KDD2023 GNN論文合集
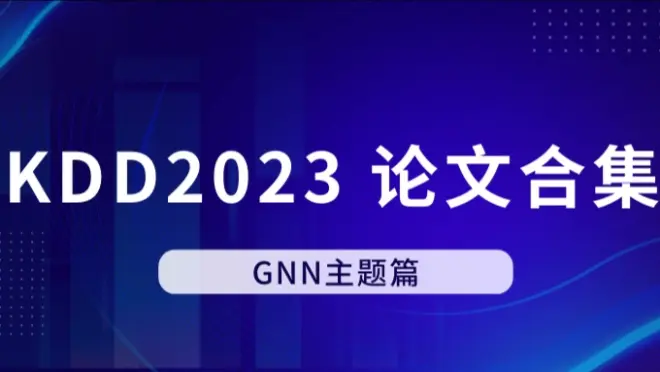
ACM?SIGKDD(國(guó)際數(shù)據(jù)挖掘與知識(shí)發(fā)現(xiàn)大會(huì),簡(jiǎn)稱KDD)會(huì)議始于1989年,是數(shù)據(jù)挖掘領(lǐng)域歷史最悠久、規(guī)模最大的國(guó)際頂級(jí)學(xué)術(shù)會(huì)議,也是首個(gè)引入大數(shù)據(jù)、數(shù)據(jù)科學(xué)、預(yù)測(cè)分析、眾包等概念的會(huì)議,每年吸引了大量數(shù)據(jù)挖掘、機(jī)器學(xué)習(xí)、大數(shù)據(jù)和人工智能等領(lǐng)域的研究學(xué)者、從業(yè)人員參與。
AMiner通過(guò)AI技術(shù),對(duì) KDD2023 收錄的會(huì)議論文進(jìn)行了分類(lèi)整理,今日分享的是GNN主題論文?。ㄓ捎谄P(guān)系,本篇只展現(xiàn)部分論文,點(diǎn)擊文末鏈接可直達(dá)KDD頂會(huì)頁(yè)面,查看所有論文)
1.Spatial Heterophily Aware Graph Neural Networks
鏈接:https://www.aminer.cn/pub/6493c733d68f896efad19bfa/
2.All in One: Multi-task Prompting for Graph Neural Networks
鏈接:https://www.aminer.cn/pub/64a63bbad68f896efaec478f/
3.GraphGLOW: Universal and Generalizable Structure Learning for Graph Neural Networks
鏈接:https://www.aminer.cn/pub/64927546d68f896efa88a31b/
4.Learning Strong Graph Neural Networks with Weak Information
鏈接:https://www.aminer.cn/pub/6476d20cd68f896efaf727d4/
5.Graph Neural Bandits
鏈接:https://www.aminer.cn/pub/6433f6b990e50fcafd6ef10f/
6.WinGNN: Dynamic Graph Neural Networks with Random Gradient Aggregation Window
鏈接:https://www.aminer.cn/pub/64af9a093fda6d7f065a6ec4/
7.E-commerce Search via Content Collaborative Graph Neural Network
鏈接:https://www.aminer.cn/pub/64af9a033fda6d7f065a6982/
8.Localised Adaptive Spatial-Temporal Graph Neural Network
鏈接:https://www.aminer.cn/pub/64af9a073fda6d7f065a6d31/
9.Leveraging Relational Graph Neural Network for Transductive Model Ensemble
鏈接:https://www.aminer.cn/pub/64af9a0a3fda6d7f065a6fdd/
10.Narrow the Input Mismatch in Deep Graph Neural Network Distillation
鏈接:https://www.aminer.cn/pub/64af9a013fda6d7f065a6752/
11.Certified Edge Unlearning for Graph Neural Networks
鏈接:https://www.aminer.cn/pub/64af9a013fda6d7f065a66e3/
12.Enhancing Node-Level Adversarial Defenses by Lipschitz Regularization of Graph Neural Networks
鏈接:https://www.aminer.cn/pub/64af9a093fda6d7f065a6f7b/
13.When to Pre-Train Graph Neural Networks? From Data Generation Perspective!
鏈接:https://www.aminer.cn/pub/64af9a0a3fda6d7f065a6fe9/
14.Towards graph-level anomaly detection via deep evolutionary mapping
鏈接:https://www.aminer.cn/pub/6433f67c90e50fcafd6da665/
15.MixupExplainer: Generalizing Explanations for Graph Neural Networks with Data Augmentation
鏈接:https://www.aminer.cn/pub/64af99fd3fda6d7f065a6347/
16.Pyramid Graph Neural Network: A Graph Sampling and Filtering Approach for Multi-scale Disentangled Representations
鏈接:https://www.aminer.cn/pub/64af99fd3fda6d7f065a6396/
17.MGNN: Graph Neural Networks Inspired by Distance Geometry Problem
鏈接:https://www.aminer.cn/pub/64af9a003fda6d7f065a6623/
18.Augmenting Recurrent Graph Neural Networks with a Cache
鏈接:https://www.aminer.cn/pub/64af9a013fda6d7f065a66fb/
19.AdaProp: Learning Adaptive Propagation for Graph Neural Network based Knowledge Graph Reasoning
鏈接:https://www.aminer.cn/pub/64af9a013fda6d7f065a67cf/
20.Financial Default Prediction via Motif-preserving Graph Neural Network with Curriculum Learning
鏈接:https://www.aminer.cn/pub/64af9a083fda6d7f065a6dff/
21.QTIAH-GNN: Quantity and Topology Imbalance-aware Heterogeneous Graph Neural Network for Bankruptcy Prediction
鏈接:https://www.aminer.cn/pub/64af9a083fda6d7f065a6e1d/
22.Multiplex Heterogeneous Graph Neural Network with Behavior Pattern Modeling
鏈接:https://www.aminer.cn/pub/64af9a083fda6d7f065a6e2a/
23.Interpretable Sparsification of Brain Graphs: Better Practices and Effective Designs for Graph Neural Networks
鏈接:https://www.aminer.cn/pub/64af9a093fda6d7f065a6f27/

如何使用ChatPaper讀文獻(xiàn)?
為了讓更多科研人更高效的獲取文獻(xiàn)知識(shí),AMiner基于GLM-130B大模型能力,開(kāi)發(fā)了Chatpaper,幫助科研人快速提高檢索、閱讀論文效率,獲取最新領(lǐng)域研究動(dòng)態(tài),讓科研工作更加游刃有余。
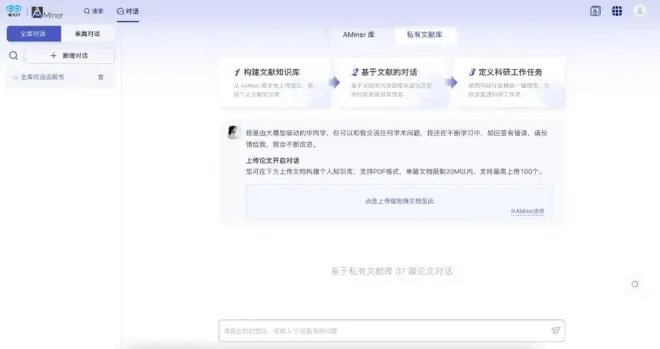
ChatPaper是一款集檢索、閱讀、知識(shí)問(wèn)答于一體的對(duì)話式私有知識(shí)庫(kù),AMiner希望通過(guò)技術(shù)的力量,讓大家更加高效地獲取知識(shí)。
ChatPaper:https://www.aminer.cn/chat/g