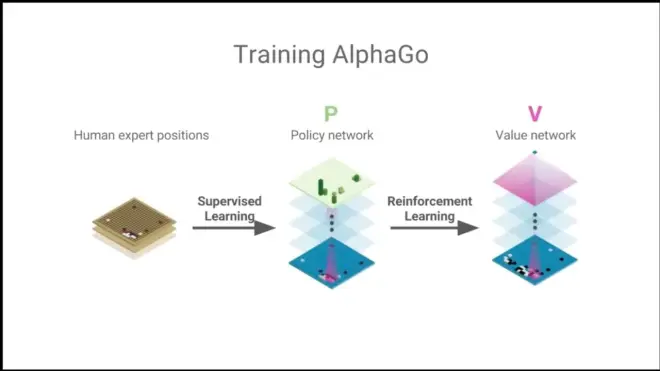
Pipelining.
Play games with itself once the policy network is constructed.
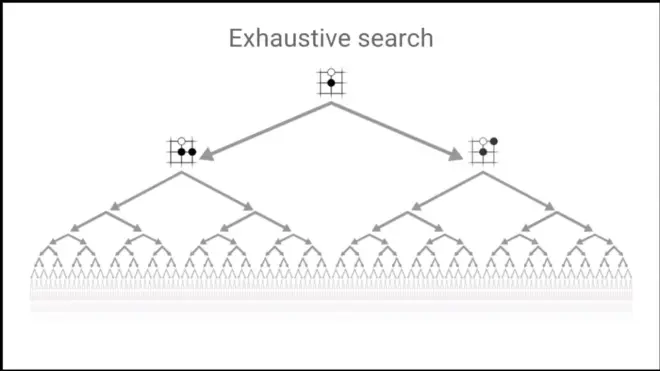
How to make search Tree more tractable?
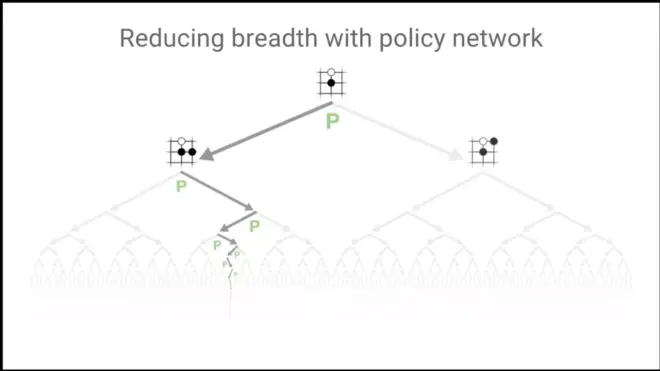
Just consider a handful.
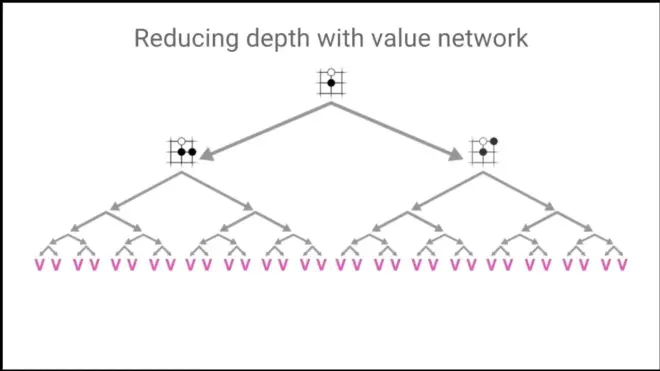
Value network reduces the depth. Replace it with only one single network.
