R語言可視化探索BRFSS數(shù)據(jù)并邏輯回歸Logistic回歸預測中風
原文鏈接:http://tecdat.cn/?p=9195
?
加載包
library(ggplot2)
載入資料
load("brfss2013.RData")
第1部分:關于數(shù)據(jù)
行為風險因素監(jiān)視系統(tǒng)(BRFSS)是美國的年度電話調(diào)查。BRFSS旨在識別成年人口中的危險因素并報告新興趨勢。例如,詢問受訪者飲食和每周的體育鍛煉,艾滋病毒/艾滋病狀況,可能的煙草使用,免疫接種,健康狀況,健康天數(shù)-與健康相關的生活質(zhì)量,獲得醫(yī)療保健,睡眠不足,高血壓意識,膽固醇意識,慢性健康狀況,飲酒,水果和蔬菜消費,關節(jié)炎負擔和安全帶使用。
數(shù)據(jù)采集:
數(shù)據(jù)收集過程在brfss_codebook中進行了說明。通過進行座機電話調(diào)查和基于蜂窩電話的調(diào)查,從美國所有50個州,哥倫比亞特區(qū),波多黎各,關島和美屬薩摩亞,密克羅尼西亞聯(lián)邦和帕勞收集了數(shù)據(jù)。固定電話樣本已使用了不成比例的分層抽樣(DSS),并且隨機選擇了蜂窩電話受訪者,每個受訪者具有相同的選擇概率。我們正在處理的數(shù)據(jù)集包含330個變量,2013年共進行491、775次觀測。缺失值用“ NA”表示。
推廣性:
樣本數(shù)據(jù)應使我們能夠推廣到感興趣的人群。它是對491,775名18歲以上美國成年人的調(diào)查。它基于大量分層的隨機樣本。潛在偏見與無回應,不完整的訪談,價值觀缺失和便利偏見有關。
因果關系:
BRFSS是一項觀察研究,只能建立變量之間的相關性/關聯(lián)性,因此無法建立因果關系。
第2部分:研究問題
研究問題1:
在過去30天內(nèi),身心健康狀況不佳的天數(shù)分布是否因性別而異?
研究問題2:
受訪者接受采訪的月份與受訪者自我報告的健康感知之間是否存在關聯(lián)?
研究問題3:
收入和醫(yī)療保險之間有關聯(lián)嗎?
研究問題4:
吸煙,飲酒,膽固醇,血壓,體重和中風之間是否有任何關系?最終,我想看看是否可以通過上述變量預測中風。
第3部分:探索性數(shù)據(jù)分析
研究問題1:
ggplot(aes(x=physhlth, fill=sex), data = brfss2013[!is.na(brfss2013$sex), ]) +
geom_histogram(bins=30, position = position_dodge()) + ggtitle('Number of Days Physical Health not Good in the Past 30 Days')
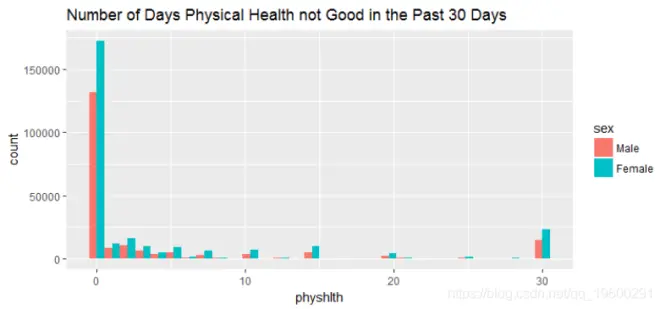
?
ggplot(aes(x=menthlth, fill=sex), data=brfss2013[!is.na(brfss2013$sex), ]) +
geom_histogram(bins=30, position = position_dodge()) + ggtitle('Number of Days Mental Health not Good in the Past 30 Days')
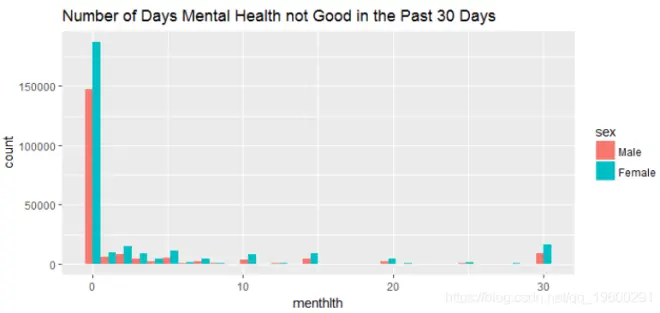
?
