畢業(yè)設(shè)計 多分類與數(shù)據(jù)預測分析
0 前言
?? 這兩年開始畢業(yè)設(shè)計和畢業(yè)答辯的要求和難度不斷提升,傳統(tǒng)的畢設(shè)題目缺少創(chuàng)新和亮點,往往達不到畢業(yè)答辯的要求,這兩年不斷有學弟學妹告訴學長自己做的項目系統(tǒng)達不到老師的要求。
為了大家能夠順利以及最少的精力通過畢設(shè),今天學長分享
?? ?
??學長這里給一個題目綜合評分(每項滿分5分)
難度系數(shù):3分
工作量:3分
創(chuàng)新點:3分
畢設(shè)幫助,選題指導,技術(shù)解答,歡迎打擾,見B站個人主頁
https://space.bilibili.com/33886978
【機器學習】基于邏輯回歸,LightGBM,XGBoost額的分類預測
一.基于邏輯回歸的分類預測
Step1:庫函數(shù)導入
Step2:數(shù)據(jù)讀取/載入
Step3:數(shù)據(jù)信息簡單查看
Step4:可視化描述
Step5:利用 邏輯回歸模型 在二分類上 進行訓練和預測
Step6:利用 邏輯回歸模型 在三分類(多分類)上 進行訓練和預測
Step1:庫函數(shù)導入
Step2:模型訓練
Step3:模型參數(shù)查看
Step4:數(shù)據(jù)和模型可視化
Step5:模型預測
1.1 邏輯回歸的介紹
1.2邏輯回歸的應用
1 邏輯回歸的介紹和應用
2.Demo實踐
3.基于鳶尾花(iris)數(shù)據(jù)集的邏輯回歸分類實踐
二.基于XGBoost的分類預測
Step1:函數(shù)庫導入
Step2:數(shù)據(jù)讀取/載入
Step3:數(shù)據(jù)信息簡單查看
Step4:可視化描述
Step5:對離散變量進行編碼
Step6:利用 XGBoost 進行訓練與預測
Step7: 利用 XGBoost 進行特征選擇
Step8: 通過調(diào)整參數(shù)獲得更好的效果
XGBoost的介紹
1.2XGboost的應用
1.XGBoost與應用
2.基于天氣數(shù)據(jù)集的XGBoost分類實戰(zhàn)
一.基于邏輯回歸的分類預測
1 邏輯回歸的介紹和應用
1.1 邏輯回歸的介紹
邏輯回歸(Logistic regression,簡稱LR)雖然其中帶有"回歸"兩個字,但邏輯回歸其實是一個分類模型,并且廣泛應用于各個領(lǐng)域之中。雖然現(xiàn)在深度學習相對于這些傳統(tǒng)方法更為火熱,但實則這些傳統(tǒng)方法由于其獨特的優(yōu)勢依然廣泛應用于各個領(lǐng)域中。
而對于邏輯回歸而且,最為突出的兩點就是其模型簡單和模型的可解釋性強。
邏輯回歸模型的優(yōu)劣勢:
優(yōu)點:實現(xiàn)簡單,易于理解和實現(xiàn);計算代價不高,速度很快,存儲資源低
缺點:容易欠擬合,分類精度可能不高
1.2邏輯回歸的應用
邏輯回歸模型廣泛用于各個領(lǐng)域,包括機器學習,大多數(shù)醫(yī)學領(lǐng)域和社會科學。例如,最初由Boyd 等人開發(fā)的創(chuàng)傷和損傷嚴重度評分(TRISS)被廣泛用于預測受傷患者的死亡率,使用邏輯回歸 基于觀察到的患者特征(年齡,性別,體重指數(shù),各種血液檢查的結(jié)果等)分析預測發(fā)生特定疾?。ɡ缣悄虿?,冠心?。┑娘L險。邏輯回歸模型也用于預測在給定的過程中,系統(tǒng)或產(chǎn)品的故障的可能性。還用于市場營銷應用程序,例如預測客戶購買產(chǎn)品或中止訂購的傾向等。在經(jīng)濟學中它可以用來預測一個人選擇進入勞動力市場的可能性,而商業(yè)應用則可以用來預測房主拖欠抵押貸款的可能性。條件隨機字段是邏輯回歸到順序數(shù)據(jù)的擴展,用于自然語言處理。
邏輯回歸模型現(xiàn)在同樣是很多分類算法的基礎(chǔ)組件,比如 分類任務中基于GBDT算法+LR邏輯回歸實現(xiàn)的信用卡交易反欺詐,CTR(點擊通過率)預估等,其好處在于輸出值自然地落在0到1之間,并且有概率意義。模型清晰,有對應的概率學理論基礎(chǔ)。它擬合出來的參數(shù)就代表了每一個特征(feature)對結(jié)果的影響。也是一個理解數(shù)據(jù)的好工具。但同時由于其本質(zhì)上是一個線性的分類器,所以不能應對較為復雜的數(shù)據(jù)情況。很多時候我們也會拿邏輯回歸模型去做一些任務嘗試的基線(基礎(chǔ)水平)。
說了這些邏輯回歸的概念和應用,大家應該已經(jīng)對其有所期待了吧,那么我們現(xiàn)在開始吧?。?!
2.Demo實踐
Step1:庫函數(shù)導入
? ?? ?## ?基礎(chǔ)函數(shù)庫 ?? ?import numpy as np ? ? ?
## 導入畫圖庫
import matplotlib.pyplot as plt
import seaborn as sns
## 導入邏輯回歸模型函數(shù)
from sklearn.linear_model import LogisticRegression
Step2:模型訓練
? ?? ?##Demo演示LogisticRegression分類 ?? ?
## 構(gòu)造數(shù)據(jù)集
x_fearures = np.array([[-1, -2], [-2, -1], [-3, -2], [1, 3], [2, 1], [3, 2]])
y_label = np.array([0, 0, 0, 1, 1, 1])
## 調(diào)用邏輯回歸模型
lr_clf = LogisticRegression()
## 用邏輯回歸模型擬合構(gòu)造的數(shù)據(jù)集
lr_clf = lr_clf.fit(x_fearures, y_label) #其擬合方程為 y=w0+w1*x1+w2*x2
Step3:模型參數(shù)查看
? ?? ?## 查看其對應模型的w ?? ?print('the weight of Logistic Regression:',lr_clf.coef_) ?? ?
## 查看其對應模型的w0
print('the intercept(w0) of Logistic Regression:',lr_clf.intercept_)

Step4:數(shù)據(jù)和模型可視化
? ?? ?## 可視化構(gòu)造的數(shù)據(jù)樣本點 ?? ?plt.figure() ?? ?plt.scatter(x_fearures[:,0],x_fearures[:,1], c=y_label, s=50, cmap='viridis') ?? ?plt.title('Dataset') ?? ?plt.show()
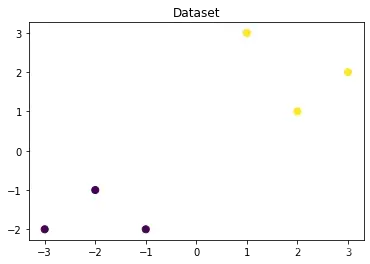
? ?? ?# 可視化決策邊界 ?? ?plt.figure() ?? ?plt.scatter(x_fearures[:,0],x_fearures[:,1], c=y_label, s=50, cmap='viridis') ?? ?plt.title('Dataset') ?? ?
nx, ny = 200, 100
x_min, x_max = plt.xlim()
y_min, y_max = plt.ylim()
x_grid, y_grid = np.meshgrid(np.linspace(x_min, x_max, nx),np.linspace(y_min, y_max, ny))
z_proba = lr_clf.predict_proba(np.c_[x_grid.ravel(), y_grid.ravel()])
z_proba = z_proba[:, 1].reshape(x_grid.shape)
plt.contour(x_grid, y_grid, z_proba, [0.5], linewidths=2., colors='blue')
plt.show()
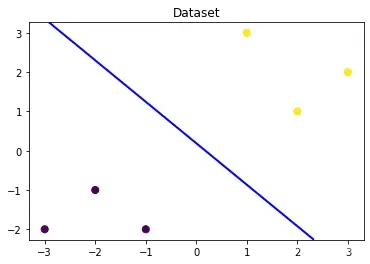
? ?? ?### 可視化預測新樣本 ?? ?