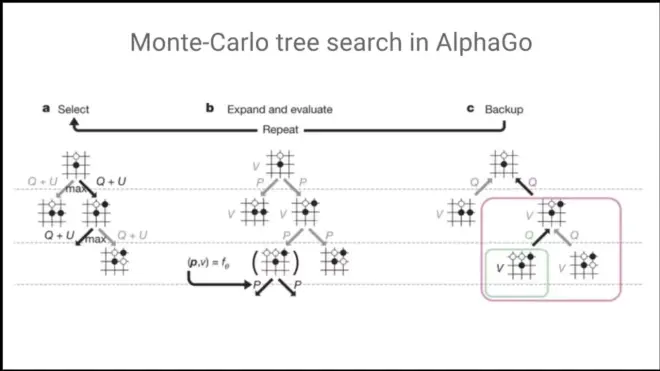
Child:樹的子集。不是什么孩子。
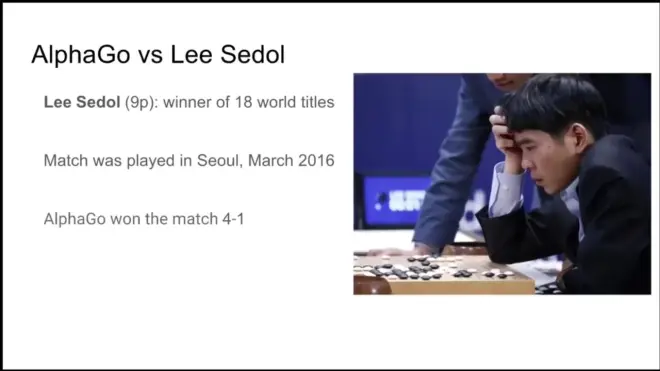
18 world titles是李昌鎬,搞錯了,是14個吧。。。
In particular, in some kind of positions, misunderstandings. delusions,
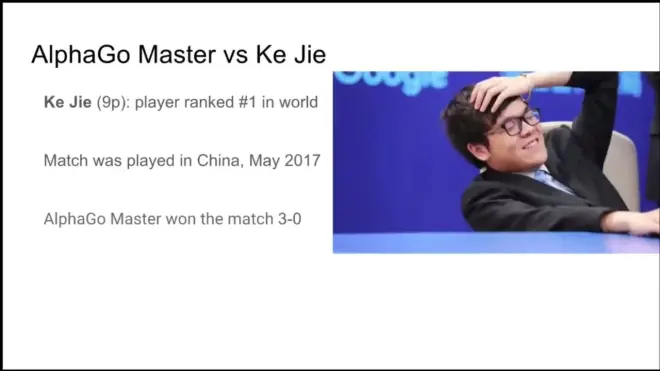
King of Baguan(大誤)
60 matches.
2023-5-3 16:14:25
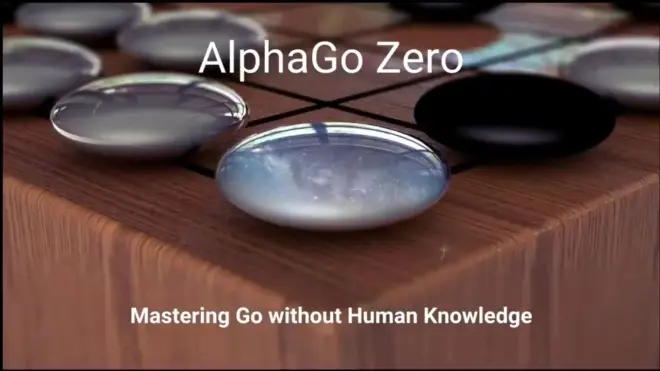
2023-5-3 21:38:22
Take every form of human knowledge and remove it from the training process, except for the rules itself.
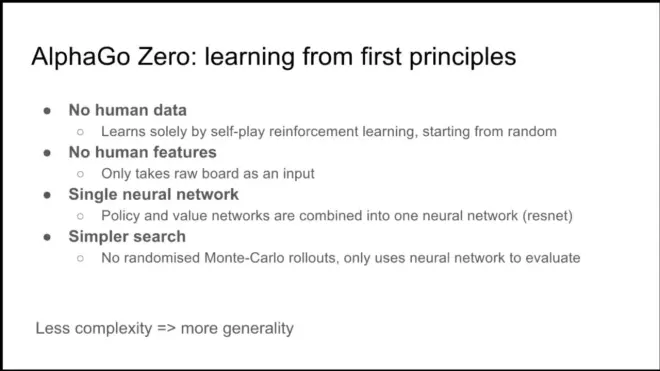
Less complexity means more generality.
