【中英雙語】AI營銷來了,這四種誤區(qū)需要先行避開
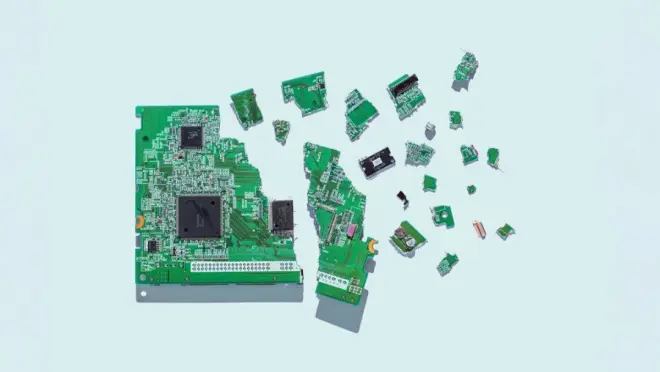
Why You Aren't Getting More from Your Marketing AI
伊娃·阿斯卡爾薩(Eva Ascarza) 邁克爾·羅斯(Michael Ross)布魯斯·哈迪(Bruce G.S. Hardie) | 文 ?
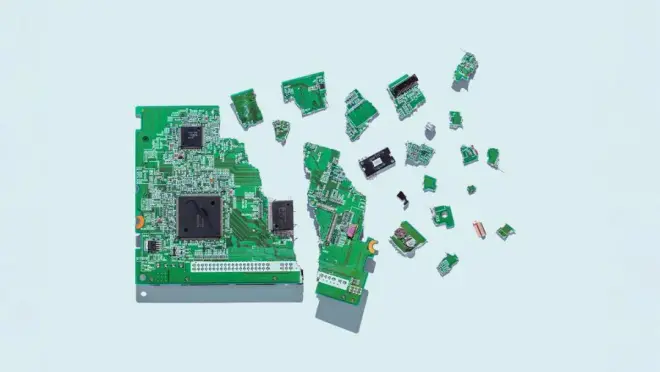
When a large telecom company’s?marketers set?out to reduce customer churn, they decided to use artificial intelligence to determine which customers were most likely to defect. Armed with the AI’s predictions, they bombarded the at-risk customers with promotions enticing them to stay. Yet many left despite the retention campaign. Why? The managers had made a fundamental error: They had asked the algorithm the wrong question. While the AI’s predictions were good, they didn’t address the real problem the managers were trying to solve.
大型電信企業(yè)的營銷人員著手降低客戶流失率,決定運用人工智能確定哪些客戶會轉(zhuǎn)投競爭對手。在AI預(yù)測的輔助下,他們用各種促銷活動“轟炸”可能流失的顧客,想吸引這部分顧客留下,但很多顧客還是離開了。原因何在?因為他們犯了一個根本性的錯誤:向算法提出的問題不對。AI預(yù)測得很準,但解決的并不是營銷者真正要嘗試解決的問題。?
That kind of scenario is all too common among companies using AI to inform business decisions. In a 2019?survey of 2,500 executivesconducted by?Sloan Management Review?and the Boston Consulting Group, 90% of respondents said that their companies had invested in AI, but fewer than 40% of them had seen business gains from it in the previous three years.?
這種情況在利用AI輔助業(yè)務(wù)決策的公司屢見不鮮。2019年《斯隆管理評論》和波士頓咨詢公司開展的一項涉及2500位高管的問卷調(diào)查中,90%的參與者表示自己的公司投資了AI,但在過去三年里看到成效的卻不到40%。?
In our academic, consulting, and nonexecutive director roles, we have studied and advised more than 50 companies, examining the main challenges they face as they seek to leverage AI in their marketing. This work has allowed us to identify and categorize the errors marketers most frequently make with AI.?
我們在學(xué)術(shù)、咨詢和非常務(wù)董事的工作中,曾對五十多家公司做過研究或提供過建議。我們考察了這些公司嘗試利用AI輔助營銷的過程中遇到的主要挑戰(zhàn)。這項工作讓我們總結(jié)出營銷者在AI應(yīng)用方面最常犯的錯誤。??
