七夕節(jié)之婚姻問(wèn)題升級(jí)版(feat.Wikipedia)*算了標(biāo)題也爭(zhēng)取下達(dá)到40字終于達(dá)到了nice!
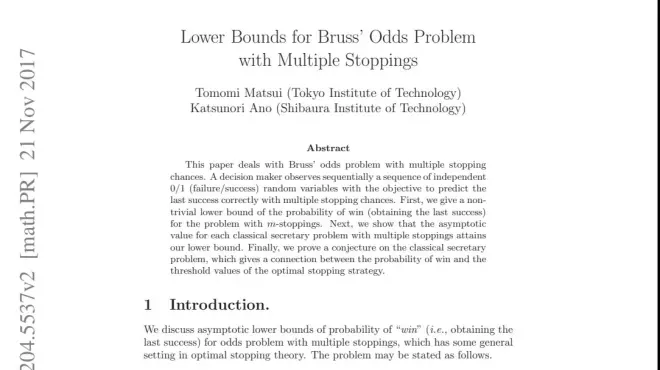
*這是編者在本文編輯的最后37個(gè)字,這是為了恰好達(dá)到30000個(gè)字的限制。
之前用秘書(shū)問(wèn)題來(lái)指導(dǎo)找不到對(duì)象的各位找到好的另一半(使用37%法則),但還是不夠,于是我花錢(qián)上Wikipedia找了升級(jí)版的秘書(shū)問(wèn)題,相信各位能找到相愛(ài)的法則,即不是找到對(duì)象就是向往不了自由,談不了戀愛(ài),*不了狗,找不著對(duì)象。下次看到各位找到對(duì)象是在下次……這樣的事情下次也不一定發(fā)生。[熱詞系列_對(duì)象][熱詞系列_不孤鳥(niǎo)][熱詞系列_對(duì)象]?


好? ? ? ? ? ,
我? ? ?們? ? ?開(kāi)? ?? 始? ???。 ( 以 下 為 https://en.wikipedia.org/wiki/Secretary_problem 內(nèi) 容?)

Secretary problem
秘書(shū)問(wèn)題
From Wikipedia, the free encyclopedia 來(lái)自維基百科,免費(fèi)的百科全書(shū)
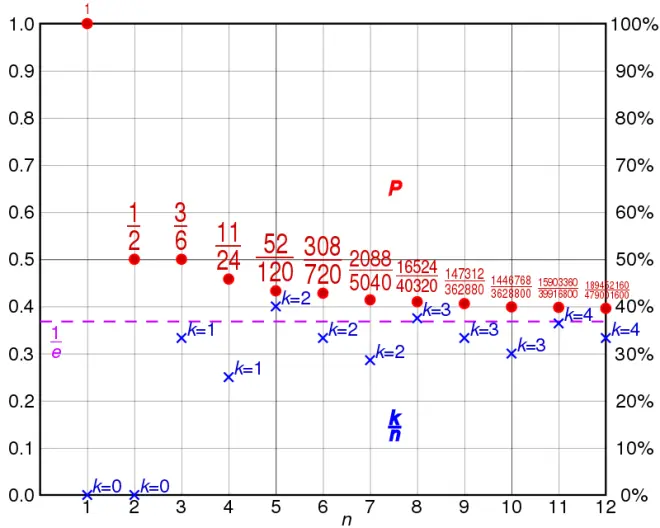
The secretary problem demonstrates a scenario involving optimal stopping theory[1][2] that is studied extensively in the fields of Applied probability, statistics, and decision theory. It is also known as the marriage problem, the sultan's dowry problem, the fussy suitor problem, the googol game, and the best choice problem.
秘書(shū)問(wèn)題證明了一個(gè)包含最優(yōu)停止理論[1][2]的場(chǎng)景,這個(gè)場(chǎng)景在應(yīng)用概率論、統(tǒng)計(jì)學(xué)和決策理論中得到了廣泛的研究。它也被稱(chēng)為婚姻問(wèn)題,蘇丹的嫁妝問(wèn)題,挑剔的追求者問(wèn)題,古格爾游戲,和最佳選擇問(wèn)題。
The basic form of the problem is the following: imagine an administrator who wants to hire the best secretary out of n{\displaystyle n}?rankable Applicants for a position. The Applicants are interviewed one by one in random order. A decision about each particular Applicant is to be made immediately after the interview. Once rejected, an Applicant cannot be recalled. During the interview, the administrator gains information sufficient to rank the Applicant among all Applicants interviewed so far, but is unaware of the quality of yet unseen Applicants. The question is about the optimal strategy (stopping rule) to maximize the probability of selecting the best Applicant. If the decision can be deferred to the end, this can be solved by the simple maximum selection algorithm of tracking the running maximum (and who achieved it), and selecting the overall maximum at the end. The difficulty is that the decision must be made immediately.
這個(gè)問(wèn)題的基本形式如下: 想象一下,一個(gè)管理人員想要從一個(gè)職位的 n 個(gè)可以確定的申請(qǐng)者中雇傭最好的秘書(shū)。申請(qǐng)人是按隨機(jī)順序逐一面試的。關(guān)于每個(gè)申請(qǐng)人的決定將在面試后立即作出。一旦被拒絕,申請(qǐng)人就不能被召回。在面試過(guò)程中,管理人員獲得的信息足以將申請(qǐng)人列入到目前為止面試過(guò)的所有申請(qǐng)人之中,但不知道尚未見(jiàn)過(guò)的申請(qǐng)人的質(zhì)量。問(wèn)題是關(guān)于最優(yōu)策略(停止規(guī)則) ,以最大限度地提高選擇最佳申請(qǐng)人的概率。如果決策可以推遲到最后,這可以通過(guò)簡(jiǎn)單的最大值選擇算法來(lái)解決,即跟蹤運(yùn)行的最大值(以及誰(shuí)達(dá)到了最大值) ,并在最后選擇總體最大值。困難在于必須立即作出決定。
?The shortest rigorous proof known so far is provided by the odds algorithm. It implies that the optimal win probability is always at least 1/e{\displaystyle 1/e}?(where e is the base of the natural logarithm), and that the latter holds even in a much greater generality. The optimal stopping rule prescribes always rejecting the first ~n/e{\displaystyle \sim n/e}?Applicants that are interviewed and then stopping at the first Applicant who is better than every Applicant interviewed so far (or continuing to the last Applicant if this never occurs). ?Sometimes this strategy is called the 1/e{\displaystyle 1/e}?stopping rule, because the probability of stopping at the best Applicant with this strategy is about 1/e{\displaystyle 1/e}?already for moderate values of n{\displaystyle n}?. One reason why the secretary problem has received so much attention is that the optimal policy for the problem (the stopping rule) is simple and selects the single best candidate about 37% of the time, irrespective of whether there are 100 or 100 million Applicants.
迄今為止已知的最短的嚴(yán)格證明是由賠率算法提供的。這意味著最佳的勝算概率至少是1/e (e 是自然對(duì)數(shù)的基礎(chǔ)) ,并且后者具有更大的普遍性。最佳停止規(guī)則規(guī)定總是拒絕第一個(gè)接受面試的申請(qǐng)人,然后在第一個(gè)比目前所有接受面試的申請(qǐng)人都要好的申請(qǐng)人面前停止(如果從未發(fā)生這種情況,則繼續(xù)拒絕最后一個(gè)申請(qǐng)人)。有時(shí)這個(gè)策略被稱(chēng)為1/e 停止規(guī)則,因?yàn)閷?duì)于 n 的中等值,這個(gè)策略在最佳應(yīng)用程序上停止的概率已經(jīng)是1/e 了。秘書(shū)問(wèn)題之所以受到如此多的關(guān)注,其中一個(gè)原因是,解決這個(gè)問(wèn)題的最佳策略(停止規(guī)則)很簡(jiǎn)單,在37% 的時(shí)間里選擇最佳候選人,而不管申請(qǐng)者是1百還是1億。

Formulation 配方
Although there are many variations, the basic problem can be stated as follows:
雖然有許多不同之處,但基本問(wèn)題可以說(shuō)明如下:
There is a single position to fill. 只有一個(gè)職位需要填補(bǔ)
There are ?有n Applicants for the position, and the value of ?申請(qǐng)這個(gè)職位的人,以及n is known. 眾所周知
The Applicants, if seen altogether, can be ranked from best to worst unambiguously. 申請(qǐng)人,如果看到一起,可以排名從最好到最差毫不含糊
The Applicants are interviewed sequentially in random order, with each order being equally likely. 申請(qǐng)人按照隨機(jī)順序接受面試,每次面試的可能性都是相同的
Immediately after an interview, the interviewed Applicant is either accepted or rejected, and the decision is irrevocable. 面試結(jié)束后,面試申請(qǐng)人要么被接受,要么被拒絕,這個(gè)決定是不可撤銷(xiāo)的
The decision to accept or reject an Applicant can be based only on the relative ranks of the Applicants interviewed so far. 接受或拒絕申請(qǐng)人的決定只能基于迄今為止所面試的申請(qǐng)人的相對(duì)級(jí)別
The objective of the general solution is to have the highest probability of selecting the best Applicant of the whole group. ?This is the same as maximizing the expected payoff, with payoff defined to be one for the best Applicant and zero otherwise. 一般解決方案的目標(biāo)是在整個(gè)群體中選擇最佳申請(qǐng)人的概率最高。這和最大化預(yù)期收益是一樣的,最佳申請(qǐng)人的預(yù)期收益定義為1,否則為0
A candidate is defined as an Applicant who, when interviewed, is better than all the Applicants interviewed previously. Skip is used to mean "reject immediately after the interview". Since the objective in the problem is to select the single best Applicant, only candidates will be considered for acceptance. The "candidate" in this context corresponds to the concept of record in permutation.
一個(gè)候選人被定義為一個(gè)申請(qǐng)人誰(shuí),當(dāng)面試,是比所有的申請(qǐng)人以前面試。Skip 的意思是“面試后立即拒絕”。由于問(wèn)題的目標(biāo)是選擇單一的最佳申請(qǐng)人,只有候選人將被考慮接受。這個(gè)上下文中的“候選者”對(duì)應(yīng)于排列中的記錄的概念。
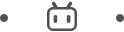
Deriving the optimal policy 推導(dǎo)最優(yōu)策略
The optimal policy for the problem is a stopping rule. Under it, the interviewer rejects the first r???1 Applicants (let Applicant M be the best Applicant among these r???1 Applicants), and then selects the first subsequent Applicant that is better than Applicant M. It can be shown that the optimal strategy lies in this class of strategies.[citation needed] (Note that we should never choose an Applicant who is not the best we have seen so far, since they cannot be the best overall Applicant.) For an arbitrary cutoff r, the probability that the best Applicant is selected is
該問(wèn)題的最優(yōu)策略是一個(gè)停止規(guī)則。在這種情況下,面試官拒絕第一個(gè) r-1申請(qǐng)人(讓申請(qǐng)人 m 是這些 r-1申請(qǐng)人中最好的申請(qǐng)人) ,然后選擇第一個(gè)后續(xù)的申請(qǐng)人優(yōu)于申請(qǐng)人 m。結(jié)果表明,最優(yōu)策略存在于這類(lèi)策略中。[需要引證](注意,我們永遠(yuǎn)不應(yīng)該選擇一個(gè)不是我們迄今為止看到的最好的申請(qǐng)人,因?yàn)樗麄儾豢赡苁亲詈玫恼w申請(qǐng)人。)對(duì)于任意的截止值 r,選擇最佳應(yīng)用程序的概率為
The sum is not defined for r = 1, but in this case the only feasible policy is to select the first Applicant, and hence P(1) = 1/n. This sum is obtained by noting that if Applicant i is the best Applicant, then it is selected if and only if the best Applicant among the first i???1 Applicants is among the first r???1 Applicants that were rejected. Letting n tend to infinity, writing?x {\displaystyle x}?as the limit of (r-1)/n, using t for (i-1)/n and dt for 1/n, the sum can be Approximated by the integral
對(duì)于 r = 1沒(méi)有定義總和,但是在這種情況下唯一可行的策略是選擇第一個(gè)申請(qǐng)者,因此 p (1) = 1/n。如果申請(qǐng)人 i 是最佳申請(qǐng)人,那么只有當(dāng)且僅當(dāng)?shù)谝慌?i-1申請(qǐng)人中的最佳申請(qǐng)人是第一批被拒絕的 r-1申請(qǐng)人之一時(shí),才會(huì)選定這一總額。設(shè) n 趨于無(wú)窮大,寫(xiě) x 為(r-1)/n 的極限,用 t 表示(i-1)/n,dt 表示1/n,和可以用積分近似
Taking the derivative of P(x) with respect to x{\displaystyle x}?, setting it to 0, and solving for x, we find that the optimal x is equal to 1/e. ?Thus, the optimal cutoff tends to n/e as n increases, and the best Applicant is selected with probability 1/e.
將 p (x)對(duì) x 的導(dǎo)數(shù)設(shè)為0,求解 x,我們發(fā)現(xiàn)最優(yōu) x 等于1/e,因此,當(dāng) n 增大時(shí),最優(yōu)截止值趨于 n/e,最優(yōu)選擇的概率為1/e。
For small values of n, the optimal r can also be obtained by standard dynamic programming methods. The optimal thresholds r and probability of selecting the best alternative P for several values of n are shown in the following table.
對(duì)于 n 的小值,也可以用標(biāo)準(zhǔn)的動(dòng)態(tài)規(guī)劃方法得到最優(yōu) r。對(duì)于 n 的幾個(gè)值,最佳閾值 r 和選擇最佳替代 p 的概率如下表所示。
n 1? ? ? ??2?? ? ? ?3? ?? ? ?4?? ? ???5? ? ? ??6? ? ? ??7? ? ? ? 8? ? ? ? 9? ? ? ??
r??1? ? ????2????????3????????4? ? ? ??5? ? ? ??6? ? ? ??7? ? ? ??8? ? ? ??9? ? ? ??
P 1.000 0.500 0.500 0.458 0.433 0.428 0.414 0.410 0.406?
The probability of selecting the best Applicant in the classical secretary problem converges toward 1/e≈0.368{\displaystyle 1/e\Approx 0.368}?.
在古典秘書(shū)問(wèn)題中,選擇最佳申請(qǐng)人的概率趨于1/e ≈0.368。

Alternative solution
This problem and several modifications can be solved (including the proof of optimality) in a straightforward manner by the odds algorithm, which also has other Applications. Modifications for the secretary problem that can be solved by this algorithm include random availabilities of Applicants, more general hypotheses for Applicants to be of interest to the decision maker, group interviews for Applicants, as well as certain models for a random number of Applicants.

