信用評(píng)分卡Credit Scorecards (1-3)
up主微信公眾號(hào)pythonEducation
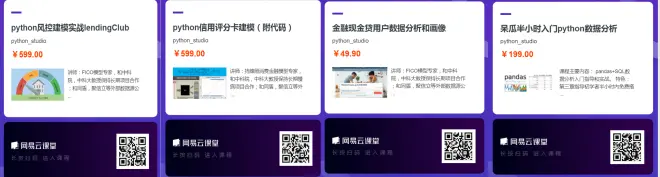
Credit Scorecards – Introduction (part 1 of 7)
http://ucanalytics.com/blogs/credit-scorecards-part-1/
Credit Scorecards in the Age of Credit Crisis
This incident took place at a friend’s party circa 2009, in the backdrop of the worst financial crisis the planet has seen for a long time. The average Joe on the street was aware of terms such as mortgaged-backed securities (MBS), sub-prime lending and credit crisis – the reasons for his plight. Back to our party, I met an informed & compassionate elderly woman and after a few minutes of chitchat, the topic came to what I do for a living. At that point, I was working on a project of developing credit-scorecard for a leading mortgage lender in Mumbai. As I started explaining the details of my job, her expression changed from curious to angst and pain. Eventually, she interrupted and said – why would you do such a thing? Is this not the reason for all the mess? I was used to this reaction and had to correct her misconception.
信用危機(jī)時(shí)代的信用記分卡
這一事件發(fā)生在大約2009年的朋友聚會(huì)上,在這個(gè)星球長(zhǎng)期以來(lái)最嚴(yán)重的金融危機(jī)背景下。 街上的喬普通知道抵押貸款支持證券(MBS),次級(jí)貸款和信貸危機(jī)等條款 - 這是他困境的原因。 回到我們的聚會(huì)上,我遇到了一位知情和富有同情心的老年婦女,經(jīng)過幾分鐘的閑聊,這個(gè)主題來(lái)到了我的生活。 那時(shí),我正在為孟買一家領(lǐng)先的抵押貸款機(jī)構(gòu)開發(fā)一個(gè)信用記分卡項(xiàng)目。 當(dāng)我開始解釋我的工作細(xì)節(jié)時(shí),她的表情從好奇變?yōu)榻箲]和痛苦。 最后,她打斷了她說(shuō) - 你為什么要做這樣的事? 這不是所有混亂的原因嗎? 我習(xí)慣了這種反應(yīng),不得不糾正她的誤解。
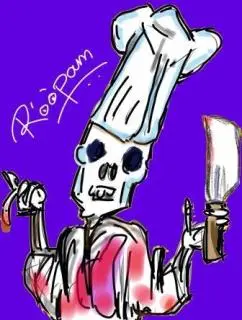
Predictive Analytics: The lurking Danger – by Roopam
Credit or application scorecards can be excellent tools for both lender and borrower to work out debt serving capability of the borrower. For lenders, scorecards can help them assess the creditworthiness of the borrower and maintain a healthy portfolio – which will eventually influence the economy as a whole. Additionally to the borrower, they can provide valuable information such as 45% of people with her socio-economic background have struggled to keep up with the EMI commitment. This could help the borrower make a well-informed decision before getting into a debt trap. Blaming science for reckless human behavior is not new.?I believe, any rigorous science with practical applications is like a sharp German blade, a master chef prepares delicious meals with it and the irresponsible leaves a deep and painful cut.
