最新綜述:計(jì)算成像前沿進(jìn)展
原文鏈接:https://mp.weixin.qq.com/s/6zArHdc_ecqETJBvrb0I2g
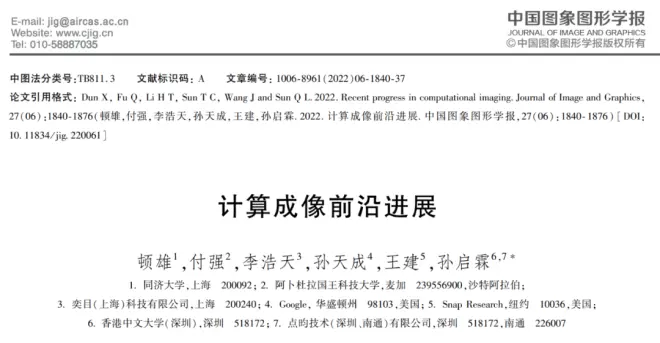
摘要:計(jì)算成像是融合光學(xué)硬件、圖像傳感器、算法軟件于一體的新一代成像技術(shù),它突破了傳統(tǒng)成像技術(shù)信息獲取深度(高動(dòng)態(tài)范圍、低照度)、廣度(光譜、光場(chǎng)、三維)的瓶頸。本文以計(jì)算成像的新設(shè)計(jì)方法、新算法和應(yīng)用場(chǎng)景為主線,通過(guò)綜合國(guó)內(nèi)外文獻(xiàn)和相關(guān)報(bào)道來(lái)梳理該領(lǐng)域的主要進(jìn)展。從端到端光學(xué)算法聯(lián)合設(shè)計(jì)、高動(dòng)態(tài)范圍成像、光場(chǎng)成像、光譜成像、無(wú)透鏡成像、低照度成像、三維成像、計(jì)算攝影等研究方向,重點(diǎn)論述計(jì)算成像領(lǐng)域的發(fā)展現(xiàn)狀、前沿動(dòng)態(tài)、熱點(diǎn)問(wèn)題和趨勢(shì)。端到端光學(xué)算法聯(lián)合設(shè)計(jì)包括了可微的衍射光學(xué)模型,折射光學(xué)模型以及基于可微光線追蹤的復(fù)雜透鏡的模型。高動(dòng)態(tài)范圍光學(xué)成像從原理到光學(xué)調(diào)制,多次曝光,多傳感器融合以及算法等層面闡述不同方法的優(yōu)點(diǎn)與缺點(diǎn)以及產(chǎn)業(yè)應(yīng)用。光場(chǎng)成像闡述了基于光場(chǎng)的三維重建技術(shù)在超分辨、深度估計(jì)和三維尺寸測(cè)量等方面國(guó)內(nèi)外的研究進(jìn)展和產(chǎn)業(yè)應(yīng)用,以及光場(chǎng)在粒子測(cè)速及三維火焰重構(gòu)領(lǐng)域的研究進(jìn)展。光譜成像闡述了當(dāng)前多通道濾光片,基于深度學(xué)習(xí)和波長(zhǎng)響應(yīng)曲線求逆問(wèn)題,以及衍射光柵,多路復(fù)用,超表面等優(yōu)化實(shí)現(xiàn)高光譜的獲取。無(wú)透鏡成像包括平面光學(xué)元件的設(shè)計(jì)和優(yōu)化,以及圖像的高質(zhì)量重建算法。低照度成像包括低照度情況下基于單幀、多幀、閃光燈、新型傳感器的圖像噪聲去除等。三維成像主要包括針對(duì)基于主動(dòng)方法的深度獲取的困難的最新的解決方案,這些困難包括強(qiáng)的環(huán)境光干擾(比如太陽(yáng)光),強(qiáng)的非直接光干擾(比如凹面的互反射,霧天的散射)等。計(jì)算攝影學(xué)是計(jì)算成像的一個(gè)分支學(xué)科,它從傳統(tǒng)攝影學(xué)發(fā)展而來(lái),更側(cè)重于使用數(shù)字計(jì)算的方式進(jìn)行圖像拍攝。在光學(xué)鏡片的物理尺寸、圖像質(zhì)量受限的情況下,如何使用合理的計(jì)算資源,繪制出用戶(hù)最滿(mǎn)意的圖像是其主要研究和應(yīng)用方向。
本文作為綜述文章發(fā)表在2022中國(guó)圖象圖形學(xué)報(bào)上。具體文章參見(jiàn):
http://www.cjig.cn/jig/ch/reader/view_abstract.aspx?file_no=20220607&flag=1
或 https://jianwang-cmu.github.io/22Survey/RecentProgressInComputationalImaging.pdf
關(guān)鍵詞:端到端成像;高動(dòng)態(tài)范圍成像;光場(chǎng)成像;光譜成像;無(wú)透鏡成像;低照度成像;三維成像;計(jì)算攝影
物理空間中,有著多種維度的信息,例如光源光譜,反射光譜、偏振態(tài)、三維形態(tài)、光線角度,材料性質(zhì)等。而成像系統(tǒng)所最終成得的像最終決定于,光源光譜,光源位置,物體表面材料光學(xué)性質(zhì)如雙向投射/散射/反射分布函數(shù),物體三維形態(tài)等。然而,傳統(tǒng)的光學(xué)成像依賴(lài)于以經(jīng)驗(yàn)驅(qū)動(dòng)的光學(xué)設(shè)計(jì),旨在優(yōu)化點(diǎn)擴(kuò)散函數(shù)(Point Spread Function, PSF),調(diào)制傳遞函數(shù)(MTF)等指標(biāo),目的是使得在探測(cè)器上獲得更清晰的圖像,更真實(shí)的色彩。通?!八?jiàn)即所得”,多維信息感知能力不足。隨著光學(xué)、新型光電器件、算法和計(jì)算資源的發(fā)展,可將它們?nèi)跒橐惑w的計(jì)算成像技術(shù)逐步解放了人們對(duì)物理空間中多維度信息感知的能力,與此同時(shí),隨著顯示技術(shù)的發(fā)展,特別是3D甚至6D電影,虛擬現(xiàn)實(shí)/增強(qiáng)現(xiàn)實(shí)(VR/AR)技術(shù)的發(fā)展,給多維度信息也提供了展示平臺(tái)。以目前對(duì)物理尺度限制嚴(yán)格的手機(jī)為例,使用從目前的趨勢(shì)看,手機(jī)廠商正跟學(xué)術(shù)界緊密結(jié)合。算法層面如高動(dòng)態(tài)范圍成像、低照度增強(qiáng)、色彩優(yōu)化、去馬賽克、噪聲去除甚至是重打光逐步應(yīng)用于手機(jī)中,除去傳統(tǒng)的圖像處理流程,神經(jīng)網(wǎng)絡(luò)邊緣計(jì)算在手機(jī)中日益成熟。光學(xué)層面如通過(guò)非球面乃至自由曲面透鏡優(yōu)化像差,通過(guò)優(yōu)化拜爾(Bayer)濾光片平衡進(jìn)光量和色彩。