ggplot(aes(x=poorhlth, fill=sex), data=brfss2013[!is.na(brfss2013$sex), ]) +
geom_histogram(bins=30, position = position_dodge()) + ggtitle('Number of Days with Poor Physical Or Mental Health in the Past 30 Days')
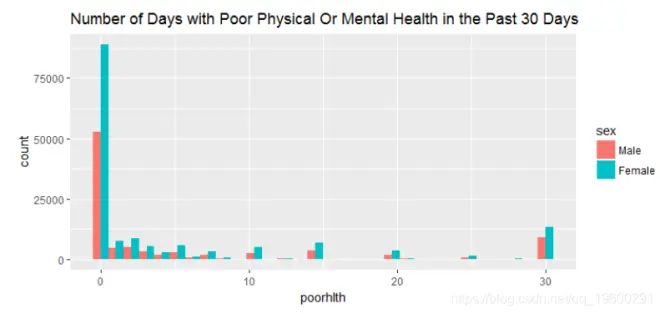
?
summary(brfss2013$sex)
## ?Male ?Female ? NA's
##201313 290455 ? ? ?7
以上三個數(shù)字顯示了過去30天內(nèi)男性和女性對身體,精神和健康狀況不佳的天數(shù)做出反應的數(shù)據(jù)分布。我們可以看到,女性受訪者比男性受訪者要多得多。
研究問題2:
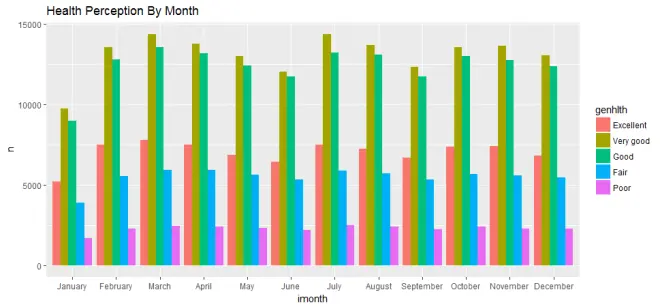
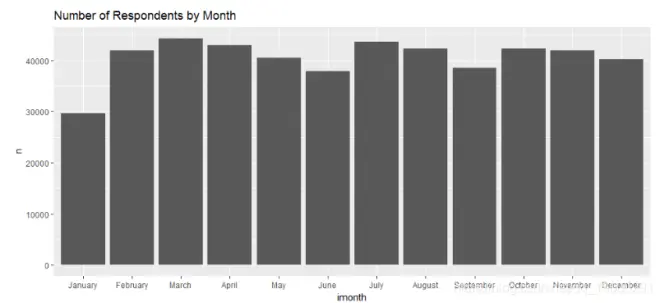
?
?
我試圖找出人們在不同月份對健康狀況的反應是否不同。例如,人們是否更有可能說自己在春季或夏季身體健康?
研究問題3:?
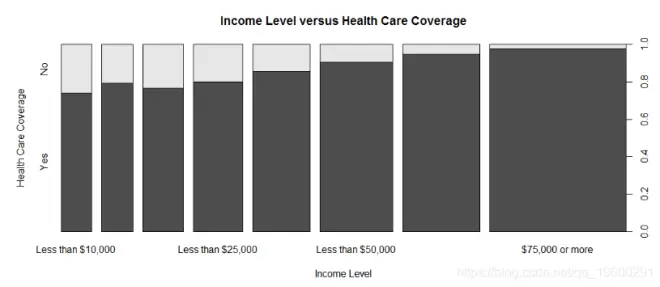
?
?
