干貨 | 基于PIE-Engine AI的UperNet-SwinTransformer模型上傳實(shí)踐——以光伏目標(biāo)提取
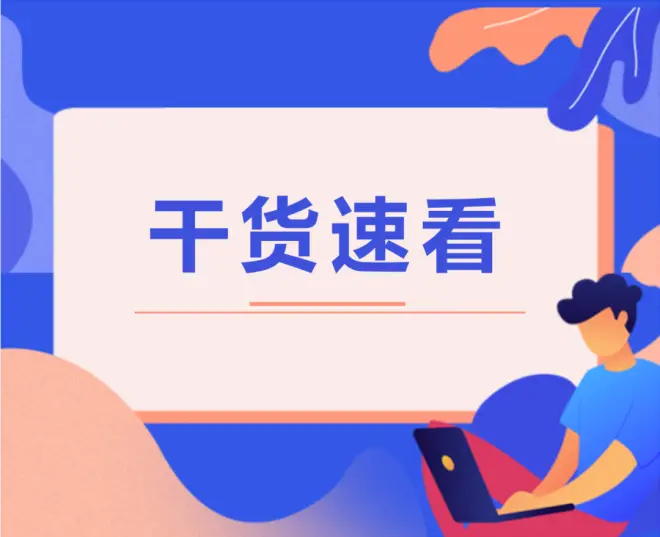
PIE-Engine AI 介紹
PIE-Engine AI 是航天宏圖自主研發(fā)的一站式遙感圖像智能解譯服務(wù)云平臺。該平臺結(jié)合空間信息技術(shù)和人工智能技術(shù),將深度學(xué)習(xí)理論與遙感解譯實(shí)踐進(jìn)行融合,全方位提升遙感數(shù)據(jù)智能化處理和解譯分析能力??蔀橛脩籼峁?shí)時(shí)、精準(zhǔn)、高效的遙感圖像在線智能解譯服務(wù),包括遙感地物語義分割、目標(biāo)檢測、變化監(jiān)測等應(yīng)用方向。
本文以 0.3m 分辨率影像光伏目標(biāo)提取為例,介紹了如何將本地訓(xùn)練的 UperNet-SwinTransformer 模型集成至 PIE-Engine AI,并實(shí)現(xiàn)智能解譯的一站式深度學(xué)習(xí)服務(wù)。
UperNet-SwinTransformer?模型簡介
//
模型概述
SwinTransformer是微軟亞洲研究院提出的新型視覺Transformer,它可以作為計(jì)算機(jī)視覺的通用骨干網(wǎng)絡(luò)。視覺領(lǐng)域與自然語言領(lǐng)域之間存在巨大差異,這帶來了使 Transformer 從自然語言領(lǐng)域適應(yīng)視覺領(lǐng)域的挑戰(zhàn)。
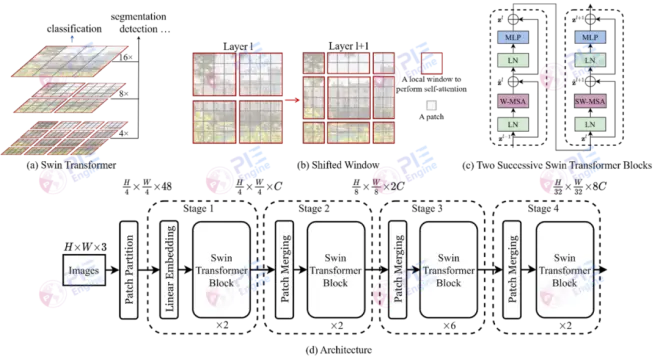
? ?為了解決這些差異,SwinTransformer 提出了一個(gè)分層的 Transformer,其表示是通過移動(dòng)窗口來計(jì)算的。通過將自注意力計(jì)算限制為不重疊的局部窗口,同時(shí)允許跨窗口連接,移位的窗口方案帶來了更高的效率。這種分層體系結(jié)構(gòu)具有在各種尺度上建模的靈活性,并且相對于圖像大小具有線性計(jì)算復(fù)雜性。SwinTransformer?的這些品質(zhì)使其可與多種視覺任務(wù)兼容。
? ?本文以 SwinTransformer 作為 UperNet 的骨干網(wǎng)絡(luò),構(gòu)建 UperNet-SwinTransformer 網(wǎng)絡(luò)進(jìn)行光伏目標(biāo)提取。采用 0.3m 分辨率的光伏語義分割數(shù)據(jù)集進(jìn)行訓(xùn)練,達(dá)到了 mIoU=96.07 的精度。
UperNet-SwinTransformer?模型上傳代碼實(shí)現(xiàn)
01
模型上傳壓縮包結(jié)構(gòu)
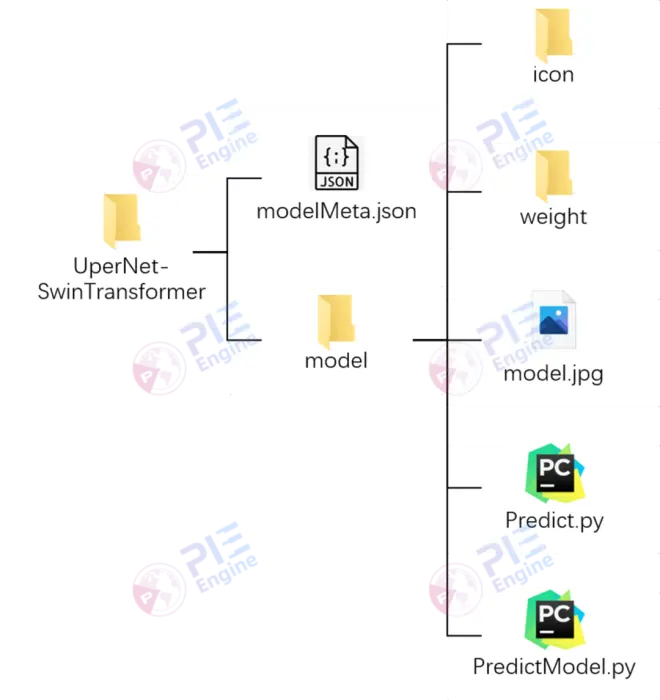
模型上傳壓縮包分為兩部分:模型描述文件和模型代碼文件夾。
1、模型描述文件
模型描述文件?modelMeta.json?包含詳細(xì)的模型信息描述,包括模型名稱、權(quán)重文件名稱、模型訓(xùn)練數(shù)據(jù)集的相關(guān)信息、網(wǎng)絡(luò)結(jié)構(gòu)相關(guān)信息、記錄圖片名稱、評價(jià)指標(biāo)等。
以下代碼給出參數(shù)說明,各參數(shù)詳細(xì)配置見示例文件。
{
?"注釋說明1":"以下為必填項(xiàng)",