Limitations 局限性
The solution of the secretary problem is only meaningful if it is justified to assume that the Applicants have no knowledge of the decision strategy employed, because early Applicants have no chance at all and may not show up otherwise.[citation needed]
秘書(shū)問(wèn)題的解決只有在以下情況下才有意義: 申請(qǐng)人對(duì)所采用的決策策略一無(wú)所知,因?yàn)樘崆吧暾?qǐng)者根本沒(méi)有機(jī)會(huì),也可能不會(huì)出現(xiàn)。[需要引證]
One important drawback for Applications of the solution of the classical secretary problem is that the number of Applicants n{\displaystyle n}?must be known in advance, which is rarely the case. ?One way to
overcome this problem is to suppose that the number of Applicants is a
random variable N{\displaystyle N}?with a known distribution of??(Presman and Sonin, 1972). For this model, the optimal solution is in
general much harder, however. Moreover, the optimal success probability
is now no longer around 1/e but typically lower. This can be
understood in the context of having a "price" to pay for not knowing the
number of Applicants. However, in this model the price is high.
Depending on the choice of the distribution of N{\displaystyle N}?,
the optimal win probability can Approach zero. Looking for ways to cope
with this new problem led to a new model yielding the so-called 1/e-law
of best choice.
解決傳統(tǒng)秘書(shū)問(wèn)題應(yīng)用中的一個(gè)重要缺點(diǎn)是,必須事先知 道申請(qǐng)人數(shù) n,而這種情況很少發(fā)生。克服這個(gè)問(wèn)題的一個(gè)方法是假設(shè)申請(qǐng)人數(shù)是一個(gè)隨機(jī)變量 n,其分布是 p (n = k) k = 1,2,something (Presman and Sonin,1972)。然而,對(duì)于這個(gè)模型來(lái)說(shuō),最優(yōu)解通常要困難得多。此外,最佳成功概率現(xiàn)在不再是1/e 左右,而是通常更低。這可以理解為,不知道申請(qǐng)人的數(shù)量就要付出”代價(jià)”。然而,在這個(gè)模型中,價(jià)格很高。根據(jù) n 分布的選擇,最優(yōu)勝率可以接近于零。尋找應(yīng)對(duì)這一新問(wèn)題的方法,導(dǎo)致了一種新的模式,產(chǎn)生了所謂的最佳選擇的1/e 法則。

1/e-law of best choice?1/e法則的最佳選擇
The
essence of the model is based on the idea that life is sequential and
that real-world problems pose themselves in real time. Also, it is
easier to estimate times in which specific events (arrivals of
Applicants) should occur more frequently (if they do) than to estimate
the distribution of the number of specific events which will occur. This
idea led to the following Approach, the so-called unified Approach (1984):
這個(gè)模型的本質(zhì)是基于這樣一個(gè)觀點(diǎn),即生活是有序的,現(xiàn)實(shí)世界的問(wèn)題是實(shí)時(shí)出現(xiàn)的。此外,比起估計(jì)將要發(fā)生的特定事件的數(shù)量分布,估計(jì)特定事件(申請(qǐng)人到達(dá))應(yīng)該更頻繁發(fā)生的時(shí)間(如果發(fā)生的話(huà))要容易得多。這一思想導(dǎo)致了以下的方法,即所謂的統(tǒng)一方法(1984年) :
The model is defined as follows: An Applicant must be selected on some time interval [0,T]{\displaystyle [0,T]}?from an unknown number N{\displaystyle N}?of rankable Applicants. The goal is to maximize the probability of selecting only the best under the hypothesis that all arrival orders of different ranks are equally likely. Suppose that all Applicants have the same, but independent to each other, arrival time density f{\displaystyle f}?on [0,T]{\displaystyle [0,T]}?and let ?F{\displaystyle F}?denote the corresponding arrival time distribution function, that is
該模型的定義如下: 申請(qǐng)人必須在一定時(shí)間間隔[0,t ]從一個(gè)未知數(shù) n 的可贖回的申請(qǐng)人中選出。目標(biāo)是在假設(shè)不同等級(jí)的所有到達(dá)順序都是相等的情況下,最大化只選擇最佳的概率。假設(shè)所有申請(qǐng)人都具有相同但彼此獨(dú)立的到達(dá)時(shí)間密度 f [0,t ] ,并且 f 表示相應(yīng)的到達(dá)時(shí)間分布函數(shù),即
,
.
Let τ{\displaystyle \tau } be such that F(τ)=1/e.{\displaystyle F(\tau )=1/e.}?Consider the strategy to wait and observe all Applicants up to time τ{\displaystyle \tau }?and then to select, if possible, the first candidate after time τ{\displaystyle \tau }?which is better than all preceding ones. Then this strategy, called 1/e-strategy, has the following properties:
設(shè) τ 為 f (τ) = 1/e,考慮在 τ 之前等待并觀察所有申請(qǐng)者的策略,如果可能的話(huà),選擇時(shí)間 τ 之后的第一個(gè)候選者,這個(gè)候選者優(yōu)于之前的所有候選者。那么這個(gè)策略,稱(chēng)為1/e-strategy,有以下屬性:
The 1/e-strategy
1/e 策略
(i) yields for all ?(i)所有人的收益N{\displaystyle N} { displaystyle n }?a success probability of at least 1/e, 成功的概率至少是1/e,
(ii) is the unique strategy guaranteeing this lower success probability bound 1/e, and the bound is optimal, (ii)是保證這個(gè)較低成功概率上界1/e 的唯一策略,并且上界是最優(yōu)的,
(iii) selects, if there is at least one Applicant, none at all with probability exactly 1/e. (iii)選擇,如果至少有一名申請(qǐng)人,則沒(méi)有任何一名申請(qǐng)人的概率恰好為1/e
The 1/e-law, proved in 1984 by F. Thomas Bruss, came as a surprise. The reason was that a value of about 1/e had been considered before as being out of reach in a model for unknown N{\displaystyle N}?, whereas this value 1/e was now achieved as a lower bound for the success probability, and this in a model with arguably much weaker hypotheses (see e.g. Math. Reviews 85:m).
1984年,托馬斯?布魯斯(f. Thomas Bruss)證明了1/e 定律,這讓人感到意外。原因是,在一個(gè)未知 n 的模型中,大約1/e 的值以前被認(rèn)為是達(dá)不到的,而這個(gè)值1/e 現(xiàn)在是作為成功概率的一個(gè)下限而達(dá)到的,而這個(gè)下限在一個(gè)假設(shè)可能要弱得多的模型中(例如,數(shù)學(xué))。評(píng)論85: m)。
The 1/e-law is sometimes confused with the solution for the classical secretary problem described above because of the similar role of the number 1/e. However, in the 1/e-law, this role is more general. The result is also stronger, since it holds for an unknown number of Applicants and since the model based on an arrival time distribution F is more tractable for Applications.
由于數(shù)字1/e 的作用相似,1/e 法有時(shí)會(huì)與上述經(jīng)典秘書(shū)問(wèn)題的解決方案相混淆。然而,在1/e 法中,這種作用更為一般。這個(gè)結(jié)果也更有說(shuō)服力,因?yàn)樗m用于未知數(shù)量的申請(qǐng)者,而且基于到達(dá)時(shí)間分布的模型對(duì)于應(yīng)用程序來(lái)說(shuō)更易于處理。

The game of googol 古戈?duì)柕挠螒?/span>
According to Ferguson(1989)[1], the secretary problem Appeared for the first time in print when it was featured by Martin Gardner in his February 1960 Mathematical Games column in Scientific American.[1] Here is how Gardner 1966 formulated it: "Ask someone to take as many slips of paper as he pleases, and on each slip write a different positive number. The numbers may range from small fractions of 1 to a number the size of a googol (1 followed by a hundred zeroes) or even larger. These slips are turned face down and shuffled over the top of a table. One at a time you turn the slips face up. The aim is to stop turning when you come to the number that you guess to be the largest of the series. You cannot go back and pick a previously turned slip. If you turn over all the slips, then of course you must pick the last one turned."
根據(jù) Ferguson (1989)[1]的說(shuō)法,秘書(shū)問(wèn)題在1960年2月 Martin Gardner 在《科學(xué)美國(guó)人》的數(shù)學(xué)游戲?qū)谥惺状纬霈F(xiàn)。[1]加德納1966年是這樣闡述的: “讓某人隨心所欲地拿紙條,并在每張紙條上寫(xiě)上不同的正數(shù)。這些數(shù)字可以是1的小分?jǐn)?shù),也可以是10的100次方(1后面100個(gè)0,巨大的數(shù)字),甚至更大。這些紙條正面朝下,拖曳在桌 面上。一次一個(gè),你把紙條正面朝上。這樣做的目的是當(dāng)你到達(dá)一個(gè)數(shù)字時(shí)停止轉(zhuǎn)動(dòng),你猜這個(gè)數(shù)字是這個(gè)系列中最大的。你不能回到過(guò)去,拿一張之前翻過(guò)的紙 條。如果你把所有的紙條都翻過(guò)來(lái),那么當(dāng)然你必須把最后一張翻過(guò)來(lái)?!?/span>
In the article "Who solved the Secretary problem?" Ferguson(1989)[1] pointed out that the secretary problem remained unsolved as it was stated by M. Gardner, that is as a two-person zero-sum game with two antagonistic players. In this game Alice, the informed player, writes secretly distinct numbers on n{\displaystyle n}?cards. Bob, the stopping player, observes the actual values and can stop turning cards whenever he wants, winning if the last card turned has the overall maximal number. The difference with the basic secretary problem is that Bob observes the actual values written on the cards, which he can use in his decision procedures. The numbers on cards are Analogous to the numerical qualities of Applicants in some versions of the secretary problem. The joint probability distribution of the numbers is under the control of Alice.
在文章“誰(shuí)解決了秘書(shū)問(wèn)題?”弗格森(1989) [1]指出,秘書(shū)問(wèn)題仍然沒(méi)有解決,因?yàn)樗怯杉拥录{說(shuō),這是一個(gè)兩人零和對(duì)策,兩個(gè)敵對(duì)的參與者。在這個(gè)游戲中,知情的玩家愛(ài)麗絲在 n 張卡片上秘密寫(xiě)下不同的數(shù)字。停牌玩家鮑勃觀察實(shí)際數(shù)值,可以隨時(shí)停止翻牌,如果最后一張牌翻出的牌總數(shù)最大,他就贏?;久貢?shū)問(wèn)題的不同之處在于鮑勃觀 察卡片上寫(xiě)的實(shí)際價(jià)值,這些價(jià)值可以用于他的決策過(guò)程??ㄆ系臄?shù)字類(lèi)似于秘書(shū)問(wèn)題的某些版本中申請(qǐng)者的數(shù)字質(zhì)量。數(shù)字的聯(lián)合分布在 Alice 的控制之下。
Bob wants to guess the maximal number with the highest possible probability, while Alice's goal is to keep this probability as low as possible. It is not optimal for Alice to sample the numbers independently from some fixed distribution, and she can play better by choosing random numbers in some dependent way. For n=2{\displaystyle n=2}?Alice has no minimax strategy, which is closely related to a paradox of T. Cover. But for n>2{\displaystyle n>2}?the game has a solution: Alice can choose random numbers (which are dependent random variables) in such a way that Bob cannot play better than using the classical stopping strategy based on the relative ranks (Gnedin 1994).
鮑勃想用最高的概率來(lái)猜測(cè)最大的數(shù)字,而愛(ài)麗絲的目標(biāo) 是盡可能保持最低的概率。對(duì)于愛(ài)麗絲來(lái)說(shuō),獨(dú)立于某個(gè)固定分布的數(shù)字樣本并不是最優(yōu)的,她可以通過(guò)某種相關(guān)的方式選擇隨機(jī)數(shù)來(lái)發(fā)揮更好的作用。對(duì)于 n = 2,Alice 沒(méi)有極大極小策略,這與 t. Cover 悖論密切相關(guān)。但是對(duì)于 n > 2,游戲有一個(gè)解決方案: Alice 可以選擇隨機(jī)數(shù)(相依隨機(jī)變量) ,這樣 Bob 就不能比使用基于相對(duì)等級(jí)的經(jīng)典停止策略玩得更好(Gnedin 1994)。