一般而言,高收入受訪者比低收入受訪者更有可能獲得醫(yī)療保健。
研究問題4:
為了回答這個問題,我將使用以下變量:
smoke100:抽至少100支香煙
avedrnk2:過去30天每天平均含酒精飲料
bphigh4:曾經(jīng)血壓過高
tellhi2:高膽固醇血癥
weight2:報告的磅數(shù)
cvdstrk3:曾經(jīng)被診斷為中風
首先,將上述變量轉換為數(shù)字,并查看這些數(shù)字變量之間的相關性。
corr.matrix <- cor(selected_brfss)
corrplot(corr.matrix, main="\n\nCorrelation Plot of Smoke, Alcohol, Blood pressure, Cholesterol, and Weight", method="number")
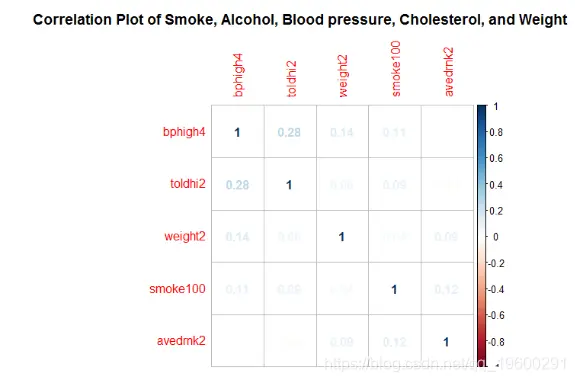
?
似乎沒有任何兩個數(shù)字變量具有很強的相關性。
Logistic回歸預測中風
將答案“是,但女性僅在懷孕期間告知”和“告訴臨界點或高血壓前”回答為“是”。
將“ NA”值替換為“否”。
stroke$bphigh4 <- replace(stroke$bphigh4, which(is.na(stroke$bphigh4)), "No")
stroke$toldhi2 <- replace(stroke$toldhi2, which(is.na(stroke$toldhi2)), "No")
stroke$cvdstrk3 <- replace(stroke$cvdstrk3, which(is.na(stroke$cvdstrk3)), "No")
stroke$smoke100 <- replace(stroke$smoke100, which(is.na(stroke$smoke100)), 'No')
平均替換“ NA”值。
mean(stroke$avedrnk2,na.rm = T)
##[1] 2.209905
stroke$avedrnk2 <- replace(stroke$avedrnk2, which(is.na(stroke$avedrnk2)), 2)
看一下將用于建模的數(shù)據(jù)。
head(stroke)
summary(stroke)
## ? bphigh4 toldhi2 cvdstrk3 weight2 smoke100 avedrnk2
##1 ? ? Yes ? ? Yes ? ? ? No ? ? 154 ? ? ?Yes ? ? ? ?2
##2 ? ? ?No ? ? ?No ? ? ? No ? ? ?30 ? ? ? No ? ? ? ?2
##3 ? ? ?No ? ? ?No ? ? ? No ? ? ?63 ? ? ?Yes ? ? ? ?4
##4 ? ? ?No ? ? Yes ? ? ? No ? ? ?31 ? ? ? No ? ? ? ?2
##5 ? ? Yes ? ? ?No ? ? ? No ? ? 169 ? ? ?Yes ? ? ? ?2
##6 ? ? Yes ? ? Yes ? ? ? No ? ? 128 ? ? ? No ? ? ? ?2
## ?bphigh4 ? ? ?toldhi2 ? ? ?cvdstrk3 ? ? ? ?weight2 ? ? ? smoke100
## No :284107 ? Yes:183501 ? Yes: 20391 ? Min. ? : ?1.00 ? Yes:215201
## Yes:207668 ? No :308274 ? No :471384 ? 1st Qu.: 43.00 ? No :276574
## ? ? ? ? ? ? ? ? ? ? ? ? ? ? ? ? ? ? ? ?Median : 73.00
## ? ? ? ? ? ? ? ? ? ? ? ? ? ? ? ? ? ? ? ?Mean ? : 80.22
## ? ? ? ? ? ? ? ? ? ? ? ? ? ? ? ? ? ? ? ?3rd Qu.:103.00
## ? ? ? ? ? ? ? ? ? ? ? ? ? ? ? ? ? ? ? ?Max. ? :570.00
## ? ?avedrnk2
## Min. ? : 1.000
## 1st Qu.: 2.000
## Median : 2.000
## Mean ? : 2.099
## 3rd Qu.: 2.000
## Max. ? :76.000
二進制結果。
在整理和清理數(shù)據(jù)之后,現(xiàn)在我們可以擬合模型。
Logistic回歸模型擬合
summary(model)
##Call:
##glm(formula = cvdstrk3 ~ ., family = binomial(link = "logit"),
