【中英雙語(yǔ)】對(duì)你的企業(yè)而言,AI 不必太金貴
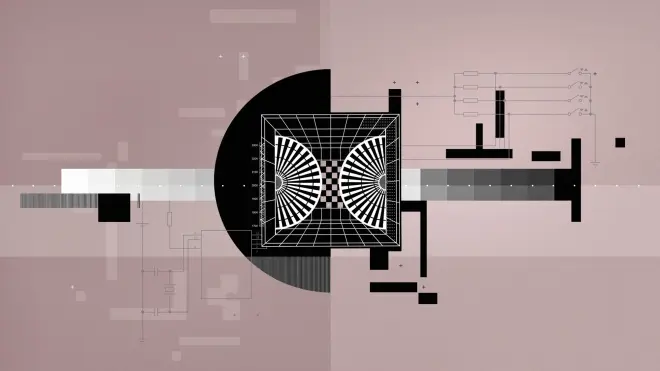
AI Doesn’t Have to Be Too Complicated or Expensive for Your Business
by?Andrew Ng
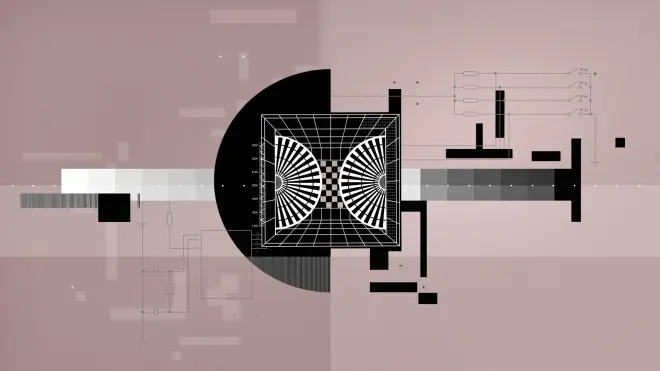
Despite the vast potential of artificial intelligence (AI), it hasn’t caught hold in most industries. Sure, it has transformed consumer internet companies such as Google, Baidu, and Amazon — all massive and? data-rich with hundreds of millions of users. But for projections that AI will create?$13 trillion?of value a year to come true, industries such as manufacturing, agriculture, and healthcare still need to find ways to make this technology work for them. Here’s the problem: The playbook that these consumer internet companies use to build their AI systems — where a single one-size-fits-all AI system can serve massive numbers of users — won’t work for these other industries.
盡管AI的潛力巨大,但它尚未在大多數(shù)行業(yè)立足。當(dāng)然,它已經(jīng)改變了谷歌、百度和亞馬遜等消費(fèi)類(lèi)互聯(lián)網(wǎng)企業(yè)——所有這些公司規(guī)模都很龐大,擁有數(shù)億用戶(hù)的大量數(shù)據(jù)。不過(guò),要實(shí)現(xiàn)AI每年創(chuàng)造13萬(wàn)億美元價(jià)值這一預(yù)測(cè),制造業(yè)、農(nóng)業(yè)和醫(yī)療衛(wèi)生等行業(yè)仍然需要找到辦法讓這項(xiàng)技術(shù)為它們服務(wù)。問(wèn)題在于:這些消費(fèi)類(lèi)互聯(lián)網(wǎng)企業(yè)用來(lái)構(gòu)建其AI系統(tǒng)的行動(dòng)手冊(cè)——一個(gè)一體萬(wàn)用的AI系統(tǒng)可以為大量用戶(hù)服務(wù)——對(duì)其他這些行業(yè)不起作用。
Instead, these legacy industries will need a large number of bespoke solutions that are adapted to their many diverse use cases. This doesn’t mean that AI won’t work for these industries, however. It just means they need to take a different approach.
相反,這些傳統(tǒng)產(chǎn)業(yè)將需要大量定制的解決方案,以適應(yīng)其眾多不同的用例。然而,這并不意味著AI不適于這些行業(yè)。而只意味著他們需要采取不同的方法。
To bridge this gap and unleash AI’s full potential, executives in all industries should adopt a new, data-centric approach to building AI. Specifically, they should aim to build AI systems with careful attention to ensuring that the data clearly conveys what they need the AI to learn. This requires focusing on data that covers important cases and is consistently labeled, so that the AI can learn from this data what it is supposed to do. In other words, the key to creating these valuable AI systems is that we need teams that can program with data rather than program with code.