Heuristic performance 啟發(fā)式性能
The remainder of the article deals again with the secretary problem for a known number of Applicants.
文章的其余部分再次涉及已知數(shù)量的申請(qǐng)人的秘書(shū)問(wèn)題。
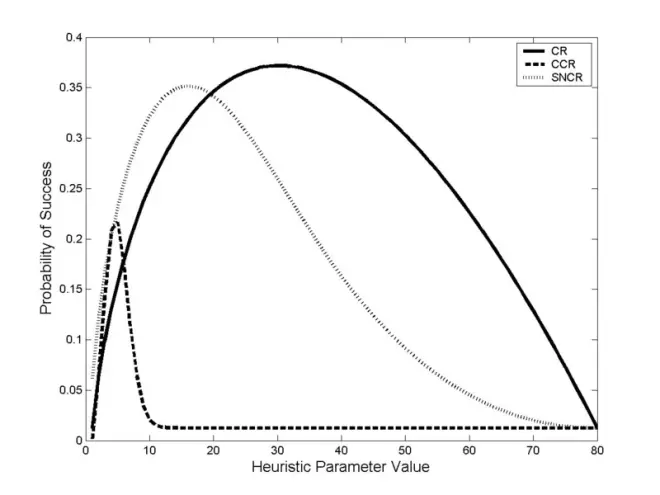
Stein, Seale & Rapoport 2003 derived the expected success probabilities for several psychologically plausible heuristics that might be employed in the secretary problem. The heuristics they examined were:
2003年,Stein,Seale & Rapoport 推導(dǎo)出了幾種可能用于秘書(shū)問(wèn)題的心理學(xué)上合理的啟發(fā)式方法的預(yù)期成功概率。他們調(diào)查的啟發(fā)式問(wèn)題是:
The cutoff rule (CR): Do not accept any of the first ?截止規(guī)則(CR) : 不要接受任何第一個(gè)y Applicants; thereafter, select the first encountered candidate (i.e., an Applicant with relative rank 1). This rule has as a special case the optimal policy for the classical secretary problem for which ?然后,選擇第一個(gè)遇到的候選人(即相對(duì)職級(jí)1的申請(qǐng)人)。該規(guī)則作為一個(gè)特例,對(duì)于經(jīng)典的秘書(shū)問(wèn)題具有最優(yōu)策略y?=?r.
Candidate count rule (CCR): Select the ?候選人計(jì)數(shù)規(guī)則(CCR) : 選擇y-th encountered candidate. Note, that this rule does not necessarily skip any Applicants; it only considers how many candidates have been observed, not how deep the decision maker is in the Applicant sequence. 遇到的候選人。請(qǐng)注意,這條規(guī)則并不一定忽略任何申請(qǐng)人; 它只考慮有多少候選人被遵守,而不考慮決策者在申請(qǐng)人序列中的深度
Successive non-candidate rule (SNCR): Select the first encountered candidate after observing ?連續(xù)非候選規(guī)則(SNCR) : 在觀察后選擇第一個(gè)遇到的候選者y non-candidates (i.e., Applicants with relative rank?>?1). 非候選人(即相對(duì)職級(jí) > 1的申請(qǐng)人)
Each heuristic has a single parameter y. The figure (shown on right) displays the expected success probabilities for each heuristic as a function of y for problems with n?=?80.
每種啟發(fā)式方法都有一個(gè)參數(shù) y。該圖(如右圖所示)顯示了對(duì)于 n = 80的問(wèn)題,每個(gè)啟發(fā)式算法的預(yù)期成功概率作為 y 的函數(shù)。

Cardinal payoff variant 基數(shù)回報(bào)變量
Finding the single best Applicant might seem like a rather strict objective. One can imagine that the interviewer would rather hire a higher-valued Applicant than a lower-valued one, and not only be concerned with getting the best. That is, the interviewer will derive some value from selecting an Applicant that is not necessarily the best, and the derived value increases with the value of the one selected.
找到一個(gè)最好的申請(qǐng)人似乎是一個(gè)相當(dāng)嚴(yán)格的目標(biāo)。我們可以想象,面試官寧愿雇傭一個(gè)價(jià)值較高的應(yīng)聘者,也不愿雇傭一個(gè)價(jià)值較低的應(yīng)聘者,而且不僅僅關(guān)心如何得到最好的應(yīng)聘者。也就是說(shuō),面試官會(huì)從選擇一個(gè)不一定是最好的應(yīng)聘者中得到一些價(jià)值,并且得到的價(jià)值會(huì)隨著被選中的應(yīng)聘者的價(jià)值而增加。
To model this problem, suppose that the n{\displaystyle n}?Applicants have "true" values that are random variables X drawn i.i.d. from a uniform distribution on [0,?1]. Similar to the classical problem described above, the interviewer only observes whether each Applicant is the best so far (a candidate), must accept or reject each on the spot, and must accept the last one if he/she is reached. (To be clear, the interviewer does not learn the actual relative rank of each Applicant. He/she learns only whether the Applicant has relative rank 1.) However, in this version the payoff is given by the true value of the selected Applicant. For example, if he/she selects an Applicant whose true value is 0.8, then he/she will earn 0.8. The interviewer's objective is to maximize the expected value of the selected Applicant.
為了對(duì)這個(gè)問(wèn)題進(jìn)行建模,假設(shè) n 個(gè)申請(qǐng)人的“真”值是隨機(jī)變量 x 從[0,1]上的均勻分布中抽取的 i.i.i.i.d. 。與上面描述的經(jīng)典問(wèn)題類(lèi)似,面試官只是觀察每個(gè)應(yīng)聘者是否是目前為止最好的(候選人) ,必須當(dāng)場(chǎng)接受或拒絕每個(gè)人,如果他/她被錄取,必須接受最后一個(gè)人。(需要說(shuō)明的是,面試官并不知道每個(gè)應(yīng)聘者的實(shí)際相對(duì)排名。他/她只知道申請(qǐng)人是否有相對(duì)等級(jí)1。)然而,在這個(gè)版本中,回報(bào)是由被選中的申請(qǐng)人的真實(shí)價(jià)值決定的。例如,如果他/她選擇一個(gè)真實(shí)值為0.8的申請(qǐng)人,那么他/她將獲得0.8。面試官的目標(biāo)是最大化被選中的應(yīng)聘者的期望價(jià)值。
Since the Applicant's values are i.i.d. draws from a uniform distribution on [0,?1], the expected value of the tth Applicant given that??is given by
由于申請(qǐng)人的值是從[0,1]上的均勻分布中得到的 i.i.i.d,所以在 xt = max { x1,x2,... ,xt }的情況下,tth(第t個(gè)) 申請(qǐng)人的期望值是由
As in the classical problem, the optimal policy is given by a threshold, which for this problem we will denote by c{\displaystyle c}?, at which the interviewer should begin accepting candidates. Bearden 2006 showed that c is either??or?
. (In fact, whichever is closest to?
.) This follows from the fact that given a problem with n{\displaystyle n}?Applicants, the expected payoff for some arbitrary threshold 1≤c≤n{\displaystyle 1\leq c\leq n}?is
在經(jīng)典問(wèn)題中,最優(yōu)策略由一個(gè)閾值給出,對(duì)于這個(gè)問(wèn)題,我們將用 c 表示,從這個(gè)閾值開(kāi)始,面試官應(yīng)該開(kāi)始接受候選人。Bearden 2006年的研究表明,c 要么是“根號(hào)n向下取整”,要么是“根號(hào)n向上取整”。(事實(shí)上,無(wú)論哪個(gè)最接近 n。)這源于這樣一個(gè)事實(shí),即給定一個(gè)有 n 個(gè)申請(qǐng)人的問(wèn)題,某個(gè)任意閾值1≤ c ≤ n 的預(yù)期收益是
Differentiating Vn(c){\displaystyle V_{n}(c)}?with respect to c, one gets
關(guān)于 c 的微分式 Vn (c) ,得到
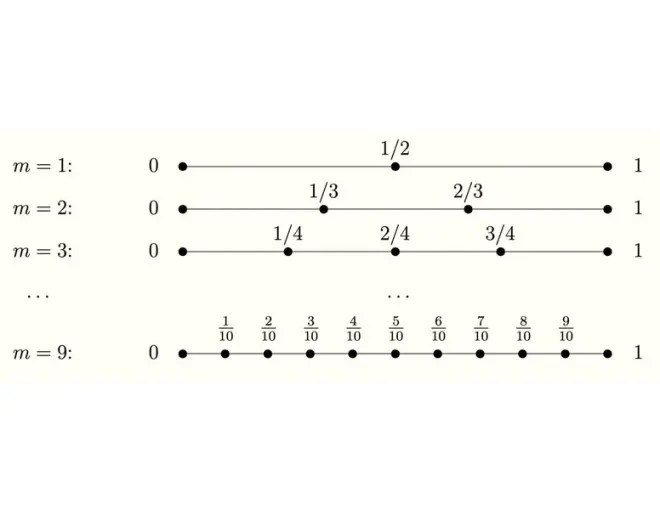
Since??for all permissible values of c{\displaystyle c}?, we find that V{\displaystyle V}?is maximized at?
. Since V is convex in c{\displaystyle c}?, the optimal integer-valued threshold must be either?
?or?
. Thus, for most values of n{\displaystyle n}?the interviewer will begin accepting Applicants sooner in the cardinal
payoff version than in the classical version where the objective is to
select the single best Applicant. Note that this is not an asymptotic
result: It holds for all n{\displaystyle n}?.
However, this is not the optimal policy to maximize expected value from
a known distribution. In the case of a known distribution, optimal play
can be calculated via dynamic programming.
由于對(duì)于所有 c 的許可標(biāo)準(zhǔn)均采用?2V/?c2 < 0,我們發(fā)現(xiàn)在 c = n 時(shí) v 最大。因?yàn)?v 在 c 中是凸的,所以最佳整數(shù)值閾值必須是 “根號(hào)n向下取整”?或 “根號(hào)n向上取整”。因此,對(duì)于 n 的大多數(shù)值,面試官在基本支付版本中開(kāi)始接受應(yīng)聘者的時(shí)間要比在傳統(tǒng)版本中開(kāi)始接受應(yīng)聘者的時(shí)間要短,傳統(tǒng)版本的目標(biāo)是選擇最好的應(yīng)聘者。注意這不是一個(gè)漸近結(jié)果: 它適用于所有的 n。然而,這不是從已知分布最大化期望值的最優(yōu)策略。在已知分布的情況下,最佳發(fā)揮可以通過(guò)動(dòng)態(tài)規(guī)劃計(jì)算。
A more general form of this problem introduced by Palley and Kremer (2014)[3] assumes that as each new Applicant arrives, the interviewer observes their rank relative to all of the Applicants that have been observed previously. This model is consistent with the notion of an interviewer learning as they continue the search process by accumulating a set of past data points that they can use to evaluate new candidates as they arrive. A benefit of this so-called partial-information model is that decisions and outcomes achieved given the relative rank information can be directly compared to the corresponding optimal decisions and outcomes if the interviewer had been given full information about the value of each Applicant. This full-information problem, in which Applicants are drawn independently from a known distribution and the interviewer seeks to maximize the expected value of the Applicant selected, was originally solved by Moser (1956),[4] Sakaguchi (1961),[5] and Karlin (1962).
Palley 和 Kremer (2014)[3]提出了這個(gè)問(wèn)題的一個(gè)更普遍的形式,假設(shè)每一個(gè)新的申請(qǐng)者到來(lái)時(shí),面試官都會(huì)觀察他們相對(duì)于之前所觀察到的所有申請(qǐng)者的排名。這個(gè)模型符合面試官在繼續(xù)搜索過(guò)程中學(xué)習(xí)的概念,通過(guò)積累一系列過(guò)去的數(shù)據(jù)點(diǎn),他們可以用這些數(shù)據(jù)點(diǎn)來(lái)評(píng)估新的應(yīng)聘者。這種所謂的部分信息模型的一個(gè)好處是,如果面試官獲得了關(guān)于每個(gè)申請(qǐng)人價(jià)值的全部信息,那么根據(jù)相對(duì)排名信息所作出的決定和取得的結(jié)果可以直接與相應(yīng)的最佳決定和結(jié)果進(jìn)行比較。這個(gè)完全信息問(wèn)題是由 Moser (1956) ,Sakaguchi (1961) ,[5]和 Karlin (1962)最初解決的。
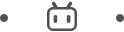
Other modifications 其他修訂
There are several variants of the secretary problem that also have simple and elegant solutions.
秘書(shū)問(wèn)題有幾種變體,也有簡(jiǎn)單而優(yōu)雅的解決方案。
One variant replaces the desire to pick the best with the desire to pick the second-best.[6][7][8] Robert J. Vanderbei
calls this the "postdoc" problem arguing that the "best" will go to
Harvard. ?For this problem, the probability of success for an even
number of Applicants is exactly?. This probability tends
to 1/4 as n tends to infinity illustrating the fact that it is easier to
pick the best than the second-best.
一種變體取代了選擇最好的人的愿望,取而代之的是選擇次好的人。羅伯特 · j · 范德貝把這個(gè)問(wèn)題稱(chēng)為“博士后”問(wèn)題,他認(rèn)為“最好的”應(yīng)該去哈佛。對(duì)于這個(gè)問(wèn)題,偶數(shù)申請(qǐng)者成功的概率正好是0.25 n2n (n-1)。這個(gè)概率趨向于1/4,因?yàn)?n 趨向于無(wú)窮大,這說(shuō)明選擇最好的比選擇次好的容易。
For a second variant, the number of selections is specified to be
greater than one. ?In other words, the interviewer is not hiring just
one secretary but
rather is, say, admitting a class of students from an Applicant pool. ?
Under the assumption that success is achieved if and only if all the
selected candidates
are superior to all of the not-selected candidates, it is again a
problem that can be solved. ?It was shown in Vanderbei 1980
that when n is even and the desire is to select exactly half the
candidates, the optimal strategy yields a success probability of?.?
對(duì)于第二個(gè)變體,選擇的數(shù)目被指定為大于一。換句話(huà)說(shuō),面試官不是只雇傭一個(gè)秘書(shū),而是從應(yīng)聘者中錄取一類(lèi)學(xué)生。假定只有當(dāng)且僅當(dāng)所有被選中的候選人都優(yōu)于所有未被選中的候選人時(shí),才能取得成功,這又是一個(gè)可以解決的問(wèn)題。Vanderbei 1980年的研究表明,當(dāng) n 為偶 數(shù)且希望選出正好一半的候選者時(shí),最優(yōu)策略產(chǎn)生1 n/2 + 1的成功概率。
Another variant is that of selecting the best k{\displaystyle k}?secretaries out of a pool of n{\displaystyle n}?, again in an on-line algorithm. This leads to a strategy related to the classic one and cutoff threshold of?for which the classic problem is a special case Ghirdar 2009.
另一種變體是從 n 個(gè)秘書(shū)池中選擇最好的 k 個(gè)秘書(shū),同樣是在聯(lián)機(jī)算法中。這導(dǎo)致了一個(gè)與經(jīng)典的策略和0.25 n2/n (n-1)的臨界閾值相關(guān)的經(jīng)典問(wèn)題是一個(gè)特例 Ghirdar 2009。

