什么是數(shù)據(jù)科學(xué)?《What is data science》 by Mike Loukides翻譯和精讀02
Where data comes from
數(shù)據(jù)從哪里來(lái)
Data is everywhere: your government, your web server, your business partners, even your body. While we aren’t drowning in a sea of data, we’re finding that almost everything can (or has) been?instrumented. At O’Reilly, we frequently combine publishing industry data from Nielsen BookScan?with our own sales data, publicly available Amazon data, and even job data to see what’s happening in the publishing industry. Sites like Infochimps?and Factual?provide access to many large datasets, including climate data, MySpace activity streams, and game logs from sporting events. Factual enlists users to update and improve its datasets, which cover topics as diverse as endocrinologists to hiking trails.
數(shù)據(jù)分布在任何一個(gè)地方:你的政府,你的網(wǎng)絡(luò)服務(wù)器,你的商業(yè)伙伴,甚至你的身體。當(dāng)我們沒(méi)有在數(shù)據(jù)的海洋中溺亡,我們發(fā)現(xiàn)幾乎每一樣?xùn)|西都能(或已經(jīng))能被物聯(lián)網(wǎng)化。在O’Reilly, 尼爾森圖書(shū)調(diào)查機(jī)構(gòu)的出版業(yè)數(shù)據(jù)和我們自己的銷(xiāo)售數(shù)據(jù),公開(kāi)可用的亞馬遜數(shù)據(jù),甚至給ne遇見(jiàn)出版業(yè)發(fā)生什么的工作數(shù)據(jù),我們頻繁地將這些數(shù)據(jù)結(jié)合起來(lái)。像Infochimps和Factual這樣的網(wǎng)址提供很多大型數(shù)據(jù)集的借口,包括氣候數(shù)據(jù),MySpace活動(dòng)流,和體育比賽項(xiàng)目的比賽日志。Factual贊助用戶(hù)來(lái)更新和提升它的數(shù)據(jù)集,涵蓋了從內(nèi)分泌學(xué)家到徒步旅行路線(xiàn)的多樣主題。
?
?
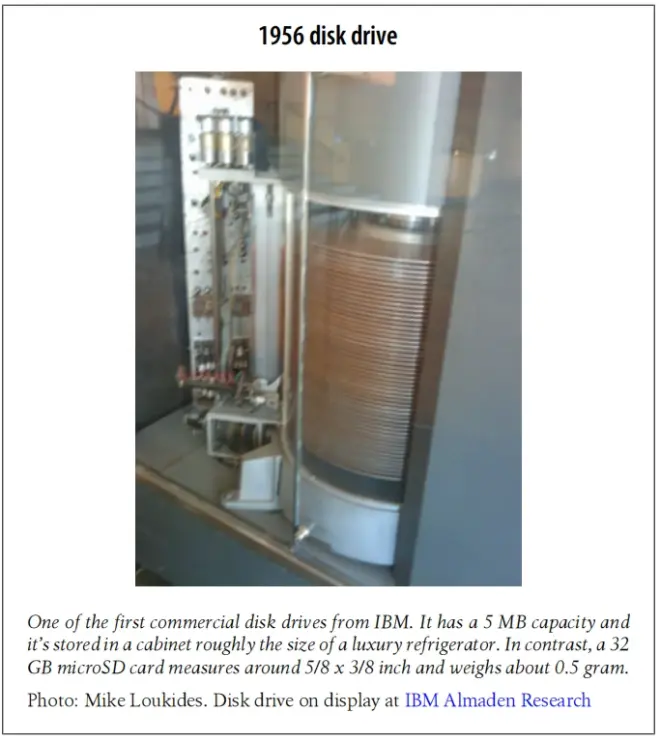
?
IBM第一批商業(yè)磁盤(pán)驅(qū)動(dòng)器的其中一個(gè),它有5MB的容量,被放在一個(gè)大概相當(dāng)于豪華電冰箱大小的柜子里。相反,一個(gè)32GB的microSD卡測(cè)量大概是5/8 x 3/8 英寸,大約重0.5克。
圖片:Mike Loukides. 在IBM Almaden Research陳列的磁盤(pán)驅(qū)動(dòng)器。
?