plt.figure()
## new point 1
x_fearures_new1 = np.array([[0, -1]])
plt.scatter(x_fearures_new1[:,0],x_fearures_new1[:,1], s=50, cmap='viridis')
plt.annotate(s='New point 1',xy=(0,-1),xytext=(-2,0),color='blue',arrowprops=dict(arrowstyle='-|>',connectionstyle='arc3',color='red'))
## new point 2
x_fearures_new2 = np.array([[1, 2]])
plt.scatter(x_fearures_new2[:,0],x_fearures_new2[:,1], s=50, cmap='viridis')
plt.annotate(s='New point 2',xy=(1,2),xytext=(-1.5,2.5),color='red',arrowprops=dict(arrowstyle='-|>',connectionstyle='arc3',color='red'))
## 訓練樣本
plt.scatter(x_fearures[:,0],x_fearures[:,1], c=y_label, s=50, cmap='viridis')
plt.title('Dataset')
# 可視化決策邊界
plt.contour(x_grid, y_grid, z_proba, [0.5], linewidths=2., colors='blue')
plt.show()
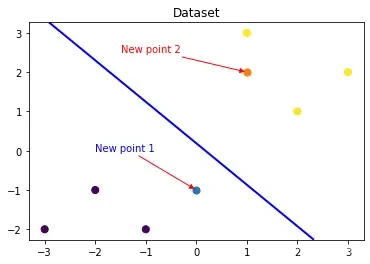
Step5:模型預測
? ?? ?## 在訓練集和測試集上分別利用訓練好的模型進行預測 ?? ?y_label_new1_predict = lr_clf.predict(x_fearures_new1) ?? ?y_label_new2_predict = lr_clf.predict(x_fearures_new2) ?? ?
print('The New point 1 predict class:\n',y_label_new1_predict)
print('The New point 2 predict class:\n',y_label_new2_predict)
## 由于邏輯回歸模型是概率預測模型(前文介紹的 p = p(y=1|x,\theta)),所以我們可以利用 predict_proba 函數(shù)預測其概率
y_label_new1_predict_proba = lr_clf.predict_proba(x_fearures_new1)
y_label_new2_predict_proba = lr_clf.predict_proba(x_fearures_new2)
print('The New point 1 predict Probability of each class:\n',y_label_new1_predict_proba)
print('The New point 2 predict Probability of each class:\n',y_label_new2_predict_proba)
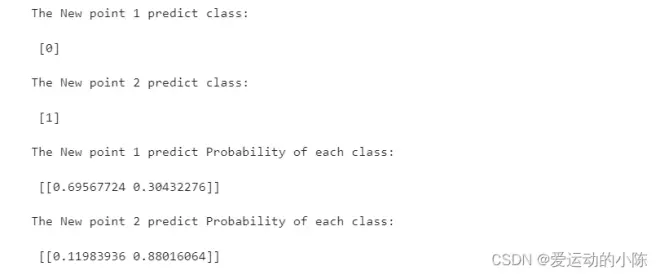
可以發(fā)現(xiàn)訓練好的回歸模型將X_new1預測為了類別0(判別面左下側(cè)),X_new2預測為了類別1(判別面右上側(cè))。其訓練得到的邏輯回歸模型的概率為0.5的判別面為上圖中藍色的線。
3.基于鳶尾花(iris)數(shù)據(jù)集的邏輯回歸分類實踐
本次我們選擇鳶花數(shù)據(jù)(iris)進行方法的嘗試訓練,該數(shù)據(jù)集一共包含5個變量,其中4個特征變量,1個目標分類變量。共有150個樣本,目標變量為 花的類別 其都屬于鳶尾屬下的三個亞屬,分別是山鳶尾 (Iris-setosa),變色鳶尾(Iris-versicolor)和維吉尼亞鳶尾(Iris- virginica)。包含的三種鳶尾花的四個特征,分別是花萼長度(cm)、花萼寬度(cm)、花瓣長度(cm)、花瓣寬度(cm),這些形態(tài)特征在過去被用來識別物種。
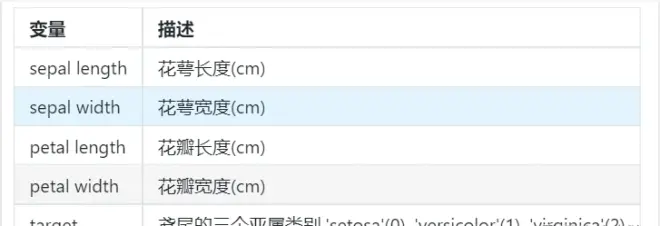
Step1:庫函數(shù)導入
? ?? ?## ?基礎(chǔ)函數(shù)庫 ?? ?import numpy as np ? ? ?import pandas as pd ?? ?
## 繪圖函數(shù)庫
import matplotlib.pyplot as plt
import seaborn as sns
Step2:數(shù)據(jù)讀取/載入
? ?? ?## 我們利用 sklearn 中自帶的 iris 數(shù)據(jù)作為數(shù)據(jù)載入,并利用Pandas轉(zhuǎn)化為DataFrame格式 ?? ?from sklearn.datasets import load_iris ?? ?data = load_iris() #得到數(shù)據(jù)特征 ?? ?iris_target = data.target #得到數(shù)據(jù)對應的標簽 ?? ?iris_features = pd.DataFrame(data=data.data, columns=data.feature_names) #利用Pandas轉(zhuǎn)化為DataFrame格式
Step3:數(shù)據(jù)信息簡單查看
? ?? ?## 利用.info()查看數(shù)據(jù)的整體信息 ?? ?iris_features.info()
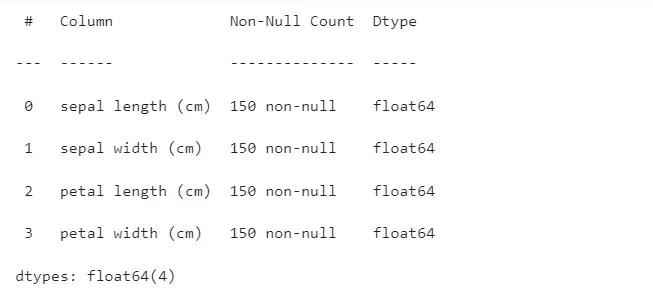
? ?? ? ?? ?## 進行簡單的數(shù)據(jù)查看,我們可以利用 .head() 頭部.tail()尾部 ?? ?iris_features.head()
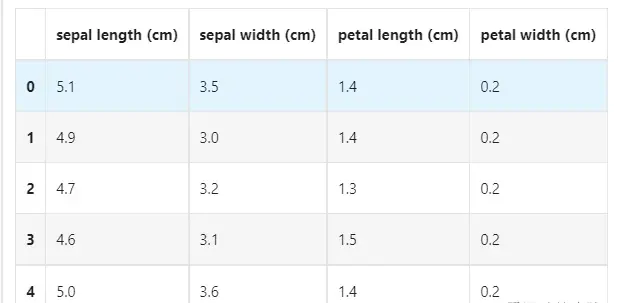
? ?? ? ?? ?## 其對應的類別標簽為,其中0,1,2分別代表'setosa', 'versicolor', 'virginica'三種不同花的類別。 ?? ?iris_target
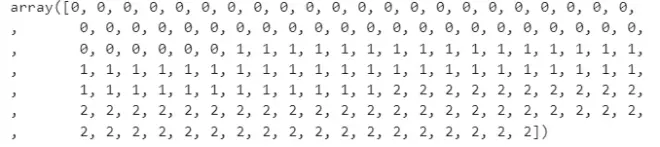
? ?? ?## 利用value_counts函數(shù)查看每個類別數(shù)量 ?? ?pd.Series(iris_target).value_counts()
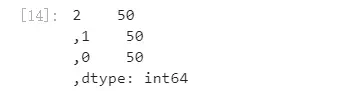
? ?? ?## 對于特征進行一些統(tǒng)計描述 ?? ?iris_features.describe()
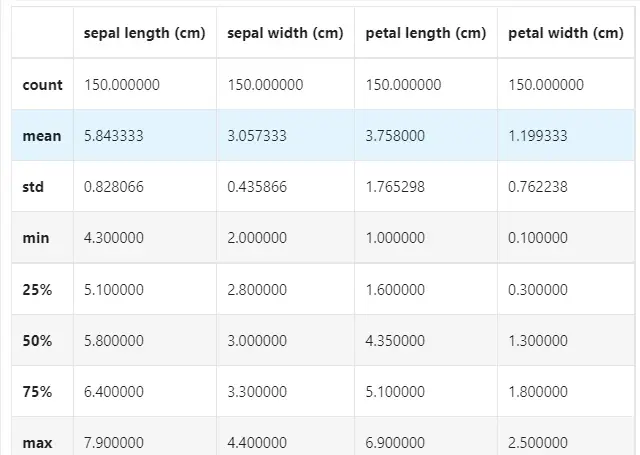
從統(tǒng)計描述中我們可以看到不同數(shù)值特征的變化范圍。
Step4:可視化描述
? ?? ?## 合并標簽和特征信息 ?? ?iris_all = iris_features.copy() ##進行淺拷貝,防止對于原始數(shù)據(jù)的修改 ?? ?iris_all['target'] = iris_target ?? ?## 特征與標簽組合的散點可視化 ?? ?sns.pairplot(data=iris_all,diag_kind='hist', hue= 'target') ?? ?plt.show()
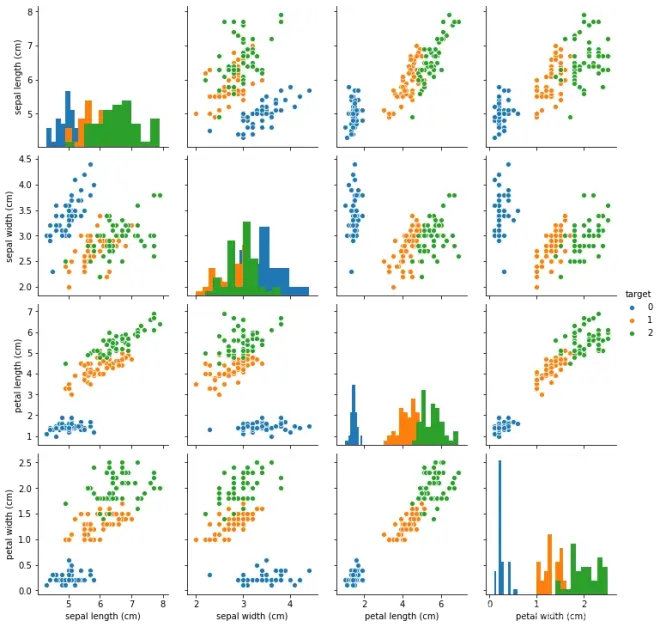
上圖可以發(fā)現(xiàn),在2D情況下不同的特征組合對于不同類別的花的散點分布,以及大概的區(qū)分能力。
? ?? ?for col in iris_features.columns: ?? ? ? ?sns.boxplot(x='target', y=col, saturation=0.5,palette='pastel', data=iris_all) ?? ? ? ?plt.title(col) ?? ? ? ?plt.show()
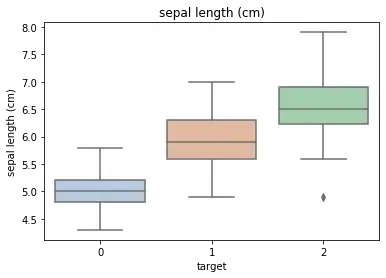
?