It learns solely by self-play reinforcement learning, starting from random.
No handcraft features. The only thing Neural network sees is the raw board as input.
Combined the policy and value networks as one (a Residual network, ResNet)
No randomised Monte-Carlo rollouts, only uses neural network to evaluate.
By doing less, to make more general. (聯(lián)邦學(xué)習(xí)?)
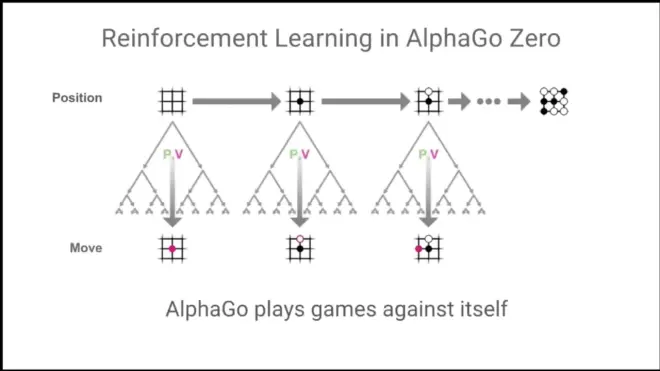
It is doing self learning. It becomes its own teacher.
From each position, Monte-Carlo tree search (random search)
Play and run the search, rinse and repeat.
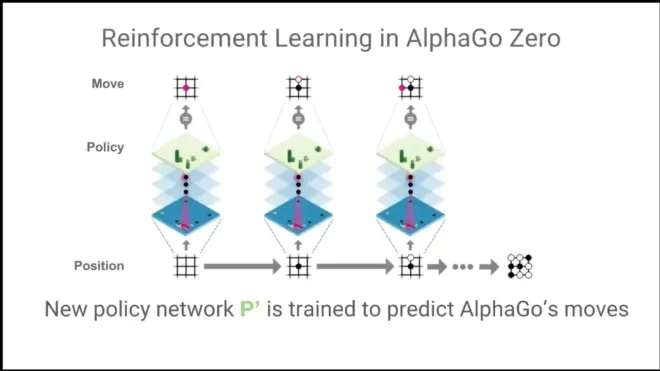
New policy network P' is trained to predict AlphaGo's Moves.
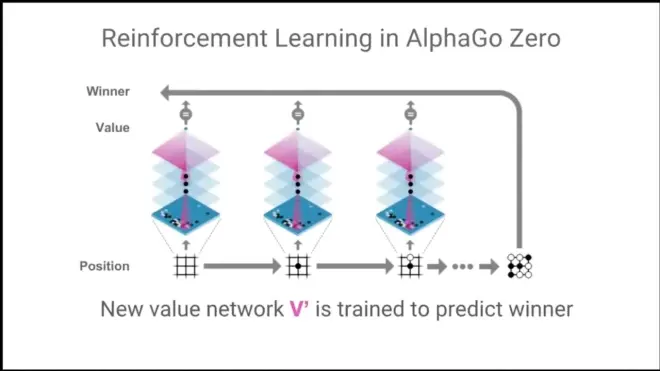
New Value network V' to predict winner
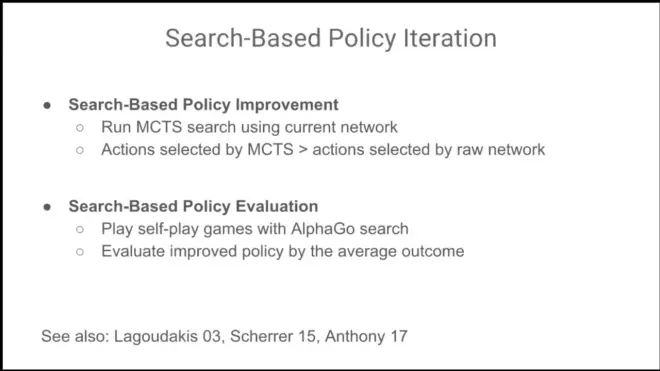
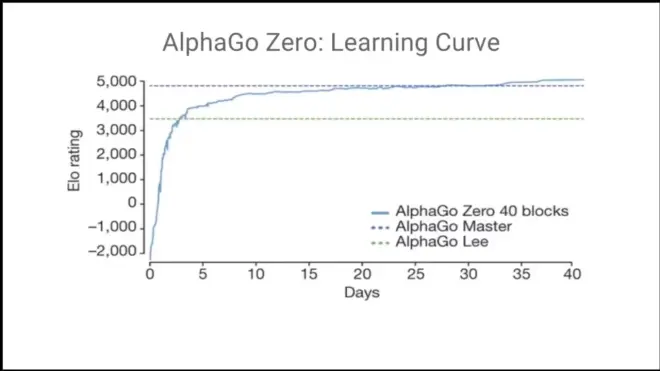
//This means that The game of Go is mathematically modelled.
