人工智能的大統(tǒng)一理論? | 智源大會-「人工智能的數(shù)理基礎」專題論壇
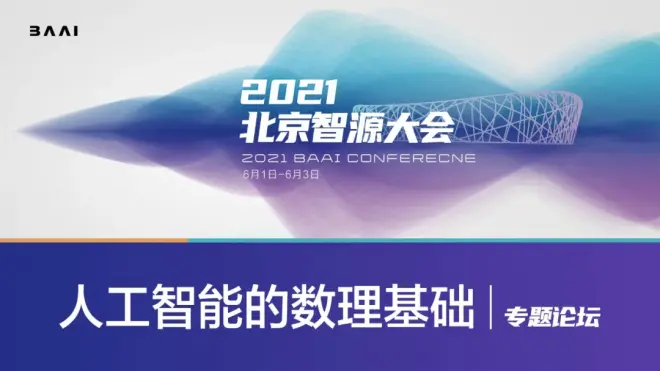
當下人工智能面臨著可計算性、可解釋性、泛化性、穩(wěn)定性等諸多重大理論挑戰(zhàn),如何打破基于計算機實驗和認知神經(jīng)科學的人工智能傳統(tǒng)建模范式,建立起以數(shù)學與統(tǒng)計理論為第一原理的新一代人工智能方法論,依然是待開墾的處女地,也是數(shù)學家們不可多得的機遇。
議程?
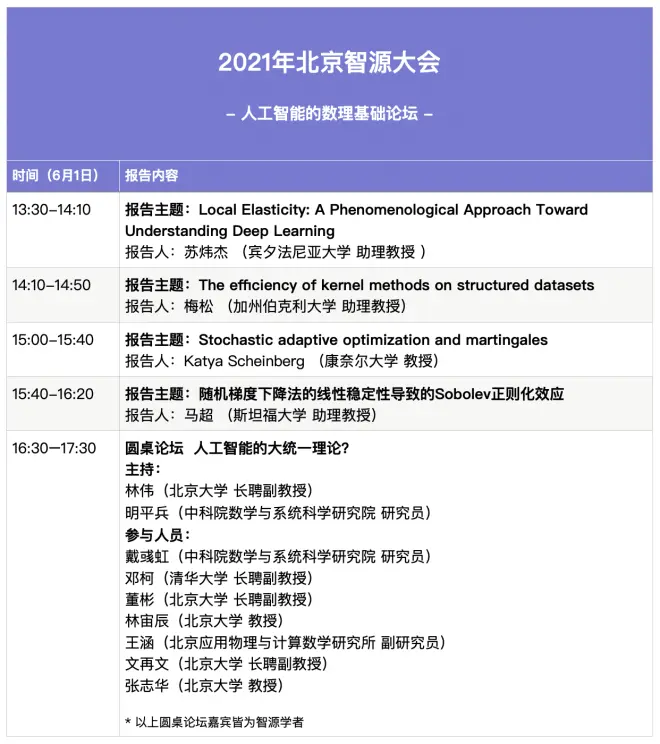
掃碼加入「AI數(shù)理基礎」論壇交流群,參與相關(guān)話題討論
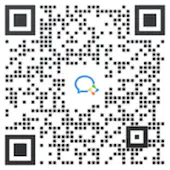
嘉賓介紹
論壇主席-張平文
張平文,教授,中國科學院院士,發(fā)展中國家科學院院士,北京大學副校長 ,北京大學科學與工程計算中心主任,大數(shù)據(jù)分析與應用技術(shù)國家工程實驗室主任。研究方向:復雜流體的多尺度建模與計算,移動網(wǎng)格方向與應用,多尺度分析與計算。

報告嘉賓-蘇煒杰
蘇煒杰,現(xiàn)任賓夕法尼亞大學沃頓商學院助理教授。2011年本科畢業(yè)于北京大學數(shù)學科學學院,2016年在斯坦福大學獲得統(tǒng)計學博士學位。蘇煒杰曾獲得2016年Theodore Anderson數(shù)理統(tǒng)計博士論文獎,2019年美國自然科學基金頒發(fā)的CAREER Award,和2020年斯隆研究獎。研究興趣包括機器學習特別是深度學習的理論基礎、隱私數(shù)據(jù)分析、高維統(tǒng)計,以及優(yōu)化理論。
報告主題:Local Elasticity: A Phenomenological Approach Toward Understanding Deep Learning
報告摘要:Motivated by the iterative nature of training neural networks, we ask: If the weights of a neural network are updated using the induced gradient on an image of a tiger, how does this update impact the prediction of the neural network at another image (say, an image of another tiger, a cat, or a plane)? To address this question, I will introduce a phenomenon termed local elasticity. Roughly speaking, our experiments show that modern deep neural networks are locally elastic in the sense that the change in prediction is likely to be most significant at another tiger and least significant at a plane, at late stages of the training process. I will illustrate some implications of local elasticity by relating it to the neural tangent kernel and improving on the generalization bound for uniform stability. Moreover, I will introduce a phenomenological model for simulating neural networks, which suggests that local elasticity may result from feature sharing between semantically related images and the hierarchical representations of high-level features. Finally, I will offer a local-elasticity-focused agenda for future research toward a theoretical foundation for deep learning.