Multiple choice problem 多項(xiàng)選擇題
A player is allowed r{\displaystyle r}?choices, and he wins if any choice is the best.Gilbert & Mosteller 1966
showed that an optimal strategy is given by a threshold strategy
(cutoff strategy). ?An optimal strategy belongs to the class of
strategies defined by a set of threshold numbers?, where?
. The first choice is to be used on the first candidates starting with?th Applicant, and once the first choice is used, second choice is to be used on the first candidate starting with th Applicant, and so on.
一個(gè)玩家可以有 r 個(gè)選擇,如果任何一個(gè)選擇是最好的,那么他就贏了。Gilbert & Mosteller 1966證明了一個(gè)最優(yōu)策略是由一個(gè)閾值策略(截止策略)給出的。最優(yōu)策略屬于由一組閾值數(shù)字(a1,a2,... ,ar)定義的策略類(lèi),其中 a1 < a2 < ar。第一個(gè)選擇用于第一個(gè)申請(qǐng)人,第一個(gè)選擇用于第二個(gè)申請(qǐng)人,第二個(gè)選擇用于第一個(gè)申請(qǐng)人,以此類(lèi)推。
Gilbert and Mosteller showed that?
.
For further cases that r=5,6,...,10{\displaystyle r=5,6,...,10}?, see Matsui & Ano 2016 (for example?).?
Gilbert 和 Mosteller 證明(a1n,a2n,a3n,a4n)→(e-1,e-32,e-4724,e-27611152)(n →∞)。關(guān)于 r = 5,6,... ,10的進(jìn)一步情況,見(jiàn) Matsui & Ano 2016(例如 a5n → e-41626371474560)。
When r=2{\displaystyle r=2}?, the probability of win converges to??(Gilbert & Mosteller 1966).Matsui & Ano 2016 showed that for any positive integer r{\displaystyle r}?, the probability of win (of r{\displaystyle r}?choice secretary problem) converges to?
?where?
. Thus, the probability of win converges to?
?and?
?when r=3,4{\displaystyle r=3,4}?respectively.
當(dāng) r = 2時(shí),獲勝概率收斂到 e-1 + e-32(n →∞)(Gilbert & Mosteller 1966) 。Matsui & Ano 2016證明了對(duì)于任意正整數(shù) r,r 選擇秘書(shū)問(wèn)題的勝算概率收斂于 p1 + p2 + something + pr,其中 pi = limn →∞。因此,當(dāng) r = 3,4時(shí),勝利的概率分別收斂到 e-1 + e-32 + e-4724和 e-1 + e-32 + e-4724 + e-27611152。

Experimental studies
Experimental psychologists and economists have studied the decision behavior of actual people in secretary problem situations.[9] In large part, this work has shown that people tend to stop searching too soon. This may be explained, at least in part, by the cost of evaluating candidates. In real world settings, this might suggest that people do not search enough whenever they are faced with problems where the decision alternatives are encountered sequentially. For example, when trying to decide at which gas station along a highway to stop for gas, people might not search enough before stopping. If true, then they would tend to pay more for gas than if they had searched longer. The same may be true when people search online for airline tickets. Experimental research on problems such as the secretary problem is sometimes referred to as behavioral operations research.
實(shí)驗(yàn)心理學(xué)家和經(jīng)濟(jì)學(xué)家研究了實(shí)際人在秘書(shū)問(wèn)題情境中 的決策行為。[9]在很大程度上,這項(xiàng)研究表明,人們往往過(guò)早地停止搜索。這至少部分可以用評(píng)估候選人的成本來(lái)解釋。在現(xiàn)實(shí)世界的環(huán)境中,這可能意味著當(dāng) 人們遇到?jīng)Q策選擇依次出現(xiàn)的問(wèn)題時(shí),他們搜索的不夠。例如,當(dāng)試圖決定在高速公路上的哪個(gè)加油站加油時(shí),人們可能在停車(chē)前搜索不夠。如果這是真的,那么他 們將傾向于支付更多的天然氣比如果他們搜索更長(zhǎng)的時(shí)間。當(dāng)人們?cè)诰W(wǎng)上搜索機(jī)票時(shí),情況可能也是如此。對(duì)秘書(shū)問(wèn)題等問(wèn)題的實(shí)驗(yàn)研究有時(shí)被稱(chēng)為行為操作研究。

Neural correlates
While there is a substantial body of neuroscience research on information integration, or the representation of belief, in perceptual decision-making tasks using both animal[10][11] and human subjects,[12] there is relatively little known about how the decision to stop gathering information is arrived at.
Researchers have studied the neural bases of solving the secretary problem in healthy volunteers using functional MRI.[13] A Markov decision process (MDP) was used to quantify the value of continuing to search versus committing to the current option. Decisions to take versus decline an option engaged parietal and dorsolateral prefrontal cortices, as well ventral striatum, anterior insula, and anterior cingulate. Therefore, brain regions previously implicated in evidence integration and reward representation encode threshold crossings that trigger decisions to commit to a choice.

History
The secretary problem was Apparently introduced in 1949 by Merrill M. Flood, who called it the fiancée problem in a lecture he gave that year. He referred to it several times during the 1950s, for example, in a conference talk at Purdue on 9 May 1958, and it eventually became widely known in the folklore although nothing was published at the time. In 1958 he sent a letter to Leonard Gillman, with copies to a dozen friends including Samuel Karlin and J. Robbins, outlining a proof of the optimum strategy, with an Appendix by R. Palermo who proved that all strategies are dominated by a strategy of the form "reject the first p unconditionally, then accept the next candidate who is better". (See Flood (1958).)
The first publication was Apparently by Martin Gardner in Scientific American, February 1960. He had heard about it from John H. Fox Jr., and L. Gerald Marnie, who had independently come up with an equivalent problem in 1958; they called it the "game of googol". Fox and Marnie did not know the optimum solution; Gardner asked for advice from Leo Moser, who (together with J. R. Pounder) provided a correct Analysis for publication in the magazine. Soon afterwards, several mathematicians wrote to Gardner to tell him about the equivalent problem they had heard via the grapevine, all of which can most likely be traced to Flood's original work.
The 1/e-law of best choice is due to F. Thomas Bruss (1984).
Ferguson (1989) has an extensive bibliography and points out that a similar (but different) problem had been considered by Arthur Cayley in 1875 and even by Johannes Kepler long before that.
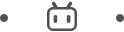
Combinatorial generalization
The secretary problem can be generalized to the case where there are multiple different jobs. Again, there are n{\displaystyle n}?Applicants coming in random order. When a candidate arrives, she reveals a set of nonnegative numbers. Each value specifies her qualification for one of the jobs. The administrator not only has to decide whether or not to take the Applicant but, if so, also has to assign her permanently to one of the jobs. The objective is to find an assignment where the sum of qualifications is as big as possible. This problem is identical to finding a maximum-weight matching in an edge-weighted bipartite graph where the n{\displaystyle n}?nodes of one side arrive online in random order. Thus, it is a special case of the online bipartite matching problem.
By a generalization of the classic algorithm for the secretary problem, it is possible to obtain an assignment where the expected sum of qualifications is only a factor of e{\displaystyle e}?less than an optimal (offline) assignment.[14]

See also
Economics portal
Mathematics portal
Assignment problem
Odds algorithm
Optimal stopping
Robbins' problem
Search theory
Stable marriage problem

Notes
Ferguson, Thomas S. (August 1989). "Who Solved the Secretary Problem?". Statistical Science. 4 (3): 282–289. doi:10.1214/ss/1177012493.
Hill, Theodore P. (2009). "Knowing When to Stop". American Scientist. 97 (2): 126–133. doi:10.1511/2009.77.126. ISSN?1545-2786 – via (For French translation, see cover story in the July issue of Pour la Science (2009)).
Palley, Asa B.; Kremer, Mirko (8 July 2014). "Sequential Search and Learning from Rank Feedback: Theory and Experimental Evidence". Management Science. 60 (10): 2525–2542. doi:10.1287/mnsc.2014.1902. ISSN?0025-1909.
Moser, Leo (1956). "On a problem of Cayley". Scripta Math. 22: 289–292.
Sakaguchi, Minoru (1 June 1961). "Dynamic programming of some sequential sampling design". Journal of Mathematical Analysis and Applications. 2 (3): 446–466. doi:10.1016/0022-247X(61)90023-3. ISSN?0022-247X.
Rose, John S. (1982). "Selection of nonextremal candidates from a random sequence". J. Optim. Theory Appl. 38 (2): 207–219. doi:10.1007/BF00934083. ISSN?0022-3239. S2CID?121339045.
Szajowski, Krzysztof (1982). "Optimal choice of an object with ath rank". Matematyka Stosowana. Annales Societatis Mathematicae Polonae, Series III. 10 (19): 51–65. doi:10.14708/ma.v10i19.1533. ISSN?0137-2890.
Vanderbei, Robert J. (21 June 2021). "The postdoc variant of the secretary problem". Mathematica Applicanda. Annales Societatis Mathematicae Polonae, Series III. 49 (1): 3–13. ISSN?2299-4009.
Bearden, Murphy, and Rapoport, 2006; Bearden, Rapoport, and Murphy, 2006; Seale and Rapoport, 1997; ?2006; Bearden,Rapoport,and Murphy,2006; Seale and Rapoport,1997;Palley and Kremer, 2014
Shadlen, M. N.; Newsome, W. T. (23 January 1996). "Motion perception: seeing and deciding". Proceedings of the National Academy of Sciences. 93 (2): 628–633. Bibcode:1996PNAS...93..628S. doi:10.1073/pnas.93.2.628. PMC?40102. PMID?8570606.
Roitman, Jamie D.; Shadlen, Michael N. (1 November 2002). "Response of Neurons in the Lateral Intraparietal Area during a Combined Visual Discrimination Reaction Time Task". The Journal of Neuroscience. 22 (21): 9475–9489. doi:10.1523/JNEUROSCI.22-21-09475.2002. PMC?6758024. PMID?12417672.
Heekeren, Hauke R.; Marrett, Sean; Ungerleider, Leslie G. (9 May 2008). "The neural systems that mediate human perceptual decision making". Nature Reviews Neuroscience. 9 (6): 467–479. doi:10.1038/nrn2374. PMID?18464792. S2CID?7416645.
Costa, V. D.; Averbeck, B. B. (18 October 2013). "Frontal-Parietal and Limbic-Striatal Activity Underlies Information Sampling in the Best Choice Problem". Cerebral Cortex. 25 (4): 972–982. doi:10.1093/cercor/bht286. PMC?4366612. PMID?24142842.
Kesselheim, Thomas; Radke, Klaus; T?nnis, Andreas; V?cking, Berthold (2013). "An Optimal Online Algorithm for Weighted Bipartite Matching and Extensions to Combinatorial Auctions". Algorithms – ESA 2013. Lecture Notes in Computer Science. 8125. pp.?589–600. doi:10.1007/978-3-642-40450-4_50. ISBN?978-3-642-40449-8.