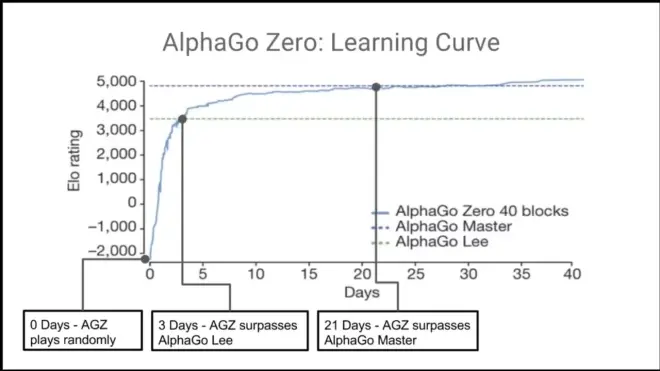
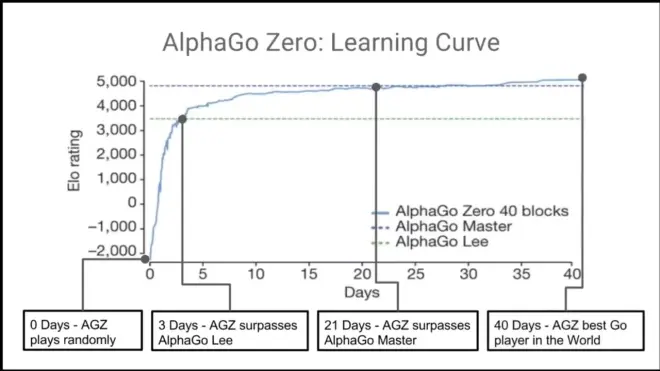
The best "player".
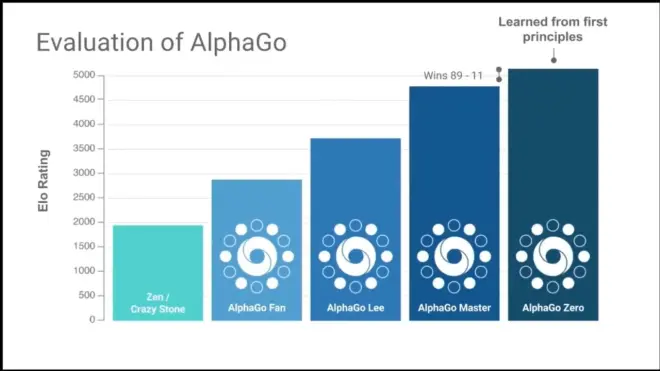
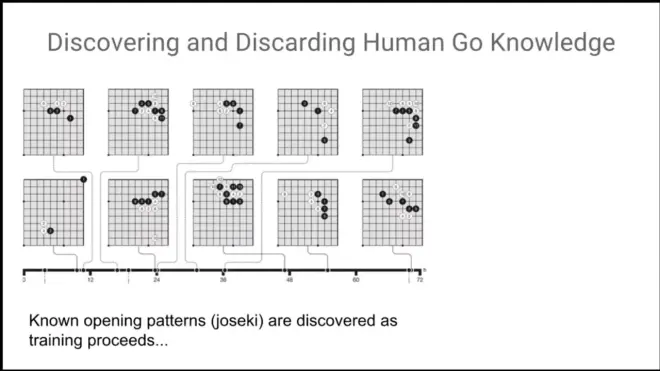
It discovers the Josekis itself.