為了彌合這一差距并釋放AI的全部潛力,所有行業(yè)的高管都應(yīng)該采用新的、以數(shù)據(jù)為中心的方法來(lái)構(gòu)建AI。具體來(lái)說(shuō),他們?cè)谥铝τ跇?gòu)建AI系統(tǒng)目標(biāo)時(shí)應(yīng)該小心注意確保數(shù)據(jù)清楚地傳達(dá)他們需要讓AI學(xué)習(xí)的內(nèi)容。這就需要專(zhuān)注于囊括重要案例、并進(jìn)行了連貫標(biāo)記的數(shù)據(jù),以便AI能夠從這些數(shù)據(jù)中學(xué)習(xí)它應(yīng)該要做的事情。換言之,創(chuàng)建這些有價(jià)值的AI系統(tǒng)的關(guān)鍵是我們需要能夠使用數(shù)據(jù)編程而不是代碼編程的團(tuán)隊(duì)。
Why adopting AI outside of tech can be so hard
為何在科技企業(yè)之外采用AI如此之難
Why isn’t AI widely used outside consumer internet companies? The top challenges facing AI adoption in other industries include:
為何AI沒(méi)有在消費(fèi)類(lèi)互聯(lián)網(wǎng)企業(yè)之外得到廣泛使用?其他行業(yè)采用AI面臨的最大挑戰(zhàn)包括:
1.?Small datasets.?In a consumer internet company with huge numbers of users, engineers have millions of data points that their AI can learn from. But in other industries, the dataset sizes are much smaller. For example, can you build an AI system that learns to detect a defective automotive component after seeing only 50 examples? Or to detect a rare disease after learning from just 100 diagnoses? Techniques built for 50 million data points don’t work when you have only 50 data points.
? ?數(shù)據(jù)集規(guī)模小。在一家擁有大量用戶(hù)的消費(fèi)類(lèi)互聯(lián)網(wǎng)公司中,工程師擁有數(shù)百萬(wàn)個(gè)數(shù)據(jù)點(diǎn),他們的AI可以從中學(xué)習(xí)。但在其他行業(yè),數(shù)據(jù)集的規(guī)模要小得多。比如,你是否能夠建立一個(gè)AI系統(tǒng),在只看了50個(gè)例子之后就學(xué)會(huì)檢測(cè)有缺陷的汽車(chē)部件?或者僅僅學(xué)習(xí)了100例病例診斷之后就能發(fā)現(xiàn)一種罕見(jiàn)疾病?當(dāng)你只有50個(gè)數(shù)據(jù)點(diǎn)時(shí),構(gòu)建5000萬(wàn)個(gè)數(shù)據(jù)點(diǎn)的技術(shù)不起作用。?
2.?Cost of customization.?Consumer internet companies employ dozens or hundreds of skilled engineers to build and maintain monolithic AI systems that create tremendous value — say, an online ad system that generates more than $1 billion in revenue per year. But in other industries, there are numerous $1-5 million projects, each of which needs a custom AI system. For example, each factory manufacturing a different type of product might require a custom inspection system, and every hospital, with its own way of coding health records, might need its own AI to process its patient data. The aggregate value of these hundreds of thousands of these projects is massive; but the economics of an individual project might not support hiring a large, dedicated AI team to build and maintain it. This problem is exacerbated by the ongoing shortage of AI talent, which further drives up these costs.
? ?定制成本。消費(fèi)類(lèi)互聯(lián)網(wǎng)公司會(huì)雇用數(shù)十或數(shù)百名技術(shù)熟練的工程師來(lái)構(gòu)建和維護(hù)能夠創(chuàng)造巨大價(jià)值的龐大AI系統(tǒng)——比如,每年產(chǎn)生超過(guò)10億美元收入的在線(xiàn)廣告系統(tǒng)。但在其他行業(yè),有許多100萬(wàn)-500萬(wàn)美元的項(xiàng)目,每個(gè)項(xiàng)目都需要一個(gè)定制的AI系統(tǒng)。比如,每一家制造不同類(lèi)型產(chǎn)品的工廠可能需要定制的檢查系統(tǒng),每一家醫(yī)院因有自己的病歷編碼方式可能需要自己的AI來(lái)處理患者數(shù)據(jù)。這些數(shù)以十萬(wàn)計(jì)的項(xiàng)目總價(jià)值十分巨大;但是單個(gè)項(xiàng)目的經(jīng)濟(jì)規(guī)模可能無(wú)法支持聘用一個(gè)大型、專(zhuān)門(mén)的AI團(tuán)隊(duì)來(lái)構(gòu)建和維護(hù)它。這一問(wèn)題因AI人才的持續(xù)短缺而加劇,從而進(jìn)一步推高了這些成本。
3.?Gap between proof of concept and production.?Even when an AI system works in the lab, a massive amount of engineering is needed to deploy it in production. It is not unusual for teams to celebrate a successful proof of concept, only to realize that they still have another 12-24 months of work before the system can be deployed and maintained.