Much of the data we currently work with is the direct consequence of Web 2.0, and of Moore’s Law applied to data. The web has people spending more time online, and leaving a trail of data wherever they go. Mobile applications leave an even richer data trail, since many of them are annotated with geolocation, or involve video or audio, all of which can be mined. Point-of-sale devices and frequent-shopper’s cards make it possible to capture all of your retail transactions, not just the ones you make online. All of this data would be useless if we couldn’t store it, and that’s where Moore’s Law comes in. Since the early ‘80s, processor speed has increased from 10 MHz?to 3.6 GHz -- an
increase of 360 (not counting increases in word length and number of cores). ?But we’ve seen much bigger increases in storage capacity, on every level. RAM has moved from $1,000/MB to roughly $25/GB -- a price reduction of about 40000, to say nothing of the reduction in size and increase in speed. Hitachi made the first gigabyte disk drives?in 1982, weighing in at roughly 250 pounds;
now terabyte drives are consumer equipment, and a 32 GB microSD card weighs about half a gram. Whether you look at bits per gram, bits per dollar, or raw capacity, storage has more than kept pace with the increase of CPU speed.
當(dāng)下很多我們處理的數(shù)據(jù)是Web 2.0和摩爾定律應(yīng)用到數(shù)據(jù)上的直接結(jié)果。網(wǎng)絡(luò)讓人們花更多事件在線(xiàn)上,并在他們?cè)L問(wèn)的地方留下一條數(shù)據(jù)的蹤跡。移動(dòng)應(yīng)用留下一條更為豐富的數(shù)據(jù)蹤跡,因?yàn)楹芏喾N移動(dòng)應(yīng)用由地理定位注解,或涉及視頻語(yǔ)音,所有這些(數(shù)據(jù))都可以被挖掘。銷(xiāo)售點(diǎn)設(shè)備和??偷模ㄙ?gòu)物)卡使得捕捉所有你們的零售交易成為可能,而不只是你們?cè)诰€(xiàn)上做的(交易)。如果我們不存儲(chǔ)它(指數(shù)據(jù)),那么所有的這些數(shù)據(jù)都將是無(wú)用的,那就是摩爾定律到達(dá)的地方。從20世紀(jì)80年代早期開(kāi)始,處理器速度從10 MHz 提高到3.6GHz——提高了360倍(這還沒(méi)算上字長(zhǎng)和內(nèi)核數(shù)量的增加)。但是我們已經(jīng)看到了在存儲(chǔ)容量上的大的多的增長(zhǎng),在各個(gè)層面上。RAM從每MB1000美元降到了大約每GB25美元——價(jià)格下降了大約4萬(wàn)倍,更不必說(shuō)體積的減少和速度的提升。日立公司在1982年制作了第一個(gè)千兆字節(jié)的磁盤(pán)驅(qū)動(dòng)器,重大約250磅;現(xiàn)在萬(wàn)億字節(jié)驅(qū)動(dòng)是消費(fèi)者標(biāo)配,一個(gè)32G的存儲(chǔ)卡重大概0.5克。不管你是否關(guān)注每克的字節(jié)數(shù),每美元的字節(jié)數(shù)或原始容量,存儲(chǔ)不僅僅是跟上CPU速度增長(zhǎng)的步伐。
?
注:摩爾定律是英特爾創(chuàng)始人之一戈登·摩爾的經(jīng)驗(yàn)之談,其核心內(nèi)容為:集成電路上可以容納的晶體管數(shù)目在大約每經(jīng)過(guò)18個(gè)月到24個(gè)月便會(huì)增加一倍。換言之,處理器的性能大約每?jī)赡攴槐叮瑫r(shí)價(jià)格下降為之前的一半。這里是用處理速度的爆炸性增長(zhǎng)來(lái)類(lèi)比數(shù)據(jù)的爆炸性增長(zhǎng)。
字長(zhǎng)是CPU一次能并行處理的二進(jìn)制位數(shù)。各個(gè)層面——指的是計(jì)算機(jī)的寄存器,內(nèi)存,外存都在容量的不斷告訴增長(zhǎng)中。
?