本文圍繞端到端光學(xué)算法聯(lián)合設(shè)計(jì)、高動(dòng)態(tài)范圍成像、光場(chǎng)成像、光譜成像、無(wú)透鏡成像、偏振成像、低照度成像、主動(dòng)三維成像、計(jì)算攝影等具體實(shí)例全面闡述當(dāng)前計(jì)算成像發(fā)展現(xiàn)狀、前沿動(dòng)態(tài),熱點(diǎn)問(wèn)題、發(fā)展趨勢(shì)和應(yīng)用指導(dǎo)。任務(wù)框架如圖1所示。
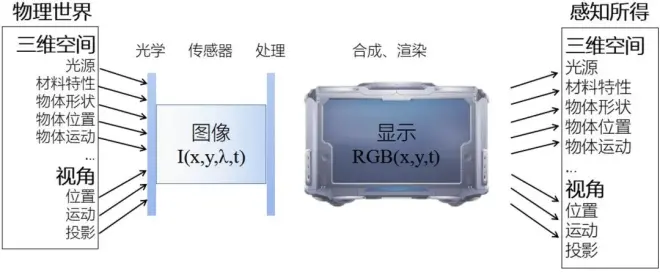
端到端光學(xué)算法聯(lián)合設(shè)計(jì)(end-to-end camera design)是近年來(lái)新興起的熱點(diǎn)分支,對(duì)一個(gè)成像系統(tǒng)而言,通過(guò)突破光學(xué)設(shè)計(jì)和圖像后處理之間的壁壘,找到光學(xué)和算法部分在硬件成本、加工可行性、體積重量、成像質(zhì)量、算法復(fù)雜度以及特殊功能間的最佳折中,從而實(shí)現(xiàn)在設(shè)計(jì)要求下的最優(yōu)方案。端到端光學(xué)算法聯(lián)合設(shè)計(jì)的突破為手機(jī)廠商、工業(yè)、車(chē)載、空天探測(cè)、國(guó)防等領(lǐng)域提供了簡(jiǎn)單化的全新解決方案,在降低光學(xué)設(shè)計(jì)對(duì)人員經(jīng)驗(yàn)依賴(lài)的同時(shí),將圖像后處理同時(shí)自動(dòng)優(yōu)化,為相機(jī)的設(shè)計(jì)提供了更多的自由度,也將輕量化、特殊功能等計(jì)算攝影問(wèn)題提供了全新的解決思路。其技術(shù)路線如圖2所示。
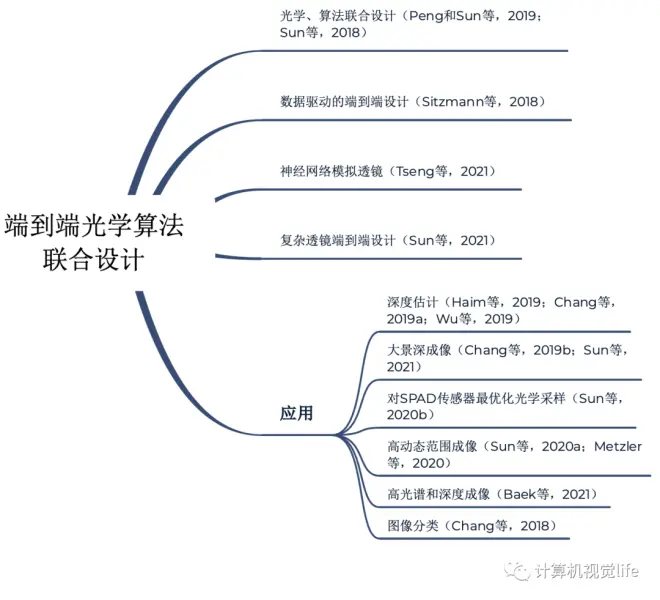
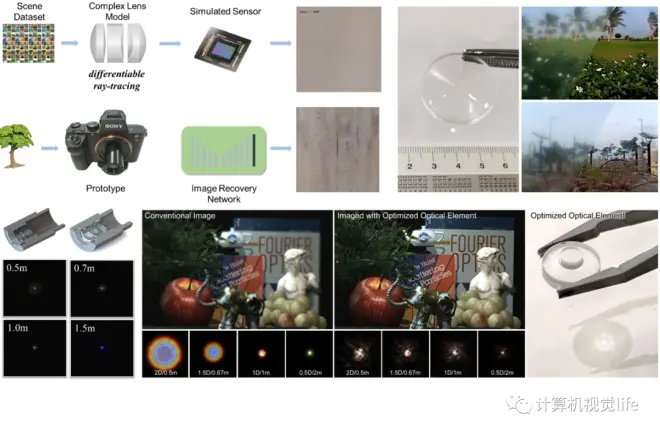
圖2?端到端光學(xué)算法聯(lián)合設(shè)計(jì)技術(shù)路線
高動(dòng)態(tài)范圍成像(high dynamic range imaging,HDR)在計(jì)算圖形學(xué)與攝影中,是用來(lái)實(shí)現(xiàn)比普通數(shù)位圖像技術(shù)更大曝光動(dòng)態(tài)范圍(最亮和最暗細(xì)節(jié)的比率)的技術(shù)。攝影中,通常用曝光值(Exposure Value,EV)的差來(lái)描述動(dòng)態(tài)范圍,1EV對(duì)應(yīng)于兩倍的曝光比例并通常被稱(chēng)為一檔(1 stops)。自然場(chǎng)景最大動(dòng)態(tài)范圍約22檔,城市夜景可達(dá)約40檔,人眼可以捕捉約10~14檔的動(dòng)態(tài)范圍。高動(dòng)態(tài)范圍成像一般指動(dòng)態(tài)范圍大于13檔或8000:1(78dB),主要包括獲取、處理、存儲(chǔ)、顯示等環(huán)節(jié)。高動(dòng)態(tài)范圍成像旨在獲取更亮和更暗處細(xì)節(jié),從而帶來(lái)更豐富的信息,更震撼的視覺(jué)沖擊力。高動(dòng)態(tài)范圍成像不僅是目前手機(jī)相機(jī)核心競(jìng)爭(zhēng)力之一,也是工業(yè)、車(chē)載相機(jī)的基本要求。其技術(shù)路線如圖3所示。
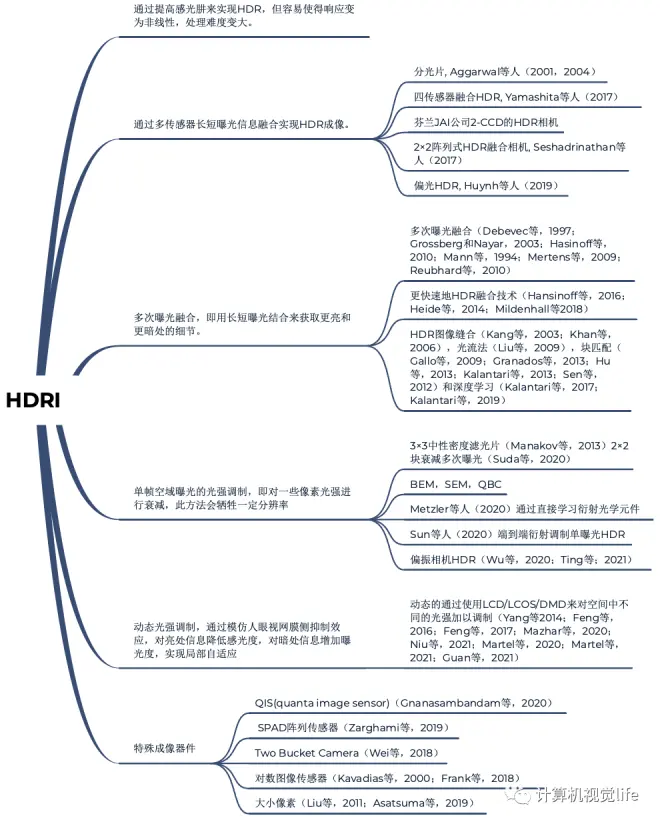
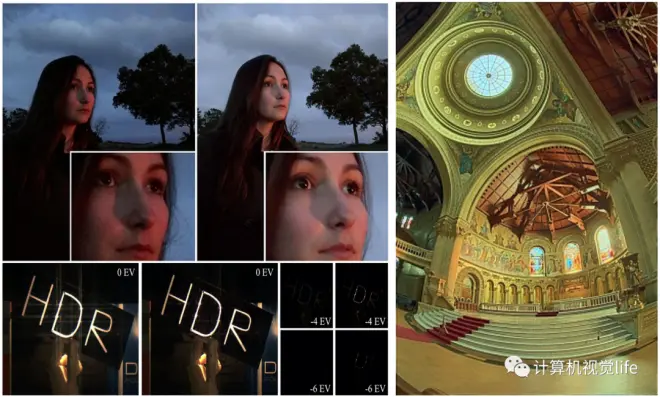
圖3?高動(dòng)態(tài)范圍成像技術(shù)路線
光場(chǎng)成像(light field imaging,LFI)能夠同時(shí)記錄光線的空間位置和角度信息,是三維測(cè)量的一種新方法。經(jīng)過(guò)近些年的發(fā)展,逐漸成為一種新興的非接觸式測(cè)量技術(shù),自從攝影被發(fā)明以來(lái),圖像捕捉就涉及在場(chǎng)景的二維投影中獲取信息。然而,光場(chǎng)不僅提供二維投影,還增加了另一個(gè)維度,即到達(dá)該投影的光線的角度。光場(chǎng)擁有關(guān)于光陣列方向和場(chǎng)景二維投影的信息,并且可以實(shí)現(xiàn)不同的功能。例如,可以將投影移動(dòng)到不同的焦距,這使用戶(hù)能夠在采集后自由地重新聚焦圖像。此外,還可以更改捕獲場(chǎng)景的視角。目前已逐漸應(yīng)用于工業(yè)、虛擬現(xiàn)實(shí)、生命科學(xué)和三維流動(dòng)測(cè)試等領(lǐng)域,幫助快速獲得真實(shí)的光場(chǎng)信息和復(fù)雜三維空間信息。其技術(shù)路線如圖4所示。