報告嘉賓-梅松
梅松博士于2020年起就任加州大學伯克利分校統(tǒng)計系助理教授;此前于2014年獲得北京大學數(shù)學系學士學位,2020年獲得斯坦福大學計算數(shù)學與工程系博士學位。梅松博士的研究將統(tǒng)計物理理論應用于理論機器學習和高維概率統(tǒng)計,特別是提出了雙層神經(jīng)網(wǎng)絡優(yōu)化的平均場理論,并發(fā)展了隨機特征方法與神經(jīng)切向核模型的泛化理論。
報告主題:The efficiency of kernel methods on structured datasets
報告摘要:Inspired by the proposal of tangent kernels of neural networks (NNs), a recent research line aims to design kernels with a better generalization performance on standard datasets. Indeed, a few recent works showed that certain kernel machines perform as well as NNs on certain datasets, despite their separations in specific cases implied by theoretical results. Furthermore, it was shown that the induced kernels of convolutional neural networks perform much better than any former handcrafted kernels. These empirical results pose a theoretical challenge to understanding the performance gaps in kernel machines and NNs in different scenarios. In this talk, we show that data structures play an essential role in inducing these performance gaps. We consider a few natural data structures, and study their effects on the performance of these learning methods. Based on a fine-grained high dimensional asymptotics framework of analyzing random features models and kernel machines, we show the following: 1) If the feature vectors are nearly isotropic, kernel methods suffer from the curse of dimensionality, while NNs can overcome it by learning the best low-dimensional representation; 2) If the feature vectors display the same low-dimensional structure as the target function (the spiked covariates model), this curse of dimensionality becomes milder, and the performance gap between kernel methods and NNs become smaller; 3) On datasets that display some invariance structure (e.g., image dataset), there is a quantitative performance gain of using invariant kernels (e.g., convolutional kernels) over inner product kernels. Beyond explaining the performance gaps, these theoretical results can further provide some intuitions towards designing kernel methods with better performance.

報告嘉賓-Katya Scheinberg
Katya Scheinberg,康奈爾大學教授。在加入康奈爾大學之前,她是里海大學工業(yè)和系統(tǒng)工程系Harvey E. Wagner講座教授。她本科就讀于莫斯科大學,并在哥倫比亞大學獲得博士學位。2010年加入Lehigh之前,她曾在IBM T.J. Watson研究中心擔任研究人員超過10年。Katya的主要研究領域是針對連續(xù)優(yōu)化中的各種問題開發(fā)實用算法(及其理論分析),如凸優(yōu)化、無導數(shù)優(yōu)化、機器學習、二次規(guī)劃等。2015年,她與安迪·康恩(Andy Conn)和路易斯·維森特(Luis Vicente)共同獲得了拉格朗日獎(Lagrange Prize)。2019年,她被Informs Optimization Society授予Farkas獎。
報告主題:Stochastic adaptive optimization and martingales
報告摘要:We will present a general framework which models standard methods such as line search and trust region methods in a stochastic setting via analysis of stochastic processes and their stopping time. We will show how this framework models some variants of stochastic line search and how analyzing the stopping time gives us a high probability bound (and bound in expectation) on the complexity of the line search.? This framework provides strong convergence analysis under weaker conditions than alternative approaches in the literature.

報告嘉賓-馬超?
馬超,2016年本科畢業(yè)于北京大學數(shù)學科學學院,后赴普林斯頓大學應用數(shù)學系攻讀博士,并于2020年取得博士學位,導師為鄂維南教授。此后于斯坦福大學任Szego助理教授至今。馬超的主要研究方向為神經(jīng)網(wǎng)絡模型的數(shù)學基礎,尤其是其優(yōu)化與泛化表現(xiàn)。此外,他也致力于深度學習方法在科學計算問題中的應用。
報告主題:The Sobolev regularization effect from the linear stability of sgd(隨機梯度下降法的線性穩(wěn)定性導致的Sobolev正則化效應)
報告摘要:我們探索了神經(jīng)網(wǎng)絡模型的輸入層中輸入數(shù)據(jù)與參數(shù)矩陣的乘法關(guān)系,并由此關(guān)系建立了模型函數(shù)關(guān)于參數(shù)的梯度和關(guān)于數(shù)據(jù)的梯度間的聯(lián)系。利用此聯(lián)系,我們證明最小值的平緩程度對模型函數(shù)(關(guān)于數(shù)據(jù))的Sobolev半范數(shù)具有正則化作用,此結(jié)果在理論上解釋了為何平緩的最優(yōu)值通常具有較好的泛化表現(xiàn)。隨后,我們將最優(yōu)值的平緩程度推廣到最優(yōu)值處不同訓練數(shù)據(jù)對應目標函數(shù)的梯度的高階矩,并利用最優(yōu)值處的線性穩(wěn)定理論證明隨機梯度下降法對這些矩具有控制作用,并進一步由前述乘法關(guān)系推導出隨機梯度下降法對模型函數(shù)的Sobolev半范數(shù)的正則化效應。最終,基于以上Sobolev正則化效應以及關(guān)于數(shù)據(jù)分布的必要假設我們?yōu)殡S機梯度下降法推導出一組泛化誤差估計。

