KDD2023丨表征學(xué)習(xí)論文合集
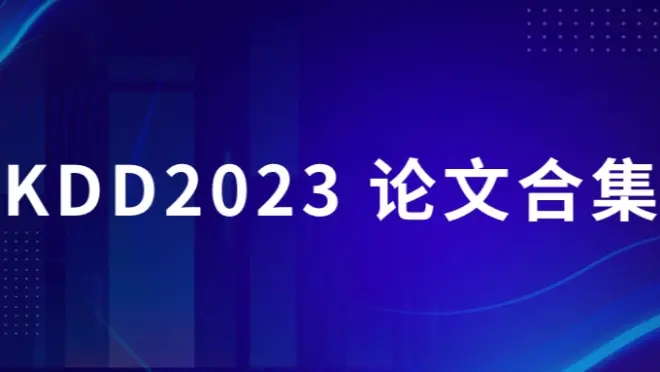
ACM SIGKDD(國(guó)際數(shù)據(jù)挖掘與知識(shí)發(fā)現(xiàn)大會(huì),簡(jiǎn)稱KDD)會(huì)議始于1989年,是數(shù)據(jù)挖掘領(lǐng)域歷史最悠久、規(guī)模最大的國(guó)際頂級(jí)學(xué)術(shù)會(huì)議,也是首個(gè)引入大數(shù)據(jù)、數(shù)據(jù)科學(xué)、預(yù)測(cè)分析、眾包等概念的會(huì)議,每年吸引了大量數(shù)據(jù)挖掘、機(jī)器學(xué)習(xí)、大數(shù)據(jù)和人工智能等領(lǐng)域的研究學(xué)者、從業(yè)人員參與。
AMiner通過(guò)AI技術(shù),對(duì) KDD2023 收錄的會(huì)議論文進(jìn)行了分類整理,今日分享的是表征學(xué)習(xí)主題論文!(由于篇幅關(guān)系,本篇只展現(xiàn)部分論文,點(diǎn)擊閱讀原文可直達(dá)KDD頂會(huì)頁(yè)面查看所有論文)
1.DCdetector: Dual Attention Contrastive Representation Learning for Time Series Anomaly Detection
鏈接:https://www.aminer.cn/pub/6492753bd68f896efa888f46/
2.GENERALIZED MATRIX LOCAL LOW RANK REPRESENTATION BY RANDOM PROJECTION AND SUBMATRIX PROPAGATION
鏈接:https://www.aminer.cn/pub/6433f6bc90e50fcafd6efdfd/
3.Joint Pre-training and Local Re-training: Transferable Representation Learning on Multi-source Knowledge Graphs
鏈接:https://www.aminer.cn/pub/647eaf51d68f896efad41d32/
4.Task Relation-aware Continual User Representation Learning
鏈接:https://www.aminer.cn/pub/647eaf35d68f896efad40763/
5.Dense Representation Learning and Retrieval for Tabular Data Prediction
鏈接:https://www.aminer.cn/pub/64af99fd3fda6d7f065a62e9/
6.Efficient and Effective Edge-wise Graph Representation Learning
鏈接:https://www.aminer.cn/pub/64af99fe3fda6d7f065a63b4/
7.CARL-G: Clustering-Accelerated Representation Learning on Graphs
鏈接:https://www.aminer.cn/pub/64af99fe3fda6d7f065a63ce/
8.LightPath: Lightweight and Scalable Path Representation Learning
鏈接:https://www.aminer.cn/pub/64af9a0b3fda6d7f065a70cd/
9.Urban Region Representation Learning with OpenStreetMap Building Footprints
鏈接:https://www.aminer.cn/pub/64af9a0b3fda6d7f065a70d1/
10.Representation Learning on Hyper-Relational and Numeric Knowledge Graphs with Transformers
鏈接:https://www.aminer.cn/pub/647572e0d68f896efa7b7983/
11.Expert Knowledge-Aware Image Difference Graph Representation Learning for Difference-Aware Medical Visual Question Answering
鏈接:https://www.aminer.cn/pub/64af9a023fda6d7f065a686d/
12.DyTed: Disentangled Representation Learning for Discrete-time Dynamic Graph
鏈接:https://www.aminer.cn/pub/64af9a093fda6d7f065a6eac/
13.Heterformer: Transformer-based Deep Node Representation Learning on Heterogeneous Text-Rich Networks
鏈接:https://www.aminer.cn/pub/64af9a093fda6d7f065a6eb0/

如何使用ChatPaper讀文獻(xiàn)?
為了讓更多科研人更高效的獲取文獻(xiàn)知識(shí),AMiner基于GLM-130B大模型能力,開(kāi)發(fā)了Chatpaper,幫助科研人快速提高檢索、閱讀論文效率,獲取最新領(lǐng)域研究動(dòng)態(tài),讓科研工作更加游刃有余。
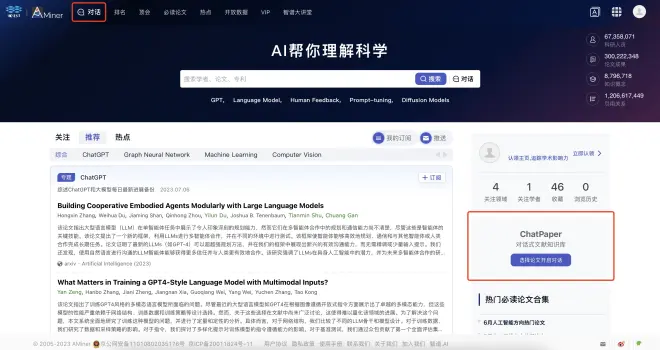
ChatPaper是一款集檢索、閱讀、知識(shí)問(wèn)答于一體的對(duì)話式私有知識(shí)庫(kù),AMiner希望通過(guò)技術(shù)的力量,讓大家更加高效地獲取知識(shí)。
ChatPaper:https://www.aminer.cn/chat/g
KDD頂會(huì):https://www.aminer.cn/conf/5ea1b22bedb6e7d53c00c41b/KDD2023