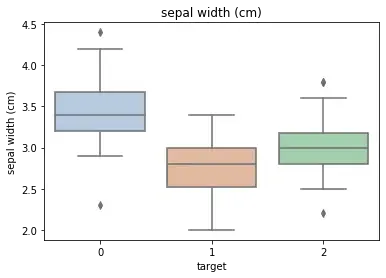
?
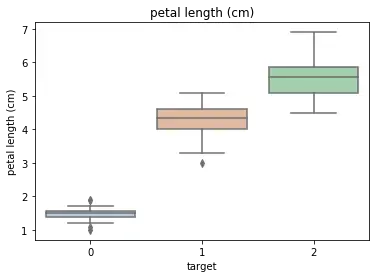
?
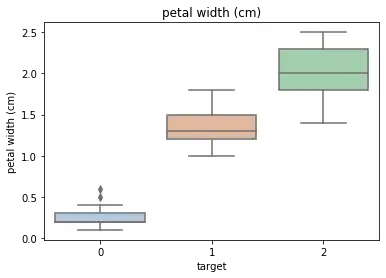
? 利用箱型圖我們也可以得到不同類別在不同特征上的分布差異情況。
? ?? ?# 選取其前三個特征繪制三維散點圖 ?? ?from mpl_toolkits.mplot3d import Axes3D ?? ?
fig = plt.figure(figsize=(10,8))
ax = fig.add_subplot(111, projection='3d')
iris_all_class0 = iris_all[iris_all['target']==0].values
iris_all_class1 = iris_all[iris_all['target']==1].values
iris_all_class2 = iris_all[iris_all['target']==2].values
# 'setosa'(0), 'versicolor'(1), 'virginica'(2)
ax.scatter(iris_all_class0[:,0], iris_all_class0[:,1], iris_all_class0[:,2],label='setosa')
ax.scatter(iris_all_class1[:,0], iris_all_class1[:,1], iris_all_class1[:,2],label='versicolor')
ax.scatter(iris_all_class2[:,0], iris_all_class2[:,1], iris_all_class2[:,2],label='virginica')
plt.legend()
plt.show()
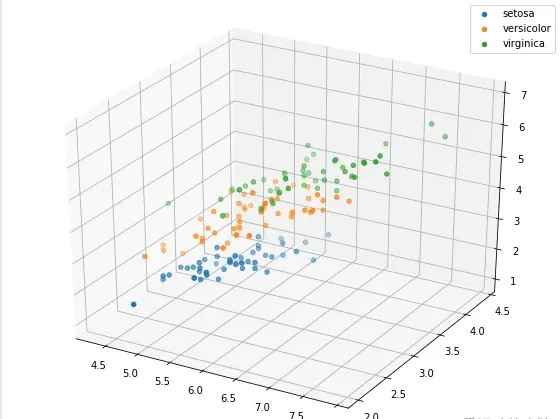
Step5:利用 邏輯回歸模型 在二分類上 進行訓練和預測
? ?? ?## 為了正確評估模型性能,將數(shù)據(jù)劃分為訓練集和測試集,并在訓練集上訓練模型,在測試集上驗證模型性能。 ?? ?from sklearn.model_selection import train_test_split ?? ?
## 選擇其類別為0和1的樣本 (不包括類別為2的樣本)
iris_features_part = iris_features.iloc[:100]
iris_target_part = iris_target[:100]
## 測試集大小為20%, 80%/20%分
x_train, x_test, y_train, y_test = train_test_split(iris_features_part, iris_target_part, test_size = 0.2, random_state = 2020)
## 從sklearn中導入邏輯回歸模型
from sklearn.linear_model import LogisticRegression
## 定義 邏輯回歸模型
clf = LogisticRegression(random_state=0, solver='lbfgs')
# 在訓練集上訓練邏輯回歸模型
clf.fit(x_train, y_train)
## 查看其對應的w
print('the weight of Logistic Regression:',clf.coef_)
## 查看其對應的w0
print('the intercept(w0) of Logistic Regression:',clf.intercept_)

? ?? ?## 在訓練集和測試集上分布利用訓練好的模型進行預測 ?? ?train_predict = clf.predict(x_train) ?? ?test_predict = clf.predict(x_test)
? ?? ? ?? ?from sklearn import metrics ?? ?
## 利用accuracy(準確度)【預測正確的樣本數(shù)目占總預測樣本數(shù)目的比例】評估模型效果
print('The accuracy of the Logistic Regression is:',metrics.accuracy_score(y_train,train_predict))
print('The accuracy of the Logistic Regression is:',metrics.accuracy_score(y_test,test_predict))
## 查看混淆矩陣 (預測值和真實值的各類情況統(tǒng)計矩陣)
confusion_matrix_result = metrics.confusion_matrix(test_predict,y_test)
print('The confusion matrix result:\n',confusion_matrix_result)
# 利用熱力圖對于結(jié)果進行可視化
plt.figure(figsize=(8, 6))
sns.heatmap(confusion_matrix_result, annot=True, cmap='Blues')
plt.xlabel('Predicted labels')
plt.ylabel('True labels')
plt.show()
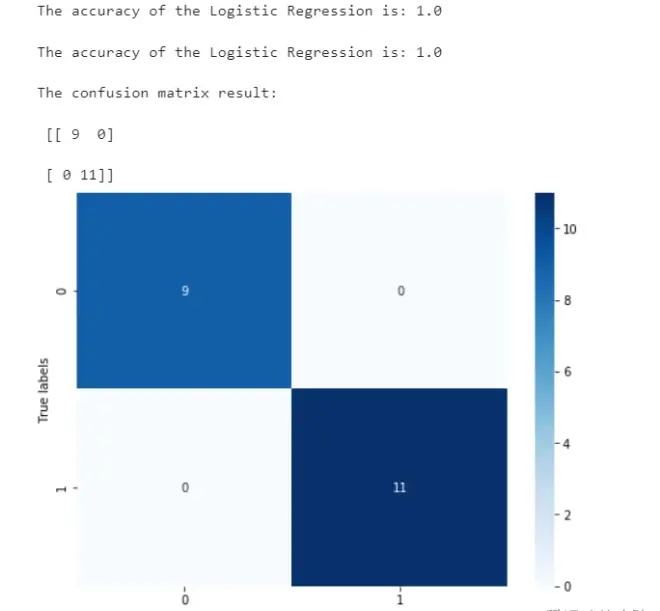
我們可以發(fā)現(xiàn)其準確度為1,代表所有的樣本都預測正確了。
Step6:利用 邏輯回歸模型 在三分類(多分類)上 進行訓練和預測
? ?? ?## 測試集大小為20%, 80%/20%分 ?? ?x_train, x_test, y_train, y_test = train_test_split(iris_features, iris_target, test_size = 0.2, random_state = 2020)
其余代碼與二分類相同
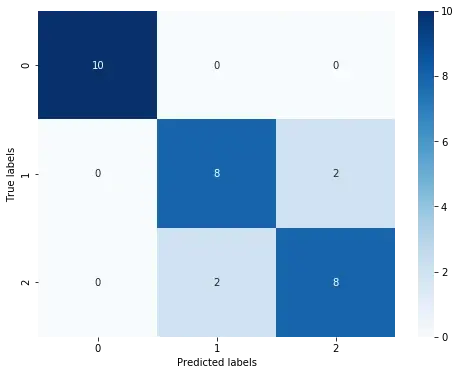
?