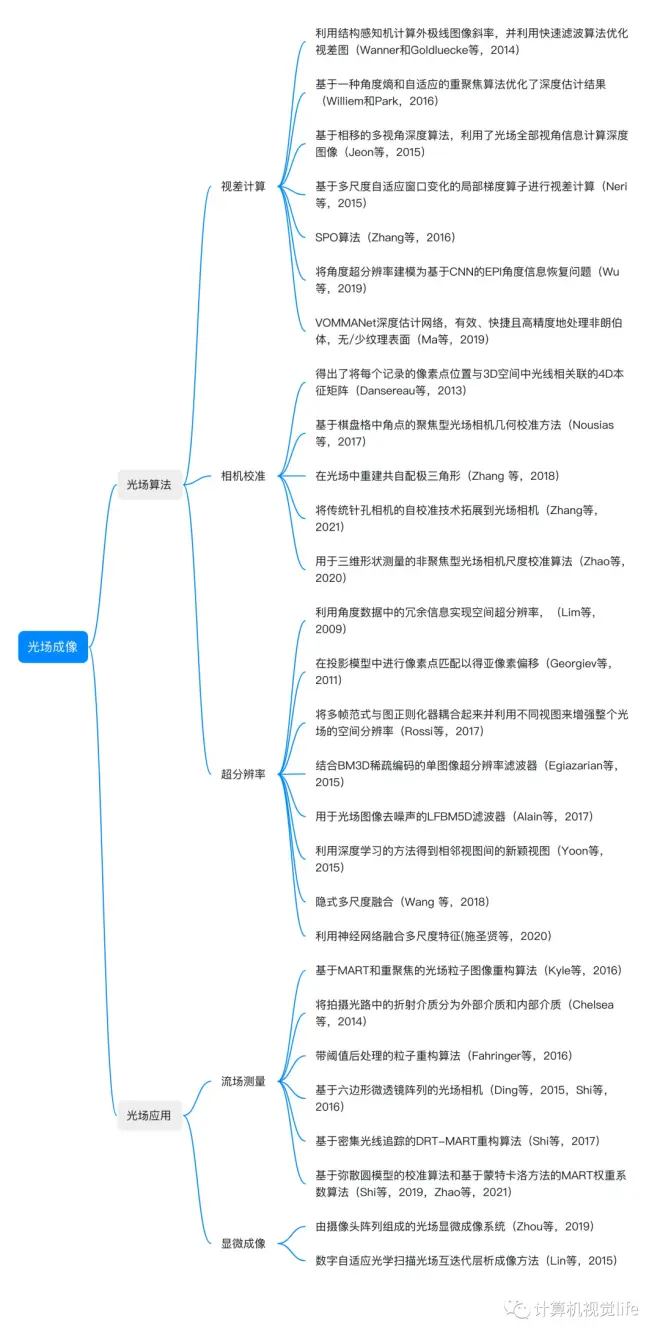
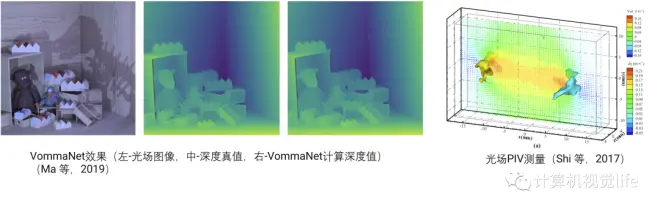
圖4 光場(chǎng)成像技術(shù)路線
圖中所列參考文獻(xiàn)(向上滑動(dòng)即可查看全部)
·光場(chǎng)算法[1]Levoy M, Zhang Z, McDowall I.Recording and controlling the 4D light field in a microscope using microlens arrays[J].//Journal of microscopy, 2009, 235(2): 144-162.[2]Cheng Z, Xiong Z, Chen C, et al. Light Field Super-Resolution: A Benchmark[C] //Proceedings of the IEEE Conference on Computer Vision and Pattern Recognition Workshops. 2019.[3]Lim J G, Ok H W, Park B K, et al. Improving the spatail resolution based on 4D light field data[C]//2009 16th IEEE International Conference on Image Processing (ICIP). IEEE, 2009: 1173-1176.[4]Georgiev T, Chunev G, Lumsdaine A.Superresolution with the focused plenoptic camera[C] //Computational Imaging IX.International Society for Optics and Photonics, 2011, 7873: 78730X.[5]Alain M, Smolic A.Light field super-resolution via LFBM5D sparse coding[C]//2018 25th IEEE international conference on image processing (ICIP).IEEE, 2018: 2501-2505.[6]Rossi M, Frossard P.Graph-based light field super-resolution[C]//2017 IEEE 19th International Workshop on Multimedia Signal Processing (MMSP).IEEE, 2017: 1-6.[7]Yoon Y, Jeon H G, Yoo D, et al. Learning a deep convolutional network for light-field image super-resolution[C]//Proceedings of the IEEE international conference on computer vision workshops. 2015: 24-32.[8]Goldluecke B.Globally consistent depth labeling of 4D light fields[C]// Computer Vision and Pattern Recognition.IEEE, 2012:41-48.[9]Wanner S, Goldluecke B.Variational Light Field Analysis for Disparity Estimation and Super-Resolution[J].//IEEE Transactions on Pattern Analysis & Machine Intelligence, 2014, 36(3):606-619.[10]Tao M W, Hadap S, Malik J, et al. Depth from Combining Defocus and Correspondence Using Light-Field Cameras[C] // IEEE International Conference on Computer Vision. IEEE, 2013:673-680.[11]Jeon H G, Park J, Choe G, et al. Accurate depth map estimation from a lenslet light field camera[C] // Computer Vision and Pattern Recognition. IEEE, 2015:1547-1555.