The importance of Moore’s law as applied to data isn’t just geek pyrotechnics. Data expands to fill the space you have to store it. The more storage is available, the more data you will find to put into it. The data exhaust you leave behind whenever you surf the web, friend someone on Facebook, or make a purchase in your local supermarket, is all carefully collected and analyzed. Increased storage capacity demands increased sophistication in the analysis and use of that data. That’s the foundation of data science.
摩爾定律應(yīng)用到數(shù)據(jù)的重要性不止是極客的魔術(shù)展示。數(shù)據(jù)膨脹到填滿(mǎn)你存儲(chǔ)數(shù)據(jù)的空間。更多的存儲(chǔ)是可用的,更多的數(shù)據(jù)你會(huì)發(fā)現(xiàn)將其放進(jìn)存儲(chǔ)中。當(dāng)你在網(wǎng)上沖浪后留下的數(shù)據(jù)廢氣,在臉書(shū)上與人交友,在你本地的超市做一次采購(gòu),這些數(shù)據(jù)都會(huì)被仔細(xì)地收集和分析。增長(zhǎng)的存儲(chǔ)容量要求對(duì)那些數(shù)據(jù)的分析和使用提出了更高水平的要求。這是數(shù)據(jù)科學(xué)的基石。
?
注:exhaust除了動(dòng)詞,還可以作名詞。The data exhaust you leave behind whenever you surf the web, friend someone on Facebook, or make a purchase in your local supermarket, is all carefully collected and analyzed. 這個(gè)長(zhǎng)句子是被動(dòng)語(yǔ)態(tài),主語(yǔ)The data exhaust,動(dòng)詞is,collected and anyalyzed表示被收集和分析。而exhaust要么是及物動(dòng)詞,或者是名詞。這里只能是名詞。data exhaust你以為你留下的數(shù)據(jù)是廢氣,會(huì)被排放然后無(wú)蹤跡,但不是,你的蹤跡,數(shù)據(jù)會(huì)被用心保存。
?
So, how do we make that data useful? The first step of any data analysis project is “data conditioning,” or getting data into a state where it’s usable. We are seeing more data in formats that are easier to consume: Atom data feeds, web services, microformats, and other newer technologies provide data in formats that’s directly machine-consumable. But old-style screen scraping?hasn’t died, and isn’t going to die. Many sources of “wild data” are extremely messy. They aren’t well-behaved XML files with all the metadata nicely in place. The foreclosure data used in “Data Mashups in R” was posted on a public website by the Philadelphia county sheriff’s office. This data was presented as an HTML file that was probably generated automatically from a spreadsheet. If you’ve ever seen the HTML that’s generated by Excel, you know that’s going to be fun to process.
因此,我們?cè)撊绾巫寯?shù)據(jù)有用?任何數(shù)據(jù)分析工程的第一步是“數(shù)據(jù)調(diào)節(jié)”,或者說(shuō)讓數(shù)據(jù)進(jìn)入可使用的狀態(tài)。我們發(fā)現(xiàn)格式的數(shù)據(jù)更容易處理:Atom data feeds(一種技術(shù)提供的數(shù)據(jù)),網(wǎng)絡(luò)服務(wù),微格式化,和其它提供機(jī)器可以直接處理的格式的數(shù)據(jù)的更新的技術(shù)。但是老式的screen scraping(一種屏幕抓取技術(shù))還未消亡,也不會(huì)即將消亡?!耙吧鷶?shù)據(jù)”的多種來(lái)源極其混亂。它們不是表現(xiàn)良好的XML文件——所有的元數(shù)據(jù)都很好地放在適當(dāng)?shù)奈恢??!禗ata Mashup in R》中使用的喪失抵押品贖回權(quán)數(shù)據(jù)被費(fèi)城縣警長(zhǎng)辦公室發(fā)布在一個(gè)公開(kāi)網(wǎng)址上。這些數(shù)據(jù)是以很有可能由電子表格自動(dòng)生成的HTML文件的形式呈現(xiàn)。如果你曾經(jīng)見(jiàn)過(guò)由Excel生成的HTML,你知道那些(數(shù)據(jù),指HTML數(shù)據(jù))數(shù)據(jù)處理起來(lái)是很開(kāi)心有趣的。
?