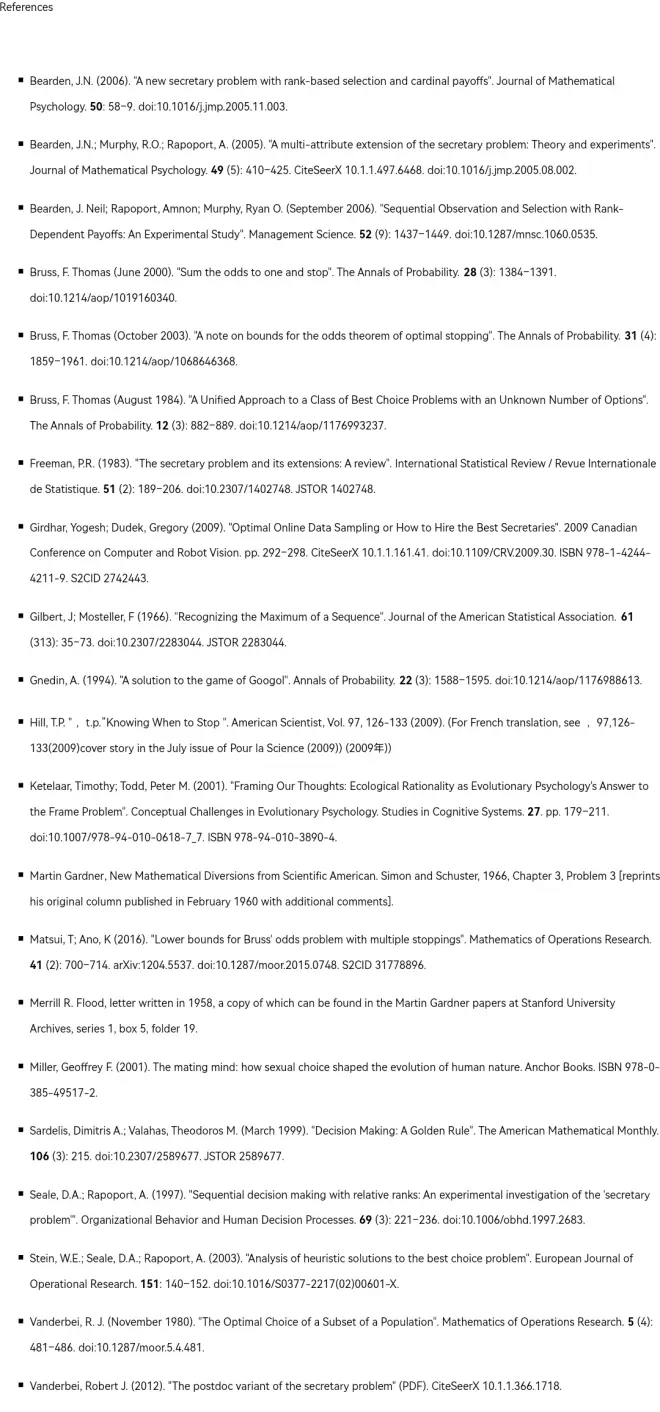

OEISsequence A054404 (Number of daughters to wait before picking in sultan's dowry problem with n daughters)?
Weisstein, Eric W. "Sultan's Dowry Problem". MathWorld.
Neil Bearden. "Optimal Search (Secretary Problems)". Archived from the original on 4 January 2017.
Optimal Stopping and Applications?book by Thomas S. Ferguson
External links
Wikipedia END
上文提到的帶有多次停頓的 bruss 賠率問(wèn)題的下界
