精選了61篇3D點云頂會論文及源碼分享!含2023最新
目前2D圖像識別技術(shù)早已成熟,但2D的圖像信息只有XY兩個維度,而3D點云能夠提供三維世界的信息,因此在自動駕駛、機器人和增強現(xiàn)實等各種領(lǐng)域都得到了廣泛的應(yīng)用。
近年來隨著深度學(xué)習(xí)的發(fā)展,作為計算機視覺新興研究熱點的3D點云技術(shù)也在不斷更新迭代,目前在數(shù)據(jù)獲取、處理、分析和應(yīng)用等方面仍然存在許多挑戰(zhàn)。
學(xué)姐這次整理了近三年各大頂會中3D點云方向的論文,共61篇,有想法發(fā)paper的同學(xué)速看:
掃碼添加小享,回復(fù)“3D點云”??
免費獲取全部論文+源碼合集
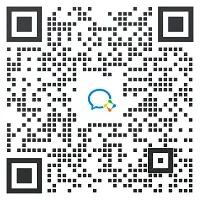
CVPR 2023
Attention-based Point Cloud Edge Sampling
一句話概括:本文提出一種非生成式基于注意力的點云邊緣采樣方法APES,通過提取點云輪廓上的顯著點,在多個基準任務(wù)上表現(xiàn)出較好的性能。
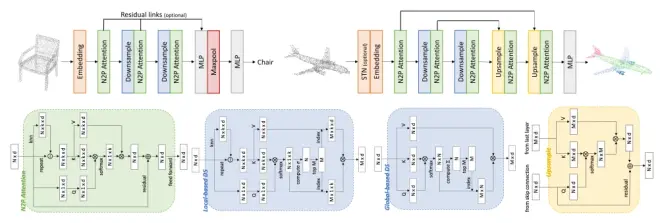
IterativePFN: True Iterative Point Cloud Filtering
一句話概括:本文提出了迭代點云過濾網(wǎng)絡(luò)IterativePFN,它由多個IterationModule組成,在單個網(wǎng)絡(luò)內(nèi)部模擬真實的迭代過濾過程。作者使用新穎的損失函數(shù)訓(xùn)練該網(wǎng)絡(luò),該損失函數(shù)在每次迭代中使用自適應(yīng)的真值目標,以捕捉訓(xùn)練期間中間過濾結(jié)果之間的關(guān)系。
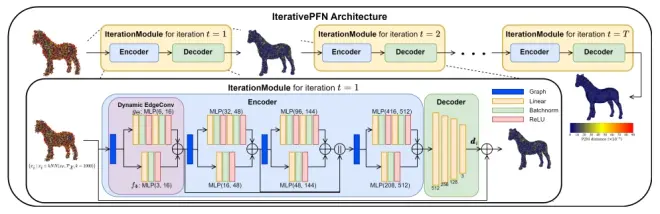
ULIP: Learning a Unified Representation of Language, Images, and Point Clouds for 3D Understanding
一句話概括:本文提出了ULIP,通過使用來自圖像、文本和3D點云三種模態(tài)的對象組對進行預(yù)訓(xùn)練,學(xué)習(xí)統(tǒng)一的多模態(tài)表示。為克服訓(xùn)練三元組不足的問題,ULIP利用預(yù)訓(xùn)練的圖像文本模型來獲得共享的視覺語義空間,然后使用少量自動合成的三元組學(xué)習(xí)與之對齊的3D表示空間。
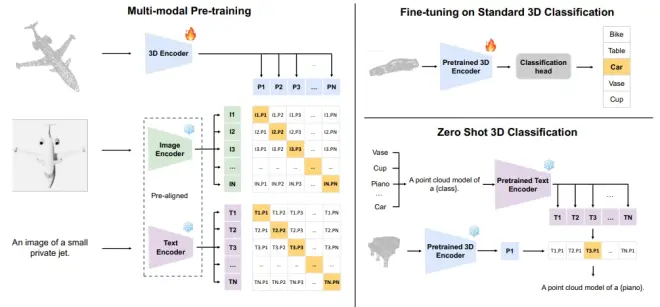
SCPNet: Semantic Scene Completion on Point Cloud
一句話概括:論文提出了三個方法來提高語義場景完成任務(wù)的性能:1)重新設(shè)計完成子網(wǎng)絡(luò),使用多路徑模塊聚合多尺度特征;2)設(shè)計師生知識蒸餾,將多幀模型的稠密語義知識遷移到單幀模型;3)使用泛光分割標簽校正完成標簽,去除動態(tài)物體。