注:excel就是spreadsheet的一種。最后一句話(huà)的意思是,如果不是嚴(yán)格格式的數(shù)據(jù),處理起來(lái)會(huì)很痛苦。
?
Data conditioning can involve cleaning up messy HTML with tools like Beautiful Soup, natural language processing to parse plain text in English and other languages, or even getting humans to do the dirty work. You’re likely to be dealing with an array of data sources, all in different forms. It would be nice if?there was a standard set of tools to do the job, but there isn’t. To do data conditioning, you have to be ready for whatever comes, and be willing to use anything from ancient Unix utilities such as awk?to XML parsers and machine learning libraries. Scripting languages, such as Perl?and Python, are essential.
數(shù)據(jù)調(diào)節(jié)包含使用像Beautiful Soup這樣的工具進(jìn)行混亂的HTML的清理,自然語(yǔ)言處理來(lái)解析英語(yǔ)和其它語(yǔ)言的純文本,或甚至讓人們來(lái)處理dirty work。你很有可能要處理一堆的數(shù)據(jù)來(lái)源,都是不同的格式。要是有一系列標(biāo)準(zhǔn)工具來(lái)做這項(xiàng)工作就好了,但是沒(méi)有。要做數(shù)據(jù)調(diào)整,你必須要為即將到來(lái)的一切做準(zhǔn)備,還要愿意使用任何工具,從古老的Unix程序比如awk到XML parsers和機(jī)器學(xué)習(xí)庫(kù)。腳本語(yǔ)言,像Perl和Python,是必要的。
?
注:李飛飛曾經(jīng)將重復(fù)簡(jiǎn)單含金量很低的數(shù)據(jù)標(biāo)注工作稱(chēng)為dirty work。
?
Once you’ve parsed the data, you can start thinking about the quality of your data. Data is frequently missing or incongruous. If data is missing, do you simply ignore the missing points? That isn’t always possible. If data is incongruous, do you decide that something is wrong with badly behaved data (after
all, equipment fails), or that the incongruous data is telling its own story, which may be more interesting? It’s reported that the discovery of ozone layer depletion was delayed because automated data collection tools discarded readings that were too low1. In data science, what you have is frequently all you’re going to get. It’s usually impossible to get “better” data, and you have no alternative but to work with the data at hand.
一旦你已經(jīng)解析了數(shù)據(jù),你可以開(kāi)始思考你數(shù)據(jù)的質(zhì)量。數(shù)據(jù)常常是缺少的,或是不一致的。如果數(shù)據(jù)是缺失的,你會(huì)簡(jiǎn)單地忽視丟失的點(diǎn)嗎?那并不是在所有情況下都可能。如果數(shù)據(jù)是不一致的,你是判定表現(xiàn)不好的數(shù)據(jù)出現(xiàn)問(wèn)題(畢竟,裝備失?。?,還是不一致的數(shù)據(jù)在訴說(shuō)它自己的故事,哪一種更有意思?報(bào)告聲稱(chēng)臭氧層破壞的發(fā)現(xiàn)被推遲了,因?yàn)樽詣?dòng)數(shù)據(jù)收集工具放棄讀?。ǔ粞鯇樱┖艿偷臄?shù)據(jù)1。在數(shù)據(jù)科學(xué)中,你已經(jīng)有的都是你即將獲得的。通常來(lái)說(shuō)不可能獲得“更好的”數(shù)據(jù),并且你別無(wú)選擇只能處理手上的數(shù)據(jù)。
?
If the?problem involves human language, understanding the data adds another dimension to the problem. Roger Magoulas, who runs the data analysis group at O’Reilly, was recently searching a database for Apple job listings requiring geolocation skills. While that sounds like a simple task, the trick was disambiguating “Apple” from many job postings in the growing Apple industry. To do it well you need to understand the grammatical structure of a job posting; you need to be able to parse the English. And that problem is showing up more and more frequently. Try using Google Trends?to figure out what’s happening with the Cassandra?database or the Python?language, and you’ll get a sense of the problem. Google has indexed many, many websites about large snakes. Disambiguation is never an easy task, but tools like the Natural Language Toolkit?library can make it simpler.