Failure to Ask the Right Question
沒能提出正確的問題
The real concern of the managers at our telecom firm should not have been identifying potential defectors; it should have been figuring out how to use marketing dollars to reduce churn. Rather than asking the AI who was most likely to leave, they should have asked who could best be persuaded to stay—in other words, which customers considering jumping ship would be most likely to respond to a promotion. Just as politicians direct their efforts at swing voters, managers should target actions toward swing customers. By giving the AI the wrong objective, the telecom marketers squandered their money on swaths of customers who were going to defect anyway and?underinvested?in customers they should have doubled down on.
上述電信公司的營銷經(jīng)理真正關(guān)心的并不是找出可能流失的顧客,而是設(shè)法利用營銷資金降低流失率。與其問AI最有可能離開的是哪些顧客,不如問打算離開的顧客里哪些人最有可能被說服繼續(xù)留下——換言之,考慮離開的顧客里有哪些會對促銷活動做出回應(yīng)。就像政客爭取游離選民一樣,管理者也應(yīng)當瞄準尚未確定心意的游離客戶。電信公司的營銷團隊向AI提出錯誤的問題,把錢浪費在無論如何都會離開的一小部分客戶身上,而對原本應(yīng)該雙倍投入的游離客戶卻投資不足。?
In a similar case, marketing managers at a gaming company wanted to encourage users to spend more money while they were playing its game. The marketers asked the data science team to figure out what new features would most increase users’ engagement. The team used algorithms to tease out the relationship between possible features and the amount of time customers spent playing, ultimately predicting that offering prizes and making the public ranking of users’ positions more prominent would keep people in the game longer. The company made adjustments accordingly, but new revenues didn’t follow. Why not? Because managers, again, had asked the AI the wrong question: how to increase players’ engagement rather than how to increase their in-game spending. Because most users didn’t spend money inside the game, the strategy fell flat.??
還有另一個相似的例子:某家游戲公司希望鼓勵用戶在游戲里多花錢。營銷者讓數(shù)據(jù)科學(xué)團隊找出最能增加用戶投入度的新功能。團隊用算法整理出了可以添加的新功能和玩家投入的時間之間的關(guān)系,最后做出預(yù)測:提供獎勵并突出用戶排名,能讓玩家停留時間增加。公司于是做出相應(yīng)的調(diào)整,但收入并無起色。原因何在?因為管理者又問錯了問題:增加玩家投入度,并不等于增加玩家給游戲充的錢,因為大部分用戶玩游戲不會花錢。這個戰(zhàn)略失敗了。? ?
At both companies, marketing managers failed to think carefully about the business problem being addressed and the prediction needed to inform the best decision. AI would have been extremely valuable?if?it had predicted which telecom customers would be most persuadable and which game features would increase players’ spending.?
兩個公司的營銷經(jīng)理都沒有仔細考慮需要解決的問題究竟是什么、做出最佳決策需要怎樣的預(yù)測。假如用AI預(yù)測哪些用戶能被挽留、哪些游戲功能最能促使玩家花錢,將會發(fā)揮極大的作用。
Failure to Recognize the Difference Between the Value of Being Right and the Costs of Being Wrong
沒有分別認識到問對問題的價值和問錯問題的代價
AI’s predictions should be as accurate as possible, shouldn’t they? Not necessarily. A bad forecast can be extremely expensive in some cases but less so in others; likewise, superprecise forecasts create more value in some situations than in others. Marketers—and, even more critically, the data science teams they rely on—often overlook this.
AI的預(yù)測應(yīng)當盡可能地準確,不是嗎?不一定。不好的預(yù)測在部分情況下可能令企業(yè)付出異常昂貴的代價,但其他時候的花費則會低一些;同樣,極其準確的預(yù)測在部分情況下能夠發(fā)揮更大的價值。營銷者乃至其背后的數(shù)據(jù)科學(xué)團隊都常常忽略這一點。? ??
Consider the consumer goods company whose data scientists proudly announced that they’d increased the accuracy of a new sales-volume forecasting system, reducing the error rate from 25% to 17%. Unfortunately, in improving the system’s overall accuracy, they increased its precision with low-margin products while reducing its accuracy with high-margin products. Because the cost of underestimating demand for the high-margin offerings substantially outweighed the value of correctly forecasting demand for the low-margin ones, profits fell when the company implemented the new, “more accurate” system.