信用卡或應(yīng)用程序記分卡可以成為貸款人和借款人計(jì)算借款人償債能力的絕佳工具。 對(duì)于貸方而言,記分卡可以幫助他們?cè)u(píng)估借款人的信譽(yù)并維持健康的投資組合 - 這最終將影響整個(gè)經(jīng)濟(jì)。 除借款人外,他們還可以提供有價(jià)值的信息,例如45%具有社會(huì)經(jīng)濟(jì)背景的人都在努力跟上EMI的承諾。 這可以幫助借款人在陷入債務(wù)陷阱之前做出明智的決定。 為魯莽的人類行為指責(zé)科學(xué)并不新鮮。 我相信,任何具有實(shí)際應(yīng)用的嚴(yán)謹(jǐn)科學(xué)就像一把鋒利的德國(guó)刀片,一位大廚用它準(zhǔn)備可口的飯菜,而不負(fù)責(zé)任的會(huì)留下深刻而痛苦的切口。
Scorecards and Predictive Analytics
In the following series, we will explore the practitioners’ approach for developing and maintaining a scorecard. At a very high-level, credit scorecards have their roots in the classification problem in statistics & data mining. The classification problems?present an extremely broad methodology/thought-process that has multiple business applications. A few applications for classification problem are:
? Application or credit scorecards to assess repayment risk of the borrower
? Image analytics of MRI to identify if the cancer is benevolent or malignant
? Behavioral models to identify the most probable future action of the customer
? Identification of potential drug targets in the protein structure
? Fraud detection models
? Sentiment analysis of Tweets and Facebook posts
? Cross/up sell propensity models
? Campaign response models
? Insurance ratings
在下面的系列中,我們將探討從業(yè)者開發(fā)和維護(hù)記分卡的方法。 在非常高的層次上,信用記分卡的根源在于統(tǒng)計(jì)和數(shù)據(jù)挖掘中的分類問題。 分類問題提供了一個(gè)極其廣泛的方法/思維過程,具有多個(gè)業(yè)務(wù)應(yīng)用程序。 一些分類問題的應(yīng)用是:
?應(yīng)用程序或信用記分卡,用于評(píng)估借款人的還款風(fēng)險(xiǎn)
?MRI的圖像分析,以確定癌癥是仁慈的還是惡性的
?行為模型,用于識(shí)別客戶最可能的未來(lái)行為
?鑒定蛋白質(zhì)結(jié)構(gòu)中的潛在藥物靶標(biāo)
?欺詐檢測(cè)模型
?推文和Facebook帖子的情緒分析
?交叉/向上銷售傾向模型
?活動(dòng)響應(yīng)模型
?保險(xiǎn)評(píng)級(jí)
For that matter, there are subtle links between credit scorecards and other models mentioned above. The details of these models could be drastically different but the underlining idea for these models is linked to the classification problem. In this series, I shall focus on credit or application scorecard methodology but will try to bring in other another scorecards and models whenever possible.
就此而言,信用記分卡與上述其他模型之間存在微妙的聯(lián)系。 這些模型的細(xì)節(jié)可能截然不同,但這些模型的強(qiáng)調(diào)理念與分類問題有關(guān)。 在本系列中,我將重點(diǎn)介紹信用卡或應(yīng)用記分卡方法,但會(huì)嘗試盡可能引入其他記分卡和模型。
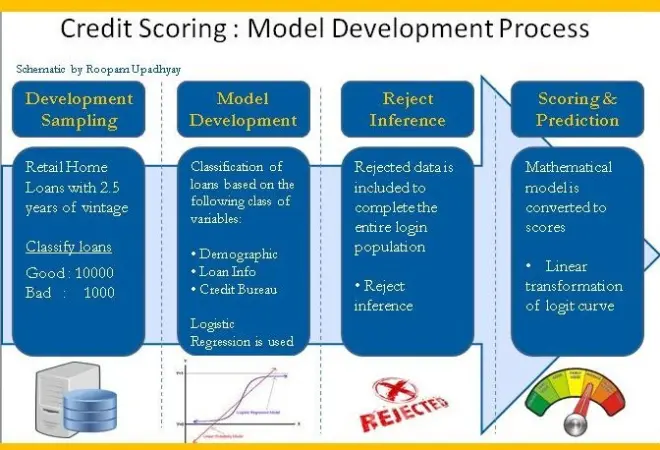
Credit Scoring: Development Stages of Credit Scorecard – by Roopam
Flow of Subsequent Articles
The flow of subsequent articles in the series will be as following
1. Classification problem and sampling
2. Variable selection and coarse classing
3. Predictive Models
4. Logistic regression and scorecards
5. Model validation
6. Application and business process integration
后續(xù)文章的流程
該系列中后續(xù)文章的流程如下
1.分類問題和抽樣
2.變量選擇和粗略分類
3.預(yù)測(cè)模型
4.邏輯回歸和記分卡
5.模型驗(yàn)證
6.應(yīng)用程序和業(yè)務(wù)流程集成
Books for Credit Scorecards?