[12]Neri A, Carli M, Battisti F.A multi-resolution approach to depth field estimation in dense image arrays[C] //IEEE International Conference on Image Processing.IEEE, 2015:3358-3362.[13]Strecke M, Alperovich A, Goldluecke B. Accurate Depth and Normal Maps from Occlusion-Aware Focal Stack Symmetry[C] //Computer Vision and Pattern Recognition. IEEE, 2017:2529-2537.[14]Dansereau D G, Pizarro O, Williams S B. Decoding, calibration and rectification for lenselet-based plenoptic cameras[C] //Proceedings of the IEEE conference on computer vision and pattern recognition. 2013: 1027-1034.[15]Nousias S, Chadebecq F, Pichat J, et al. Corner-based geometric calibration of multi-focus plenoptic cameras[C] //Proceedings of the IEEE International Conference on Computer Vision. 2017: 957-965.[16]Zhu H, Wang Q.Accurate disparity estimation in light field using ground control points[J].//Computational Visual Media, 2016, 2(2):1-9.[17]Zhang, S., Sheng, H., Li, C., Zhang, J.and Xiong, Z., 2016.Robust depth estimation for light field via spinning parallelogram operator.//Computer Vision and Image Understanding, 145, pp.148-159.[18]Zhang Y, Lv H, Liu Y, Wang H, Wang X, Huang Q, Xiang X, Dai Q.Light-field depth estimation via epipolar plane image analysis and locally linear embedding.IEEE Transactions on Circuits and Systems for Video Technology[J].2016, 27(4):739-47.[19]Ma H , ?Qian Z , ?Mu T , et al.Fast and Accurate 3D Measurement Based on Light-Field Camera and Deep Learning[J].//Sensors, 2019, 19(20):4399.·光場(chǎng)應(yīng)用[1]Lin X, Wu J, Zheng G, Dai Q. 2015. Camera array based light field microscopy. Biomedical Optics Express, 6(9): 3179-89[2]Shi, S., Ding, J., New, T.H.and Soria, J., 2017.Light-field camera-based 3D volumetric particle image velocimetry with dense ray tracing reconstruction technique.//Experiments in Fluids, 58(7), pp.1-16.[3]Shi, S., Wang, J., Ding, J., Zhao, Z.and New, T.H., 2016.Parametric study on light field volumetric particle image velocimetry.Flow Measurement and Instrumentation, 49, pp.70-88.[4]Shi, S., Ding, J., Atkinson, C., Soria, J.and New, T.H., 2018.A detailed comparison of single-camera light-field PIV and tomographic PIV.Experiments in Fluids, 59(3), pp.1-13.[5]Shi, S., Ding, J., New, T.H., Liu, Y.and Zhang, H., 2019.Volumetric calibration enhancements for single-camera light-field PIV.Experiments in Fluids, 60(1), p.21.