? ? 概念驗(yàn)證和用于生產(chǎn)之間的時(shí)間差。即使AI系統(tǒng)在實(shí)驗(yàn)室奏效,要在生產(chǎn)中部署它也還需要大量的工程。團(tuán)隊(duì)?wèi)c祝概念驗(yàn)證成功,卻發(fā)現(xiàn)在系統(tǒng)部署和維護(hù)之前,他們還有12-24個(gè)月的工作,這是很正常的。
For AI to realize its full potential, we need a systematic approach to solving these problems across all industries. The data-centric approach to AI, supported by tools designed for building, deploying, and maintaining AI applications — called machine learning operations (MLOps) platforms — will make this possible. Companies that adopt this approach faster will have a leg up relative to competitors.
為了讓AI充分發(fā)揮潛力,我們需要一種系統(tǒng)性的方法來(lái)解決各行各業(yè)的這些問(wèn)題。這種以數(shù)據(jù)為中心應(yīng)對(duì)AI的方法在旨在用來(lái)構(gòu)建、部署和維護(hù)AI應(yīng)用程序的工具——機(jī)器學(xué)習(xí)操作(MLOps)平臺(tái)的支持下,將有可能讓這變成現(xiàn)實(shí)。更快采用這一方法的企業(yè)將獲得比競(jìng)爭(zhēng)對(duì)手更大的優(yōu)勢(shì)。
Data-centric AI development?
以數(shù)據(jù)為中心的AI開(kāi)發(fā)
AI systems are made up of software — the computer program that includes an AI model — and data, the information used to train the model. For example, to build an AI system for automated inspection in manufacturing, an AI engineer might create software that implements a deep learning algorithm, that is then shown a dataset comprising pictures of good and defective parts, so it can learn to distinguish between them.
AI系統(tǒng)由軟件——包括某種AI模型的計(jì)算機(jī)程序——和數(shù)據(jù)(用于培育模型的信息)組成。比如,為了構(gòu)建一個(gè)用于制造業(yè)自動(dòng)化檢查的AI系統(tǒng),AI工程師可能會(huì)創(chuàng)建可以執(zhí)行深度學(xué)習(xí)算法的軟件,然后向其顯示一個(gè)包含優(yōu)質(zhì)零件和有缺陷零件圖片的數(shù)據(jù)集,這樣它可以學(xué)會(huì)區(qū)分這些零件。
Over the last decade, a lot of AI research was driven by software-centric development (also called model-centric development) in which the data is fixed, and teams attempt to optimize or invent new programs to learn well from the available data. Many tech companies had large datasets from millions of consumers, and they used it to drive a lot of innovation in AI.
在過(guò)去的十年中,許多AI研究都是由以軟件為中心的開(kāi)發(fā)(也稱(chēng)為以模型為中心的開(kāi)發(fā))所推動(dòng)的,數(shù)據(jù)在這種開(kāi)發(fā)中是固定的。團(tuán)隊(duì)試圖優(yōu)化或發(fā)明新的程序,以便好好地從現(xiàn)有數(shù)據(jù)中學(xué)習(xí)。許多科技企業(yè)擁有源自數(shù)百萬(wàn)消費(fèi)者的大型數(shù)據(jù)集,他們利用這些數(shù)據(jù)來(lái)推動(dòng)AI的大量創(chuàng)新。
But at AI’s current level of sophistication, the bottleneck for many applications is getting the right data to feed to the software. We’ve heard about the benefits of?big data, but we now know that for many applications, it is more fruitful to focus on making sure we have?good data?— data that clearly illustrates the concepts we need the AI to learn. This means, for example, the data should be reasonably comprehensive in its coverage of important cases and labeled consistently. Data is food for AI, and modern AI systems need not only calories, but also high-quality nutrition.