例如,某消費品公司的數(shù)據(jù)科學(xué)團隊驕傲地宣布他們提升了新的銷售量預(yù)測系統(tǒng)的準確度,將誤差率從25%降低到17%??上嵘丝傮w準確度,低利潤產(chǎn)品相關(guān)的準確度也一并提升,高利潤產(chǎn)品的準確度卻有所下降。低估高利潤產(chǎn)品需求的代價遠遠高于準確預(yù)測低利潤產(chǎn)品需求的價值,因此這個“更準確”的新系統(tǒng)反而導(dǎo)致公司利潤下降。???
It’s important to recognize that AI’s predictions can be wrong in different ways. In addition to over- or underestimating results, they can give false positives (for instance, identifying customers who actually stay as probable defectors) or false negatives (identifying customers who subsequently leave as unlikely defectors). The marketer’s job is to analyze the relative cost of these types of errors, which can be very different. But this issue is often ignored by, or not even communicated to, the data science teams that build prediction models, who then assume all errors are equally important, leading to expensive mistakes.???
必須認識到AI預(yù)測會出現(xiàn)各種不同的偏誤。除了高估和低估,還有可能出現(xiàn)誤報(比如將原本就會留下的顧客判定為可能離開)和漏報(將會離開的顧客判定為不會離開)。這幾類偏誤的相對成本相差很大,營銷者需要對此進行分析??墒?,這個問題往往被建立預(yù)測模型的數(shù)據(jù)科學(xué)團隊忽略,或者根本不會告知數(shù)據(jù)團隊。于是數(shù)據(jù)團隊默認所有偏誤都同等重要,造成昂貴的錯誤。
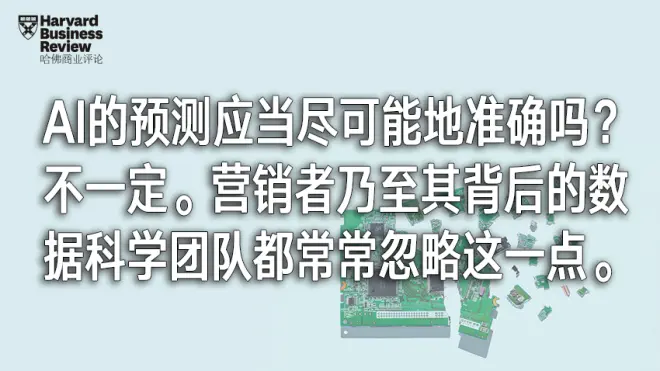
Failure to Leverage Granular Predictions
未能利用更細致的預(yù)測? ??
Firms generate torrents of customer and operational data, which standard AI tools can use to make detailed, high-frequency predictions. But many marketers don’t exploit that capability and keep operating according to their old decision-making models. Take the hotel chain whose managers meet weekly to adjust prices at the location level despite having AI that can update demand forecasts for different room types on an hourly basis. Their decision-making process remains a relic of an antiquated booking system.
企業(yè)會產(chǎn)生大量的顧客數(shù)據(jù)和運營數(shù)據(jù),標準化AI工具可以用于進行高頻率的細致預(yù)測。但許多營銷者并不開發(fā)這種能力,依然用舊的決策模型指導(dǎo)運營。例如某連鎖酒店,管理者每周開會調(diào)整區(qū)域價格,卻將可以每小時更新不同房型需求預(yù)測的AI閑置。他們的決策流程依然延續(xù)著過時的預(yù)訂系統(tǒng)的做法。? ? ?
Another major impediment is managers’ failure to get the granularity and frequency of their decisions right. In addition to reviewing the pace of their decision-making, they should ask whether decisions based on aggregate-level predictions should draw on more finely tuned predictions. Consider a marketing team deciding how to allocate its ad dollars on keyword searches on Google and Amazon. The data science team’s current AI can predict the lifetime value of customers acquired through those channels. However, the marketers might get a higher return on ad dollars by using more-granular predictions about customer lifetime value per keyword per channel.