2021智源大會群英薈萃,已確認出席嘉賓包括圖靈獎得主Yoshua Bengio、David Patterson,以及人工智能各領域多位世界級的專家。本屆大會采用線上+線下模式,線上報名已開啟,線下參會和官網(wǎng)即將公開。
大會官網(wǎng):https://2021.baai.ac.cn/
線上報名:
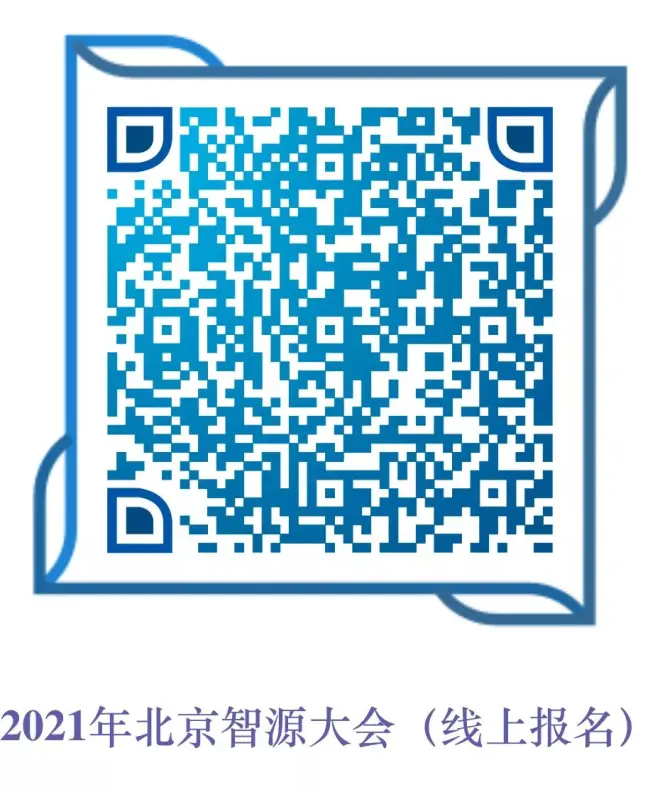
線下報名:
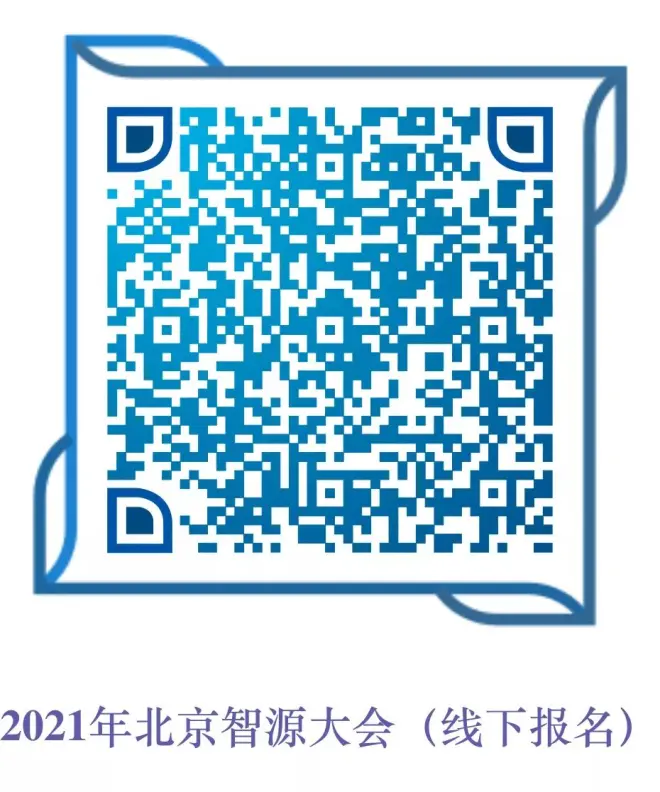
人工智能領域不容錯過的內(nèi)行分享,等你一同見證!