https://arxiv.org/pdf/1204.5537.pdf
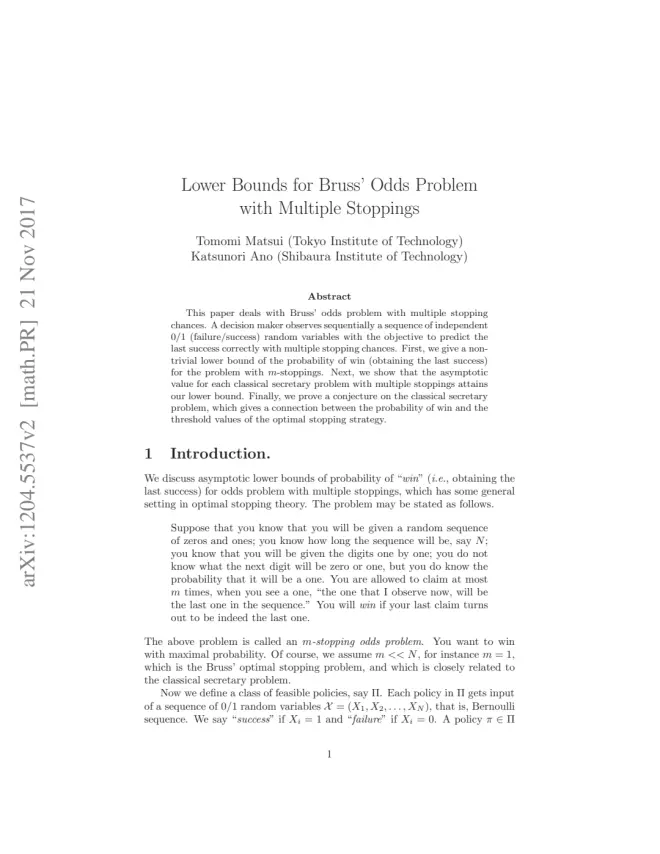
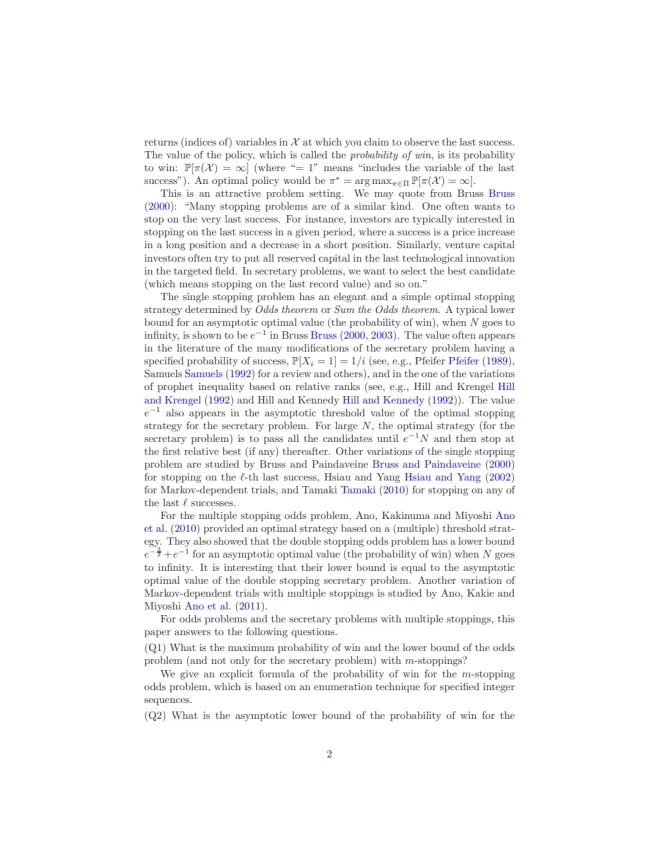
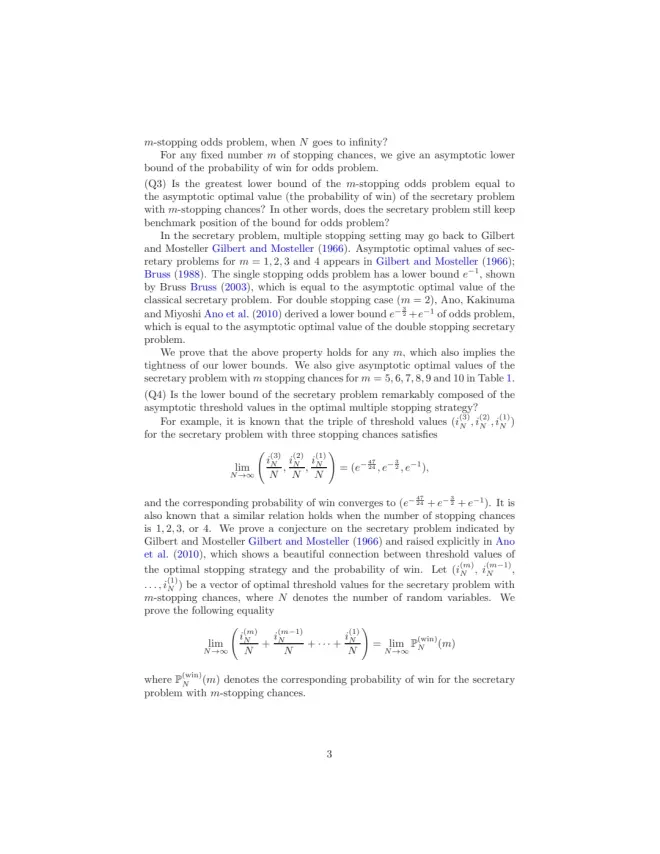
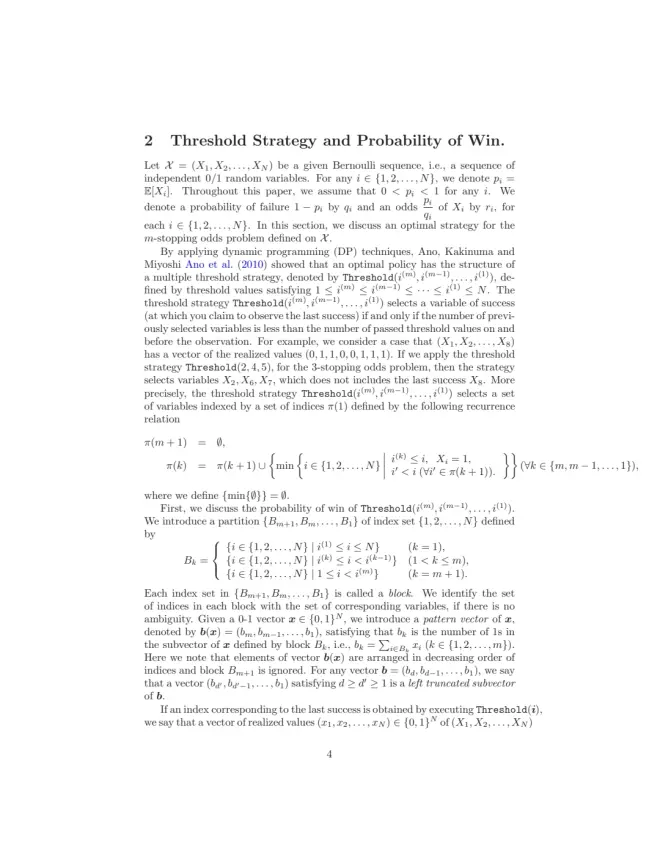
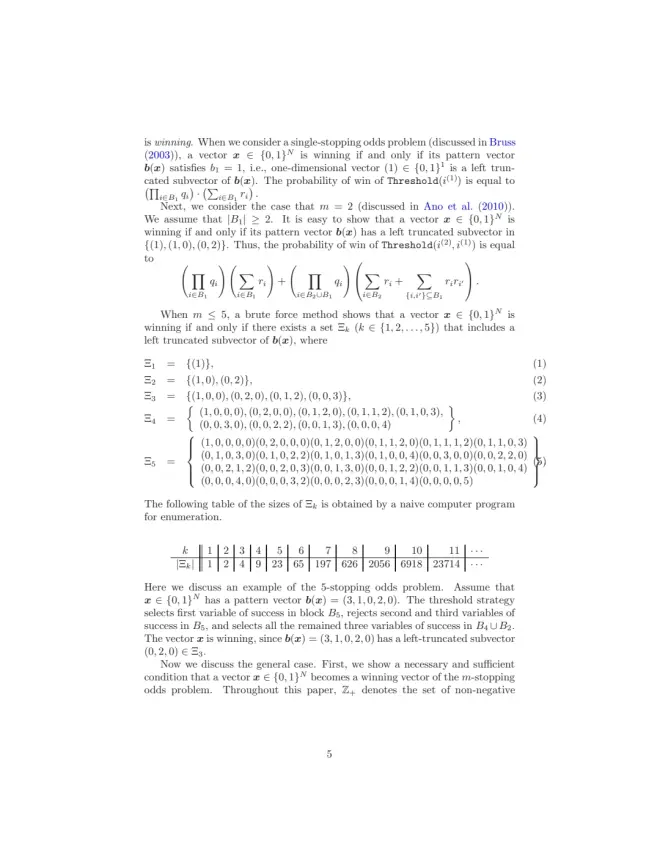
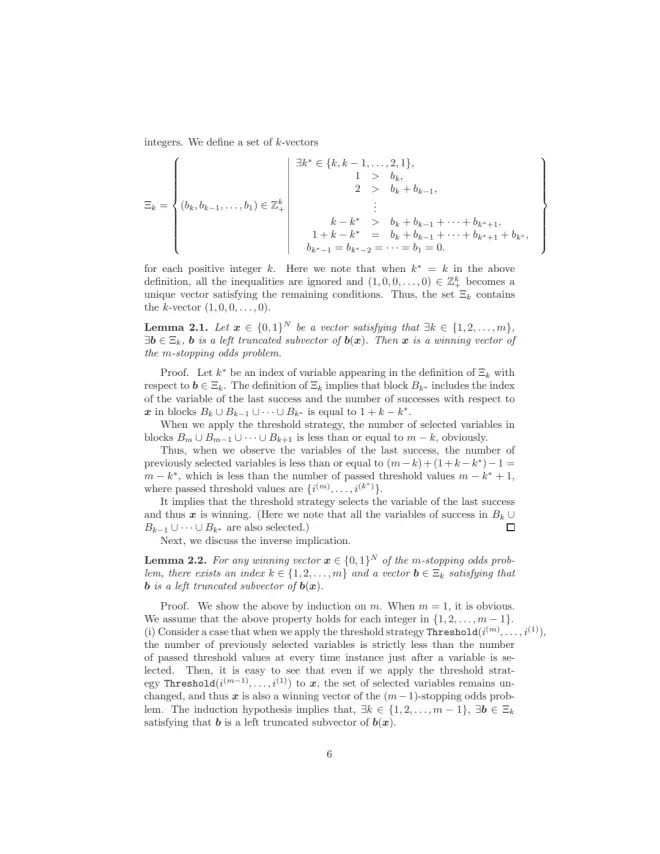
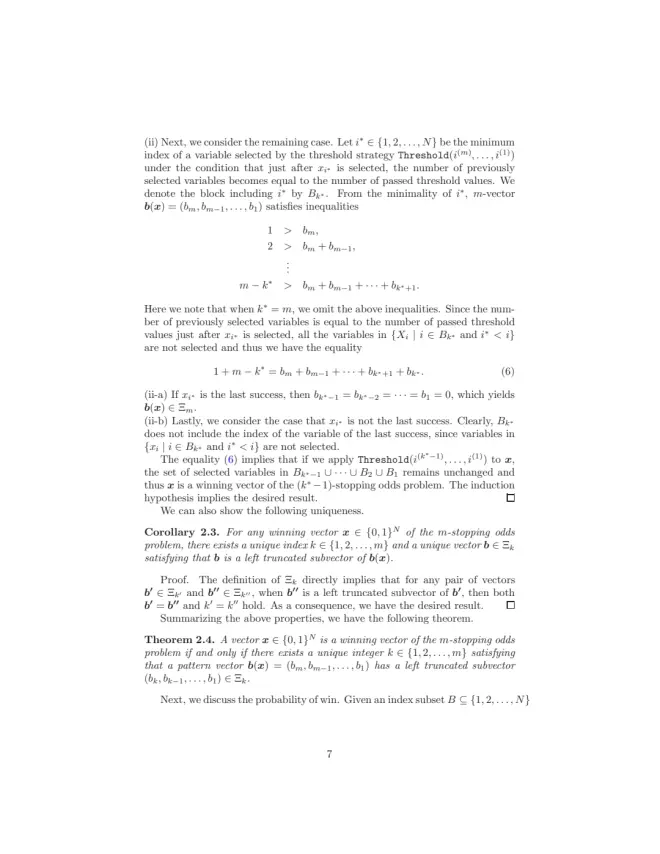
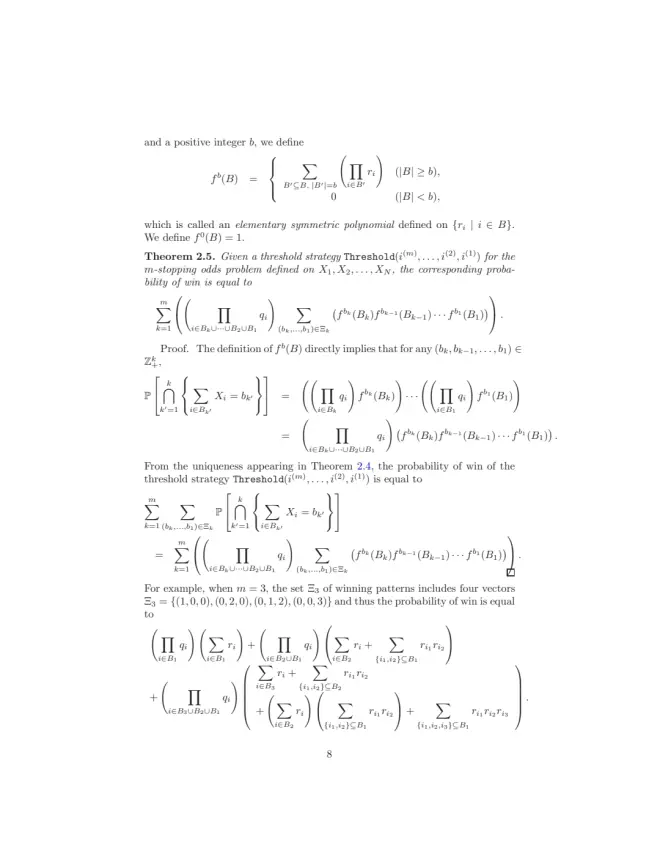
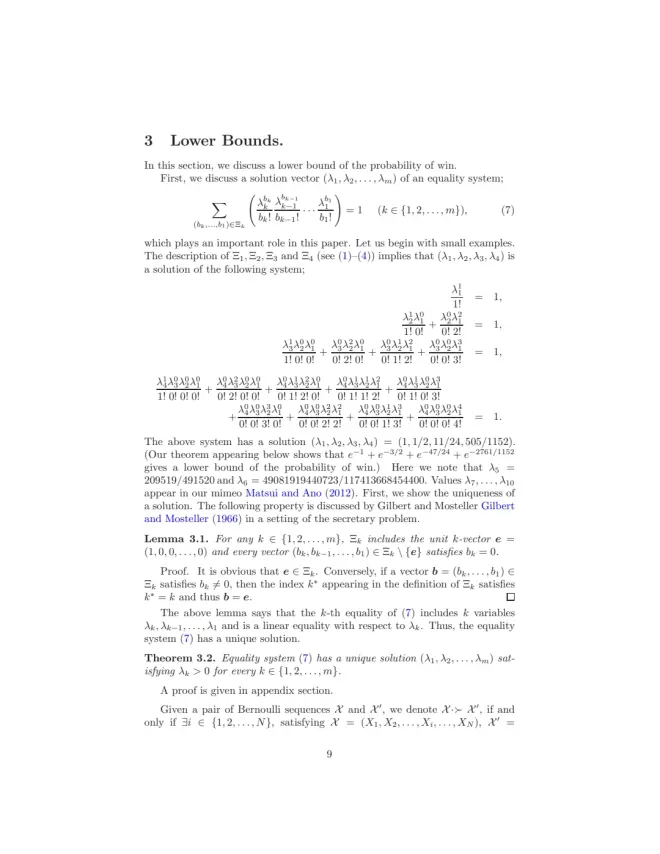