2023-5-3 21:50:20
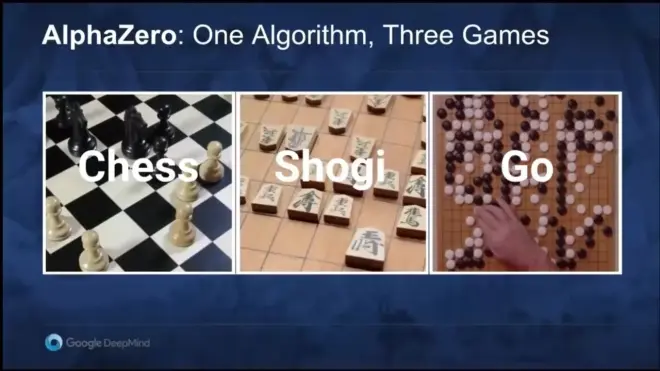
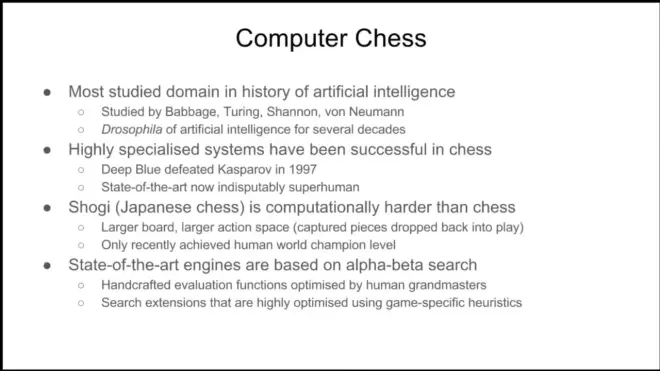
Handcrafted evaluation functions are optimised by human grandmasters.
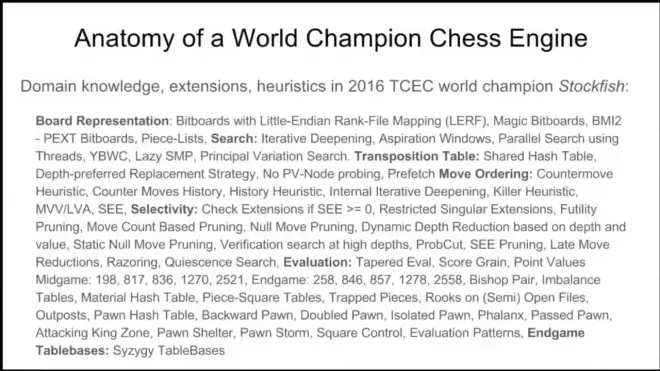
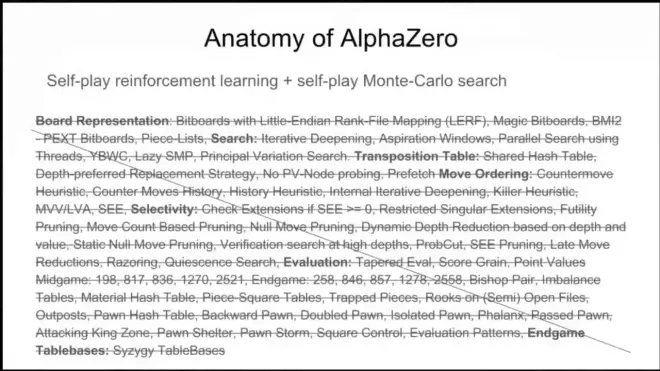