I have compiled a list of books you may find useful while learning about analytical scorecards. The first four of these books have more or less the same flow, with Anderson’s book (#4) a little more detailed. However, you could choose any one of these four books without losing much .The last book (#5) is a collection of articles / papers by practitioners and academicians and is quite interesting.
信用記分卡的書籍
在編寫分析記分卡時(shí),我編制了一份您可能會(huì)發(fā)現(xiàn)有用的書籍清單。 這些書中的前四本或多或少都有相同的流程,而安德森的書(#4)更為詳細(xì)。 但是,您可以選擇這四本書中的任何一本,而不會(huì)損失太多。最后一本書(#5)是一組由從業(yè)者和學(xué)者組成的文章/論文,非常有趣。
1. Credit Risk Scorecards: Developing and Implementing Intelligent Credit Scoring – Naeem Siddiqi
2. Credit Scoring, Response Modeling, and Insurance Rating: A Practical Guide to Forecasting Consumer Behavior – Steven Finlay
3. Credit Scoring for Risk Managers: The Handbook for Lenders – Elizabeth Mays and Niall Lynas
4. The Credit Scoring Toolkit: Theory and Practice for Retail Credit Risk Management and Decision Automation – Raymond Anderson
5. Credit Risk Models – Elizabeth Mays
Sign-off Note
Look forward to sharing my views on predictive analytics and hearing back from you. See you soon with the second part of this series.
Credit Scorecards – Classification Problem (part 2 of 7)
http://ucanalytics.com/blogs/credit-scorecards-classification-problem-part-2/
?
Classification Problem in Statistics & Data Mining
I must say I was shocked when Amishi, a girl little over three years old, announced that going forward she is only friends with my wife and not me. Her reason for the breakup was that I am a boy and girls can only be friends with girls. She has learned this social norm from her friends at the preschool. I still remember the way she modeled for me in her swimsuit and umbrella just a few months ago. She was aware of the boy-girl difference even then, it is just she has learned this weird social norm now. The point over here is that toddlers can distinguish genders without much effort. Nature has given us a built-in equation to classify gender through a mere glance with a high degree of precision. Imagine a similar mechanism to distinguish between good and bad borrowers. You are talking about every banker’s dream. However, evolution has trained us to mate not to lend.
我必須說(shuō),當(dāng)三十歲的女孩Amishi宣布前進(jìn)時(shí),她只是與我的妻子而不是我的朋友,我感到震驚。 分手的原因是我是男孩,女孩只能是女孩的朋友。 她從幼兒園的朋友那里學(xué)到了這種社會(huì)規(guī)范。 幾個(gè)月前,我還記得她在泳衣和雨傘中為我塑造的方式。 即便如此,她也意識(shí)到了男女之間的差異,現(xiàn)在只是她已經(jīng)學(xué)會(huì)了這種奇怪的社會(huì)規(guī)范。 這里的重點(diǎn)是,幼兒可以毫不費(fèi)力地區(qū)分性別。 大自然給了我們一個(gè)內(nèi)置的方程式,通過高度精確的一瞥來(lái)對(duì)性別進(jìn)行分類。 想象一下類似的機(jī)制來(lái)區(qū)分好的和壞的借款人。 你在談?wù)撁總€(gè)銀行家的夢(mèng)想。 然而,進(jìn)化訓(xùn)練我們交配不放貸。
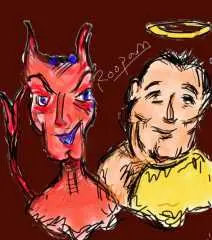
Predictive Analytics: Classification Problem – by Roopam
As I have mentioned in the previous article, scorecards have their roots in the classification problem in statistics and data mining. The idea with most classification problems is to create a mathematical equation to distinguish dichotomous variables. These variables can only take two values such as
? Male/ Female
? Good / Bad
? Yes / No
? God / Devil
? Happy / Sad
? Sales / No Sales
The list can go on until eternity. The reason why most business problems try to model dichotomies is that it is easy to comprehend for us humans. We must appreciate that dichotomies are ?never absolute and have degrees attached to them. For example, I am 80% good and 20% bad – at least I would like to believe this. I shall keep Pareto’s 80-20 principle away from this i.e. my 20% bad is responsible for my 80% of behavior.