然而,在AI目前的發(fā)展水平上,許多應(yīng)用程序的瓶頸在于獲得正確的數(shù)據(jù)提供給軟件。我們已經(jīng)聽(tīng)說(shuō)了大數(shù)據(jù)的好處,但我們現(xiàn)在知道,對(duì)于許多應(yīng)用程序而言,專(zhuān)注于確保我們擁有優(yōu)質(zhì)的數(shù)據(jù)會(huì)更富有成效——這些數(shù)據(jù)清楚地說(shuō)明了我們需要讓AI學(xué)習(xí)的概念。這意味著數(shù)據(jù)在對(duì)重要案例的覆蓋面上應(yīng)該適當(dāng)全面并進(jìn)行連貫標(biāo)記。數(shù)據(jù)是AI的食糧,現(xiàn)代AI系統(tǒng)不僅需要卡路里,還需要高質(zhì)量的營(yíng)養(yǎng)。
Shifting your focus from software to data offers an important advantage: it relies on the people you already have on staff. In a time of great AI talent shortage, a data-centric approach allows many subject matter experts who have vast knowledge of their respective industries to contribute to the AI system development.
將重點(diǎn)從軟件轉(zhuǎn)變到數(shù)據(jù)提供了一個(gè)重大好處:它依賴(lài)的是你的現(xiàn)有員工。在AI人才嚴(yán)重短缺的時(shí)代,以數(shù)據(jù)為中心的方法允許許多在各自行業(yè)擁有淵博知識(shí)的主題專(zhuān)家為AI系統(tǒng)的開(kāi)發(fā)盡力。
For example, most factories have workers that are highly skilled at defining and identifying what counts as a defect (is a 0.2mm scratch a defect? or is it so small that it doesn’t matter?). If we expect each factory to ask its workers to invent new AI software as a way to get that factory the bespoke solution it needs, progress will be slow. But we instead build and provide tools to empower these domain experts to engineer the data — by allowing them to express their knowledge about manufacturing through providing data to the AI — their odds of success will be much higher.
比如,多數(shù)工廠的工人都非常擅長(zhǎng)定義和識(shí)別什么才算缺陷(0.2mm的劃痕是否是缺陷?還是說(shuō)它小得無(wú)關(guān)緊要?)。如果我們期望每家工廠要求其工人發(fā)明新的AI軟件,以此讓工廠獲得其所需的定制解決方案,那么進(jìn)展會(huì)十分遲緩。但是,如果我們轉(zhuǎn)而構(gòu)建并提供工具,使這些領(lǐng)域?qū)<夷軌蛟O(shè)計(jì)數(shù)據(jù)——通過(guò)向AI提供數(shù)據(jù)、使他們能夠表達(dá)自己在制造業(yè)方面的知識(shí)——他們成功的幾率會(huì)高得多。
Make building and using AI systematic and repeatable?
讓構(gòu)建和使用AI變得系統(tǒng)化且可重復(fù)
The shift toward data-centric AI development is being enabled by the emerging field of MLOps, which provides tools that make building, deploying, and maintaining AI systems easier than ever before. Tools that are geared to help produce high-quality datasets, in particular, hold the key to addressing the challenges of small datasets, high cost of customization, and the long road to getting an AI project into production outlined above.
向以數(shù)據(jù)為中心的AI開(kāi)發(fā)的轉(zhuǎn)變是由新興的MLOps領(lǐng)域?qū)崿F(xiàn)的,該領(lǐng)域提供了比以往任何時(shí)候都更容易構(gòu)建、部署和維護(hù)AI系統(tǒng)的工具。要特別指出的是,用來(lái)幫助生成高質(zhì)量數(shù)據(jù)集的工具對(duì)于解決數(shù)據(jù)集小、定制成本高以及AI項(xiàng)目應(yīng)用于生產(chǎn)的時(shí)間長(zhǎng)等挑戰(zhàn)至關(guān)重要。
How, exactly? First, ensuring high-quality data means that AI systems will be able to learn from the smaller datasets available in most industries. Second, by making it possible for a business’ domain experts, rather than AI experts, to engineer the data, the ability to use AI will become more accessible to all industries. And third, MLOps platforms provide much of the scaffolding software needed to take an AI system to production, so teams no longer have to develop this software. This allows teams to deploy AI systems — and bridge the gap between proof of concept and production weeks or months rather than years.
到底怎樣才能做到?首先,確保數(shù)據(jù)高質(zhì)量意味著AI系統(tǒng)能夠從多數(shù)行業(yè)可用的較小數(shù)據(jù)集中學(xué)習(xí)。其次,通過(guò)讓企業(yè)的領(lǐng)域?qū)<叶茿I專(zhuān)家能夠設(shè)計(jì)數(shù)據(jù),所有行業(yè)都可以更容易地使用AI。第三,MLOps平臺(tái)提供了將AI系統(tǒng)應(yīng)用于生產(chǎn)所需的許多腳手架軟件,因此團(tuán)隊(duì)不必再開(kāi)發(fā)這種軟件。這使得團(tuán)隊(duì)能夠部署AI系統(tǒng)——并將概念驗(yàn)證與用于生產(chǎn)之間的時(shí)間差縮短至數(shù)周或數(shù)月,而不是幾年。
The vast majority of valuable AI projects have yet to be imagined. And even for projects that teams are already working on, the gap that leads to deployment in production remains to be bridged — indeed, Accenture estimates that?80% to 85% of companies’?AI projects are in the proof-of-concept stage.
