銀行案例學(xué)習(xí)實(shí)例2_數(shù)據(jù)細(xì)分和衍生變量
up主微信公眾號pythonEducation
python金融風(fēng)控評分卡模型和數(shù)據(jù)分析微專業(yè)課:http://dwz.date/b9vv
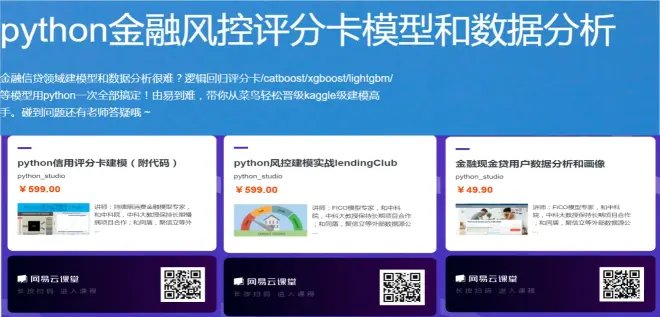
Sherlock Holmes & Data Visualization
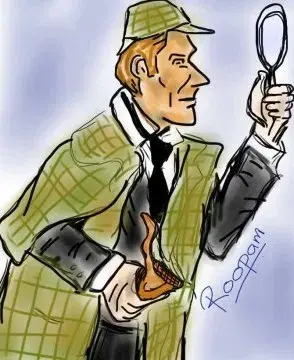
Sherlock Holmes – by Roopam
As a kid, a friend of mine used to own a Sherlock Holmes toy kit – the source of envy for all the other friends. The kit had a Sherlock Holmes cap, a pipe, a watch and a magnifying glass. The magnifying glass was the most coveted item in the kit. The pleasure of focusing the magnifying glass on an object and seeing it in detail to derive meaning was my first lesson in detective investigation – something that I still relish as an analyst. This is also the core of data visualization. Later, I learned more about Mr. Holmes through the books written by Sir Arthur Conon Doyle. The first book, A Study in Scarlet, describes Mr. Holmes’ inclination for scientific knowledge and the science of deduction – analysis. I realized being a detective is no different from being an experimental scientist or analyst. You start with gathering a set of observations, using which you built your case through logic and deduction. The following quote by Mr. Holmes’ perfectly describes the process of investigation – when you have eliminated the impossible, whatever remains, however improbable, must be the truth
小時候,我的一個朋友曾經(jīng)擁有一個夏洛克·福爾摩斯玩具包 - 這是所有其他朋友羨慕的源頭。該套件有一個Sherlock Holmes帽,一個管子,一塊手表和一個放大鏡。放大鏡是套件中最令人垂涎的項(xiàng)目。將放大鏡聚焦在物體上并詳細(xì)觀察它以獲得意義的樂趣是我在偵探調(diào)查中的第一課 - 我仍然喜歡作為分析師。這也是數(shù)據(jù)可視化的核心。后來,我通過阿瑟·康恩·道爾爵士寫的書,更多地了解了霍姆斯先生。第一本書“血色研究”描述了霍姆斯先生對科學(xué)知識和演繹科學(xué)的傾向 - 分析。我意識到自己是一名偵探與做實(shí)驗(yàn)科學(xué)家或分析師并無二致。您首先收集一組觀察結(jié)果,通過邏輯和演繹來構(gòu)建您的案例?;裟匪瓜壬囊韵乱酝昝赖孛枋隽苏{(diào)查的過程 - 當(dāng)你消除了不可能的事物時,無論遺骸多么不可能,都必須是真理
Data Visualization – A Case Study Example
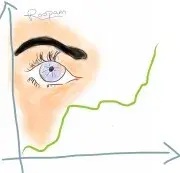
In our last article, we started with a case study example about CyndiCat bank that has disbursed 60816 auto loans in the quarter between April–June 2012. You were playing the role of the Chief Risk Officer (CRO) for this bank. Additionally, you had noticed around 2.5% of bad rate or 1524 bad loans out of total 60816 disbursed loans. You started with a hunch預(yù)感/直覺 about the?relationship between the age of the borrowers and the bad rates. After your analysis, you observed a?definitive inversely proportional relationship between the two. Age of the borrowers certainly seemed like a strong contender for your credit risk model. You are feeling good and want to find a few more variables for your multivariate model.?(Read the previous article)
在我們的上一篇文章中,我們從一個關(guān)于CyndiCat銀行的案例研究示例開始,該銀行在2012年4月至6月期間在該季度發(fā)放了60816個汽車貸款。您擔(dān)任該銀行首席風(fēng)險官(CRO)的角色。 此外,在60816筆已發(fā)放貸款中,您注意到約2.5%的不良利率或1524筆不良貸款。?你開始預(yù)感借款人的年齡與不良利率之間的關(guān)系。?在分析之后,您觀察到兩者之間的確定的反比關(guān)系。?借款人的年齡當(dāng)然似乎是您信用風(fēng)險模型的有力競爭者。 您感覺良好,并希望為您的多變量模型找到更多變量。 (閱讀上一篇文章)
國內(nèi)某些數(shù)據(jù)測試,年齡與?壞客戶率成正比,也許還有第三方因素決定,比如政策法規(guī)。美國政策嚴(yán)格。
?