正如我在上一篇文章中提到的,記分卡的根源在于統(tǒng)計(jì)和數(shù)據(jù)挖掘中的分類問題。 大多數(shù)分類問題的想法是創(chuàng)建一個(gè)數(shù)學(xué)方程來(lái)區(qū)分二分變量。 這些變量只能采用兩個(gè)值,例如
?男/女
? 好壞
?是/否
?上帝/魔鬼
?快樂/悲傷
?銷售/無(wú)銷售
這份清單可以持續(xù)到永恒。 大多數(shù)商業(yè)問題試圖模擬二分法的原因是它很容易理解我們?nèi)祟悺?我們必須明白,二分法從來(lái)都不是絕對(duì)的,是有度的。?例如,我80%好,20%壞 - 至少我想相信這一點(diǎn)。 我將保持帕累托的80-20原則遠(yuǎn)離這一點(diǎn),即我的20%不好對(duì)我80%的行為負(fù)責(zé)。
Credit Scorecards Development?–?Problem Statement & Sampling(壞客戶定義是靈活的)
In the case of credit scorecards, the problem statement is to distinguish analytically between the good and bad borrowers. Hence, the first task is to define a good and a bad borrower. For most loan products, good and bad credit is defined in the following way
1. Good loan: never or once missed on the EMI payment
2. Bad loan: ever missed 3 consecutive EMIs in a row (i.e. 90 days-past-due)
Additionally, for tagging someone good or bad, you need to observe his or her behavior for a significant length of time. This length of time varies from product to product based on the tenor of the loan. For home loans, with a tenor of 20 years, 2-3 years is a reasonable observation period.
However, there is nothing sacrosanct about the above definition and can be modified at the discretion of the analyst. Roll-rate analysis and vintage analysis are the two analytical tools you may want to consider while constructing the above definition.
信用記分卡開發(fā) - 問題陳述和抽樣
在信用記分卡的情況下,問題陳述是在好的和壞的借款人之間進(jìn)行分析。因此,第一個(gè)任務(wù)是定義一個(gè)好的和壞的借款人。對(duì)于大多數(shù)貸款產(chǎn)品,信用良好和不良以下列方式定義
1.良好的貸款:永遠(yuǎn)或曾一次逾期
2.不良貸款:連續(xù)3次錯(cuò)過EMI(即90天過期)
此外,為了標(biāo)記好人或壞人,你需要在很長(zhǎng)一段時(shí)間內(nèi)觀察他或她的行為。根據(jù)貸款期限,這段時(shí)間因產(chǎn)品而異。對(duì)于房屋貸款,期限為20年,2 - 3年是合理的觀察期。
但是,對(duì)于上述定義沒有什么神圣不可侵犯的,可以由分析師自行決定修改。滾動(dòng)率分析和復(fù)古分析是您在構(gòu)建上述定義時(shí)可能需要考慮的兩種分析工具。
Sampling Strategy for Credit Scorecards
A few years ago, I did a daylong workshop on Statistical Inference for a large German shipping & cargo company in Mumbai. At the time of Q&A session the Vice President of operations asked a tricky question, what is a good sample size to achieve good precision? He was looking for a one-size-fits-all answer and I wish it were that simple. The sample size depends on the degree of similarity or homogeneity of the population in question. For example, what do you think is a good sample size to answer the following two questions?
1. What is the salinity of the Pacific Ocean?
2. Is there another planet with intelligent life in the Universe?
In terms of population size, a number of drops in the ocean and planets in the Universe is similar. A couple of drops of water are enough to answer the first question since the salinity of oceans is fairly constant. On the other hand, the second question is a black swan problem. You may need to visit every single planet to rule our possibility of an intelligent form of life.
For credit scorecard development, the accepted rule of thumb for sample size is at least 1000 records of both good and bad loans. There is no reason why you cannot build a scorecard with a smaller sample size (say 500 records). However, the analyst needs to be cautious in doing so because a higher degree of randomness creeps in a small data sample. Additionally, it is also advisable to keep the sample window as short as possible i.e. a financial quarter or two while scorecard development. Further, the sample is divided into two pieces – usually, 70 % for development and remaining for validation sample. We discuss the development and validation sample in detail in the subsequent sections of this series.