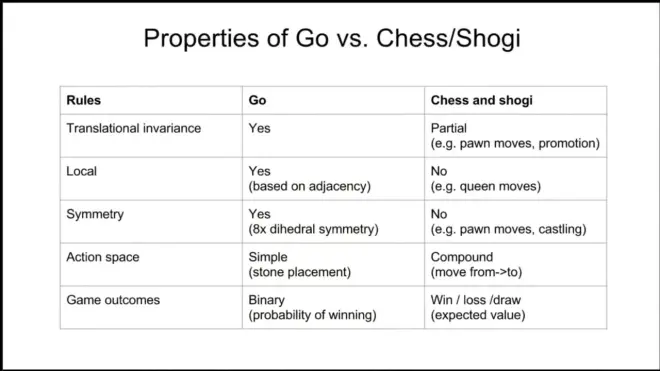
No draws:指三劫循環(huán)被ban了。2023-5-3 21:54:43
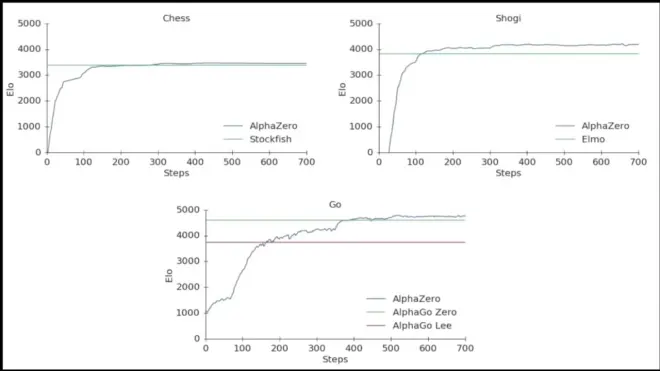
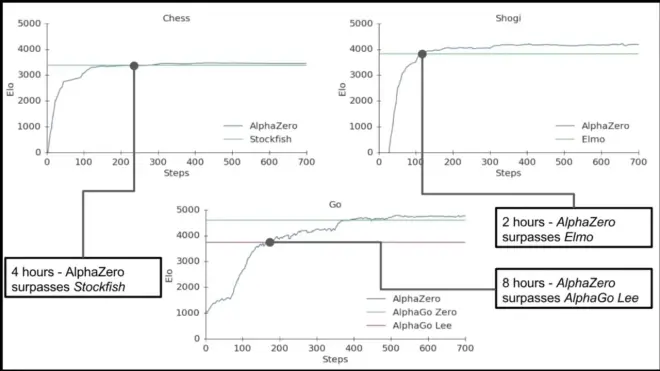
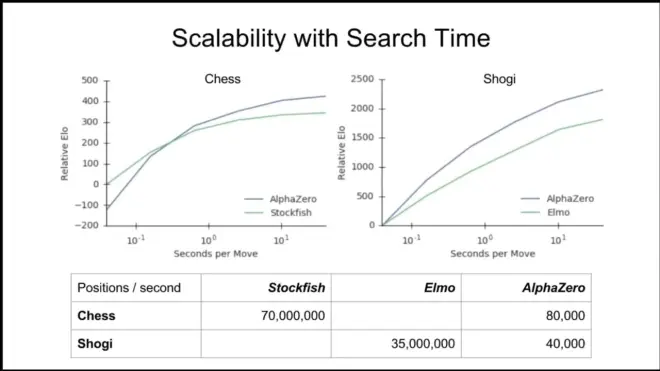
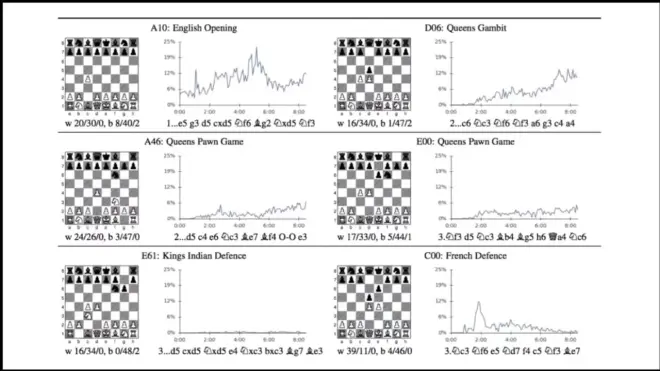
Conclude the moves beyond games.