通過結(jié)果我們可以發(fā)現(xiàn),其在三分類的結(jié)果的預測準確度上有所下降,其在測試集上的準確度為: ? 86.67 ? % ? ,這是由于’versicolor’(1)和 ‘virginica’(2)這兩個類別的特征,我們從可視化的時候也可以發(fā)現(xiàn),其特征的邊界具有一定的模糊性(邊界類別混雜,沒有明顯區(qū)分邊界),所有在這兩類的預測上出現(xiàn)了一定的錯誤。
二.基于XGBoost的分類預測
1.XGBoost與應用
XGBoost的介紹
XGBoost是2016年由華盛頓大學陳天奇老師帶領(lǐng)開發(fā)的一個可擴展機器學習系統(tǒng)。嚴格意義上講XGBoost并不是一種模型,而是一個可供用戶輕松解決分類、回歸或排序問題的軟件包。它內(nèi)部實現(xiàn)了梯度提升樹(GBDT)模型,并對模型中的算法進行了諸多優(yōu)化,在取得高精度的同時又保持了極快的速度,在一段時間內(nèi)成為了國內(nèi)外數(shù)據(jù)挖掘、機器學習領(lǐng)域中的大規(guī)模殺傷性武器。
更重要的是,XGBoost在系統(tǒng)優(yōu)化和機器學習原理方面都進行了深入的考慮。毫不夸張的講,XGBoost提供的可擴展性,可移植性與準確性推動了機器學習計算限制的上限,該系統(tǒng)在單臺機器上運行速度比當時流行解決方案快十倍以上,甚至在分布式系統(tǒng)中可以處理十億級的數(shù)據(jù)。
XGBoost的主要優(yōu)點:
簡單易用。相對其他機器學習庫,用戶可以輕松使用XGBoost并獲得相當不錯的效果。
高效可擴展。在處理大規(guī)模數(shù)據(jù)集時速度快效果好,對內(nèi)存等硬件資源要求不高。
魯棒性強。相對于深度學習模型不需要精細調(diào)參便能取得接近的效果。
XGBoost內(nèi)部實現(xiàn)提升樹模型,可以 自動處理缺失值 。
XGBoost的主要缺點:
相對于深度學習模型無法對時空位置建模,不能很好地捕獲圖像、語音、文本等高維數(shù)據(jù)。
在擁有海量訓練數(shù)據(jù),并能找到合適的深度學習模型時,深度學習的精度可以遙遙領(lǐng)先XGBoost。
1.2XGboost的應用
XGBoost在機器學習與數(shù)據(jù)挖掘領(lǐng)域有著極為廣泛的應用。據(jù)統(tǒng)計在2015年Kaggle平臺上29個獲獎方案中,17只隊伍使用了XGBoost;在2015年KDD- Cup中,前十名的隊伍均使用了XGBoost,且集成其他模型比不上調(diào)節(jié)XGBoost的參數(shù)所帶來的提升。這些實實在在的例子都表明,XGBoost在各種問題上都可以取得非常好的效果。
同時,XGBoost還被成功應用在工業(yè)界與學術(shù)界的各種問題中。例如商店銷售額預測、高能物理事件分類、web文本分類;用戶行為預測、運動檢測、廣告點擊率預測、惡意軟件分類、災害風險預測、在線課程退學率預測。雖然領(lǐng)域相關(guān)的數(shù)據(jù)分析和特性工程在這些解決方案
2.基于天氣數(shù)據(jù)集的XGBoost分類實戰(zhàn)
數(shù)據(jù)集:
Step1:函數(shù)庫導入
? ?? ?## ?基礎(chǔ)函數(shù)庫 ?? ?import numpy as np ? ? ?import pandas as pd ?? ?
## 繪圖函數(shù)庫
import matplotlib.pyplot as plt
import seaborn as sns
本次我們選擇天氣數(shù)據(jù)集進行方法的嘗試訓練,現(xiàn)在有一些由氣象站提供的每日降雨數(shù)據(jù),我們需要根據(jù)歷史降雨數(shù)據(jù)來預測明天會下雨的概率。樣例涉及到的測試集數(shù)據(jù)test.csv與train.csv的格式完全相同,但其RainTomorrow未給出,為預測變量。
數(shù)據(jù)的各個特征描述如下:
Step2:數(shù)據(jù)讀取/載入
? ?? ?## 我們利用Pandas自帶的read_csv函數(shù)讀取并轉(zhuǎn)化為DataFrame格式 ?? ?
data = pd.read_csv('train.csv')
Step3:數(shù)據(jù)信息簡單查看
? ?? ?## 利用.info()查看數(shù)據(jù)的整體信息 ?? ?data.info()
? ?? ? ?? ?## 進行簡單的數(shù)據(jù)查看,我們可以利用 .head() 頭部.tail()尾部 ?? ?data.head()
由于變量太多,這里只展示部分變量
這里我們發(fā)現(xiàn)數(shù)據(jù)集中存在NaN,一般的我們認為NaN在數(shù)據(jù)集中代表了缺失值,可能是數(shù)據(jù)采集或處理時產(chǎn)生的一種錯誤。這里我們采用-1將缺失值進行填補,還有其他例如“中位數(shù)填補、平均數(shù)填補”的缺失值處理方法有興趣的可以查看我的另一篇博客:
,這里詳細的解釋的數(shù)據(jù)預處理的基本操作.? ?? ?data = data.fillna(-1) ?? ?data.tail()
? ?? ? ?? ?## 利用value_counts函數(shù)查看訓練集標簽的數(shù)量 ?? ?pd.Series(data['RainTomorrow']).value_counts()
No 82786 ? ,Yes 23858 ? ,Name: RainTomorrow, dtype: int64
我們發(fā)現(xiàn)數(shù)據(jù)集中的負樣本數(shù)量遠大于正樣本數(shù)量,這種常見的問題叫做“數(shù)據(jù)不平衡”問題,在某些情況下需要進行一些特殊處理。解決數(shù)據(jù)不平衡的辦法有數(shù)據(jù)變換或者數(shù)據(jù)插補等等。
? ?? ?## 對于特征進行一些統(tǒng)計描述 ?? ?data.describe()
Step4:可視化描述
## 合并標簽和特征信息 ? ?iris_all = iris_features.copy() ##進行淺拷貝,防止對于原始數(shù)據(jù)的修改 ? ?iris_all['target'] = iris_target ? ?## 特征與標簽組合的散點可視化 ? ?sns.pairplot(data=iris_all,diag_kind='hist', hue= 'target') ? ?plt.show()
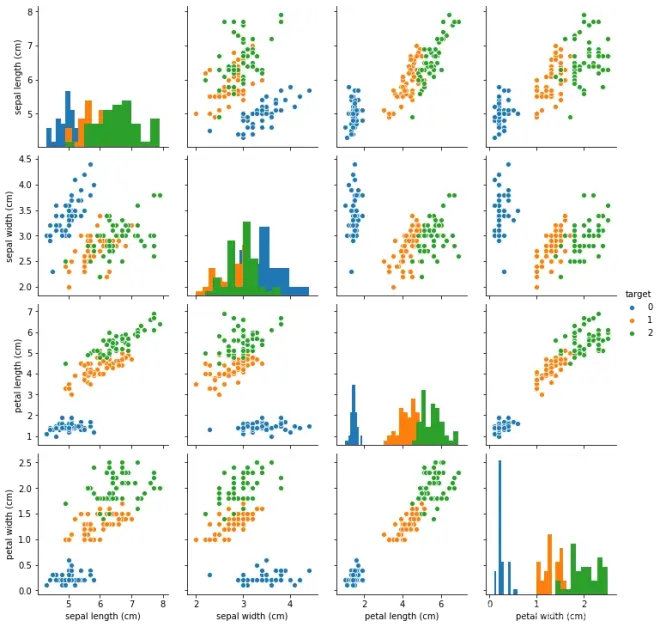
上圖可以發(fā)現(xiàn),在2D情況下不同的特征組合對于不同類別的花的散點分布,以及大概的區(qū)分能力。
? ? ? ?for col in iris_features.columns: ? ? ? ?sns.boxplot(x='target', y=col, saturation=0.5,palette='pastel', data=iris_all) ? ? ? ?plt.title(col) ? ? ? ?plt.show()
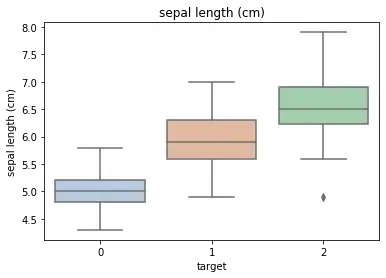
?
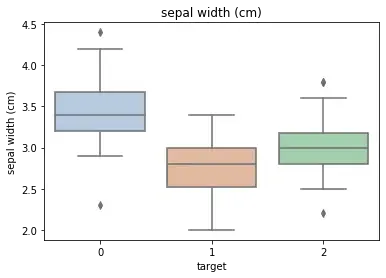
?