?"name": "光伏提取模型",
?"weight": "pv.pth",
?"dataset": {
? ?"label":[{"name":"background","title":"背景","color":"rgb(0,0,0)","value":"0"},
? ? ?{"name":"pv","title":"光伏","color":"rgb(255,0,0)","value":"1"}],
? ?"imageSize": "1024,1024",
? ?"bands": "3",
? ?"dataType": "Byte"
?},
?"注釋說明2":"以下為選填項(xiàng)",
?"labels": "0.3m光伏",
?"description": "該模型是基于pytorch 1.7深度學(xué)習(xí)框架,使用0.3m分辨率光伏數(shù)據(jù)集進(jìn)行訓(xùn)練",
?"network": {
? ?"thumbnail": "model.jpg"
?},
?"icon":"存放模型的原始及預(yù)測圖片,圖片名稱需統(tǒng)一,詳情查看REAMDE.md",
?"evaluate":{
? ?"accuary": "98.14%",
? ?"description":{"miou": "miou"},
? ?"best":{"miou": 0.9607}
?}
}
←左右滑動(dòng)查看更多→
2、模型代碼文件夾
模型代碼文件夾?model?主要包含 icon、weight 等文件夾和Predict.py、PredictModel.py 等腳本文件,相關(guān)命名及編寫規(guī)范在示例文件進(jìn)行了說明。
??icon?文件夾存放預(yù)測模型圖片,命名規(guī)則參考 modelMeta.json,若無相關(guān)圖片展示,則刪除該文件夾。
??weight 文件夾存放權(quán)重文件,名稱需與 modelMeta.json 中完全一致。
??model.jpg?上傳網(wǎng)絡(luò)結(jié)構(gòu)圖。
??Predict.py 智能解譯腳本,主要包括輸入輸出兩部分內(nèi)容:
輸入:
image_path(圖片路徑)、weight_path(權(quán)重文件路徑)、gpu_num(使用gpu個(gè)數(shù));
輸出:
(1)語義分割、變化檢測:tif影像及對應(yīng)矢量;
(2)目標(biāo)識別:geojson目標(biāo)框文件及對應(yīng)矢量。
??PredictModel.py 模型發(fā)布腳本,主要包括輸入輸出兩部分內(nèi)容:
輸入:
image_path(圖片路徑)、weight_path(權(quán)重文件路徑)、gpu_num(使用gpu個(gè)數(shù));
輸出:
(1)語義分割、變化檢測:單波段圖片二進(jìn)制流;
(2)目標(biāo)識別:geojson目標(biāo)框二進(jìn)制流。
02
影像智能解譯?pipeline?及核心代碼實(shí)現(xiàn)
用戶需在?Predict.py 文件中編寫影像預(yù)測代碼,從而使上傳的模型能完成影像智能解譯任務(wù)。影像預(yù)測代碼需實(shí)現(xiàn)以下功能:

以下代碼給出影像預(yù)測主函數(shù)?prediction_image 的示例。
def prediction_image(**platform_arguments):
? ?