信用記分卡的抽樣策略
幾年前,我為孟買的一家大型德國(guó)航運(yùn)和貨運(yùn)公司舉辦了為期一天的統(tǒng)計(jì)推斷研討會(huì)。在問答環(huán)節(jié)時(shí),運(yùn)營(yíng)副總裁提出了一個(gè)棘手的問題,即獲得良好精度的樣本量是多少?他正在尋找一個(gè)通用的答案,我希望它很簡(jiǎn)單。樣本量取決于所討論的群體的相似程度或同質(zhì)性。例如,您認(rèn)為回答以下兩個(gè)問題的樣本量是多少?
1.太平洋的鹽度是多少?
2.宇宙中還有另一個(gè)擁有智慧生命的星球嗎?
就人口規(guī)模而言,宇宙中海洋和行星的數(shù)量下降是相似的。由于海洋的鹽度相當(dāng)穩(wěn)定,幾滴水足以回答第一個(gè)問題。另一方面,第二個(gè)問題是黑天鵝問題。您可能需要訪問每個(gè)星球來(lái)統(tǒng)治我們生活的智能生活的可能性。
對(duì)于信用記分卡開發(fā),樣本大小的公認(rèn)經(jīng)驗(yàn)法則是至少1000個(gè)好的和壞的貸款記錄。沒有理由不能建立樣本量較小的記分卡(比如500條記錄)。但是,分析師需要謹(jǐn)慎行事,因?yàn)檩^小程度的隨機(jī)性會(huì)在小數(shù)據(jù)樣本中蔓延。此外,還建議盡可能縮短樣本窗口,即在記分卡開發(fā)時(shí)用一個(gè)或兩個(gè)季度數(shù)據(jù)。此外,樣品分為兩部分 - 通常70%用于顯影,剩余用于驗(yàn)證樣品。我們將在本系列的后續(xù)章節(jié)中詳細(xì)討論開發(fā)和驗(yàn)證示例。
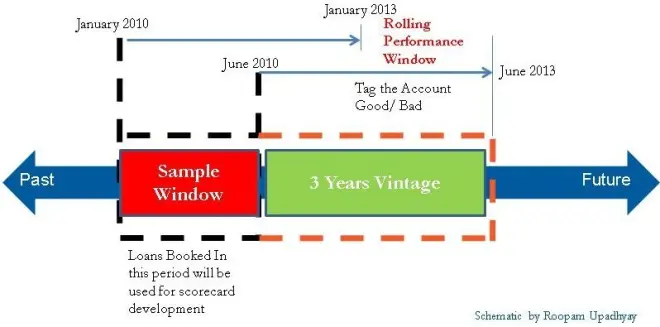
Credit Scorecard Development: Sampling Strategy – by Roopam
Sign-off Note
In the next article, we will discuss an important topic of variables classing and coarse classing for credit scorecards. See you soon
Credit Scorecards – Variables Selection (part 3 of 7)
?http://ucanalytics.com/blogs/credit-scorecards-variables-selection-part-3/
Variables Selection in Predictive Analytics
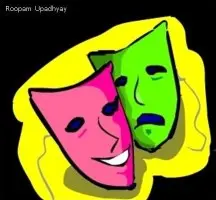
Predictive Analytics: Variables Selection – by Roopam
The following story goes back to the time when I just started my transition from physics to business. I met this investment banker* in his mid-thirties during a Friday night party. After gulping down a few pints of beer, his mood became a bit somber and he told me how he hates his job. However, he had a plan of working his ass off until he retires at 45. Then he will do everything that makes him happy. I was thoroughly confused, how could someone debar himself from an emotion – happiness – for so many years and rediscover it later? I was wondering about the recipe for happiness –?raindrops on roses and whiskers on kittens. An individual’s happiness is a tricky thing; however, I shall attempt to tackle this issue in my later article on logistic regression. For now, let us try to explore how states measure the collective well-being of their people. I shall use this topic of population well-being to explore an interesting topic in analytical scorecard development: variables selection.