The Case Study Example Continues…
You also believe that income of the applicants should have some sort of relationship with the bad rates. You are feeling confident about your understanding of the tools you have used last time around i.e. histogram and normalized histogram (overlaid with good / bad borrowers). You immediately start by plotting an equal interval histogram and observe the following:
您還認(rèn)為申請人的收入應(yīng)該與不良利率有某種關(guān)系。 您對自己對上次使用的工具的理解充滿信心,即直方圖和標(biāo)準(zhǔn)化直方圖(覆蓋好/壞借款人)。 您可以立即繪制相等的間隔直方圖,并觀察以下內(nèi)容:
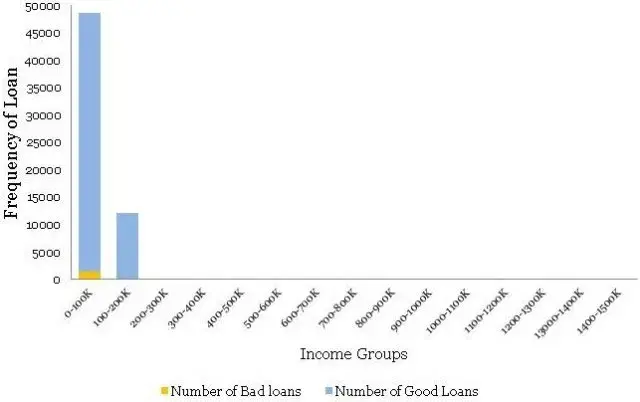
Ouch! This is nothing like the smooth bell curve histogram you have observed for the age groups. Even the normalized histogram, shown below, is completely uninformative.
哎喲! 這與您在年齡組中觀察到的平滑鐘形曲線直方圖完全不同。 即使是如下所示的標(biāo)準(zhǔn)化直方圖也完全無法提供信息。
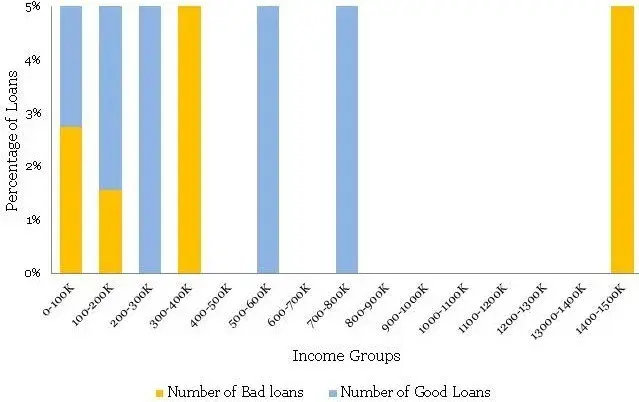
So, what is going on here? Income, unlike age, has a few extreme outliers – almost invisible in the histogram. There is a High-Net worth-Individual (HNI) with $1.47 million annual salary and few other outliers in the middle. Incidentally, this loan to the HNI customer has gone bad – quite unfortunate for the Bank. Have a look at the distribution table – almost 99.8% population is in the first two income buckets.