絕大多數(shù)有價(jià)值的AI項(xiàng)目尚待構(gòu)想。即使是各團(tuán)隊(duì)已經(jīng)在進(jìn)行的項(xiàng)目,逐漸在生產(chǎn)中部署的時(shí)間差仍有待縮短——事實(shí)上,埃森哲估計(jì),80%到85%的企業(yè)的AI項(xiàng)目處于概念驗(yàn)證階段。
Here’re some things companies can do right now:
以下是企業(yè)現(xiàn)在可以做的一些事情:
1. Instead of merely focusing on the?quantity?of data you collect, also consider the?quality,? make sure it? clearly illustrates the concepts we need the AI to learn.
? ?不要只關(guān)注所收集數(shù)據(jù)的數(shù)量,也要關(guān)注質(zhì)量,確保它清楚地說(shuō)明了我們需要讓AI學(xué)習(xí)的概念。
2. Make sure your team considers taking a data-centric approach rather than a software-centric approach. Many AI engineers, including many with strong academic or research backgrounds, were trained to take a software-centric approach; urge them to adopt data-centric techniques as well.
? ? 確保團(tuán)隊(duì)考慮采用以數(shù)據(jù)為中心的方法,而不是以軟件為中心的方法。許多AI工程師,包括許多具有強(qiáng)大學(xué)術(shù)或研究背景的工程師,接受過(guò)的是以軟件為中心的方法培訓(xùn);要敦促他們也采用以數(shù)據(jù)為中心的技術(shù)。
3.?For any AI project that you intend to take to production, be sure to plan the deployment process and provide MLOps tools to support it. For example, even while building a proof of concept system, urge the teams to begin developing a longer-term plan for data management, deployment, and AI system monitoring and maintenance.
? ? 對(duì)于打算應(yīng)用于生產(chǎn)的任何AI項(xiàng)目,請(qǐng)確保對(duì)部署過(guò)程進(jìn)行規(guī)劃并提供MLOps工具予以支持。比如,即使在構(gòu)建概念驗(yàn)證系統(tǒng)時(shí),也要敦促團(tuán)隊(duì)開(kāi)始制定長(zhǎng)期計(jì)劃進(jìn)行數(shù)據(jù)管理、部署以及AI系統(tǒng)的監(jiān)控和維護(hù)。
It’s possible for AI to become a thriving asset outside of data-rich consumer internet businesses, but has yet to hit its stride in other industries. But because of this, the greatest untapped opportunity for AI may lie in taking it to these other industries. Just as electricity has transformed every industry, AI is on a path to do so too. But the next few steps on that path will require a shift in our playbook for how we build and deploy AI systems. Specifically, a new data-centric mindset, coupled with MLOps tools that allow industry domain experts to participate in the creation, deployment and maintenance of AI systems, will ensure that all industries can reap the rewards that AI can offer.
AI有可能成為數(shù)據(jù)豐富的消費(fèi)類(lèi)互聯(lián)網(wǎng)企業(yè)之外的一項(xiàng)蓬勃發(fā)展的資產(chǎn),但尚未在其他行業(yè)取得進(jìn)展。但正因?yàn)槿绱耍珹I尚未開(kāi)發(fā)的最大機(jī)會(huì)可能在于將其帶到其他行業(yè)。就像電力改變了每個(gè)行業(yè)一樣,AI也走在同樣的道路上。但這條道路上的下幾個(gè)步驟將要求我們?cè)谛袆?dòng)手冊(cè)中改變構(gòu)建和部署AI系統(tǒng)的方式。具體而言,新的以數(shù)據(jù)為中心的思維方式,加上允許行業(yè)領(lǐng)域?qū)<覅⑴cAI系統(tǒng)創(chuàng)建、部署和維護(hù)的MLOps工具,將確保所有行業(yè)都能收獲AI所能提供的回報(bào)。
吳恩達(dá)是Landing AI的創(chuàng)始人兼CEO、百度前副總裁兼首席科學(xué)家、CurSera的聯(lián)合董事長(zhǎng)兼共同創(chuàng)始人、Google Brain的前創(chuàng)始負(fù)責(zé)人,以及斯坦福大學(xué)的兼職教授。
劉雋 | 編輯