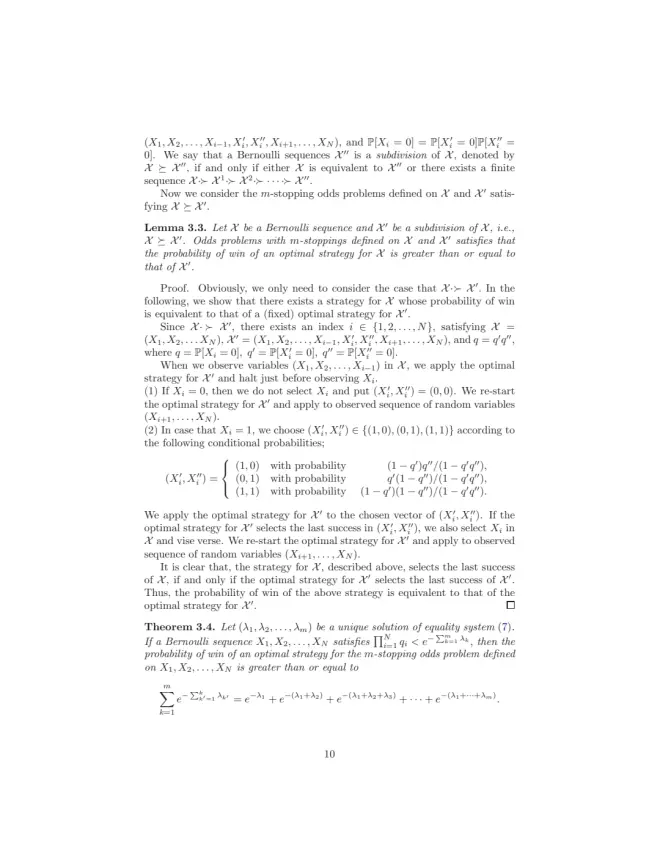
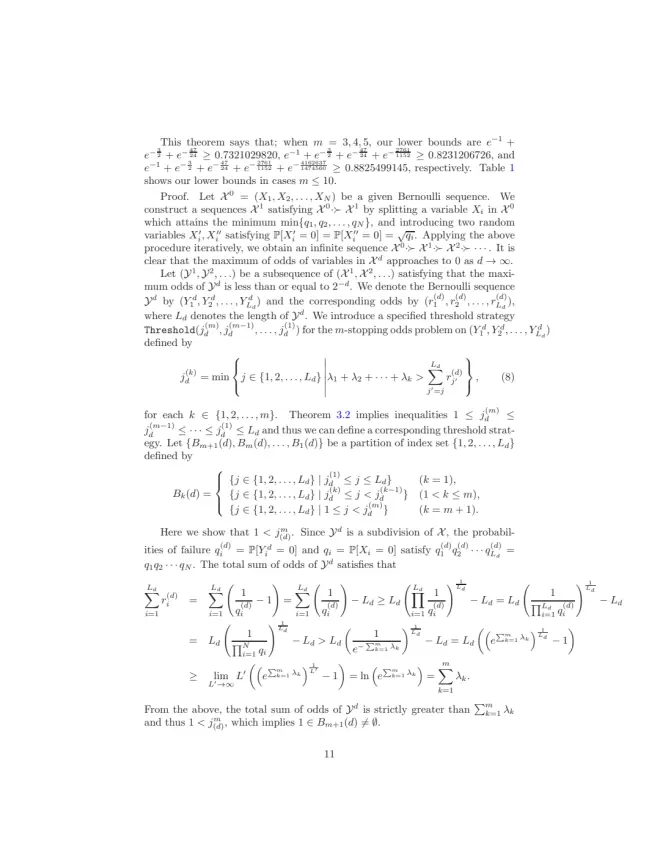
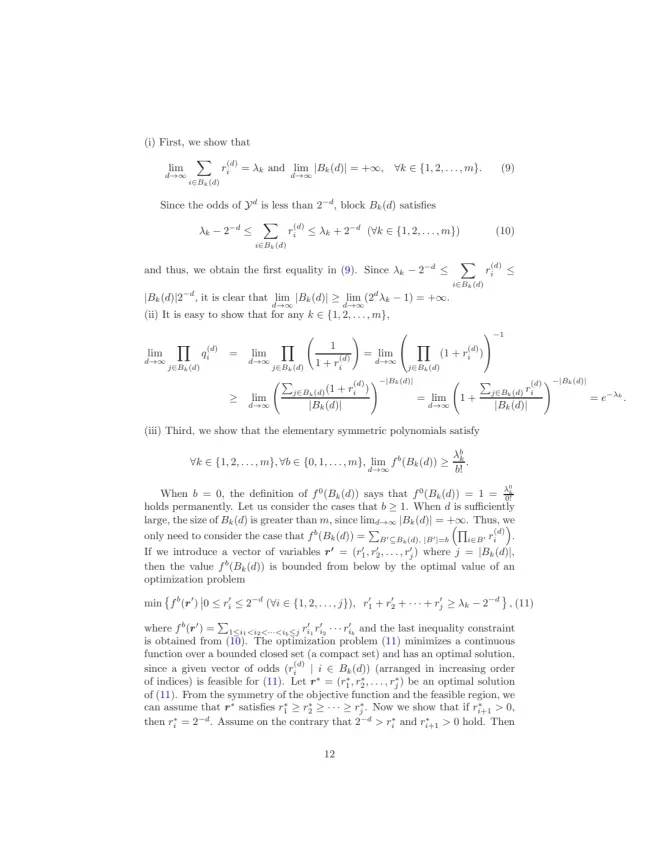
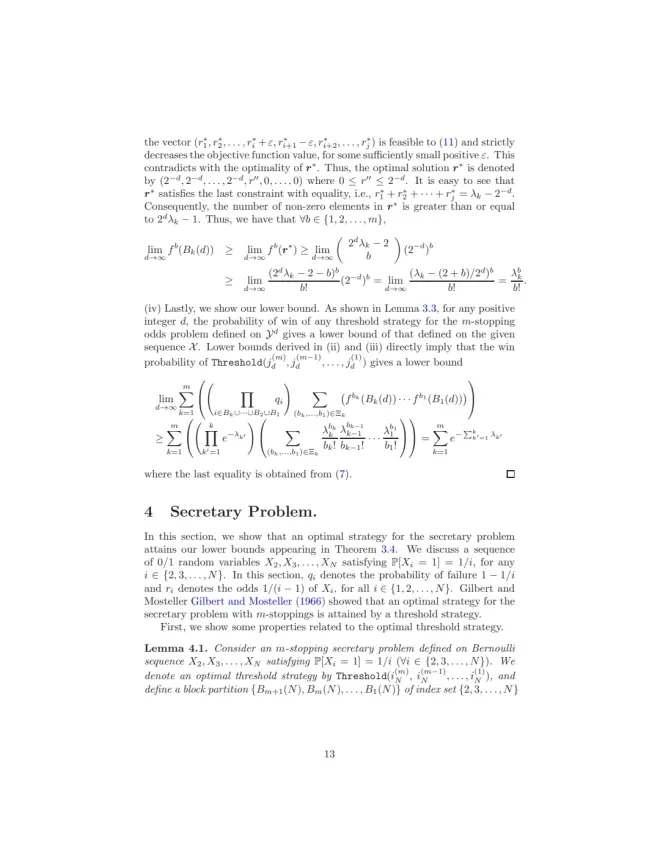
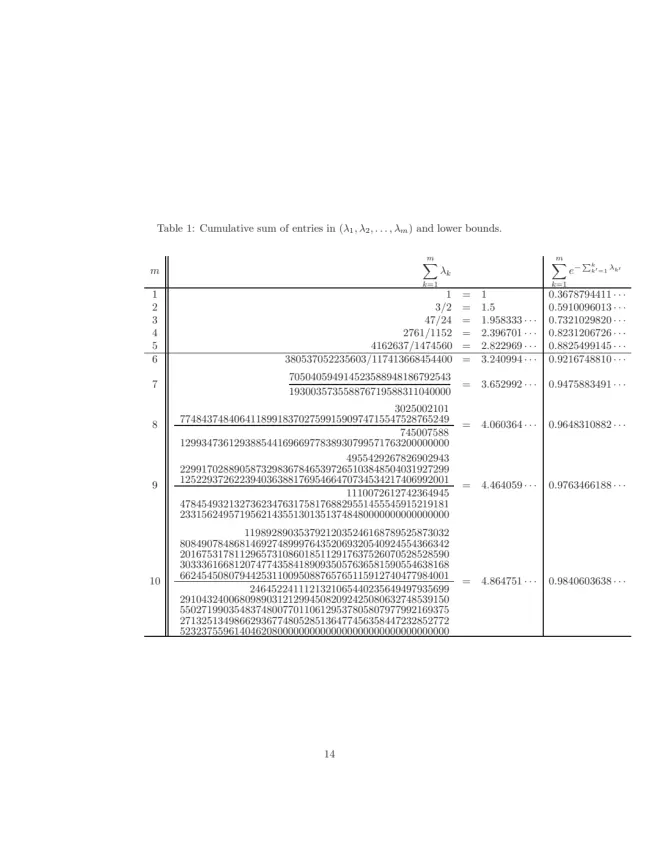
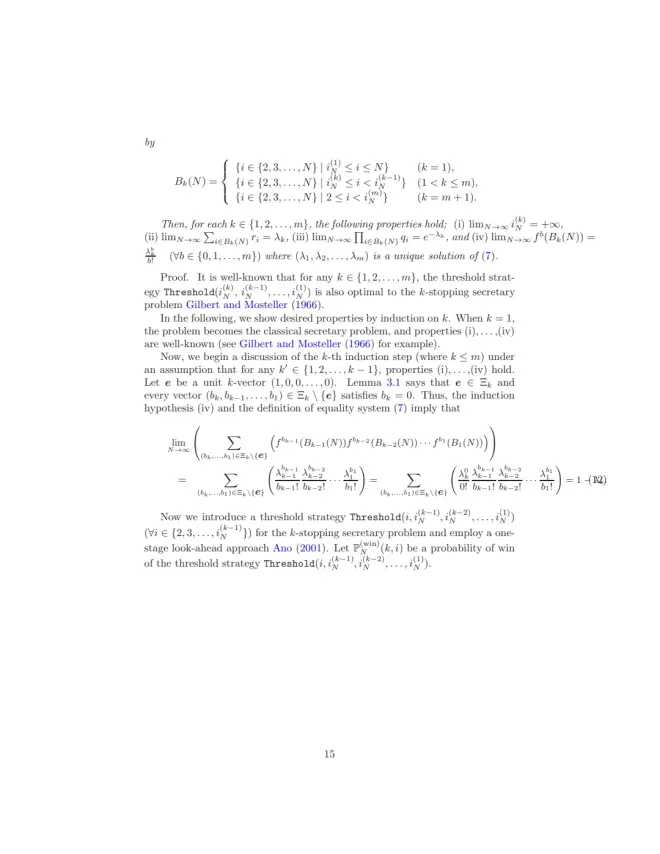
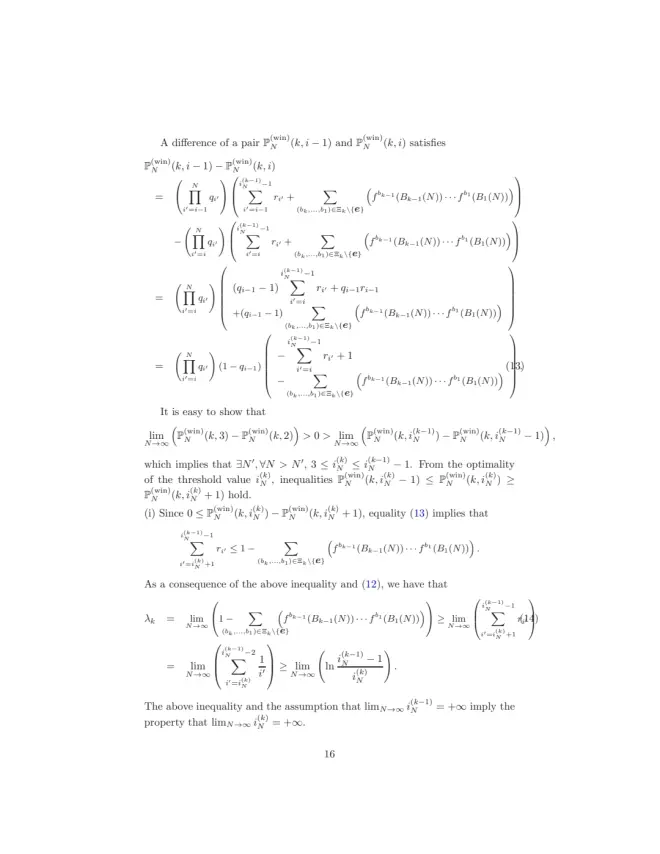
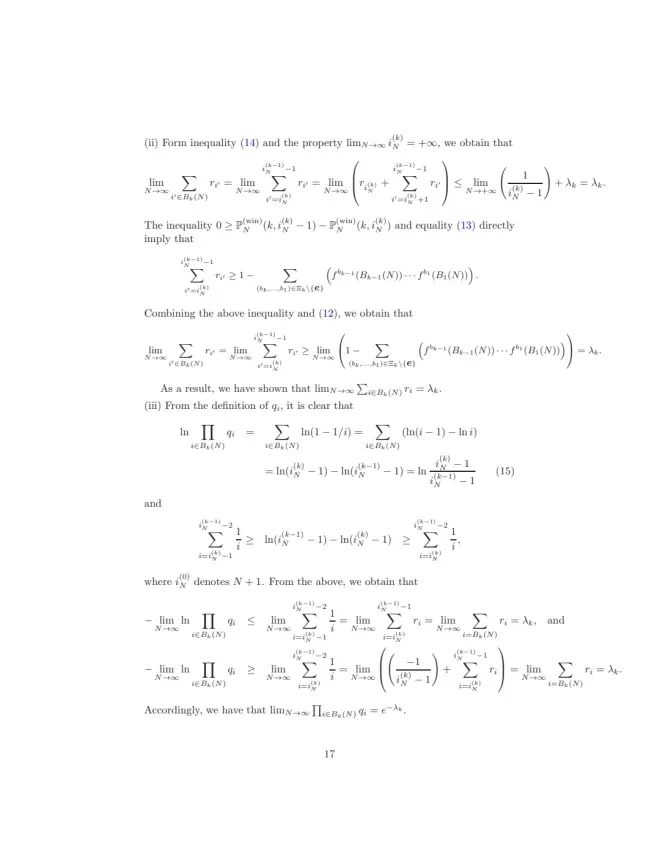
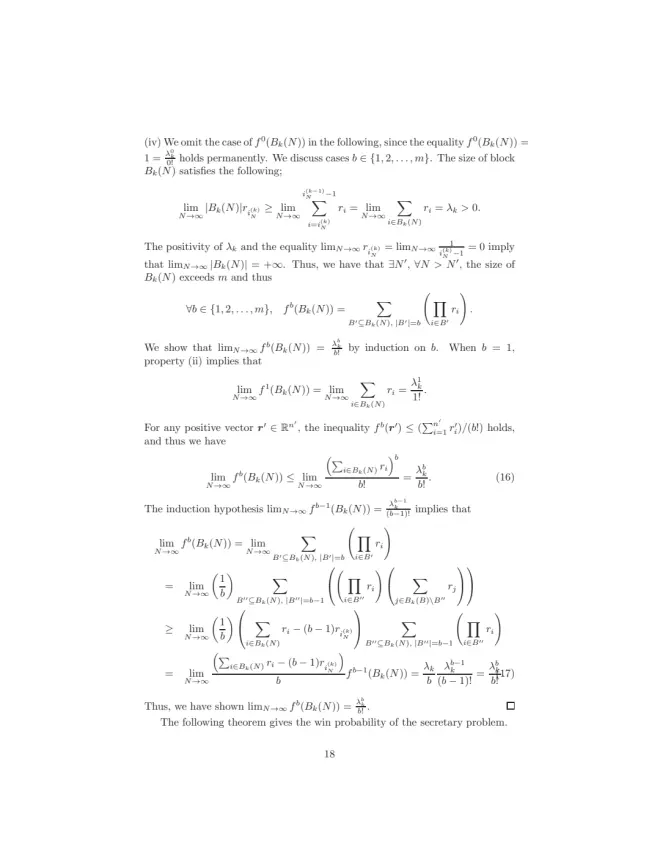
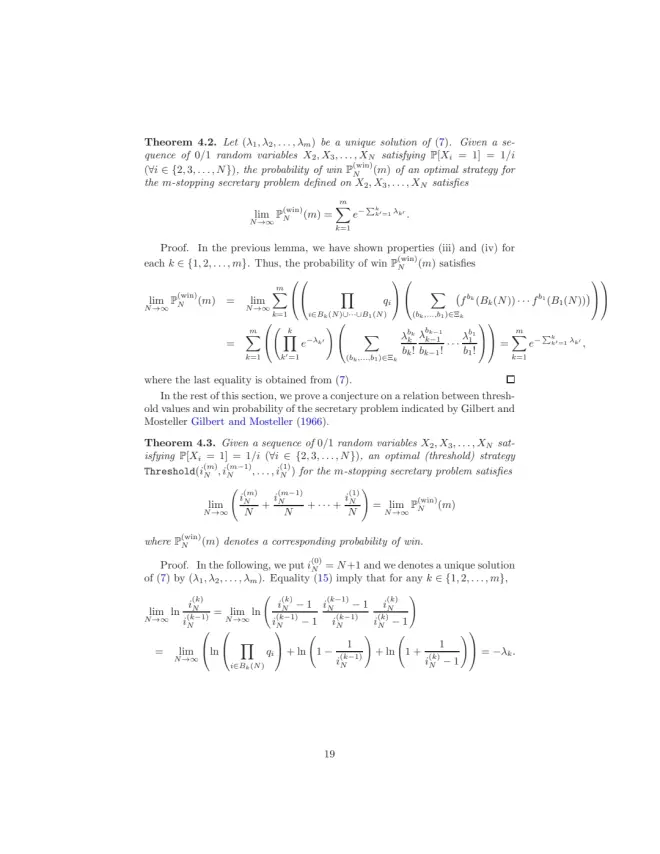
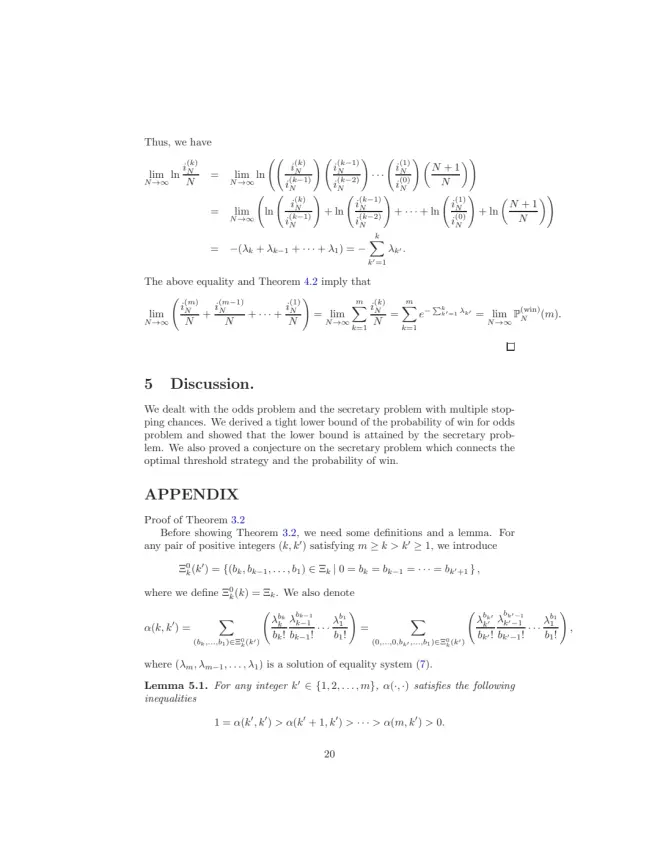