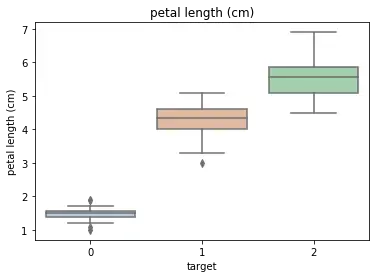
?
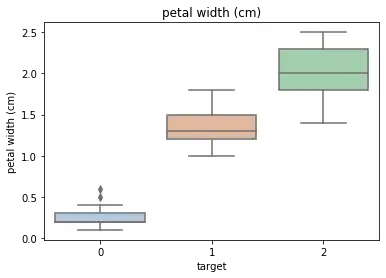
?利用箱型圖我們也可以得到不同類別在不同特征上的分布差異情況。
# 選取其前三個特征繪制三維散點圖 ? ?from mpl_toolkits.mplot3d import Axes3D ? ?
fig = plt.figure(figsize=(10,8))
ax = fig.add_subplot(111, projection='3d')
iris_all_class0 = iris_all[iris_all['target']==0].values
iris_all_class1 = iris_all[iris_all['target']==1].values
iris_all_class2 = iris_all[iris_all['target']==2].values
# 'setosa'(0), 'versicolor'(1), 'virginica'(2)
ax.scatter(iris_all_class0[:,0], iris_all_class0[:,1], iris_all_class0[:,2],label='setosa')
ax.scatter(iris_all_class1[:,0], iris_all_class1[:,1], iris_all_class1[:,2],label='versicolor')
ax.scatter(iris_all_class2[:,0], iris_all_class2[:,1], iris_all_class2[:,2],label='virginica')
plt.legend()
plt.show()
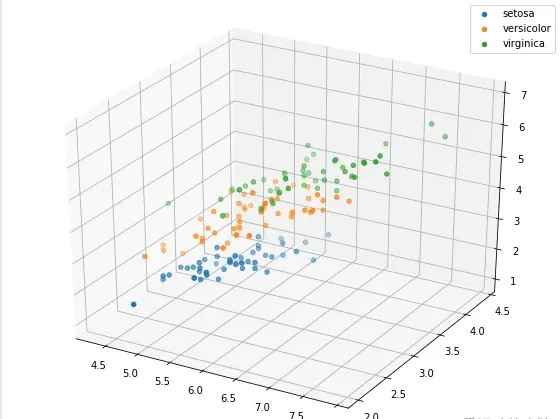
?
Step5:利用 邏輯回歸模型 在二分類上 進行訓練和預測
? ? ?## 為了正確評估模型性能,將數(shù)據(jù)劃分為訓練集和測試集,并在訓練集上訓練模型,在測試集上驗證模型性能。 ? ?from sklearn.model_selection import train_test_split ? ?
## 選擇其類別為0和1的樣本 (不包括類別為2的樣本)
iris_features_part = iris_features.iloc[:100]
iris_target_part = iris_target[:100]
## 測試集大小為20%, 80%/20%分
x_train, x_test, y_train, y_test = train_test_split(iris_features_part, iris_target_part, test_size = 0.2, random_state = 2020)
## 從sklearn中導入邏輯回歸模型
from sklearn.linear_model import LogisticRegression
## 定義 邏輯回歸模型
clf = LogisticRegression(random_state=0, solver='lbfgs')
# 在訓練集上訓練邏輯回歸模型
clf.fit(x_train, y_train)
## 查看其對應的w
print('the weight of Logistic Regression:',clf.coef_)
## 查看其對應的w0
print('the intercept(w0) of Logistic Regression:',clf.intercept_)

? ? ?## 在訓練集和測試集上分布利用訓練好的模型進行預測 ? ?train_predict = clf.predict(x_train) ? ?test_predict = clf.predict(x_test)
? ? ? ? ?from sklearn import metrics ? ?
## 利用accuracy(準確度)【預測正確的樣本數(shù)目占總預測樣本數(shù)目的比例】評估模型效果
print('The accuracy of the Logistic Regression is:',metrics.accuracy_score(y_train,train_predict))
print('The accuracy of the Logistic Regression is:',metrics.accuracy_score(y_test,test_predict))
## 查看混淆矩陣 (預測值和真實值的各類情況統(tǒng)計矩陣)
confusion_matrix_result = metrics.confusion_matrix(test_predict,y_test)
print('The confusion matrix result:\n',confusion_matrix_result)
# 利用熱力圖對于結(jié)果進行可視化
plt.figure(figsize=(8, 6))
sns.heatmap(confusion_matrix_result, annot=True, cmap='Blues')
plt.xlabel('Predicted labels')
plt.ylabel('True labels')
plt.show()
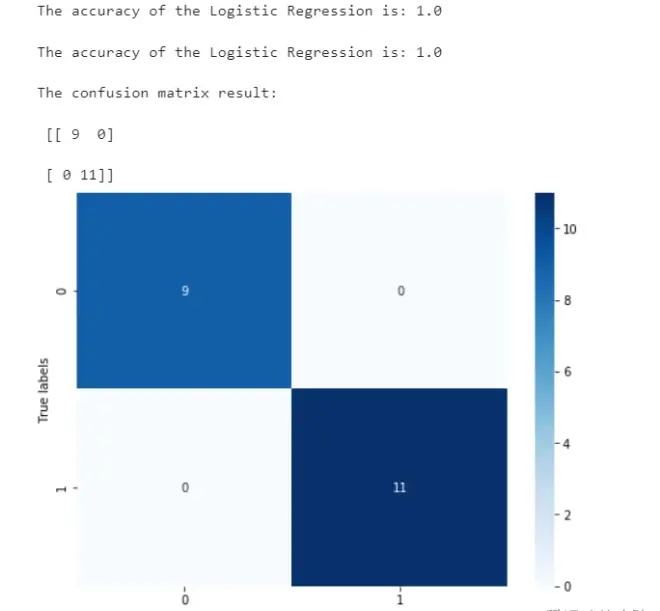
我們可以發(fā)現(xiàn)其準確度為1,代表所有的樣本都預測正確了。
? ? ? ?## 測試集大小為20%, 80%/20%分 ? ?x_train, x_test, y_train, y_test = train_test_split(iris_features, iris_target, test_size = 0.2, random_state = 2020)
其余代碼與二分類相同
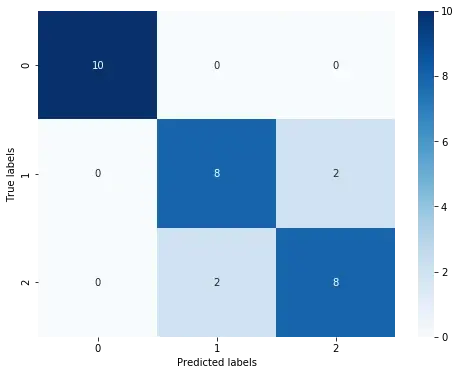
?

通過結(jié)果我們可以發(fā)現(xiàn),其在三分類的結(jié)果的預測準確度上有所下降,其在測試集上的準確度為: ?86.67 ?% ?,這是由于’versicolor’(1)和‘virginica’(2)這兩個類別的特征,我們從可視化的時候也可以發(fā)現(xiàn),其特征的邊界具有一定的模糊性(邊界類別混雜,沒有明顯區(qū)分邊界),所有在這兩類的預測上出現(xiàn)了一定的錯誤。
1.XGBoost與應用
XGBoost的介紹
XGBoost是2016年由華盛頓大學陳天奇老師帶領(lǐng)開發(fā)的一個可擴展機器學習系統(tǒng)。嚴格意義上講XGBoost并不是一種模型,而是一個可供用戶輕松解決分類、回歸或排序問題的軟件包。它內(nèi)部實現(xiàn)了梯度提升樹(GBDT)模型,并對模型中的算法進行了諸多優(yōu)化,在取得高精度的同時又保持了極快的速度,在一段時間內(nèi)成為了國內(nèi)外數(shù)據(jù)挖掘、機器學習領(lǐng)域中的大規(guī)模殺傷性武器。
更重要的是,XGBoost在系統(tǒng)優(yōu)化和機器學習原理方面都進行了深入的考慮。毫不夸張的講,XGBoost提供的可擴展性,可移植性與準確性推動了機器學習計算限制的上限,該系統(tǒng)在單臺機器上運行速度比當時流行解決方案快十倍以上,甚至在分布式系統(tǒng)中可以處理十億級的數(shù)據(jù)。
XGBoost的主要優(yōu)點:
簡單易用。相對其他機器學習庫,用戶可以輕松使用XGBoost并獲得相當不錯的效果。
高效可擴展。在處理大規(guī)模數(shù)據(jù)集時速度快效果好,對內(nèi)存等硬件資源要求不高。
魯棒性強。相對于深度學習模型不需要精細調(diào)參便能取得接近的效果。
XGBoost內(nèi)部實現(xiàn)提升樹模型,可以 自動處理缺失值 。
XGBoost的主要缺點:
相對于深度學習模型無法對時空位置建模,不能很好地捕獲圖像、語音、文本等高維數(shù)據(jù)。
在擁有海量訓練數(shù)據(jù),并能找到合適的深度學習模型時,深度學習的精度可以遙遙領(lǐng)先XGBoost。
1.2XGboost的應用
XGBoost在機器學習與數(shù)據(jù)挖掘領(lǐng)域有著極為廣泛的應用。據(jù)統(tǒng)計在2015年Kaggle平臺上29個獲獎方案中,17只隊伍使用了XGBoost;在2015年KDD- Cup中,前十名的隊伍均使用了XGBoost,且集成其他模型比不上調(diào)節(jié)XGBoost的參數(shù)所帶來的提升。這些實實在在的例子都表明,XGBoost在各種問題上都可以取得非常好的效果。
同時,XGBoost還被成功應用在工業(yè)界與學術(shù)界的各種問題中。例如商店銷售額預測、高能物理事件分類、web文本分類;用戶行為預測、運動檢測、廣告點擊率預測、惡意軟件分類、災害風險預測、在線課程退學率預測。雖然領(lǐng)域相關(guān)的數(shù)據(jù)分析和特性工程在這些解決方案
2.基于天氣數(shù)據(jù)集的XGBoost分類實戰(zhàn)
數(shù)據(jù)集:
Step1:函數(shù)庫導入
? ? ?## ?基礎(chǔ)函數(shù)庫 ? ?import numpy as np ? ?import pandas as pd ? ?