那么,這里發(fā)生了什么? 與年齡不同,收入有一些極端異常值 - 在直方圖中幾乎不可見。 有一個高凈值個人(HNI),年薪為147萬美元,中間幾乎沒有其他異常值。 順便提一下,這筆給HNI客戶的貸款變壞了 - 這對銀行來說非常不幸。 看一下分配表 - 幾乎99.8%的人口都在前兩個收入桶中。
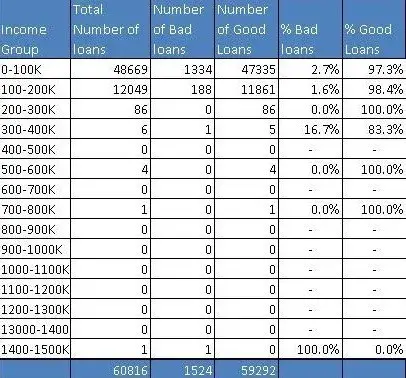
Here, as an analyst, you need to take a call whether you want to include these extreme cases, with thin data, in your model or create an income boundary for which the model is applicable for the majority of the customers. In my opinion, the latter option is a prudent choice. Going further with your exploratory analysis and data visualization,?you have decided to zoom into the regions with a predominant number of data points?i.e. first two buckets and re-plotted the histogram. The following is what you observed
在這里,作為分析師,您需要接聽電話,無論您是想在模型中包含這些具有少量數(shù)據(jù)的極端情況,還是創(chuàng)建適用于大多數(shù)客戶的模型的收入邊界。 在我看來,后一種選擇是謹(jǐn)慎的選擇。 進(jìn)一步研究探索性分析和數(shù)據(jù)可視化,您決定放大(細(xì)分)具有大量數(shù)據(jù)點(diǎn)的區(qū)域,即前兩個數(shù)據(jù)區(qū)域,并重新繪制直方圖。?以下是您觀察到的內(nèi)容
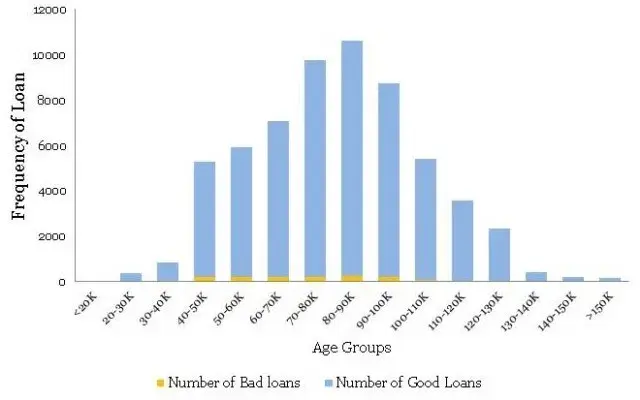
* Correction: Read X axis as Income Groups (not Age Groups)
This time, the histogram is reasonably smooth and hence does not require transformation. Presented below is the normalized histogram for the above histogram.
這次,直方圖相當(dāng)平滑,因此不需要變換。 下面給出的是上述直方圖的歸一化直方圖。
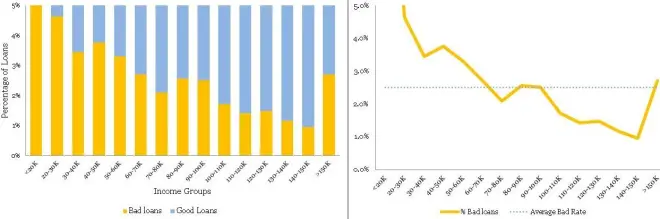
The following conclusions can be drawn from the above
? There is a definite trend in terms of the bad rates and the income groups. As the borrowers are earning a higher salary, they are less likely to default on their loans. This seems like a good insight.
? For the Last bucket i.e. >150 K, the risk jumps up – a break in the trend. This is attributed to the thin data in this bucket – not just in terms of data count but this data is also?spread across a very large interval 150 to 1500 K.
Now you have two variables that are possible governing bad rates for the borrowers – age and income. However, your further analysis of income with age shows that there is a high correlation between the two variables – 0.76 to be precise. You cannot use them both in the model because it will be problematic because of multicollinearity. The correlation between age and income makes sense. Since income is a function of years of experience for a professional, this further depends on upon her age. Hence, you have decided to drop income from the model. The leaves us with a question, is there a way of bringing income back in our multivariate model?
在不良利率和收入群體方面存在明顯的趨勢。由于借款人的薪水較高,他們不太可能拖欠貸款。這似乎是一個很好的見解。
?對于最后一桶,即> 150 K,風(fēng)險會上升 - 趨勢中斷。這歸因于此數(shù)據(jù)庫中的數(shù)據(jù)太少?- 不僅僅是數(shù)據(jù)計數(shù),而且這些數(shù)據(jù)也分布在150到1500 K的非常大的區(qū)間內(nèi)。
現(xiàn)在你有兩個變量可以控制借款人的不良利率 - 年齡和收入。然而,您對年齡收入的進(jìn)一步分析表明,兩個變量之間存在高度相關(guān)性 - 準(zhǔn)確地說是0.76。你不能在模型中使用它們,因?yàn)樗鼤蚨嘀毓簿€性而成為問題。年齡和收入之間的相關(guān)性是有道理的。由于收入是專業(yè)人士多年經(jīng)驗(yàn)的函數(shù),這進(jìn)一步取決于她的年齡。因此,您決定從模型中減少收入。給我們留下一個問題,是否有辦法將收入帶回我們的多元模型?