另一個大的障礙是管理者未能準確理解決策的粒度和頻度。在評估決策步調(diào)的時候,管理者應(yīng)當思考,基于整體預(yù)測的決策是否還應(yīng)當參考更加細致的預(yù)測進行調(diào)整。例如某營銷團隊要將廣告資金分撥給谷歌和亞馬遜上搜索關(guān)鍵詞時顯示的廣告,數(shù)據(jù)科學(xué)團隊目前的AI可以預(yù)測通過這些渠道獲得的顧客的終身價值,但若能參考關(guān)于每個渠道、每個關(guān)鍵詞的顧客終身價值的細致預(yù)測,同樣的廣告資金將能獲得更高的回報。
Communication Breakdowns
溝通不良? ? ?
In addition to constantly guarding against the types of errors we’ve described, marketing managers have to do a better job of communicating and collaborating with their data science teams—and being clear about the business problems they’re seeking to solve. That isn’t rocket science, but we often see marketing managers fall short on it.
除了提防以上誤區(qū),營銷經(jīng)理還應(yīng)當更好地與數(shù)據(jù)科學(xué)團隊交流合作,明白自己究竟要解決的是什么問題。這項工作并不復(fù)雜,但我們卻時??吹綘I銷經(jīng)理在這方面有所欠缺。? ? ?
Several things get in the way of productive collaboration. Some managers plunge into AI initiatives without fully understanding the technology’s capabilities and limitations. They may have unrealistic expectations and so pursue projects AI can’t deliver on, or they underestimate how much value AI?could?provide, so their projects lack ambition. Either situation can happen when senior managers are reluctant to reveal their lack of understanding of AI technologies.
有幾個因素會妨礙高效合作。一些管理者尚未完全理解AI技術(shù)的能力和局限就匆忙行動。他們可能抱有不切實際的期望,試圖實行AI無法實現(xiàn)的項目,抑或低估AI可以提供的價值,項目缺乏野心。高層管理者倘若不愿承認自己對AI技術(shù)理解有限,就可能會出現(xiàn)這兩種情況中的一種。? ?
Data science teams are also complicit in the communication breakdown. Often, data scientists gravitate toward projects with familiar prediction requirements, whether or not they are what marketing needs. Without guidance from marketers about how to provide value, data teams will often remain in their comfort zone. And while marketing managers may be reluctant to ask questions (and reveal their ignorance), data scientists often struggle to explain to nontechnical managers what they can and can’t do.?
數(shù)據(jù)科學(xué)團隊也有責(zé)任。數(shù)據(jù)團隊往往被預(yù)測需求較熟悉的項目吸引,不顧真正的營銷需求。如果沒有營銷者關(guān)于如何提供價值的指導(dǎo),數(shù)據(jù)團隊會一直停留在舒適區(qū)。營銷經(jīng)理可能不愿提問(因為會暴露自己的無知),而數(shù)據(jù)團隊常常很難向非技術(shù)人員解釋他們能做到什么、做不到什么。? ?
Marketing needs AI. But AI needs marketing thinking to realize its full potential. This requires the marketing and data science teams to have a constant dialogue so that they can understand how to move from a theoretical solution to something that can be implemented.
營銷需要AI。但AI需要營銷思維,才能充分發(fā)揮潛力。這需要營銷和數(shù)據(jù)科學(xué)團隊保持溝通,唯其如此才能了解如何將理論解決方案應(yīng)用于實踐。
伊娃·阿斯卡爾薩是哈佛商學(xué)院工商管理學(xué)賈克斯基家族教席副教授。邁克爾·羅斯是為零售企業(yè)提供云數(shù)據(jù)分析的Dynamic-Action公司聯(lián)合創(chuàng)始人、倫敦商學(xué)院執(zhí)行研究員。布魯斯·哈迪是倫敦商學(xué)院營銷學(xué)教授。
蔣薈蓉 | 譯 牛文靜 | 校 時青靖 | 編輯
本文有刪節(jié),原文參見《哈佛商業(yè)評論》中文版2021年7月刊《AI營銷潛力為何無法充分發(fā)揮》