以下故事可以追溯到我剛開始從物理到商業(yè)的過渡時(shí)期。 我在周五晚上的聚會(huì)期間遇到了這位投資銀行家*。 在喝了幾品脫啤酒之后,他的心情變得有些憂郁,他告訴我他是如何討厭自己的工作的。 然而,他有一個(gè)計(jì)劃工作他的屁股,直到他在45退休。然后他會(huì)做一切讓他開心的事情。 我徹底搞糊涂了,這么多年以后,有多少人會(huì)從情感 - 快樂中貶低自己,并在以后重新發(fā)現(xiàn)它? 我想知道快樂的秘訣 - 玫瑰上的雨滴和小貓的胡須。 個(gè)人的幸福是一件棘手的事情; 但是,我將在后面關(guān)于邏輯回歸的文章中嘗試解決這個(gè)問題。 現(xiàn)在,讓我們?cè)囍接懜鲊?guó)如何衡量其人民的集體福祉。 我將利用這個(gè)人口福祉主題來(lái)探索分析記分卡開發(fā)中的一個(gè)有趣話題:
Variables Selection – Lessons from GDP & GNH
The most popular measure for national prosperity, unanimously projected by economists and TV channels, is Gross Domestic Product (GDP). The equation for measuring GDP as taught in macroeconomics 101 is:
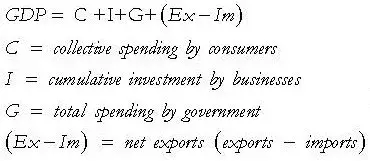
Clearly, there are 5 factors/variables that govern GDP according to this equation. The first look at GDP as a measure for national well-being seemed incomplete to me. All the variables for GDP were from commerce. They are important but cannot be the only factors for country’s well-being, more so in a highly diverse & complicated country like India.
ariables Selection - 來(lái)自GDP和GNH的經(jīng)驗(yàn)教訓(xùn)
經(jīng)濟(jì)學(xué)家和電視頻道一致預(yù)測(cè)的最受國(guó)民興趣的衡量標(biāo)準(zhǔn)是國(guó)內(nèi)生產(chǎn)總值(GDP)。 宏觀經(jīng)濟(jì)學(xué)101中教授的衡量GDP的等式是:
GDP方程式
顯然,根據(jù)這個(gè)等式,有5個(gè)因素/變量可以控制GDP。 首先將國(guó)內(nèi)生產(chǎn)總值視為衡量國(guó)家福祉的指標(biāo)對(duì)我來(lái)說(shuō)似乎不完整。 GDP的所有變量都來(lái)自商業(yè)。 它們很重要,但不能成為國(guó)家福祉的唯一因素,在印度等高度多樣化和復(fù)雜的國(guó)家更是如此。
Gross National Happiness Index – The Story of Bhutan Naresh
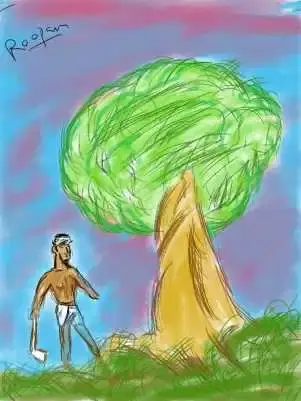
Variables Selection – by Roopam
Ok, so what else do we have? A lesser-known index is Gross National Happiness (GNH). The origins of GNH are in Bhutan. They measure their country’s progress through GNH. The term was coined and implemented by Jigme Singye Wangchuck. This name immediately takes me back to the early nineties live telecast of the SAARC summit by India’s national broadcaster Doordarshan (DD). The old-timer Hindi commentators were referring to a modest man in a bathrobe-like-attire as ‘Bhutan Naresh’ – King of Bhutan. At first glance, he did not fit well with the power horses of the south Asian region. Nevertheless, he seems to have devised a more holistic metric to?measure his country’s well-being. GNH is a combination of the following broad categories:
1. Living standard & income
2. Health coverage
3. Physiological well-being
4. Time spent at work and relaxing
5. Good governance
6. Schooling & education
7. Cultural diversity
8. Community vitality
9. Environmentalism and conservatism
There are 72 total variables in GNH measured on a scale of 0 to 1, such as daily hours of sleep and trust in media; hmmm, not a bad start! You could do your own research on GNH and let me know what you feel about it. Actually, we can work out our own formula for a GNH like metric. The idea is to select the right variables to build your model!
