Talk預(yù)告 | 亞馬遜云科技肖天駿:基于視頻的自監(jiān)督物體遮擋補(bǔ)全分割
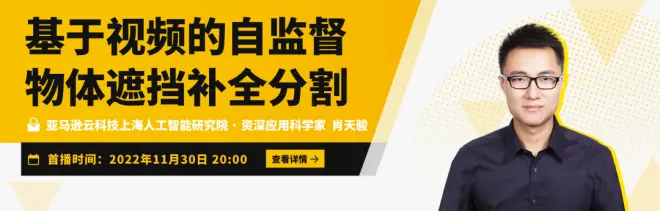
本期為TechBeat人工智能社區(qū)第458期線上Talk!
北京時(shí)間11月30日(周三)20:00,亞馬遜云科技上海人工智能研究院資深應(yīng)用科學(xué)家——肖天駿的Talk將準(zhǔn)時(shí)在TechBeat人工智能社區(qū)開播!
他與大家分享的主題是:?“基于視頻的自監(jiān)督物體遮擋補(bǔ)全分割”,屆時(shí)將詳細(xì)講解論文的母課題以物體為中心的學(xué)習(xí),其研究目標(biāo),基本方法和應(yīng)用。
Talk·信息
主題:基于視頻的自監(jiān)督物體遮擋補(bǔ)全分割
嘉賓:亞馬遜云科技上海人工智能研究院資深應(yīng)用科學(xué)家肖天駿
時(shí)間:北京時(shí)間?11月30日?(周三) 20:00
地點(diǎn):TechBeat人工智能社區(qū)
http://www.techbeat.net/
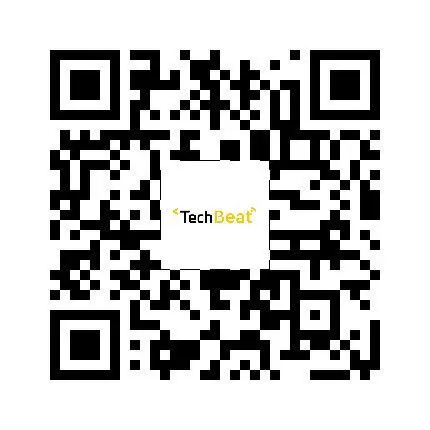
完整版怎么看
掃描下方二維碼,或復(fù)制鏈接https://datayi.cn/w/QReK1r3o瀏覽器,一鍵完成預(yù)約!上線后會(huì)在第一時(shí)間收到通知哦
Talk·介紹
物體遮擋補(bǔ)全分割(或稱Amodal物體分割)是人類視覺感知的重要能力,該任務(wù)在自動(dòng)輔助駕駛,機(jī)器人操作等領(lǐng)域都有重要應(yīng)用。相比于基于圖像學(xué)習(xí)類別先驗(yàn)的方法,我們提出用視頻進(jìn)行自監(jiān)督學(xué)習(xí)時(shí)空先驗(yàn)的方法取得了更好的分割效果,同時(shí)能夠更好地泛化到訓(xùn)練時(shí)沒有見過的類別。此外,本次talk我們還將介紹本篇論文的母課題以物體為中心的學(xué)習(xí),其研究目標(biāo),基本方法和應(yīng)用。
Talk大綱如下:
Amodal物體分割任務(wù)介紹
為什么使用視頻來解決amodal segmentation
自監(jiān)督模型設(shè)計(jì)
與image-level SOTA模型的對(duì)比實(shí)驗(yàn)及自身消融實(shí)驗(yàn)
母課題以物體為中心的學(xué)習(xí)方法介紹
Talk·預(yù)習(xí)資料
Jian Yao, Yuxin Hong, Chiyu Wang, Tianjun Xiao, Tong He, Francesco Locatello, David Wipf, Yanwei Fu, and Zheng Zhang. Self-supervised amodal video object segmentation. arXiv preprint arXiv:2210.12733, 2022.
https://www.amazon.science/publications/self-supervised-amodal-video-object-segmentation
Related work鏈接:Yan Zhu, Yuandong Tian, Dimitris Metaxas, and Piotr Dollár. Semantic amodal segmentation. In Proceedings of the IEEE conference on computer vision and pattern recognition, pages 1464–1472, 2017.https://arxiv.org/pdf/1509.01329.pdf
Xiaohang Zhan, Xingang Pan, Bo Dai, Ziwei Liu, Dahua Lin, and Chen Change Loy. Self- supervised scene de-occlusion. In Proceedings of the IEEE/CVF Conference on Computer Vision and Pattern Recognition, pages 3784–3792, 2020.https://openaccess.thecvf.com/content_CVPR_2020/papers/Zhan_Self-Supervised_Scene_De-Occlusion_CVPR_2020_paper.pdf
Object-centric related work鏈接:Francesco Locatello, Dirk Weissenborn, Thomas Unterthiner, Aravindh Mahendran, Georg Heigold, Jakob Uszkoreit, Alexey Dosovitskiy, and Thomas Kipf. Object-Centric Learning with Slot Attention. In H. Larochelle, M. Ranzato, R. Hadsell, M.F. Balcan, and H. Lin (eds.), Advances in Neural Information Processing Systems, volume 33, pp. 11525–11538. Curran Associates, Inc., 2020.https://proceedings.neurips.cc/paper/2020/file/511df98c02ab60aea1b2356c013bc0f-Paper.pdf
Longyuan Li, Jian Yao, Li Wenliang, Tong He, Tianjun Xiao, Junchi Yan, David Wipf, and Zheng Zhang. Grin: Generative relation and intention network for multi-agent trajectory prediction. In NeurIPS, 2021.https://www.amazon.science/publications/grin-generative-relation-and-intention-network-for-multi-agent-trajectory-prediction
Maximilian Seitzer, Max Horn, Andrii Zadaianchuk, Dominik Zietlow, Tianjun Xiao, Carl- Johann Simon-Gabriel, Tong He, Zheng Zhang, Bernhard Sch?lkopf, Thomas Brox, et al. Bridging the gap to real-world object-centric learning. arXiv preprint arXiv:2209.14860, 2022.https://arxiv.org/pdf/2209.14860.pdf?
