改進(jìn)的侏儒貓鼬優(yōu)化算法(IDMO)附matlab代碼
?作者簡(jiǎn)介:熱愛科研的
開發(fā)者,修心和技術(shù)同步精進(jìn),matlab項(xiàng)目合作可私信。??個(gè)人主頁(yè):Matlab科研工作室
??個(gè)人信條:格物致知。
? 內(nèi)容介紹
This paper proposes a new metaheuristic algorithm called dwarf?
?optimization algorithm (DMO) to solve the classical and CEC 2020 benchmark functions and 12 continuous/discrete engineering optimization problems. The DMO mimics the foraging behavior of the dwarf mongoose. The restrictive mode of prey capture (feeding) has dramatically affected the mongooses' social behavior and ecological adaptations to compensate for efficient family nutrition. The compensatory behavioral adaptations of the mongoose are prey size, space utilization, group size, and food provisioning. Three social groups of the dwarf mongoose are used in the proposed algorithm, the alpha group, babysitters, and the scout group. The family forage as a unit, and the alpha female initiates foraging, determines the foraging path, the distance covered, and the sleeping mounds. A certain number of the mongoose population (usually a mixture of males and females) serve as the babysitters. They remain with the young until the group returns at midday or evening. The babysitters are exchanged for the first to forage with the group (exploitation phase). The dwarf mongooses do not build a nest for their young; they move them from one sleeping mound to another and do not return to the previously foraged site. The dwarf mongoose has adopted a seminomadic way of life in a territory large enough to support the entire group (exploration phase). The nomadic behavior prevents overexploitation of a particular area. It also ensures exploration of the whole territory because no previously visited sleeping mound is returned. The performance of the proposed DMO algorithm is compared with seven other algorithms to show its effectiveness in terms of different performance metrics and statistics. In most cases, the near-optimal solutions achieved by the DMO are better than the best solutions obtained by the current state-of-the-art algorithms.? 部分代碼
%_______________________________________________________________________________________%
%? Dwarf Mongoose?
?Algorithm source codes (version 1.0)? ? ? ? ? ? ? ? ? ? ?%%? ? ? ? ? ? ? ? ? ? ? ? ? ? ? ? ? ? ? ? ? ? ? ? ? ? ? ? ? ? ? ? ? ? ? ? ? ? ? ? ? ? ? ?%
%? Developed in MATLAB R2015a (7.13)? ? ? ? ? ? ? ? ? ? ? ? ? ? ? ? ? ? ? ? ? ? ? ? ? ? %
clear all?
clc
Solution_no=50;? % Number of search agents
F_name='F1';? % Name of the test function that can be from F1 to F23
M_Iter=200;? % Maximum numbef of iterations??
[LB,UB,Dim,F_obj]=Get_F(F_name);?
[Best_FF,Best_P,conv]=IDMO(Solution_no,M_Iter,LB,UB,Dim,F_obj);??
figure('Position',[200? ? ? ? ?300? ? ? ? 770? ? ? ? ?267])
subplot(1,2,1);
func_plot(F_name);
title('Parameter space')
xlabel('x_1');
ylabel('x_2');
zlabel([F_name,'( x_1 , x_2 )'])
box on
axis tight
axis square
subplot(1,2,2);
semilogy(conv,'Color','r','LineWidth',1.5)
title('Convergence curve')
xlabel('Iteration#');
ylabel('Best score obtained so far');
box on
axis tight
axis squar
display(['The best-obtained solution by IDMO is : ', num2str(Best_P)]);
display(['The best optimal values of the objective funciton found by IDMO is : ', num2str(Best
? 運(yùn)行結(jié)果
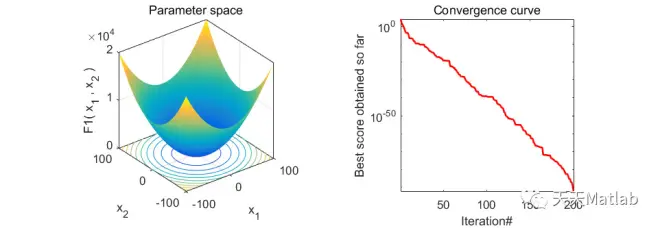
? 參考文獻(xiàn)
[1] Agushaka J O ,? Ezugwu A E ,? Abualigah L . Dwarf Mongoose Optimization Algorithm[J]. Computer Methods in Applied Mechanics and Engineering, 2022(Mar.1):391.
? 完整代碼
??部分理論引用網(wǎng)絡(luò)文獻(xiàn),若有侵權(quán)聯(lián)系博主刪除
?? 關(guān)注我領(lǐng)取海量matlab電子書和數(shù)學(xué)建模資料
?