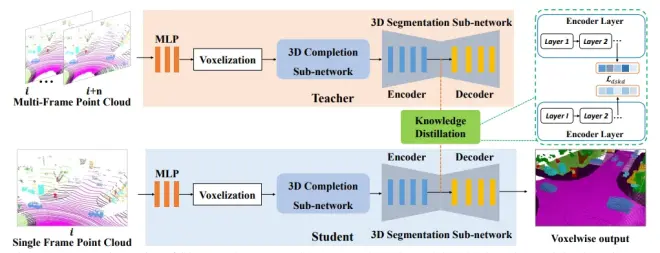
ACL-SPC: Adaptive Closed-Loop system for Self-Supervised Point Cloud Completion
一句話概括:該論文提出了一種自監(jiān)督的點云補全框架ACL-SPC,可以在同域的數(shù)據(jù)上進行訓(xùn)練和測試,克服了現(xiàn)有監(jiān)督方法需要合成數(shù)據(jù)以及域間差異的限制,使用自適應(yīng)閉環(huán)系統(tǒng)強制網(wǎng)絡(luò)輸出對輸入變化穩(wěn)定,從而實現(xiàn)無需先驗信息的點云自監(jiān)督補全。
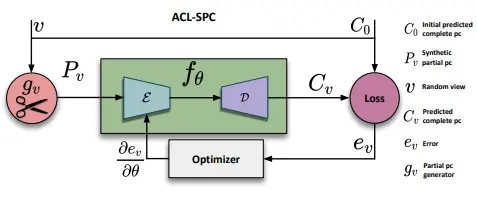
Learning Human-to-Robot Handovers from Point Clouds
一句話概括:論文提出了一個端到端的框架,用于學(xué)習(xí)基于視覺的人機交接控制策略,通過兩階段的教師-學(xué)生訓(xùn)練模式,使用運動規(guī)劃、強化學(xué)習(xí)和自監(jiān)督學(xué)習(xí)方法,實現(xiàn)了從模擬到真實的有效遷移。
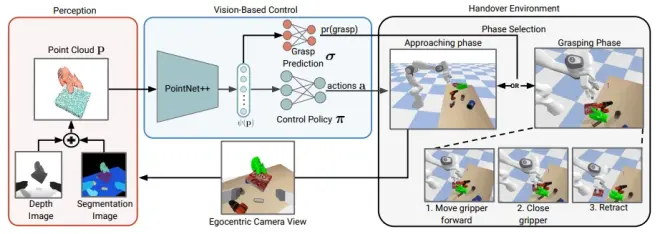
PartManip: Learning Cross-Category Generalizable Part Manipulation Policy from Point Cloud Observations
一句話概括:本文構(gòu)建了第一個大規(guī)模基于部件的跨類別物體操作基準PartManip,包含11個類別494個物體1432個任務(wù),相比現(xiàn)有工作更加復(fù)雜逼真,提出了基于部件規(guī)范化和部件感知獎勵的專家示教方法以及基于對抗學(xué)習(xí)的學(xué)生學(xué)習(xí)方法,實現(xiàn)了基于稀疏點云的通用跨類別物體操作策略學(xué)習(xí),在模擬和真實環(huán)境中都取得了 SOTA的性能。
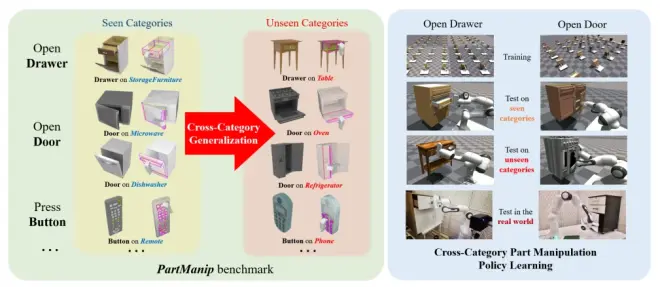
PiMAE: Point Cloud and Image Interactive Masked Autoencoders for 3D Object Detection
一句話概括:本文提出了PiMAE,這是一個跨模態(tài)的自監(jiān)督預(yù)訓(xùn)練框架,通過三個方面提升了點云和圖像這兩種常見模態(tài)之間的交互:遮擋策略交互、共享解碼器和跨模態(tài)重建模塊,相比現(xiàn)有工作取得更好的點云和圖像表示學(xué)習(xí)。
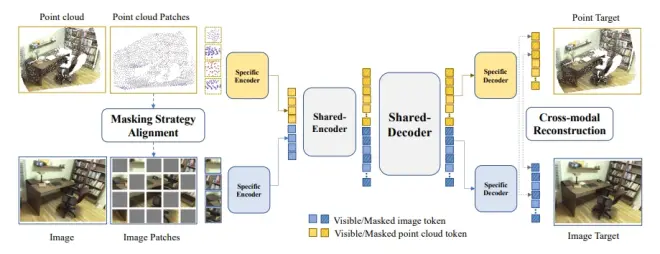
掃碼添加小享,回復(fù)“3D點云”??