## 繪圖函數(shù)庫
import matplotlib.pyplot as plt
import seaborn as sns
本次我們選擇天氣數(shù)據(jù)集進行方法的嘗試訓練,現(xiàn)在有一些由氣象站提供的每日降雨數(shù)據(jù),我們需要根據(jù)歷史降雨數(shù)據(jù)來預測明天會下雨的概率。樣例涉及到的測試集數(shù)據(jù)test.csv與train.csv的格式完全相同,但其RainTomorrow未給出,為預測變量。
數(shù)據(jù)的各個特征描述如下:
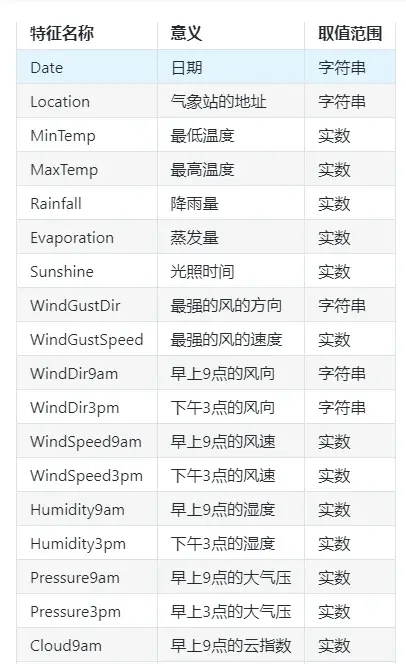
Step2:數(shù)據(jù)讀取/載入
? ? ?## 我們利用Pandas自帶的read_csv函數(shù)讀取并轉(zhuǎn)化為DataFrame格式 ? ?
data = pd.read_csv('train.csv')
Step3:數(shù)據(jù)信息簡單查看
? ? ?## 利用.info()查看數(shù)據(jù)的整體信息 ? ?data.info()
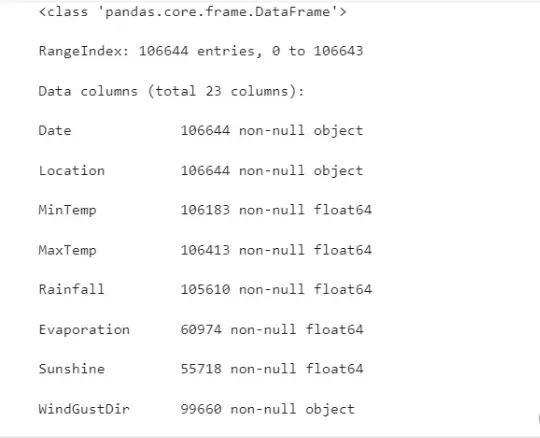
? ? ? ? ?## 進行簡單的數(shù)據(jù)查看,我們可以利用 .head() 頭部.tail()尾部 ? ?data.head()
由于變量太多,這里只展示部分變量
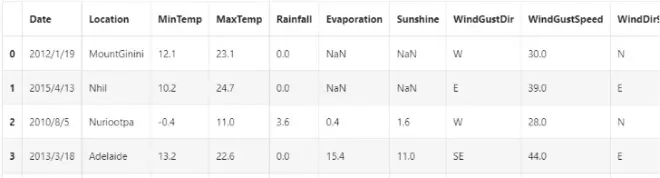
這里我們發(fā)現(xiàn)數(shù)據(jù)集中存在NaN,一般的我們認為NaN在數(shù)據(jù)集中代表了缺失值,可能是數(shù)據(jù)采集或處理時產(chǎn)生的一種錯誤。這里我們采用-1將缺失值進行填補,還有其他例如“中位數(shù)填補、平均數(shù)填補”的缺失值處理方法有興趣的可以查看我的另一篇博客:
,這里詳細的解釋的數(shù)據(jù)預處理的基本操作.? ? ?data = data.fillna(-1) ? ?data.tail()
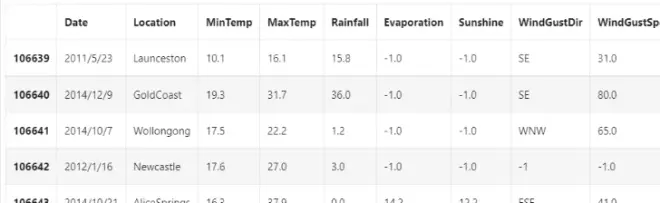
? ? ? ? ?## 利用value_counts函數(shù)查看訓練集標簽的數(shù)量 ? ?pd.Series(data['RainTomorrow']).value_counts()
No 82786 ? ,Yes 23858 ? ,Name: RainTomorrow, dtype: int64
我們發(fā)現(xiàn)數(shù)據(jù)集中的負樣本數(shù)量遠大于正樣本數(shù)量,這種常見的問題叫做“數(shù)據(jù)不平衡”問題,在某些情況下需要進行一些特殊處理。解決數(shù)據(jù)不平衡的辦法有數(shù)據(jù)變換或者數(shù)據(jù)插補等等。
? ? ?## 對于特征進行一些統(tǒng)計描述 ? ?data.describe()
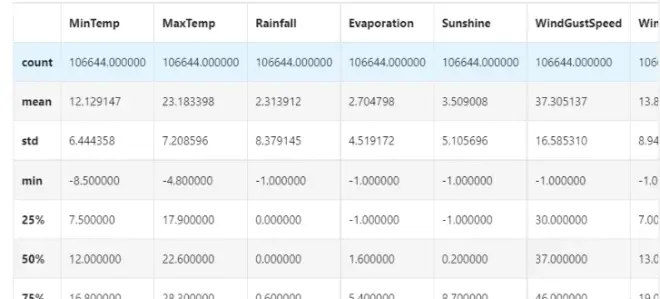
Step4:可視化描述
為了方便,我們先紀錄數(shù)字特征與非數(shù)字特征:
? ? ?numerical_features = [x for x in data.columns if data[x].dtype == np.float] ? ?numerical_features = [x for x in data.columns if data[x].dtype == np.float] ? ?
## 選取三個特征與標簽組合的散點可視化
sns.pairplot(data=data[['Rainfall',
'Evaporation',
'Sunshine'] + ['RainTomorrow']], diag_kind='hist', hue= 'RainTomorrow')
plt.show()
從上圖可以發(fā)現(xiàn),在2D情況下不同的特征組合對于第二天下雨與不下雨的散點分布,以及大概的區(qū)分能力。相對的Sunshine與其他特征的組合更具有區(qū)分能力
? ? ?for col in data[numerical_features].columns: ? ? ? ?if col != 'RainTomorrow': ? ? ? ? ? ?sns.boxplot(x='RainTomorrow', y=col, saturation=0.5, palette='pastel', data=data) ? ? ? ? ? ?plt.title(col) ? ? ? ? ? ?plt.show()
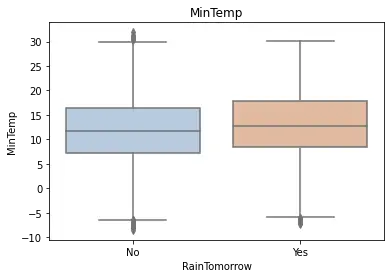
?
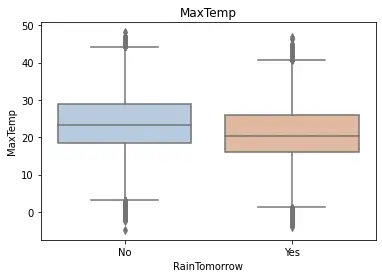
?