load_model = platform_arguments['load_model']
??
?deal_data_img = platform_arguments['deal_data_img']
?
?network_type = platform_arguments['network_type']
? ?
# 獲取讀取s3的gdal
??
?s3gdal = inint_gdal()
# 加載模型
? ?
model = get_model(load_model)
??
?# 獲取預(yù)測圖片列表
??
?inputList = platform_arguments['image_path'].split(',')
??
?# 記錄日志
? ?
log_new = {"zipOutFile": '/' + '/'.join(outputSaveDir.split('/')[2:]) + "result.zip", "total_output_size": "",
? ? ? ? ? ? ? "task_list": []}
? ?total_output_size = 0
? ?
# 遍歷圖片,進(jìn)行每張圖片預(yù)測
??
?for index, imagePath in enumerate(inputList):
process = float((index + 1) / len(inputList))
? ? ??
?log_new["process"] = process
? ? ??
?taskIdAndIndex = platform_arguments['estimate_id'] + ":" + str(index + 1)
? ? ? ?tmpfilename = os.path.basename(imagePath)
? ? ? ?
filename, extension = os.path.splitext(tmpfilename)
? ? ??
?# 重命名,例如:1_xxx_pred
? ? ??
?filename = str(index + 1) + "_" + filename + "_pred"
? ? ??
?# 輸出
? ? ? ?
outputFile = outputSaveDir + filename + ".tif"
? ? ??
?pred_utils = pred_prepare(model,s3gdal,imagePath)
? ? ??
?if network_type == 1 or network_type == 3:
? ? ? ? ? ?
# 滑窗預(yù)測圖片,生成三波段展示tif及臨時(shí)單波段tif
? ? ? ? ? ?pred_utils.pred_image_seg_change(outputFile, platform_arguments['load_size'],deal_data_img,platform_arguments['value_color'],platform_arguments['background_value'])
? ? ? ? ?
?# 生成金字塔及轉(zhuǎn)換為shp,并保存入數(shù)據(jù)庫
? ? ? ? ? ?
staticMap = shp_tif_deal(outputFile, pred_utils, taskIdAndIndex, **platform_arguments)
? ? ? ?elif network_type == 2:
? ? ? ? ? ?
# 預(yù)測圖片,生成 shp并生成geojson
? ? ? ? ? ?pred_utils.pred_image_detection(outputFile[:-4] + '.shp',platform_arguments['load_size'],platform_arguments['class_name_list'], deal_data_img,platform_arguments['value_title_map'],platform_arguments['name_title_map'],nms=0.3)
? ? ? ? ??
?# 存入數(shù)據(jù)庫
? ? ? ? ? ?
staticMap = shp_tif_deal(outputFile, pred_utils, taskIdAndIndex, **platform_arguments)
? ? ? ?else:
? ? ? ? ??
?print('輸入類型有誤!')
? ? ? ? ? ?exit(1)
? ? ??
?# 日志信息
? ? ??