Financial Ratios
組合變量-Fixed Obligation to Income Ratio固定債務(wù)和收入比率 (FOIR)?
When corporate analysts try to analyze financials of a company they often work with several financial ratios. Working with ratios has a definite advantage over working with plain vanilla variables. Combined variables often convey much higher information. Seasoned analysts understand this really well. Moreover, variables creation is a creative exercise that requires sound domain knowledge. For credit analysis, the ratio of the sum of obligations to income is highly informative since this provides an insight about percentage disposable income for the borrower.
Let us try to understand this with an example. Susan has an annual income of 100 thousand dollars. She has a home loan with an annual obligation (EMI) of 40 thousand dollars and a car loan with 10 thousand dollars. Hence, she is spending 10+40 thousand dollars on paying the EMIs out of her income of 100 thousand dollars. Her?Fixed Obligation to Income Ratio固定債務(wù)和收入比率 (FOIR)?in this case is equal to 50/100 = 50%. She is left with just 50% of her income to run her other expenses.
The following is the normalized histogram plot for FOIR.
財務(wù)比率
當(dāng)企業(yè)分析師試圖分析公司的財務(wù)狀況時,他們經(jīng)常使用多種財務(wù)比率。使用比率與使用普通的vanilla變量相比具有明顯的優(yōu)勢。組合變量通常會傳達(dá)更高的信息。經(jīng)驗(yàn)豐富的分析師非常了解這一點(diǎn)。此外,變量創(chuàng)建是一項(xiàng)創(chuàng)造性的練習(xí),需要良好的領(lǐng)域知識。對于信用分析,債務(wù)與收入之和的比率具有很高的信息量,因?yàn)檫@可以提供有關(guān)借款人可支配收入百分比的見解。
讓我們試著通過一個例子來理解這一點(diǎn)。蘇珊的年收入為10萬美元。她有一筆住房貸款,年度義務(wù)(EMI)為4萬美元,汽車貸款為1萬美元。因此,她花費(fèi)10 + 4萬美元從她的收入10萬美元中支付EMI。在這種情況下,她的固定義務(wù)收入比率(FOIR)等于50/100 = 50%。她只有50%的收入用于支付其他費(fèi)用。
以下是FOIR的標(biāo)準(zhǔn)化直方圖。
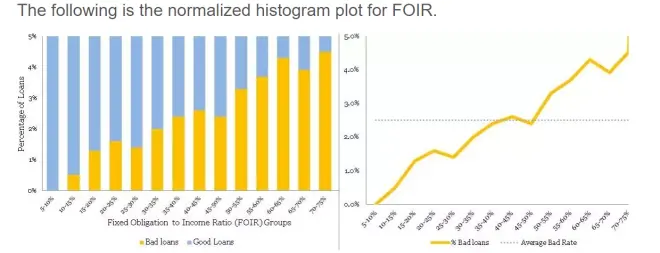
Clearly, there is a directly proportional relationship between FOIR and bad rate. Additionally, FOIR has little correlation with age, just 0.18. Now, you have another variable FOIR , along with age, for your multivariate model. Congratulations! Like, Sherlock Holmes, you are building your case evidence by evidence – a process in science.
Sign-off Note
I hope after this you are feeling inspired to pick up the magnifying glass and follow the legacy of the great Sherlock Holmes –?this time?the mystery is hiding in data!
顯然,FOIR與不良率之間存在直接比例關(guān)系。 此外,F(xiàn)OIR與年齡幾乎沒有相關(guān)性,僅為0.18。?現(xiàn)在,您的多變量模型還有另一個變量FOIR和年齡。恭喜! 就像Sherlock Holmes一樣,你正在通過證據(jù)建立你的案件證據(jù) - 一個科學(xué)過程。
簽字筆記
我希望在此之后你會感到鼓舞,拿起放大鏡并追隨偉大的夏洛克福爾摩斯的遺產(chǎn) - 這一次神秘隱藏在數(shù)據(jù)中!
博主網(wǎng)校主頁http://dwz.date/bwes
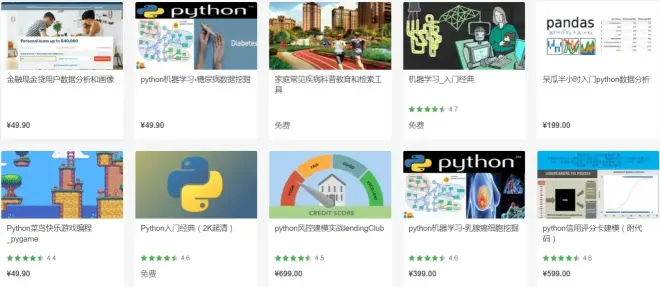