Talk·提問交流
在Talk界面下的【交流區(qū)】參與互動(dòng)!
留下你的打call??和問題??,和更多小伙伴們共同討論,被講者直接翻牌解答!
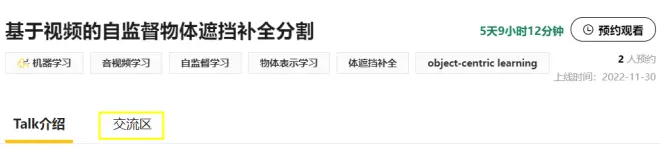
你的每一次貢獻(xiàn),我們都會(huì)給予你相應(yīng)的i豆積分,還會(huì)有驚喜獎(jiǎng)勵(lì)哦!
Talk·嘉賓介紹
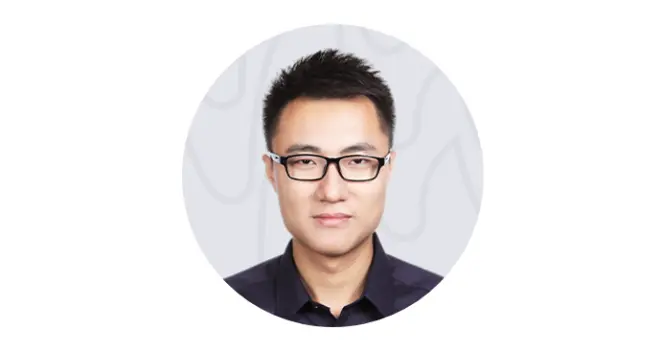
亞馬遜云科技上海人工智能研究院資深應(yīng)用科學(xué)家
肖天駿,現(xiàn)任亞馬遜云科技上海人工智能研究院資深應(yīng)用科學(xué)家。他的主要研究興趣包括物體表示學(xué)習(xí),圖神經(jīng)網(wǎng)絡(luò),以及上述方向在視頻分析,3D視覺,自動(dòng)輔助駕駛與機(jī)器人中的應(yīng)用。相關(guān)工作應(yīng)用于AWS視覺智能服務(wù)。在此之前,他任職于Tesla Autopilot團(tuán)隊(duì),擔(dān)任資深機(jī)器學(xué)習(xí)科學(xué)家,負(fù)責(zé)Autopilot中動(dòng)態(tài)與靜態(tài)物體的檢測(cè)與表示,他的工作被部署于全球上百萬輛Tesla汽車中。北大研究生期間及畢業(yè)后,分別在微軟亞洲研究院實(shí)習(xí)與微軟Bing多媒體搜索團(tuán)隊(duì)工作,參與了機(jī)器學(xué)習(xí)開源軟件Minerva,MXNet的開發(fā),主攻細(xì)粒度圖像分類,多模態(tài)語義嵌入等算法項(xiàng)目的研究,相關(guān)學(xué)術(shù)工作發(fā)表于NeurIPS, CVPR, ICCV, ECCV等學(xué)術(shù)會(huì)議。
主頁:http://tianjunxiao.com/?
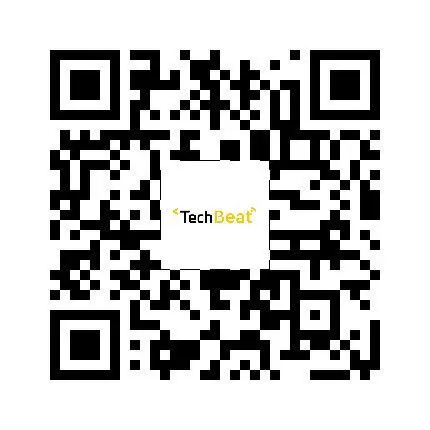
-The End-
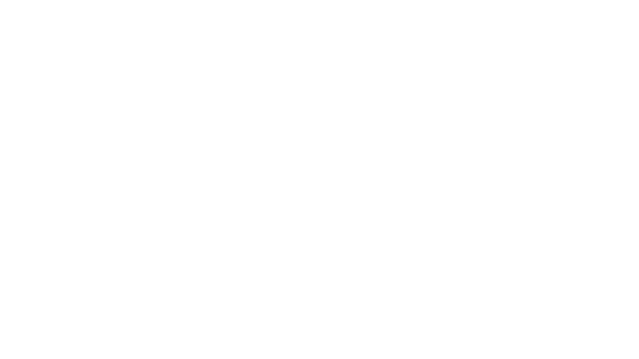
關(guān)于TechBeat人工智能社區(qū)
TechBeat (www.techbeat.net) 是一個(gè)薈聚全球華人AI精英的成長社區(qū)。 我們希望為AI人才打造更專業(yè)的服務(wù)和體驗(yàn),加速并陪伴其學(xué)習(xí)成長。 期待這里可以成為你學(xué)習(xí)AI前沿知識(shí)的高地,分享自己最新工作的沃土,在AI進(jìn)階之路上的升級(jí)打怪的根據(jù)地!
更多詳細(xì)介紹>>https://mp.weixin.qq.com/s/pTbCK_MeTk05jK2yx1RTrQ