光譜成像(spectrum imaging)由傳統(tǒng)彩色成像技術(shù)發(fā)展而來(lái),能夠獲取目標(biāo)物體的光譜信息。每個(gè)物體都有自己獨(dú)特的光譜特征,就像每個(gè)人擁有不同的指紋一樣,光譜也因此被視為目標(biāo)識(shí)別的“指紋”信息。通過(guò)獲取目標(biāo)物體在連續(xù)窄波段內(nèi)的光譜圖像,組成空間維度和光譜維度的數(shù)據(jù)立方體信息,可以極大地增強(qiáng)目標(biāo)識(shí)別和分析能力。光譜成像可作為科學(xué)研究、工程應(yīng)用的強(qiáng)有力工具,已經(jīng)廣泛應(yīng)用于軍事、工業(yè)、民用等諸多領(lǐng)域,對(duì)促進(jìn)社會(huì)經(jīng)濟(jì)發(fā)展和保障國(guó)家安全具有重要作用。例如,光譜成像對(duì)河流、沙土、植被、巖礦等地物都具有很好的識(shí)別效果,因此在精準(zhǔn)農(nóng)業(yè)、環(huán)境監(jiān)控、資源勘查、食品安全等諸多方面都具有重要應(yīng)用。特別地,光譜成像還有望用于手機(jī)、自動(dòng)駕駛汽車(chē)等終端。當(dāng)前,光譜成像已成為計(jì)算機(jī)視覺(jué)和圖形學(xué)研究的熱點(diǎn)方向之一。
無(wú)透鏡成像(lensless imaging)技術(shù)為進(jìn)一步壓縮成像系統(tǒng)的尺寸提供了一種全新的思路(Boominathan等,2022)。傳統(tǒng)的成像系統(tǒng)依賴(lài)點(diǎn)對(duì)點(diǎn)的成像模式,其系統(tǒng)極限尺寸仍受限于透鏡的焦距、孔徑、視場(chǎng)等核心指標(biāo)。無(wú)透鏡成像摒棄了傳統(tǒng)透鏡中點(diǎn)對(duì)點(diǎn)的映射模式,而是將物空間的點(diǎn)投影為像空間的特定圖案,不同物點(diǎn)在像面疊加編碼,形成了一種人眼無(wú)法識(shí)別,但計(jì)算算法可以通過(guò)解碼復(fù)原圖像信息。其在緊湊性方面具有極強(qiáng)的競(jìng)爭(zhēng)力,而且隨著解碼算法的發(fā)展,其成像分辨率也得到大大提升。因此,在可穿戴相機(jī)、便攜式顯微鏡、內(nèi)窺鏡、物聯(lián)網(wǎng)等應(yīng)用領(lǐng)域極具發(fā)展?jié)摿?。另外,其?dú)特的光學(xué)加密功能,能夠?qū)δ繕?biāo)中敏感的生物識(shí)別特征進(jìn)行有效保護(hù),在隱私保護(hù)的人工智能成像方面也具有重要意義。
低光照成像(low light imaging)也是計(jì)算攝影里的研究熱點(diǎn)一。手機(jī)攝影已經(jīng)成為了人們用來(lái)記錄生活的最常用的方式之一,手機(jī)的攝像功能也是每次發(fā)布會(huì)的看點(diǎn),夜景模式也成了各大手機(jī)廠商爭(zhēng)奪的技術(shù)制高點(diǎn)。不同手機(jī)的相機(jī)在白天的強(qiáng)光環(huán)境下拍照差異并不明顯,然而在夜晚弱光情況下則差距明顯。其原因是,成像依賴(lài)于鏡頭收集物體發(fā)出的光子,且傳感器由光電轉(zhuǎn)換、增益、模數(shù)轉(zhuǎn)換一系列過(guò)程會(huì)有不可避免的噪聲;白天光線充足,信號(hào)的信噪比高,成像質(zhì)量很高;晚上光線微弱,信號(hào)的信噪比下降數(shù)個(gè)數(shù)量級(jí),成像質(zhì)量低;部分手機(jī)搭載使用計(jì)算攝影算法的夜景模式,比如基于單幀、多幀、RYYB陣列等的去噪,有效地提高了照片的質(zhì)量。但目前依舊有很大的提升空間。低光照成像按照輸入分類(lèi)可以分為單幀輸入、多幀輸入( burst imaging)、 閃光燈輔助拍攝和傳感器技術(shù),技術(shù)路線如圖2所示。技術(shù)路線如圖5所示。
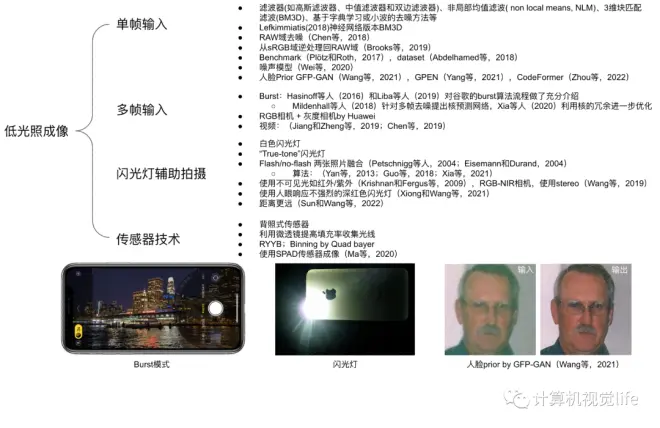
圖?5 低光照成像技術(shù)路線
圖中所列參考文獻(xiàn)(向上滑動(dòng)即可查看全部)
·單幀輸入
[1]Lefkimmiatis, S., 2018. Universal denoising networks: a novel CNN architecture for image denoising. In Proceedings of the IEEE conference on computer vision and pattern recognition (pp. 3204-3213).