如果這個(gè)難題涉及人類(lèi)語(yǔ)言,理解數(shù)據(jù)給這個(gè)難題添加了另一個(gè)維度。在O’Reilly管理數(shù)據(jù)分析小組的Roger Magoulas,最近在尋找一個(gè)需要地理定位技能的Apple工作列表數(shù)據(jù)庫(kù)。盡管那聽(tīng)起來(lái)像一個(gè)簡(jiǎn)單的任務(wù),技巧是在增長(zhǎng)中的蘋(píng)果產(chǎn)業(yè)的許多工作信息中消除“Apple”的二義性。要想把它(消除二義性)做好,你需要理解一份工作信息的語(yǔ)法結(jié)構(gòu);你需要有能力來(lái)解析英語(yǔ)(至少要知道一詞多義)。而且那個(gè)難題出現(xiàn)的越來(lái)越頻繁。試著使用谷歌趨勢(shì)來(lái)弄清楚Cassandra數(shù)據(jù)庫(kù)或Python語(yǔ)言在做什么,你會(huì)感覺(jué)到問(wèn)題的所在。谷歌已經(jīng)為很多,很多關(guān)于大型蛇(這里應(yīng)該是指Python)的網(wǎng)站編了索引。消除二義性從來(lái)不是一個(gè)簡(jiǎn)單的工作,但是像自然語(yǔ)言工具箱庫(kù)之類(lèi)的工作可以讓它簡(jiǎn)單一些。
?
注:ambiguous模糊的,有歧義的,disambiguating消除二義性。Python有蟒蛇的意思,Python是編程語(yǔ)言,但是在為編程語(yǔ)言命名之前,本意是蛇。
?
When natural language processing fails, you can replace artificial intelligence with human intelligence. That’s where services like Amazon’s Mechanical Turk?come in. If you can split your task up into a large number of subtasks that are easily described, you can use Mechanical Turk’s marketplace for cheap labor. For example, if you’re looking at job listings, and want to know which originated with Apple, you can have real people do the classification for roughly $0.01 each. If you have already reduced the set to 10,000 postings with the word “Apple,” paying humans $0.01 to classify them only costs $100.
當(dāng)自然語(yǔ)言處理失敗,你可以用人類(lèi)智能來(lái)替代人工智能。那就是比如亞馬遜的Mechanical Turk服務(wù)發(fā)揮作用的地方。如果你能將你的任務(wù)分解為大量容易被描述的子任務(wù),你就可以使用Mechanical Turk的廉價(jià)勞動(dòng)力市場(chǎng)。舉例子來(lái)說(shuō),如果你正在尋找工作列表,并想要知道哪些起源于蘋(píng)果,你可以讓真實(shí)的人來(lái)做分類(lèi),只要每條支付0.01美元。如果你已經(jīng)縮小集合到一萬(wàn)條有著“Apple”這個(gè)詞的信息,雇人0.01美元的報(bào)酬來(lái)分類(lèi)它們只需要花費(fèi)100美元。
?
注:replace A with B是用B替代A。replace A for B是用A替代B。關(guān)于marketplace for cheap labor,微軟投資的openAI的chatGPT也傳出使用成本低廉的次發(fā)達(dá)國(guó)家的勞工來(lái)處理大量數(shù)據(jù)標(biāo)注的傳聞。只能說(shuō),廉價(jià)勞動(dòng)力來(lái)進(jìn)行繁雜,技術(shù)含量不高的數(shù)據(jù)標(biāo)注工作,是初期的必然。
?
?
1.?The NASA article denies this, but also says that in 1984, they decided that the low values (which went back to the 70s) were “real.” Whether humans or software decided to ignore anomalous data, it appears that data was ignored.
腳注1:NASA報(bào)告否認(rèn)了這點(diǎn)(指放棄讀取臭氧層含量很低的數(shù)據(jù)),但也承認(rèn)在1984年,他們判定那些低值(追溯到20世紀(jì)70年代)是“真實(shí)”的。無(wú)論是人類(lèi)或軟件決定忽略異常的數(shù)據(jù),看起來(lái)數(shù)據(jù)是被忽略了。
?
?