銀行案例學習實例1_風險管理數(shù)據(jù)可視化
?banking case1
up主微信公眾號pythonEducation
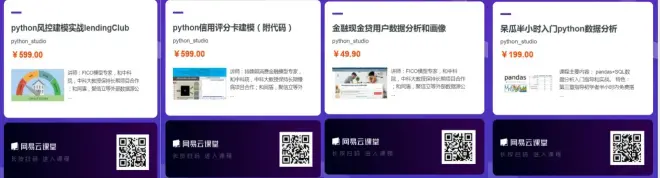
A Scientist & An Artist
幾個星期前,在文藝復興的發(fā)源地佛羅倫薩四處閑逛時,我無法逃脫達芬奇的思想:有史以來最偉大的博學者。 萊昂納多的杰出作品包括畫家,發(fā)明家,物理學家,天文學家,工程師,生物學家,解剖學家,地質學家和建筑師等稱號 - 不開玩笑! 一只聰明的貓將不得不過她九年的生命來獲得萊昂納多一生中掌握的九個冠軍頭銜。 今天,在討論數(shù)據(jù)可視化的各個方面時,我們應該向萊昂納多叔叔致敬,因為我們跨越了藝術和科學的領域。
A few weeks ago while wandering around in Florence, the birthplace of the Renaissance, I could not escape the thought of Leonardo da Vinci : the greatest polymath of all times. Leonardo’s illustrious resume contains titles such as painter, inventor, physicist, astronomer, engineer, biologist, anatomist, geologist, and architect – no kidding! A smart cat would have to live all her nine lives to acquire the nine titles Leonardo had mastered in one lifetime. Today, while discussing facets of data visualization, we should pay homage to Uncle Leonardo as we cross the realm of both art and science.
Art and Science of Data Visualization
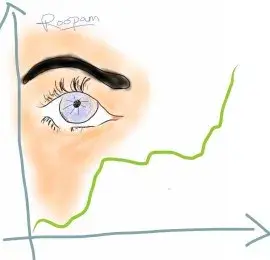
Data Visualization – by Roopam
如前所述,數(shù)據(jù)可視化既是藝術又是科學。 我個人更喜歡長時間查看數(shù)據(jù),在進入嚴格的數(shù)學建模之前以各種方式繪制數(shù)據(jù)。 你可能已經注意到我對藝術的偏愛,同時瀏覽了我在博客上所有帖子中展示的藝術作品。 這句話 - 一張圖片勝過千言萬語 - 在數(shù)據(jù)分析過程中也是如此。?如果您沒有在數(shù)據(jù)探索階段花費足夠的時間,那么分析中的模型可能會出現(xiàn)嚴重錯誤 -?這些都是關于數(shù)據(jù)可視化的。 讓我提出一個案例研究示例來解釋探索階段數(shù)據(jù)可視化的各個方面。
Data visualization, as mentioned earlier, is both art and science. I personally prefer to have a long look at the data, plotting them in various ways before jumping into rigorous mathematical modeling. You might have noticed my penchant for art while going through my artwork presented in all the posts on this blog. The saying – a picture is worth thousand words – holds true during data analysis as well. Models in analytics can go horribly wrong if you have not spent enough time on the data exploratory phase – which is all about data visualization to me. Let me present a case study example to explain the aspects of data visualization during the exploratory phase.
Banking?Case Study Example – Risk Management
假設您是CyndiCat銀行的首席風險官(CRO),該銀行在2012年4月至6月期間在該季度發(fā)放了60816個汽車貸款。今天,自貸款發(fā)放以來大約一年零四個季度,您知道貸款已經過時或者糟糕 貸款被標記為更加確定(閱讀詳細討論)。 你注意到在60816筆已發(fā)放貸款中,不良貸款率約為2.5%或1524。
在您跳轉到多變量分析和信用評分(閱讀有關信用評分的詳細討論)之前,您需要分析幾個單獨變量的不良率。 根據(jù)您的經驗,您有預感,借款人在貸款發(fā)放時的年齡是不良利率的關鍵區(qū)別因素。 因此,您根據(jù)借款人的年齡對貸款進行了劃分,并創(chuàng)建了一個類似下面的表格。
Assume you are the chief risk officer (CRO) for CyndiCat bank that has disbursed支付 60816 auto loans in the quarter between April–June 2012. Today, about a year and a quarter since the loans disbursal, you know that the loans have seasoned or bad loans?are tagged to a greater certainty?(read a detailed discussion). You have noticed a bad rate of around 2.5% or 1524 bad loans out of total 60816 disbursed loans.