國(guó)民幸福總指數(shù) - 不丹納雷什的故事
變量選擇 - 由Roopam
好的,那我們還有什么呢?一個(gè)鮮為人知的指數(shù)是國(guó)民幸??傊担℅NH)。 GNH的起源在不丹。他們通過GNH衡量他們國(guó)家的進(jìn)步。該術(shù)語(yǔ)由Jigme Singye Wangchuck創(chuàng)造和實(shí)施。這個(gè)名字讓我回到了印度國(guó)家廣播公司Doordarshan(DD)在九十年代早期的SAARC峰會(huì)現(xiàn)場(chǎng)直播。舊時(shí)的印地語(yǔ)評(píng)論員指的是一個(gè)穿著浴衣般裝扮的謙虛男人,就像不丹之王“不丹納雷什”。乍一看,他并不適合南亞地區(qū)的動(dòng)力馬。然而,他似乎已經(jīng)設(shè)計(jì)了一個(gè)更全面的衡量標(biāo)準(zhǔn)來(lái)衡量他的國(guó)家的福祉。 GNH是以下大類的組合:
1.生活水平和收入
2.健康保險(xiǎn)
3.生理健康
4.工作和放松的時(shí)間
5.善治
6.學(xué)校教育
7.文化多樣性
8.社區(qū)活力
9.環(huán)境保護(hù)主義和保守主義
GNH中有72個(gè)總變量,按0到1的等級(jí)測(cè)量,例如每天的睡眠時(shí)間和對(duì)媒體的信任;嗯,這不是一個(gè)糟糕的開始!你可以自己研究GNH,讓我知道你對(duì)它的看法。實(shí)際上,我們可以為GNH度量標(biāo)準(zhǔn)制定出我們自己的公式。我們的想法是選擇正確的變量來(lái)構(gòu)建您的模型!
Variables Selection in Credit Scoring
In data mining and statistical model building exercises, similar to credit scoring, variables selection process is performed through statistical significance – a reasonably automated process through advanced software. However, the variables are still created and measured by humans. High impact analyses in businesses are still driven by hunches. Human intelligence is not obsolete yet.
In one of the projects I did with a financial organization, the result of credit risk analysis and scoring led to redesigning of the application form. Application forms are a major source of data collection regarding the borrower. However, nobody wants to fill a lengthy form hence an optimal size of the form ensures accurate information provided by the borrower. The idea is to select the right variable and ensure accurate measurement.
There are several aspects regarding variables but I will mention just one of them here (coarse classing).
信用評(píng)分中的變量選擇
在數(shù)據(jù)挖掘和統(tǒng)計(jì)模型構(gòu)建練習(xí)中,類似于信用評(píng)分,變量選擇過程通過統(tǒng)計(jì)顯著性來(lái)執(zhí)行 - 通過高級(jí)軟件進(jìn)行合理自動(dòng)化的過程。 但是,變量仍由人類創(chuàng)造和測(cè)量。 企業(yè)的高影響力分析仍然受到預(yù)感的驅(qū)動(dòng)。 人類智慧尚未過時(shí)。
在我與金融機(jī)構(gòu)合作的一個(gè)項(xiàng)目中,信用風(fēng)險(xiǎn)分析和評(píng)分的結(jié)果導(dǎo)致了申請(qǐng)表的重新設(shè)計(jì)。 申請(qǐng)表是有關(guān)借款人的主要數(shù)據(jù)收集來(lái)源。 然而,沒有人想要填寫冗長(zhǎng)的表格,因此表格的最佳尺寸確保了借款人提供的準(zhǔn)確信息。 我們的想法是選擇正確的變量并確保準(zhǔn)確的測(cè)量。
關(guān)于變量有幾個(gè)方面,但我在這里只提到其中一個(gè)(粗略分類)。
Coarse Classing in Credit Scoring
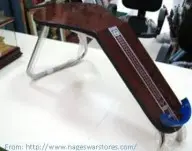
One of my favorite activities as a kid was going to a shoe store and getting my feet measured every summer before the school started. The shoe shops had a strange, miniature, slide-like device to measure foot size. It was fun to see my feet grow from one size to another every year or two. The growth was quantized i.e you are size-2 or 3 never 2.5 or 2.7. This aspect of converting measure such as 2.5 & 2.7 to 3 is called grouping, bucketing or classing. This is an integral part of creating scorecards that you will find in all the books I have listed in the first part of this blog series.