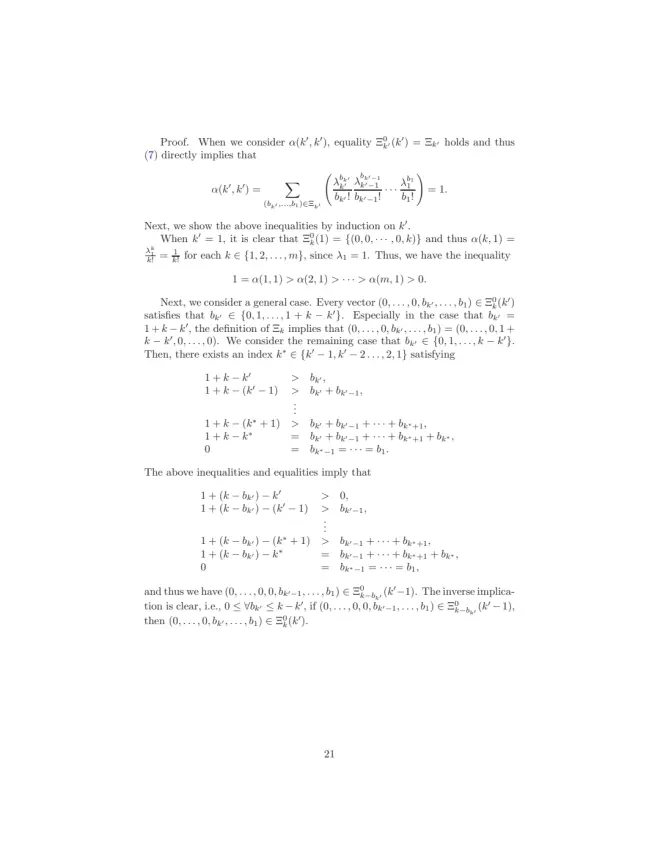
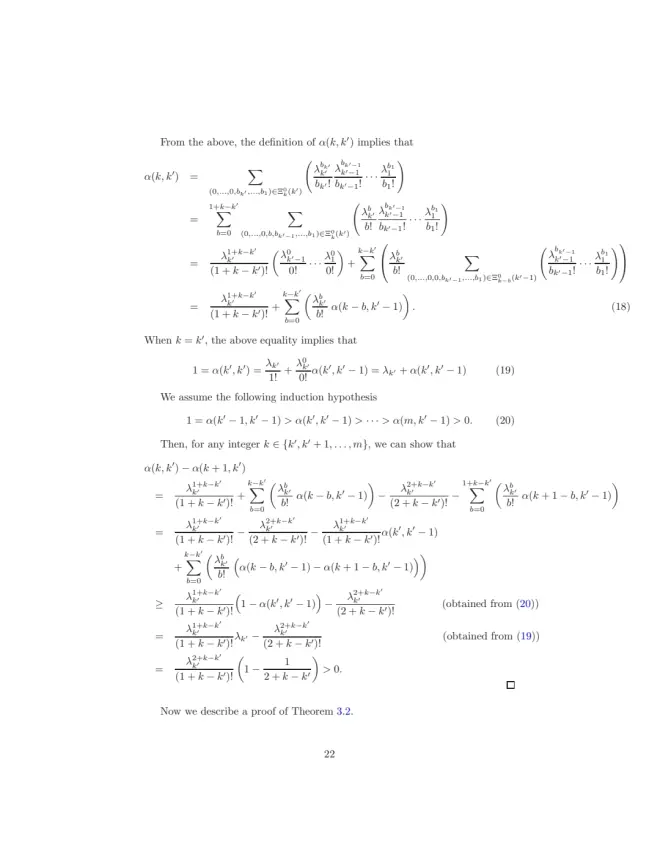
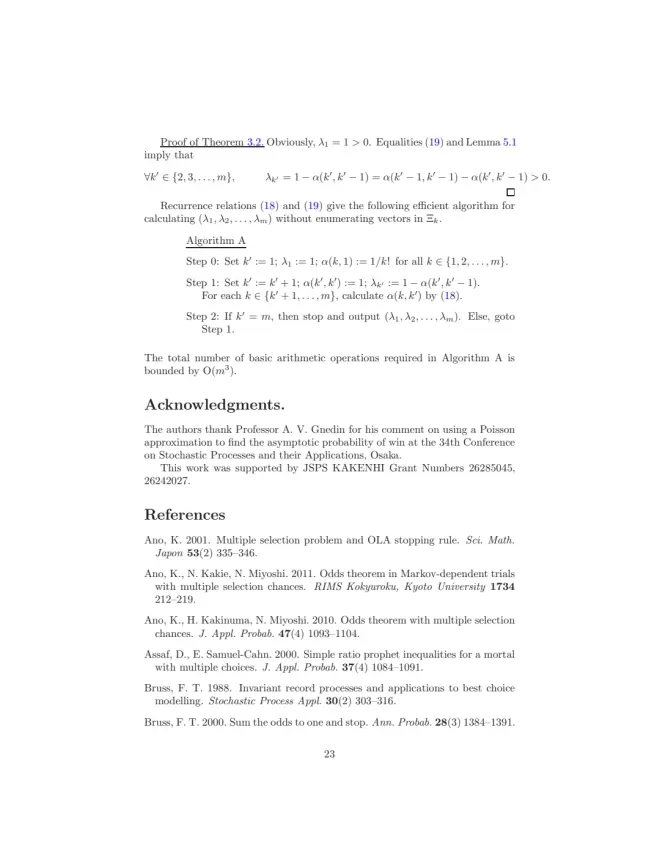
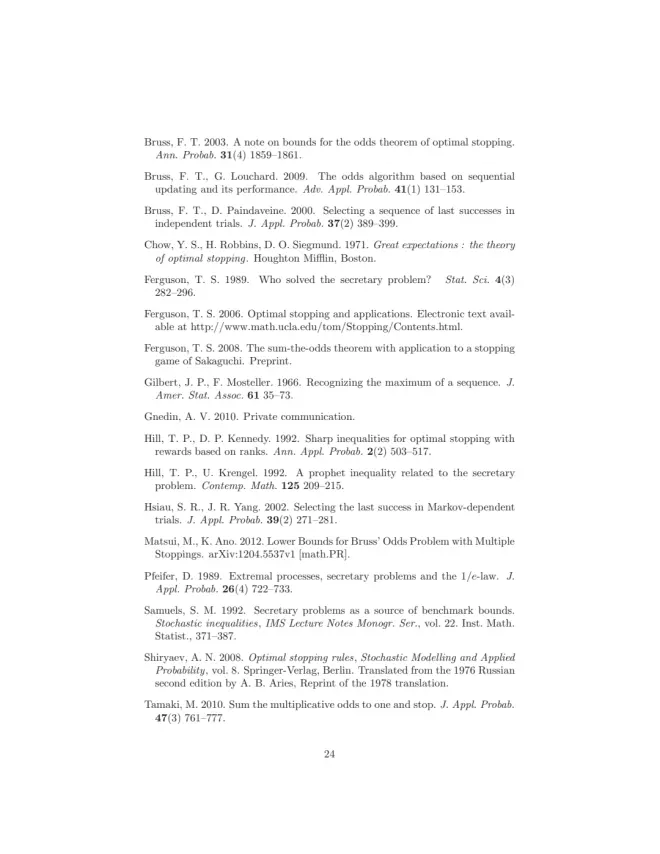
帶拒絕概率p的變體問(wèn)題
https://zlkj.in/files/smith-75.pdf
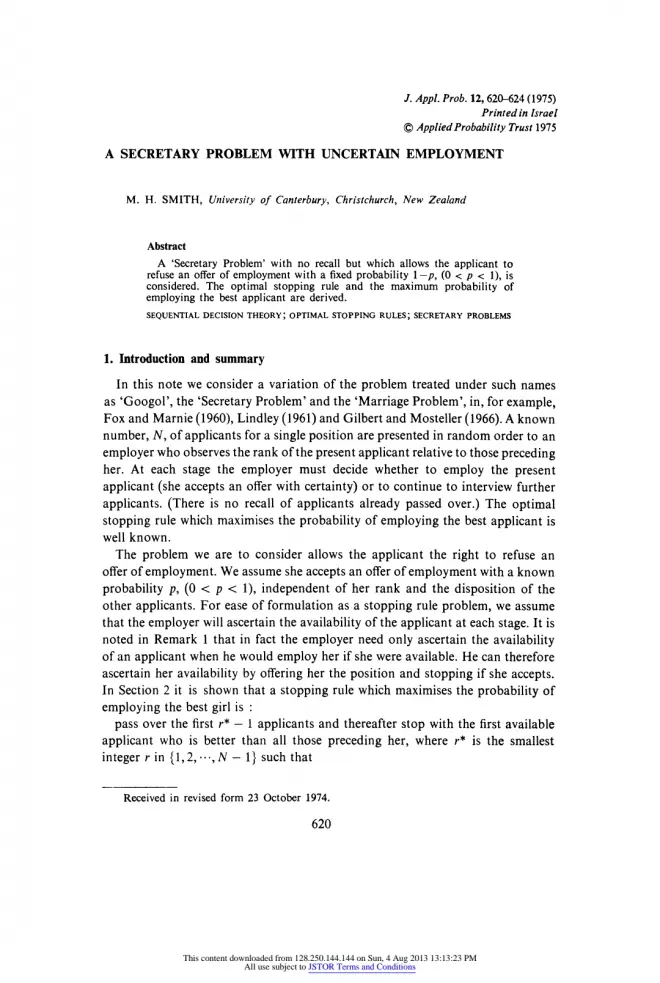
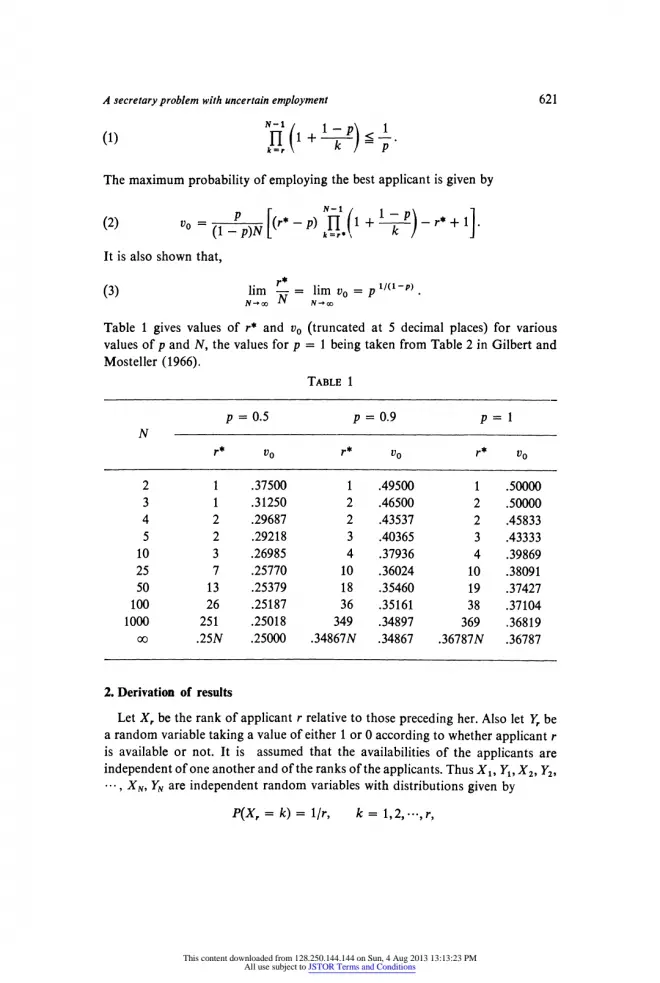
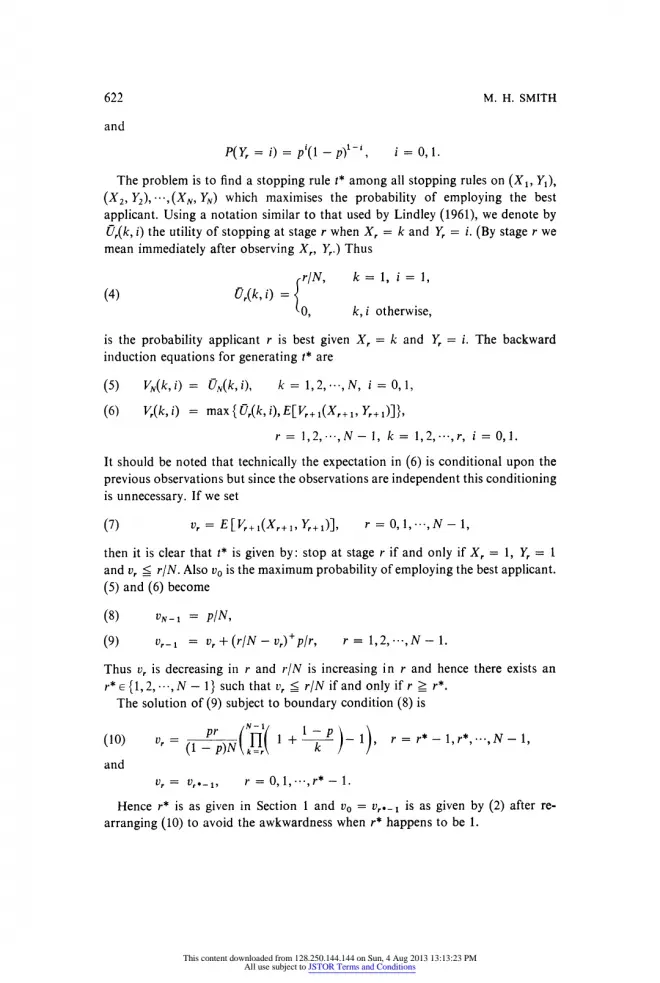
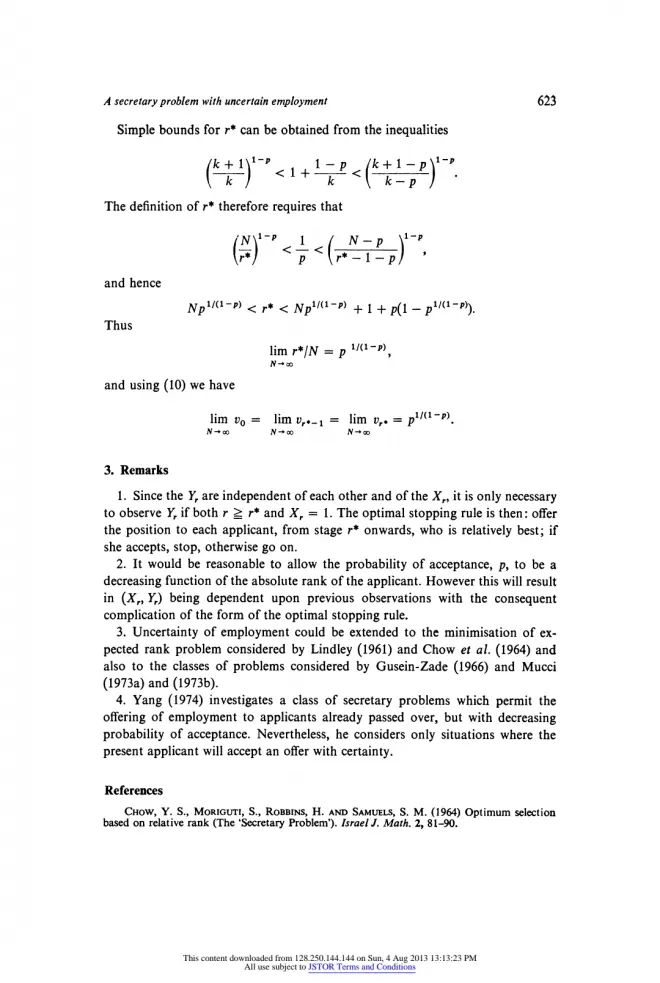
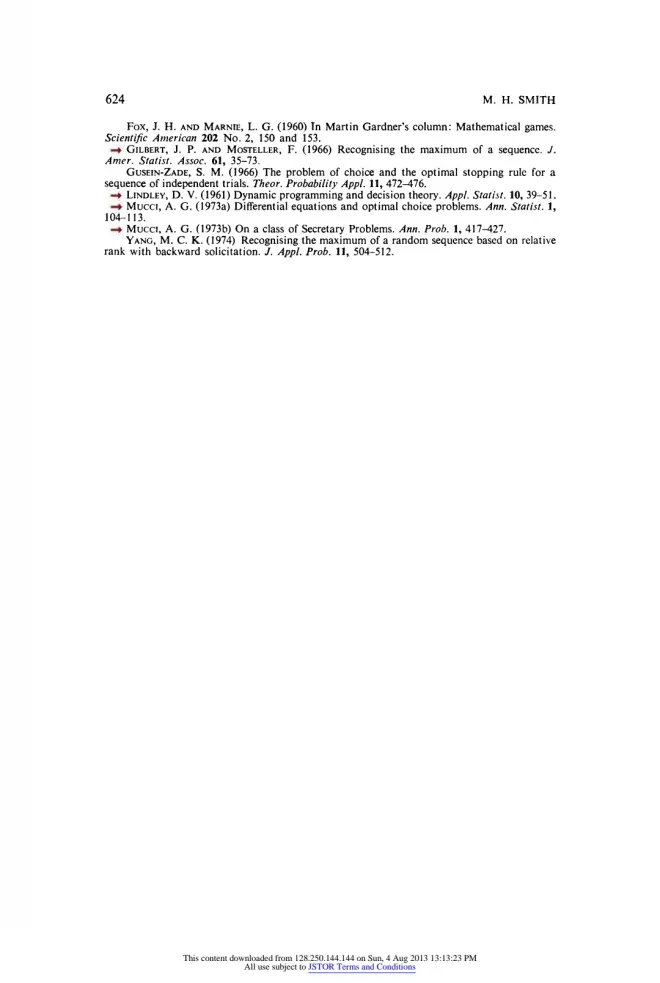