[2]Chen, C., Chen, Q., Xu, J. and Koltun, V., 2018. Learning to see in the dark. In Proceedings of the IEEE conference on computer vision and pattern recognition (pp. 3291-3300)
[3]Brooks, T., Mildenhall, B., Xue, T., Chen, J., Sharlet, D. and Barron, J.T., 2019. Unprocessing images for learned raw denoising. In Proceedings of the IEEE/CVF Conference on Computer Vision and Pattern Recognition (pp. 11036-11045).
[4]Plotz, T. and Roth, S., 2017. Benchmarking denoising algorithms with real photographs. In Proceedings of the IEEE conference on computer vision and pattern recognition (pp. 1586-1595).
[5]Abdelhamed, A., Lin, S. and Brown, M.S., 2018. A high-quality denoising dataset for smartphone cameras. In Proceedings of the IEEE Conference on Computer Vision and Pattern Recognition (pp. 1692-1700).
[6]Wei, K., Fu, Y., Yang, J. and Huang, H., 2020. A physics-based noise formation model for extreme low-light raw denoising. In Proceedings of the IEEE/CVF Conference on Computer Vision and Pattern Recognition (pp. 2758-2767).
[7]Wang, X., Li, Y., Zhang, H. and Shan, Y., 2021. Towards real-world blind face restoration with generative facial prior. In Proceedings of the IEEE/CVF Conference on Computer Vision and Pattern Recognition (pp. 9168-9178).
[8]Yang, T., Ren, P., Xie, X. and Zhang, L., 2021. Gan prior embedded network for blind face restoration in the wild. In Proceedings of the IEEE/CVF Conference on Computer Vision and Pattern Recognition (pp. 672-681).
[9]Zhou, S., Chan, K.C., Li, C. and Loy, C.C., 2022. Towards Robust Blind Face Restoration with Codebook Lookup Transformer. arXiv preprint arXiv:2206.11253.
·多幀輸入
[1]Hasinoff, S.W., Sharlet, D., Geiss, R., Adams, A., Barron, J.T., Kainz, F., Chen, J. and Levoy, M., 2016. Burst photography for high dynamic range and low-light imaging on mobile cameras. ACM Transactions on Graphics (ToG), 35(6), pp.1-12.
[2]Liba, O., Murthy, K., Tsai, Y.T., Brooks, T., Xue, T., Karnad, N., He, Q., Barron, J.T., Sharlet, D., Geiss, R. and Hasinoff, S.W., 2019. Handheld mobile photography in very low light. ACM Trans. Graph., 38(6), pp.164-1.
[3]Mildenhall, B., Barron, J.T., Chen, J., Sharlet, D., Ng, R. and Carroll, R., 2018. Burst denoising with kernel prediction networks. In Proceedings of the IEEE conference on computer vision and pattern recognition (pp. 2502-2510).
[4]Xia, Z., Perazzi, F., Gharbi, M., Sunkavalli, K. and Chakrabarti, A., 2020. Basis prediction networks for effective burst denoising with large kernels. In Proceedings of the IEEE/CVF Conference on Computer Vision and Pattern Recognition (pp. 11844-11853).
[5]Jiang, H. and Zheng, Y., 2019. Learning to see moving objects in the dark. In Proceedings of the IEEE/CVF International Conference on Computer Vision (pp. 7324-7333).
[6]Chen, C., Chen, Q., Do, M.N. and Koltun, V., 2019. Seeing motion in the dark. In Proceedings of the IEEE/CVF International Conference on Computer Vision (pp. 3185-3194).
·閃光燈
[1]Eisemann, E. and Durand, F., 2004. Flash photography enhancement via intrinsic relighting. ACM transactions on graphics (TOG), 23(3), pp.673-678.
[2]Petschnigg, G., Szeliski, R., Agrawala, M., Cohen, M., Hoppe, H. and Toyama, K., 2004. Digital photography with flash and no-flash image pairs. ACM transactions on graphics (TOG), 23(3), pp.664-672.
[3]Yan, Q., Shen, X., Xu, L., Zhuo, S., Zhang, X., Shen, L. and Jia, J., 2013. Cross-field joint image restoration via scale map. In Proceedings of the IEEE International Conference on Computer Vision (pp. 1537-1544).
[4]Guo, X., Li, Y., Ma, J. and Ling, H., 2018. Mutually guided image filtering. IEEE transactions on pattern analysis and machine intelligence, 42(3), pp.694-707.
[5]Xia, Z., Gharbi, M., Perazzi, F., Sunkavalli, K. and Chakrabarti, A., 2021. Deep Denoising of Flash and No-Flash Pairs for Photography in Low-Light Environments. In Proceedings of the IEEE/CVF Conference on Computer Vision and Pattern Recognition (pp. 2063-2072).
[6]Krishnan, D. and Fergus, R., 2009. Dark flash photography. ACM Trans. Graph., 28(3), p.96.
[7]Wang, J., Xue, T., Barron, J.T. and Chen, J., 2019, May. Stereoscopic dark flash for low-light photography. In 2019 IEEE International Conference on Computational Photography (ICCP) (pp. 1-10). IEEE.
[8]Xiong, J., Wang, J., Heidrich, W. and Nayar, S., 2021. Seeing in extra darkness using a deep-red flash. In Proceedings of the IEEE/CVF Conference on Computer Vision and Pattern Recognition (pp. 10000-10009).
[9]Sun, Z., Wang, J., Wu, Y. and Nayar, S., 2022. Seeing Far in the Dark with Patterned Flash, In European Conference on Computer Vision. Springer?
·傳感器
[1]Ma, S., Gupta, S., Ulku, A.C., Bruschini, C., Charbon, E. and Gupta, M., 2020. Quanta burst photography. ACM Transactions on Graphics (TOG), 39(4), pp.79-1.