## ? ?data = train)
##Deviance Residuals:
## ? ?Min ? ? ? 1Q ? Median ? ? ? 3Q ? ? ?Max
##-0.5057 ?-0.3672 ?-0.2109 ?-0.1630 ? 3.2363
##Coefficients:
## ? ? ? ? ? ? ?Estimate Std. Error ?z value Pr(>|z|)
##(Intercept) -3.2690106 ?0.0268240 -121.869 ?< 2e-16 ***
##bphigh4Yes ? 1.3051850 ?0.0193447 ? 67.470 ?< 2e-16 ***
##toldhi2No ? -0.5678048 ?0.0171500 ?-33.108 ?< 2e-16 ***
##weight2 ? ? -0.0009628 ?0.0001487 ? -6.476 9.41e-11 ***
##smoke100No ?-0.3990598 ?0.0163896 ?-24.348 ?< 2e-16 ***
##avedrnk2 ? ?-0.0274511 ?0.0065099 ? -4.217 2.48e-05 ***
##---
##Signif. codes: ?0 '***' 0.001 '**' 0.01 '*' 0.05 '.' 0.1 ' ' 1
##(Dispersion parameter for binomial family taken to be 1)
## ? ?Null deviance: 136364 ?on 389999 ?degrees of freedom
##Residual deviance: 126648 ?on 389994 ?degrees of freedom
##AIC: 126660
##Number of Fisher Scoring iterations: 6
解釋我的邏輯回歸模型的結果:
所有變量均具有統(tǒng)計學意義。
所有其他變量都相等,被告知血壓升高,更可能發(fā)生中風。
預測變量的負系數(shù)-tellhi2No表示,所有其他變量相等,沒有被告知血液中膽固醇水平較高,則發(fā)生中風的可能性較小。
每單位重量改變,具有沖程(相對于無沖程)的對數(shù)幾率降低0.00096。
至少抽100支香煙不抽煙,中風的可能性較小。
在過去30天內(nèi),每天平均含酒精飲料增加1個單位,中風的對數(shù)幾率降低0.027。
anova(model, test="Chisq")
##Analysis of Deviance Table
##Model: binomial, link: logit
##Response: cvdstrk3
##Terms added sequentially (first to last)
## ? ? ? ? Df Deviance Resid. Df Resid. Dev ?Pr(>Chi)
##NULL ? ? ? ? ? ? ? ? ? ?389999 ? ? 136364
##bphigh4 ? 1 ? 7848.6 ? ?389998 ? ? 128516 < 2.2e-16 ***
##toldhi2 ? 1 ? 1230.1 ? ?389997 ? ? 127285 < 2.2e-16 ***
##weight2 ? 1 ? ? 33.2 ? ?389996 ? ? 127252 8.453e-09 ***
##smoke100 ?1 ? ?584.5 ? ?389995 ? ? 126668 < 2.2e-16 ***
##avedrnk2 ?1 ? ? 19.9 ? ?389994 ? ? 126648 7.958e-06 ***
##---
##Signif. codes: ?0 '***' 0.001 '**' 0.01 '*' 0.05 '.' 0.1 ' ' 1
分析偏差表,可以看到一次添加每個變量時偏差的下降。添加bphigh4,tellhi2,smoke100會大大減少殘留偏差。盡管其他變量weight2和avedrnk2都具有較低的p值,但它們似乎對模型的改進較少。
評估模型的預測能力
##[1] "Accuracy 0.961296978629329
測試裝置上的0.96精度是非常好的結果。
繪制ROC曲線并計算AUC(曲線下的面積)
auc
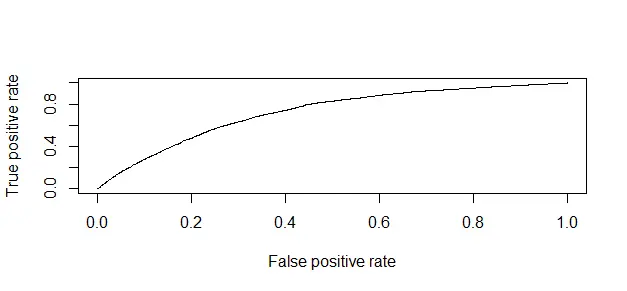
##[1] 0.7226642
最后一點,當我們分析健康狀況監(jiān)測數(shù)據(jù)時,我們必須意識到自我報告的患病率可能會有偏差,因為受訪者可能不知道其風險狀況。因此,為了獲得更精確的估計,研究人員正在使用實驗室測試以及自我報告的數(shù)據(jù)。請選中你要保存的內(nèi)容,粘貼到此文本框