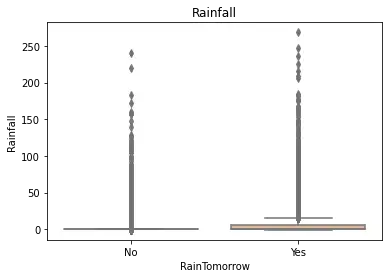
?
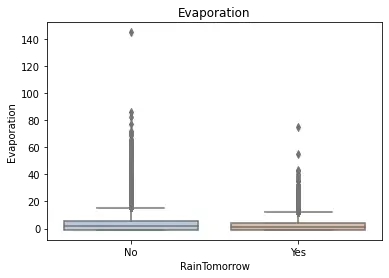
?
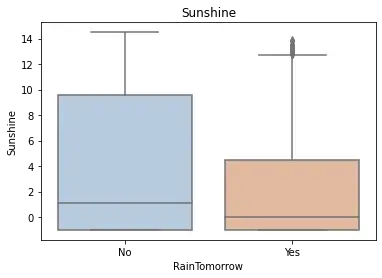
?
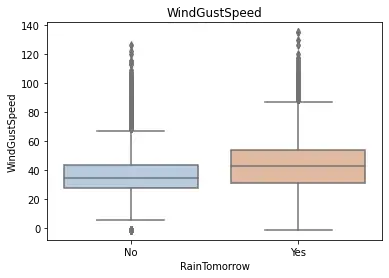
?
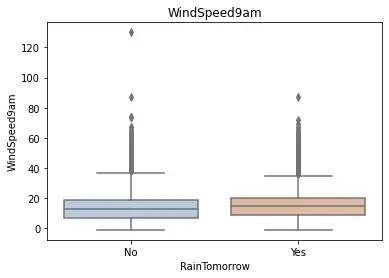
?
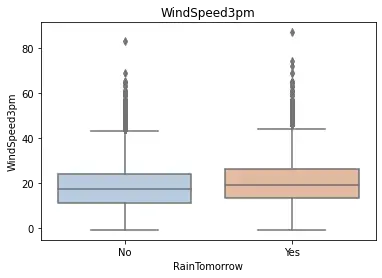
? 利用箱型圖我們也可以得到不同類別在不同特征上的分布差異情況。我們可以發(fā)現(xiàn)Sunshine,Humidity3pm,Cloud9am,Cloud3pm的區(qū)分能力較強
? ?? ?tlog = {} ?? ?for i in category_features: ?? ? ? ?tlog[i] = data
.value_counts() ?? ?flog = {} ?? ?for i in category_features: ?? ? ? ?flog[i] = data .value_counts()
? ?? ? ?? ?plt.figure(figsize=(10,10)) ?? ?plt.subplot(1,2,1) ?? ?plt.title('RainTomorrow') ?? ?sns.barplot(x = pd.DataFrame(tlog['Location']).sort_index()['Location'], y = pd.DataFrame(tlog['Location']).sort_index().index, color = "red") ?? ?plt.subplot(1,2,2) ?? ?plt.title('Not RainTomorrow') ?? ?sns.barplot(x = pd.DataFrame(flog['Location']).sort_index()['Location'], y = pd.DataFrame(flog['Location']).sort_index().index, color = "blue") ?? ?plt.show()
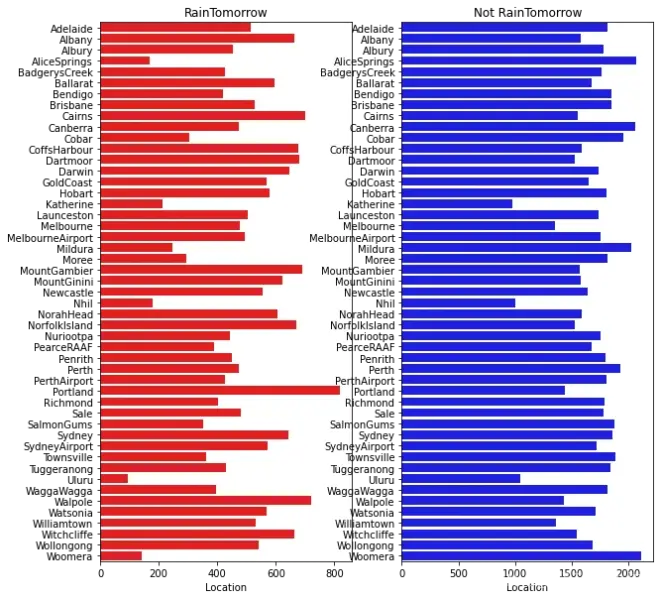
從上圖可以發(fā)現(xiàn)不同地區(qū)降雨情況差別很大,有些地方明顯更容易降雨
? ?? ?plt.figure(figsize=(10,2)) ?? ?plt.subplot(1,2,1) ?? ?plt.title('RainTomorrow') ?? ?sns.barplot(x = pd.DataFrame(tlog
).sort_index()['RainToday'], y = pd.DataFrame(tlog ).sort_index().index, color = "red") ?? ?plt.subplot(1,2,2) ?? ?plt.title('Not RainTomorrow') ?? ?sns.barplot(x = pd.DataFrame(flog ).sort_index()['RainToday'], y = pd.DataFrame(flog ).sort_index().index, color = "blue") ?? ?plt.show()
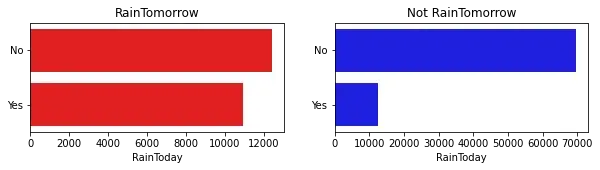
? 上圖我們可以發(fā)現(xiàn),今天下雨明天不一定下雨,但今天不下雨,第二天大概率也不下雨。
Step5:對離散變量進行編碼
由于XGBoost無法處理字符串類型的數(shù)據(jù),我們需要一些方法講字符串數(shù)據(jù)轉(zhuǎn)化為數(shù)據(jù)。一種最簡單的方法是把所有的相同類別的特征編碼成同一個值,例如女=0,男=1,狗狗=2,所以最后編碼的特征值是在 [0,特征數(shù)數(shù)量-1]之間的整數(shù)。除此之外,還有獨熱編碼、求和編碼、留一法編碼等等方法可以獲得更好的效果。
? ?? ?## 把所有的相同類別的特征編碼為同一個值 ?? ?def get_mapfunction(x): ?? ? ? ?mapp = dict(zip(x.unique().tolist(), ?? ? ? ? ? ? range(len(x.unique().tolist())))) ?? ? ? ?def mapfunction(y): ?? ? ? ? ? ?if y in mapp: ?? ? ? ? ? ? ? ?return mapp[y] ?? ? ? ? ? ?else: ?? ? ? ? ? ? ? ?return -1 ?? ? ? ?return mapfunction ?? ?for i in category_features: ?? ? ? ?data[i] = data[i].apply(get_mapfunction(data[i]))
? ?? ? ?? ?## 編碼后的字符串特征變成了數(shù)字 ?? ?
data['Location'].unique()

Step6:利用 XGBoost 進行訓練與預測
? ?? ?## 為了正確評估模型性能,將數(shù)據(jù)劃分為訓練集和測試集,并在訓練集上訓練模型,在測試集上驗證模型性能。 ?? ?from sklearn.model_selection import train_test_split ?? ?
## 選擇其類別為0和1的樣本 (不包括類別為2的樣本)
data_target_part = data['RainTomorrow']
data_features_part = data[[x for x in data.columns if x != 'RainTomorrow']]
## 測試集大小為20%, 80%/20%分
x_train, x_test, y_train, y_test = train_test_split(data_features_part, data_target_part, test_size = 0.2, random_state = 2020)
? ?? ? ?? ?## 導入XGBoost模型 ?? ?from xgboost.sklearn import XGBClassifier ?? ?## 定義 XGBoost模型 ? ? ?clf = XGBClassifier() ?? ?# 在訓練集上訓練XGBoost模型 ?? ?clf.fit(x_train, y_train)
? ?? ? ?? ?## 在訓練集和測試集上分布利用訓練好的模型進行預測 ?? ?train_predict = clf.predict(x_train) ?? ?test_predict = clf.predict(x_test) ?? ?from sklearn import metrics ?? ?