Before you jump to multivariate analysis and credit scoring?(read a detailed discussion on credit scoring), you want to analyze the bad rate across several individual variables. You have a hunch based on your experience that borrower’s age at the time of loan disbursal is a key distinguishing factor for bad rates. Therefore, you have divided the loans based on the age of the borrowers and created a table something like the one below.
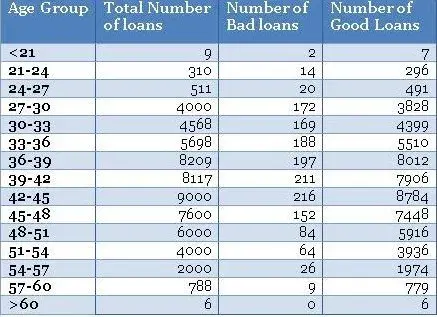
Using the above table, you have created a histogram and zoomed into the area of interest (close to the bad loans) as shown in the plots below.
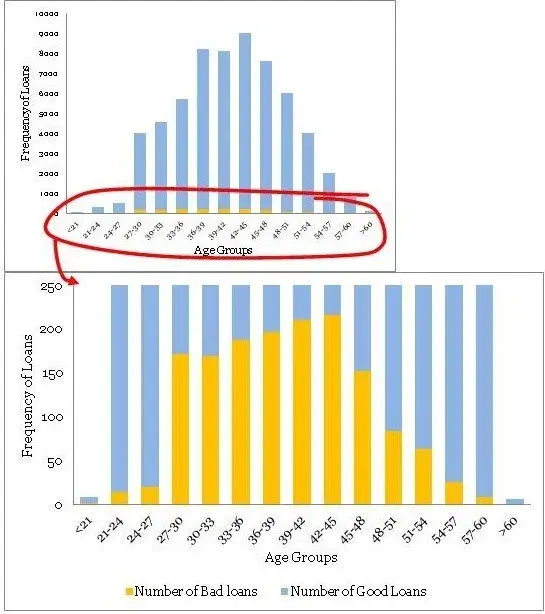
不同年齡組的貸款分配是一個相當平滑的正態(tài)分布曲線,沒有太多的異常值。年齡經常為大多數(shù)產品展示這種模式。但是,不要指望業(yè)務場景中其他常見變量的類似平滑曲線。通常,您可能必須解決變量轉換以使分布平滑。
?最大不良貸款年齡為42至45歲。這當然并不意味著風險也是最高的,但是,一旦我聽到有人在季度業(yè)務審查會議上得出類似的結論 - 這是一個愚蠢的錯誤。注意,最高貸款也在42至45年。絕對數(shù)字不能提供足夠的信息,因此我們需要創(chuàng)建一個標準化的圖。
?條紋桶(即<21和> 60年組)的數(shù)據(jù)非常薄,只有9個和6個數(shù)據(jù)點 - 處理這些薄數(shù)據(jù)時要小心。在模型開發(fā)時,修改這些邊緣桶的良好業(yè)務知識非常有用。例如,您知道對于年齡在60歲以上的貸款可能存在高風險,但在這些數(shù)據(jù)中,我們沒有足夠的證據(jù)證明這一點,因為我們沒有足夠的數(shù)據(jù)來驗證我們的假設。在這種情況下,我們應該補充正確的風險權重 - 但是,這樣做時要非常小心。
You must have noticed the following
? The distribution of loans across age groups is a reasonably smooth normally distributed curve, without too many outliers. Age often display this kind of pattern for most products. However, do not expect similar smooth curves for other commonly found variables in a business scenario. Often, you may have to resolve to variable transformation to make the distributions smooth.
? The maximum bad loans are in the age bucket 42 to 45 years. This certainly does not mean the risk is also the highest in this bucket, however, once I have heard someone drawing a similar conclusion in a quarterly business review meeting –a silly mistake. Note, the maximum loans are also in the bucket 42 to 45 years. Absolute numbers do not provide enough information hence we need to create a normalized plot.