主動(dòng)三維成像(active 3D imaging)以獲取物體或場(chǎng)景的點(diǎn)云為目的,被動(dòng)方法以雙目立體匹配為代表,但難以解決無(wú)紋理區(qū)域和有重復(fù)紋理區(qū)域的深度。主動(dòng)光方法一般更為魯棒,能夠在暗處工作,且能夠得到稠密的、精確的點(diǎn)云。主動(dòng)光方法根據(jù)使用的光的性質(zhì)可分為基于光的直線傳播如結(jié)構(gòu)光,基于光速如Time-of-fligt(TOF),包括連續(xù)波TOF(iTOF)和直接TOF(dTOF),和基于光的波的性質(zhì)如干涉儀,其中前兩種方法的主動(dòng)三維成像已廣泛使用在人們的日常生活中。雖然主動(dòng)方法通過(guò)打光的方式提高了準(zhǔn)確性,但也存在由于環(huán)境光(主要是太陽(yáng)光)、多路徑干擾(又稱(chēng)做非直接光干擾)引起的問(wèn)題,這些都在近些年的研究過(guò)程中有了很大的進(jìn)展,如圖6和圖7所示。
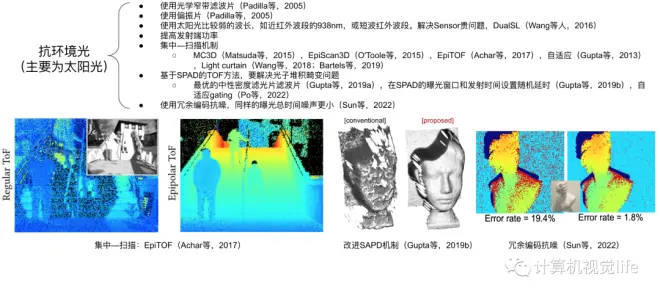
圖?6 抗環(huán)境光技術(shù)路線
圖中所列參考文獻(xiàn)(向上滑動(dòng)即可查看全部)
[1]Padilla, D.D. and Davidson, P., 2005. Advancements in sensing and perception using structured lighting techniques: An ldrd final report.
[2]Wang, J., Sankaranarayanan, A.C., Gupta, M. and Narasimhan, S.G., 2016, October. Dual structured light 3d using a 1d sensor. In European Conference on Computer Vision (pp. 383-398). Springer
[3]Matsuda, N., Cossairt, O. and Gupta, M., 2015, April. Mc3d: Motion contrast 3d scanning. In 2015 IEEE International Conference on Computational Photography (ICCP) (pp. 1-10). IEEE.
[4]O'Toole, M., Achar, S., Narasimhan, S.G. and Kutulakos, K.N., 2015. Homogeneous codes for energy-efficient illumination and imaging. ACM Transactions on Graphics (ToG), 34(4), pp.1-13.
[5]Supreeth Achar, Joseph R. Bartels, William L. ‘Red’ Whittaker, Kiriakos N. Kutulakos, Srinivasa G. Narasimhan. 2017, "Epipolar Time-of-Flight Imaging", ACM SIGGRAPH?
[6]Gupta, M., Yin, Q. and Nayar, S.K., 2013. Structured light in sunlight. In Proceedings of the IEEE International Conference on Computer Vision (pp. 545-552).
[7]Wang, J., Bartels, J., Whittaker, W., Sankaranarayanan, A.C. and Narasimhan, S.G., 2018. Programmable triangulation light curtains. In Proceedings of the European Conference on Computer Vision (ECCV) (pp. 19-34).
[8]Bartels, J.R., Wang, J., Whittaker, W. and Narasimhan, S.G., 2019. Agile depth sensing using triangulation light curtains. In Proceedings of the IEEE/CVF International Conference on Computer Vision (pp. 7900-7908).
[9]Gupta, A., Ingle, A., Velten, A. and Gupta, M., 2019. Photon-flooded single-photon 3D cameras. In Proceedings of the IEEE/CVF Conference on Computer Vision and Pattern Recognition (pp. 6770-6779).
[10]Gupta, A., Ingle, A. and Gupta, M., 2019. Asynchronous single-photon 3D imaging. In Proceedings of the IEEE/CVF International Conference on Computer Vision (pp. 7909-7918).
[11]Po, R., Pediredla, A. and Gkioulekas, I., 2022. Adaptive Gating for Single-Photon 3D Imaging. In Proceedings of the IEEE/CVF Conference on Computer Vision and Pattern Recognition (pp. 16354-16363).
[12]Sun, Z., Zhang, Y., Wu, Y., Huo, D., Qian, Y. and Wang, J., 2022. Structured Light with Redundancy Codes. arXiv preprint arXiv:2206.09243.
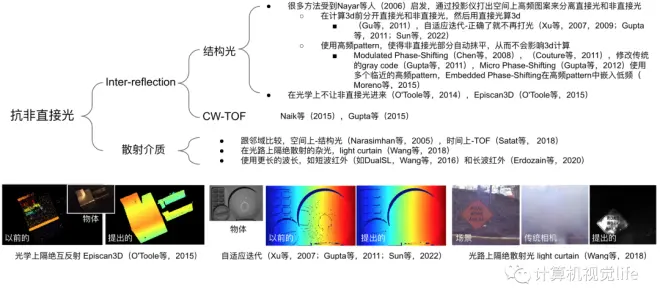
圖?7 抗非直接光技術(shù)路線
圖中所列參考文獻(xiàn)(向上滑動(dòng)即可查看全部)
[1]Nayar, S.K., Krishnan, G., Grossberg, M.D. and Raskar, R., 2006. Fast separation of direct and global components of a scene using high frequency illumination. In ACM SIGGRAPH 2006 Papers (pp. 935-944).
[2]Gu, J., Kobayashi, T., Gupta, M. and Nayar, S.K., 2011, November. Multiplexed illumination for scene recovery in the presence of global illumination. In 2011 International Conference on Computer Vision (pp. 691-698). IEEE.
[3]Xu, Y. and Aliaga, D.G., 2007, May. Robust pixel classification for 3d modeling with structured light. In Proceedings of Graphics Interface 2007 (pp. 233-240).
[4]Xu, Y. and Aliaga, D.G., 2009. An adaptive correspondence algorithm for modeling scenes with strong interreflections. IEEE Transactions on Visualization and Computer Graphics, 15(3), pp.465-480.
[5]Gupta, M., Agrawal, A., Veeraraghavan, A. and Narasimhan, S.G., 2011, June. Structured light 3D scanning in the presence of global illumination. In CVPR 2011 (pp. 713-720). IEEE.
[6]Sun, Z., Zhang, Y., Wu, Y., Huo, D., Qian, Y. and Wang, J., 2022. Structured Light with Redundancy Codes. arXiv preprint arXiv:2206.09243.
[7]Chen, T., Seidel, H.P. and Lensch, H.P., 2008, June. Modulated phase-shifting for 3D scanning. In 2008 IEEE Conference on Computer Vision and Pattern Recognition (pp. 1-8). IEEE.
[8]Couture, V., Martin, N. and Roy, S., 2011, November. Unstructured light scanning to overcome interreflections. In 2011 International Conference on Computer Vision (pp. 1895-1902). IEEE.
