【莫煩Python】強化學習 Reinforcement Learning
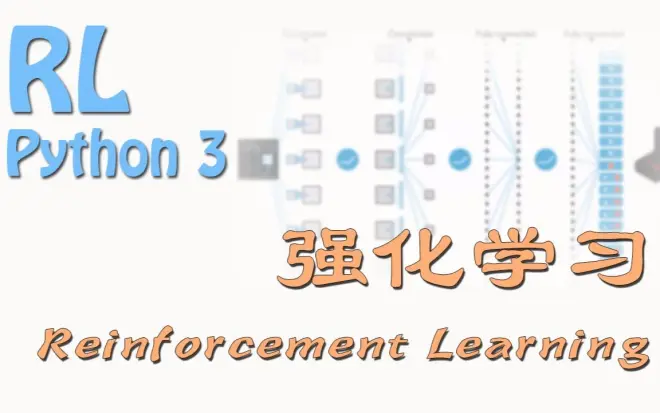
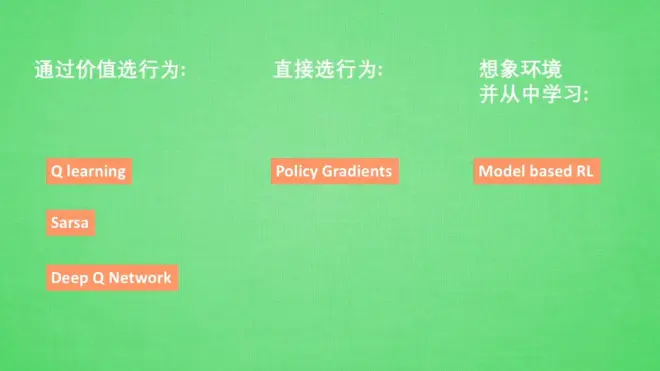
幾種算法
不斷嘗試達到目標
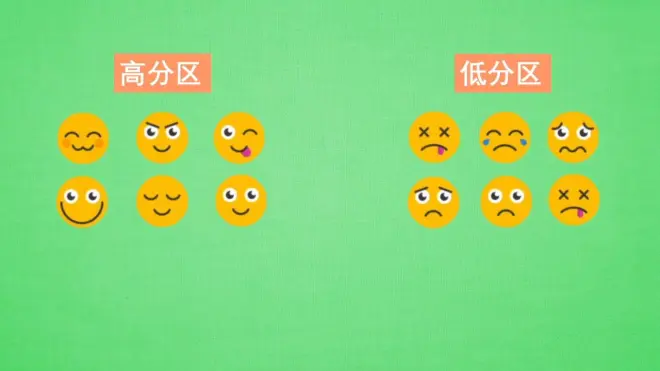
import numpy as np
import pandas as pd
class qlearning_table:
def__init__(self,actions,learning_rate=0.01,reward_decay=0.9,e_greedy=0.9)
self.actions=actions
self.lr=learning_rate
self.gamma=reward_decay
self.epsilon=e_greedy
self.q_table=pd.DataFrame(columns=self.actions)
def choose_action(self,observation):
self.check_state_exist(observation)
#choose action selection
np.random.uniform()<self.epsilon:
#choose best action
state_action=self.q_table.ix[observation,:]
state_action=state_action.reindex(np.random.permutation(state_action.index))
action=state_action.argmax()
else:
action=np.random.choice(self.action)
return action
def learn(self,s,a,r,s_):
self.check_state_exist(s_)
q_predict=self.q_table.ix[s,a]
if s_ !='terminal':
q_target=r+self.gamma*self.q_table
else:
q_target=r#next state is terminal
self.q_table.ix[s,a]+=self.lr*(q_target-q_predict)#update
def check_state_exist(self,state):
if state not in self.q_table.append(
pd.Series(
[0]*len(self.action),
index=self.q_table.columns,
name=state,))
def check_state_exist(self,state):