免費獲取全部論文+源碼合集
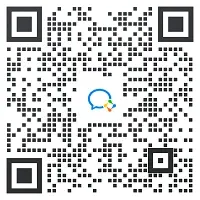
Complete-to-Partial 4D Distillation for Self-Supervised Point Cloud Sequence Representation Learning
一句話概括:本文提出了一種新的4D自監(jiān)督預(yù)訓(xùn)練方法Complete-to-Partial 4D Distillation,關(guān)鍵思想是將4D自監(jiān)督表示學(xué)習(xí)表述為一個teacher-student知識蒸餾框架,讓student在teacher的指導(dǎo)下學(xué)習(xí)有用的4D表示,從而克服了現(xiàn)有方法僅考慮靜態(tài)快照的局限,以及視頻表示學(xué)習(xí)方法局限于圖像空間流而不具3D幾何意識的問題。
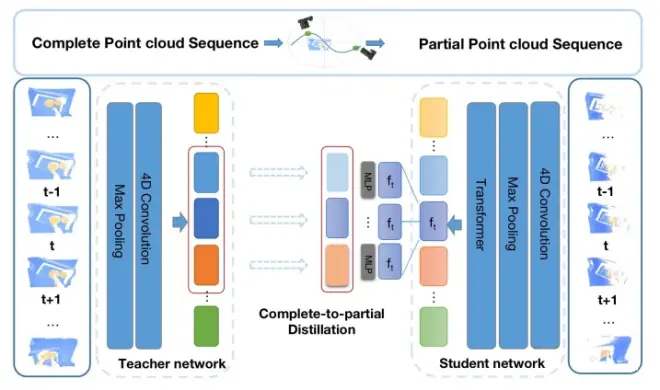
ICCV 2023
Robo3D: Towards Robust and Reliable 3D Perception against Corruptions
一句話概括:本文提出了第一個面向3D檢測和分割模型魯棒性的基準測試集Robo3D,包含來自惡劣天氣、外部干擾和傳感器故障的自然損壞,以探究這些模型在非理想場景下的可靠性。
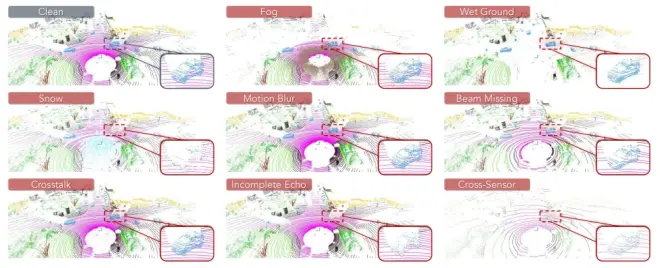
CVPR 2022
Point-BERT: Pre-training 3D Point Cloud Transformers with Masked Point Modeling
A Unified Query-based Paradigm for Point Cloud Understanding
CrossPoint: Self-Supervised Cross-Modal Contrastive Learning for 3D Point Cloud Understanding
PointCLIP: Point Cloud Understanding by CLIP
Fast Point Transformer
RCP: Recurrent Closest Point for Scene Flow Estimation on 3D Point Clouds
The Devil is in the Pose: Ambiguity-free 3D Rotation-invariant Learning via Pose-aware Convolution
ECCV 2022
Open-world Semantic Segmentation for LIDAR Point Clouds
2DPASS: 2D Priors Assisted Semantic Segmentation on LiDAR Point Clouds
CPO: Change Robust Panorama to Point Cloud Localization
diffConv: Analyzing Irregular Point Clouds with an Irregular View
CATRE: Iterative Point Clouds Alignment for Category-level Object Pose Refinement
Dual Adaptive Transformations for Weakly Supervised Point Cloud Segmentation
SeedFormer: Patch Seeds based Point Cloud Completion with Upsample Transformer
Dynamic 3D Scene Analysis by Point Cloud Accumulation
3D Siamese Transformer Network for Single Object Tracking on Point Clouds
Salient Object Detection for Point Clouds
MonteBoxFinder: Detecting and Filtering Primitives to Fit a Noisy Point Cloud
Improving RGB-D Point Cloud Registration by Learning Multi-scale Local Linear Transformation
Learning to Generate Realistic LiDAR Point Clouds
CVPR 2021
Equivariant Point Network for 3D Point Cloud Analysis
PAConv: Position Adaptive Convolution with Dynamic Kernel Assembling on Point Clouds
ICCV 2021
A Robust Loss for Point Cloud Registration