The idea of AlphaZero, take us beyond Go, into the realms of General purpose of reinforcement learning. Still in early step. The real world is not like the world of Go or chess.
One thing is interested is to progress these methods into the general ideas.
Deep reinforcements algorithms host to hole array of different approaches.
Hierachical reinforcement learning workshop.
The key Idea 2023-5-3 22:01:36
The more we take out specific knowledge, The more we can enable our algorithms to general purpose. The more we can hope that they can transfer to something more helpful that is more than the domains that we are once design and tested.
Every time you specify in something, you hurt your generality.
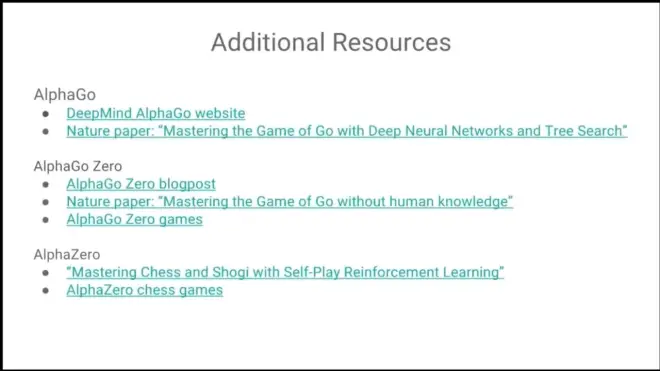
(大意)One of the things I have seen work on Chess, Superhuman level. When Analysis(跑程序的結(jié)果,落子的地方) that we do were given to humans. Humans can outperform without the computer itself. (人類會棋力大漲)I wonder if any analysis were made to see by giving this type of reinforcement learning solution, with algorithm solutions we have, can human still have new perspectives that can be added to the solution itself that we can improve(outperform) the program itself? (有沒有人機(jī)結(jié)合再次超過原有機(jī)器的下法)
(大意是說:機(jī)器學(xué)習(xí)的新招法(analysis)讓人的下法也革新了,人機(jī)結(jié)合有沒有可能讓人有新的超過程序本身?包括測出bug這種的)
Idea of combining is interesting. The Chess results were posted not long ago so that we cannot have the chance of performing these analyses(extensive investigations). The question of center programs which combine the human and machine together is interesting.
I cannot speculate the future. But The reactions of the chess community to how Alphazero plays chess is that Alphazero plays a more human-like style than previous programs. So it plays a way more aggressive and openstyle. Additionally new styles were played.
(視頻2017年的,以圍棋為例,李世石退役三番棋 vs Handuk 第一個讓2子棋又下出了神之一手,又是78手,所以第二局分先,輸了)(連笑也說過有些打蒙絕藝的招法,柯潔也是,進(jìn)行著最後的人棋倔強(qiáng);而另一些棋手成為了人工智能)而人工智能圍棋相關(guān)的也進(jìn)行了優(yōu)化。2023-5-3 22:23:19
2 more questions.
The only human knowledge fed were rules itselfs. how are the output moves represented and how to prevent the network from making an invalid move? 2023-5-3 22:23:55
We use the rule of the game, flat encoding, spatial representation.
(如何設(shè)計一個圍棋軟件)。2023-5-3 22:24:57
more about experiments. Curves you show have error bars, is it because the error bars were too small to see or are we seeing just one random result?
(實(shí)驗(yàn)相關(guān)的問題,也是基本的問題)
Runs are expensive. Runs are on Google Compute.
This is only reporting one experimental results here, and it is reproducable, where results are similar every single time.
不要問復(fù)雜的棋理棋道,而是這個。
2023-5-3 22:29:51