I have been a part of several heated discussions on the relevance of coarse class in scorecard development throughout my career. In most, if not all academic articles you will rarely see coarse classing as a technique during model development. Quite a few academicians & practitioners for a good reason believe that coarse classing results in loss of information. However, in my opinion, coarse classing has the following advantage over using raw measurement for a variable.
1. It reduces random noise that exists in raw variables – similar to averaging and yes, you lose some information here.
2. It handles extreme events – on two extremes of a variable – much better where you have thin data.
3. It handles the non-linear relationship between dependent and independent variable without a lot of effort of variable transformation from the analyst.
信用評(píng)分中的粗分類
3鞋子測(cè)量我小時(shí)候最喜歡的一項(xiàng)活動(dòng)是去一家鞋店,每年夏天在學(xué)校開始前測(cè)量我的腳。這些鞋店有一個(gè)奇怪的,微型的滑動(dòng)式設(shè)備來(lái)測(cè)量腳的大小。每年或每?jī)赡昕吹轿业哪_從一個(gè)尺寸增長(zhǎng)到另一個(gè)尺寸很有趣。增量被量化,即你的大小為2或3從不2.5或2.7。將諸如2.5和2.7之類的度量轉(zhuǎn)換為3的這一方面稱為分組,分組或分類。這是創(chuàng)建記分卡的一個(gè)組成部分,您可以在本博客系列的第一部分列出的所有書籍中找到這些記分卡。
在我的職業(yè)生涯中,我參與了幾個(gè)關(guān)于粗俗課程在記分卡開發(fā)中的相關(guān)性的熱烈討論。在大多數(shù)情況下,如果不是所有的學(xué)術(shù)文章,你很少會(huì)在模型開發(fā)過程中看到粗略的分類。相當(dāng)多的學(xué)者和從業(yè)者有充分理由相信粗略的分類會(huì)導(dǎo)致信息丟失。但是,在我看來(lái),粗略分類比使用變量的原始測(cè)量具有以下優(yōu)勢(shì)。
1.它減少了原始變量中存在的隨機(jī)噪聲 - 類似于平均值,是的,你在這里丟失了一些信息。
它處理極端事件 - 在變量的兩個(gè)極端情況下 - 在您擁有精簡(jiǎn)數(shù)據(jù)的情況下更好。
3.它處理依賴變量和自變量之間的非線性關(guān)系,而無(wú)需分析師進(jìn)行變量轉(zhuǎn)換。
Sign-off Note?
We are half way through this series on ‘Analytical Scorecard Development’ and I am enjoying writing this thoroughly. I hope as a reader you are on the same page. Scorecard building is highly technical and I have tried to discuss some aspects with easy to understand examples. However, to manage the length of the article, I am not able to get into the details. I must say that I love the details! So, if you have any queries, doubts, points-of-view or recommendations please write back on the discussion board or on my email:?roopam.up@gmail.com
參考http://ucanalytics.com/blogs/credit-scorecards-part-1/
博主網(wǎng)校主頁(yè)
?https://study.163.com/provider/400000000398149/index.htm?share=2&shareId=400000000398149
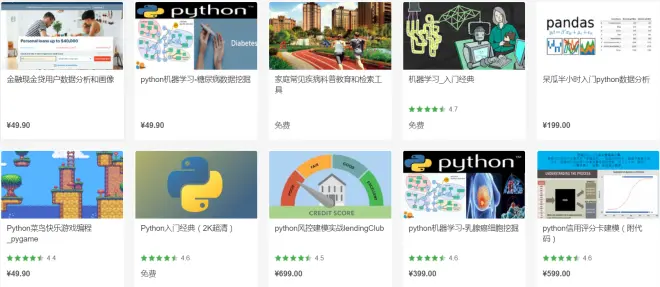