A Technical Survey and Evaluation of Traditional Point Cloud Clustering Methods for LiDAR Panoptic Segmentation
A Spoonful of Refinements Helps the Registration Error Go Down (Oral)
ABD-Net: Attention Based Decomposition Network for 3D Point Cloud Decomposition
AdaFit: Rethinking Learning-based Normal Estimation on Point Clouds
Box-Aware Feature Enhancement for Single Object Tracking on Point Clouds
CPFN: Cascaded Primitive Fitting Networks for High-Resolution Point Clouds
Deep Models with Fusion Strategies for MVP Point Cloud Registration
DRINet: A Dual-Representation Iterative Learning Network for Point Cloud Segmentation
Guided Point Contrastive Learning for Semi-supervised Point Cloud Semantic Segmentation
Learning Inner-Group Relations on Point Clouds
InstanceRefer: Cooperative Holistic Understanding for Visual Grounding on Point Clouds through Instance Multi-level Contextual Referring
ME-PCN: Point Completion Conditioned on Mask Emptiness
MVP Benchmark: Multi-View Partial Point Clouds for Completion and Registration
Out-of-Core Surface Reconstruction via Global TGV Minimization
PCAM: Product of Cross-Attention Matrices for Rigid Registration of Point Clouds
PICCOLO: Point Cloud-Centric Omnidirectional Localization
Point Cloud Augmentation with Weighted Local Transformations
PoinTr: Diverse Point Cloud Completion with Geometry-Aware Transformers (Oral)
ReDAL: Region-based and Diversity-aware Active Learning for Point Cloud Semantic Segmentation
Sampling Network Guided Cross-Entropy Method for Unsupervised Point Cloud Registration
SnowflakeNet: Point Cloud Completion by Snowflake Point Deconvolution with Skip-Transformer
Spatio-temporal Self-Supervised Representation Learning for 3D Point Clouds
Towards Efficient Point Cloud Graph Neural Networks Through Architectural Simplification
Unsupervised Learning of Fine Structure Generation for 3D Point Clouds by 2D Projection Matching
Unsupervised Point Cloud Pre-Training via View-Point Occlusion, Completion
Vis2Mesh: Efficient Mesh Reconstruction from Unstructured Point Clouds of Large Scenes with Learned Virtual View Visibility
Voxel-based Network for Shape Completion by Leveraging Edge Generation
Walk in the Cloud: Learning Curves for Point Clouds Shape Analysis
掃碼添加小享,回復(fù)“3D點云”??
免費獲取全部論文+源碼合集
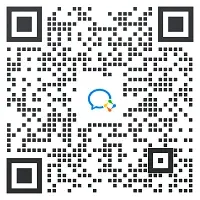