?task_list_inner = {
? ? ? ? ? ?"task_result_id": taskIdAndIndex,
? ? ? ? ? ?"output_shp_static": staticMap,
? ? ? ? ? ?"output_size": "",
? ? ? ? ? ?"input": imagePath,
? ? ? ? ? ?"output": [],
? ? ? ?}
# 記錄日志信息
create_log(task_list_inner,outputFile,total_output_size,log_new)
? ? ? ?print("[Process: {process}]".format(process=str(index + 1) + '/' + str(len(inputList))))
? ?
# 矢量進(jìn)行壓縮
create_zip()
create_zip()
03
模型上傳到 PIE-Engine AI 平臺
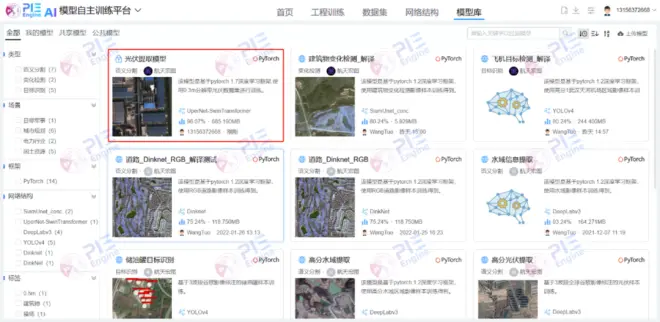
遙感影像智能解譯
//
用戶可以選擇平臺中的?UperNet-SwinTransformer?光伏提取模型,在線快速創(chuàng)建解譯任務(wù)
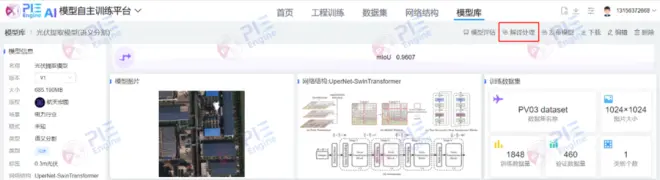
上傳待解譯評估的影像數(shù)據(jù)進(jìn)行智能解譯
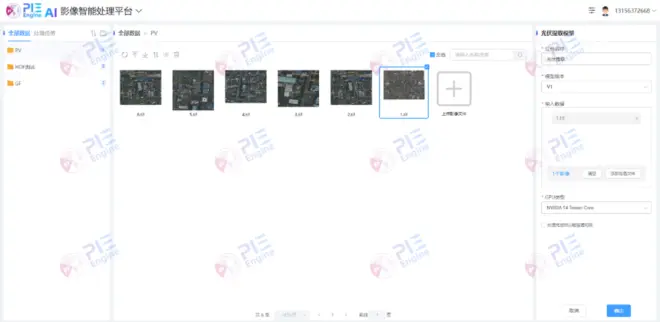
解譯結(jié)果直達(dá)應(yīng)用,支持解譯結(jié)果可視化展示及數(shù)據(jù)下載
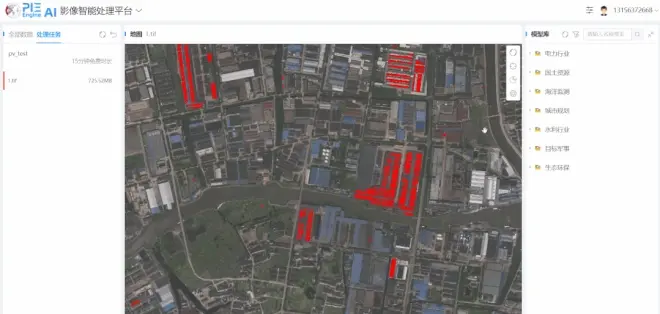
本實(shí)例基于 PIE-Engine AI 平臺完成了 UperNet-SwinTransformer 光伏提取模型從上傳到應(yīng)用的全過程,介紹了用戶上傳在本地訓(xùn)練的模型在 PIE-Engine AI 平臺進(jìn)行一體化流程的便捷性操作。
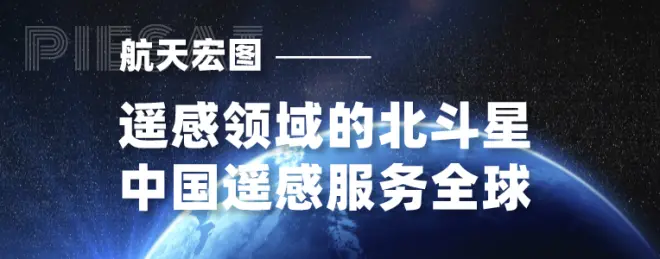