[9]Gupta, M. and Nayar, S.K., 2012, June. Micro phase shifting. In 2012 IEEE Conference on Computer Vision and Pattern Recognition (pp. 813-820). IEEE.
[10]Moreno, D., Son, K. and Taubin, G., 2015. Embedded phase shifting: Robust phase shifting with embedded signals. In Proceedings of the IEEE Conference on Computer Vision and Pattern Recognition (pp. 2301-2309).
[11]O'Toole, M., Mather, J. and Kutulakos, K.N., 2014. 3d shape and indirect appearance by structured light transport. In Proceedings of the IEEE Conference on Computer Vision and Pattern Recognition (pp. 3246-3253).
[12]O'Toole, M., Achar, S., Narasimhan, S.G. and Kutulakos, K.N., 2015. Homogeneous codes for energy-efficient illumination and imaging. ACM Transactions on Graphics (ToG), 34(4), pp.1-13.
[13]Wang, J., Bartels, J., Whittaker, W., Sankaranarayanan, A.C. and Narasimhan, S.G., 2018. Programmable triangulation light curtains. In Proceedings of the European Conference on Computer Vision (ECCV) (pp. 19-34).
[14]Naik, N., Kadambi, A., Rhemann, C., Izadi, S., Raskar, R. and Bing Kang, S., 2015. A light transport model for mitigating multipath interference in time-of-flight sensors. In Proceedings of the IEEE Conference on Computer Vision and Pattern Recognition (pp. 73-81).
[15]Gupta, M., Nayar, S.K., Hullin, M.B. and Martin, J., 2015. Phasor imaging: A generalization of correlation-based time-of-flight imaging. ACM Transactions on Graphics (ToG), 34(5), pp.1-18.
[16]Narasimhan, S.G., Nayar, S.K., Sun, B. and Koppal, S.J., 2005, October. Structured light in scattering media. In Tenth IEEE International Conference on Computer Vision (ICCV'05) Volume 1 (Vol. 1, pp. 420-427). IEEE.
[17]Satat, G., Tancik, M. and Raskar, R., 2018, May. Towards photography through realistic fog. In 2018 IEEE International Conference on Computational Photography (ICCP) (pp. 1-10). IEEE.
[18]Wang, J., Sankaranarayanan, A.C., Gupta, M. and Narasimhan, S.G., 2016, October. Dual structured light 3d using a 1d sensor. In European Conference on Computer Vision (pp. 383-398). Springer.
[19]Erdozain, J., Ichimaru, K., Maeda, T., Kawasaki, H., Raskar, R. and Kadambi, A., 2020, October. 3d Imaging For Thermal Cameras Using Structured Light. In 2020 IEEE International Conference on Image Processing (ICIP) (pp. 2795-2799). IEEE.
計(jì)算攝影學(xué)(computational photography)是計(jì)算成像的一個(gè)分支學(xué)科,它從傳統(tǒng)攝影學(xué)發(fā)展而來(lái)。傳統(tǒng)攝影學(xué)主要著眼于使用光學(xué)器件更好地進(jìn)行成像,如佳能、索尼等相機(jī)廠商對(duì)于鏡頭的研究;與之相比,計(jì)算攝影學(xué)則更側(cè)重于使用數(shù)字計(jì)算的方式進(jìn)行圖像拍攝。在過(guò)去10年中,隨著移動(dòng)端設(shè)備計(jì)算能力的迅速發(fā)展,手機(jī)攝影逐漸成為了計(jì)算攝影學(xué)研究的主要方向:在光學(xué)鏡片的物理尺寸、成像質(zhì)量受限的情況下,如何使用合理的計(jì)算資源,繪制出用戶(hù)最滿(mǎn)意的圖像。計(jì)算攝影學(xué)在近年來(lái)得到了長(zhǎng)足的發(fā)展,其研究問(wèn)題的范圍也所有擴(kuò)展,如:夜空攝影、人臉重光照、照片自動(dòng)美化等。受圖像的算法,其中重點(diǎn)介紹:自動(dòng)白平衡、自動(dòng)對(duì)焦、人工景深模擬以及連拍攝影。篇幅所限,本報(bào)告中僅介紹目標(biāo)為還原拍攝真實(shí)場(chǎng)景的真實(shí)信息的相關(guān)研究。
獨(dú)家重磅課程官網(wǎng):cvlife.net
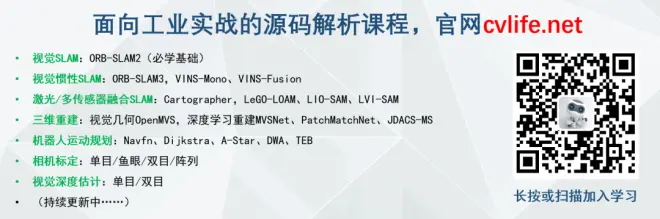
全國(guó)最大的機(jī)器人SLAM開(kāi)發(fā)者社區(qū)
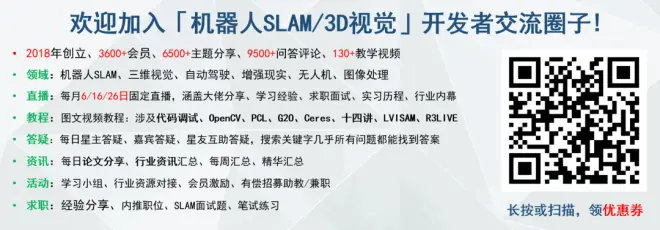
技術(shù)交流群
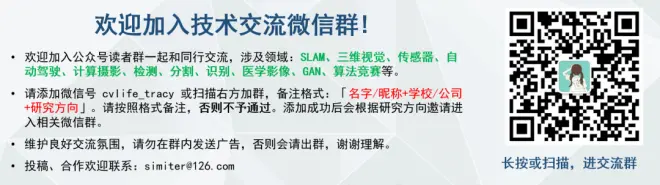
—? ?版權(quán)聲明? —
本公眾號(hào)原創(chuàng)內(nèi)容版權(quán)屬計(jì)算機(jī)視覺(jué)life所有;從公開(kāi)渠道收集、整理及授權(quán)轉(zhuǎn)載的非原創(chuàng)文字、圖片和音視頻資料,版權(quán)屬原作者。如果侵權(quán),請(qǐng)聯(lián)系我們,會(huì)及時(shí)刪除。