? The data is really thin on the fringe buckets (i.e. <21 and >60 years groups)?with only 9 and 6 data points – be careful when dealing with such thin data. Sound business knowledge to modify these fringe buckets is extremely helpful while a model development. For instance, you know that for age above 60 for loans could be highly risky, but in this data, we do not have enough evidence for the same since we do not have enough data to validate our hypothesis. We should supplement a right risk weight in such situation – however, be very careful while doing so.
Normalized Plot
The normalized plot is easy to construct. The idea is to scale each age group to 100% and overlay bad and good percentage of records on top. We could extend the table shown above to get the values for the normalized plot as shown below.
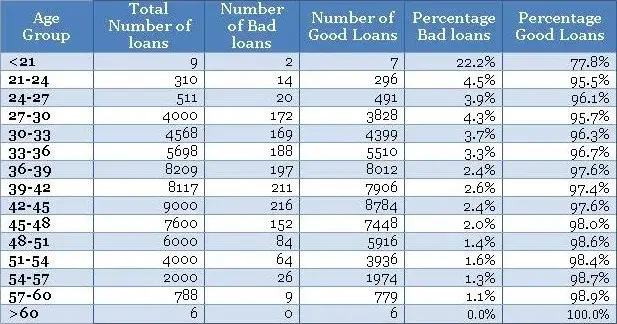
Now, once you have the table ready you could create a normalized plot quite easily, as shown below (again we have zoomed into the plot to get a clear view of bad rates).
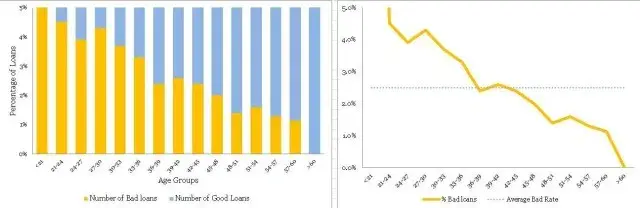
在不良率和年齡組方面存在明顯的趨勢。 隨著借款人變老,他們不太可能拖欠貸款。 這是一個很好的見解。
?同樣,條紋(即<21和> 60年組)具有薄數(shù)據(jù),不能從標準化圖獲得該信息。 因此,您需要使用頻率圖來方便地處理不同的瘦數(shù)據(jù)。 一個方便的經驗法則是在認真對待信息之前至少有10個(好的和壞的)病例的記錄 - 否則,它沒有統(tǒng)計學意義。
These plots are completely different from the original frequency count plot and presenting the information in a completely different light. The following are the things one could conclude from the plots.
? There is a definite trend in terms of the bad rates and the age groups. As the borrowers are getting older, they are less likely to default on their loans. That is a good insight.
? Again, the fringes (i.e. <21 and >60 years groups) have thin data, this information cannot be obtained from the normalized plot. Hence, you need to have the frequency plot handy to treat thin data differently. A handy rule of thumb is to have at least 10 records of both (good & bad) cases before taking the information seriously – otherwise, it is not statistically significant.
I must conclude by saying that, data visualization is the beginning of modeling process and not the destination. However, it is a good & creative beginning.
Sign-off Note
我必須總結說,數(shù)據(jù)可視化是建模過程的開始,而不是目的地。 然而,這是一個良好的創(chuàng)造性開端。
簽字筆記
憑借大數(shù)據(jù),數(shù)據(jù)分析工具和技術,科學進步和民主環(huán)境 - 我們可以生活在我們這個時代的文藝復興時期。 但是,我們需要更多的達芬奇才能讓這些時間變得非常特別。
With big data, data analysis tools & technologies, scientific progress and democratic environment – we could be living in the Renaissance of our times. However, we will need more Leonardo da Vincis to make these times really special.
博主網(wǎng)校主頁http://dwz.date/bwes
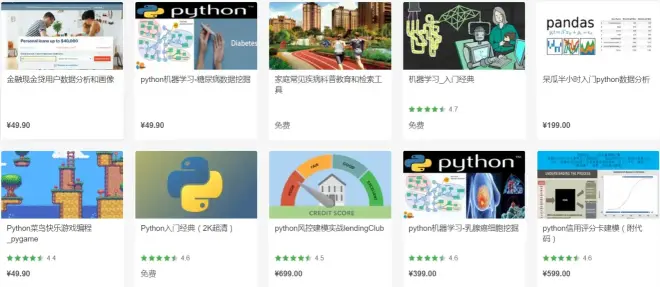