## 利用accuracy(準確度)【預測正確的樣本數(shù)目占總預測樣本數(shù)目的比例】評估模型效果
print('The accuracy of the Logistic Regression is:',metrics.accuracy_score(y_train,train_predict))
print('The accuracy of the Logistic Regression is:',metrics.accuracy_score(y_test,test_predict))
## 查看混淆矩陣 (預測值和真實值的各類情況統(tǒng)計矩陣)
confusion_matrix_result = metrics.confusion_matrix(test_predict,y_test)
print('The confusion matrix result:\n',confusion_matrix_result)
# 利用熱力圖對于結(jié)果進行可視化
plt.figure(figsize=(8, 6))
sns.heatmap(confusion_matrix_result, annot=True, cmap='Blues')
plt.xlabel('Predicted labels')
plt.ylabel('True labels')
plt.show()
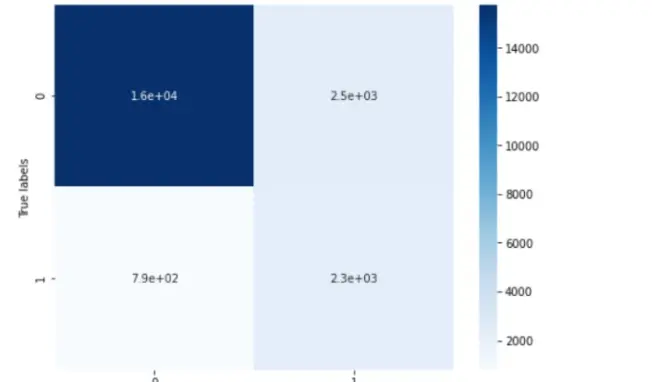
我們可以發(fā)現(xiàn)共有15759 + 2306個樣本預測正確,2470 + 794個樣本預測錯誤。
Step7: 利用 XGBoost 進行特征選擇
? ?? ?sns.barplot(y=data_features_part.columns, x=clf.feature_importances_)
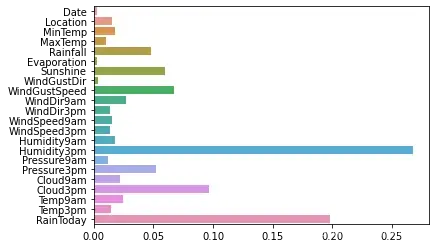
從圖中我們可以發(fā)現(xiàn)下午3點的濕度與今天是否下雨是決定第二天是否下雨最重要的因素
初次之外,我們還可以使用XGBoost中的下列重要屬性來評估特征的重要性。
weight:是以特征用到的次數(shù)來評價
gain:當利用特征做劃分的時候的評價基尼指數(shù)
cover:利用一個覆蓋樣本的指標二階導數(shù)(具體原理不清楚有待探究)平均值來劃分。
total_gain:總基尼指數(shù)
total_cover:總覆蓋
? ?? ?from sklearn.metrics import accuracy_score ?? ?from xgboost import plot_importance ?? ?
def estimate(model,data):
? ?#sns.barplot(data.columns,model.feature_importances_)
? ?ax1=plot_importance(model,importance_type="gain")
? ?ax1.set_title('gain')
? ?ax2=plot_importance(model, importance_type="weight")
? ?ax2.set_title('weight')
? ?ax3 = plot_importance(model, importance_type="cover")
? ?ax3.set_title('cover')
? ?plt.show()
def classes(data,label,test):
? ?model=XGBClassifier()
? ?model.fit(data,label)
? ?ans=model.predict(test)
? ?estimate(model, data)
? ?return ans
ans=classes(x_train,y_train,x_test)
pre=accuracy_score(y_test, ans)
print('acc=',accuracy_score(y_test,ans))
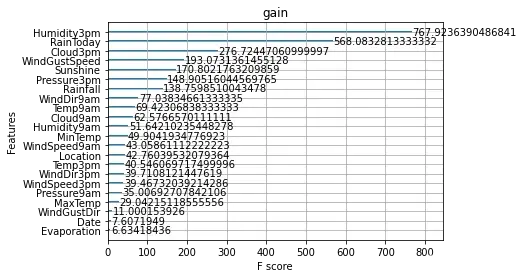
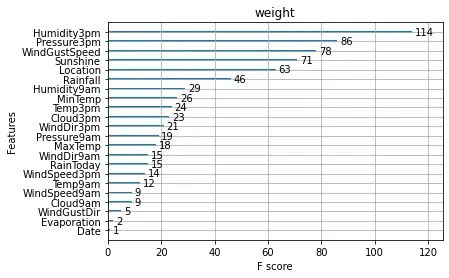
?
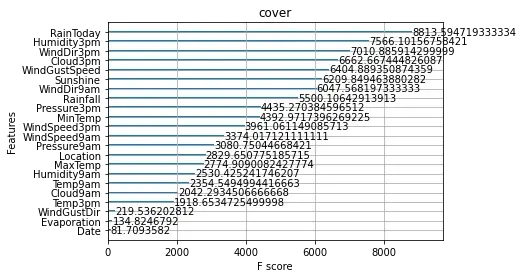
這些圖同樣可以幫助我們更好的了解其他重要特征。
Step8: 通過調(diào)整參數(shù)獲得更好的效果
XGBoost中包括但不限于下列對模型影響較大的參數(shù):
learning_rate: 有時也叫作eta,系統(tǒng)默認值為0.3。每一步迭代的步長,很重要。太大了運行準確率不高,太小了運行速度慢。
subsample:系統(tǒng)默認為1。這個參數(shù)控制對于每棵樹,隨機采樣的比例。減小這個參數(shù)的值,算法會更加保守,避免過擬合, 取值范圍零到一。
colsample_bytree:系統(tǒng)默認值為1。我們一般設(shè)置成0.8左右。用來控制每棵隨機采樣的列數(shù)的占比(每一列是一個特征)。
max_depth: 系統(tǒng)默認值為6,我們常用3-10之間的數(shù)字。這個值為樹的最大深度。這個值是用來控 ? 制過擬合的。max_depth越大,模型學習的更加具體。 ? 調(diào)節(jié)模型參數(shù)的方法有貪心算法、網(wǎng)格調(diào)參、貝葉斯調(diào)參等。這里我們采用網(wǎng)格調(diào)參,它的基本思想是窮舉搜索:在所有候選的參數(shù)選擇中,通過循環(huán)遍歷,嘗試每一種可能性,表現(xiàn)最好的參數(shù)就是最終的結(jié)果
? ?? ?## 從sklearn庫中導入網(wǎng)格調(diào)參函數(shù) ?? ?from sklearn.model_selection import GridSearchCV ?? ?
## 定義參數(shù)取值范圍
learning_rate = [0.1, 0.3, 0.6]
subsample = [0.8, 0.9]
colsample_bytree = [0.6, 0.8]
max_depth = [3,5,8]
parameters = { 'learning_rate': learning_rate,
? ? ? ? ? ? ?'subsample': subsample,
? ? ? ? ? ? ?'colsample_bytree':colsample_bytree,
? ? ? ? ? ? ?'max_depth': max_depth}
model = XGBClassifier(n_estimators = 50)
## 進行網(wǎng)格搜索
clf = GridSearchCV(model, parameters, cv=3, scoring='accuracy',verbose=1,n_jobs=-1)
clf = clf.fit(x_train, y_train)
? ?? ? ?? ?## 在訓練集和測試集上分布利用最好的模型參數(shù)進行預測 ?? ?
## 定義帶參數(shù)的 XGBoost模型
clf = XGBClassifier(colsample_bytree = 0.6, learning_rate = 0.3, max_depth= 8, subsample = 0.9)
# 在訓練集上訓練XGBoost模型
clf.fit(x_train, y_train)
train_predict = clf.predict(x_train)
test_predict = clf.predict(x_test)
## 利用accuracy(準確度)【預測正確的樣本數(shù)目占總預測樣本數(shù)目的比例】評估模型效果
print('The accuracy of the Logistic Regression is:',metrics.accuracy_score(y_train,train_predict))
print('The accuracy of the Logistic Regression is:',metrics.accuracy_score(y_test,test_predict))
## 查看混淆矩陣 (預測值和真實值的各類情況統(tǒng)計矩陣)
confusion_matrix_result = metrics.confusion_matrix(test_predict,y_test)
print('The confusion matrix result:\n',confusion_matrix_result)
# 利用熱力圖對于結(jié)果進行可視化
plt.figure(figsize=(8, 6))
sns.heatmap(confusion_matrix_result, annot=True, cmap='Blues')
plt.xlabel('Predicted labels')
plt.ylabel('True labels')
plt.show()
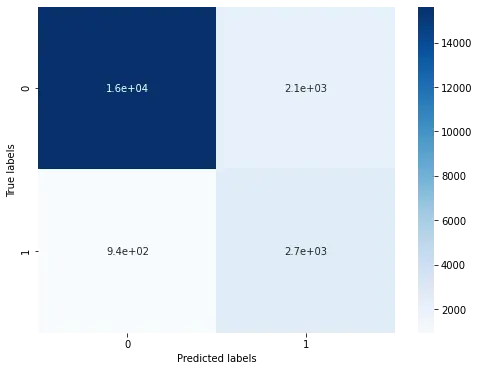
原本有2470 + 790個錯誤,現(xiàn)在有 2112 + 939個錯誤,帶來了明顯的正確率提升。
最后
畢設(shè)幫助,選題指導,技術(shù)解答,歡迎打擾,見B站個人主頁
https://space.bilibili.com/33886978