【直播預(yù)告】SFFAI 138 因果科學(xué)專題
SFFAI論壇已開放注冊
詳情點(diǎn)擊查看:https://bbs.sffai.com/d/312
盡管深度學(xué)習(xí)模型在大量任務(wù)中擁有極佳的性能,但它容易學(xué)習(xí)到虛假相關(guān)性。為了緩解這個(gè)問題,本期講者王心怡同學(xué)提出了一個(gè)基于因果關(guān)系的訓(xùn)練框架,以減少由觀察到的混雜因素而引起的虛假相關(guān)性。

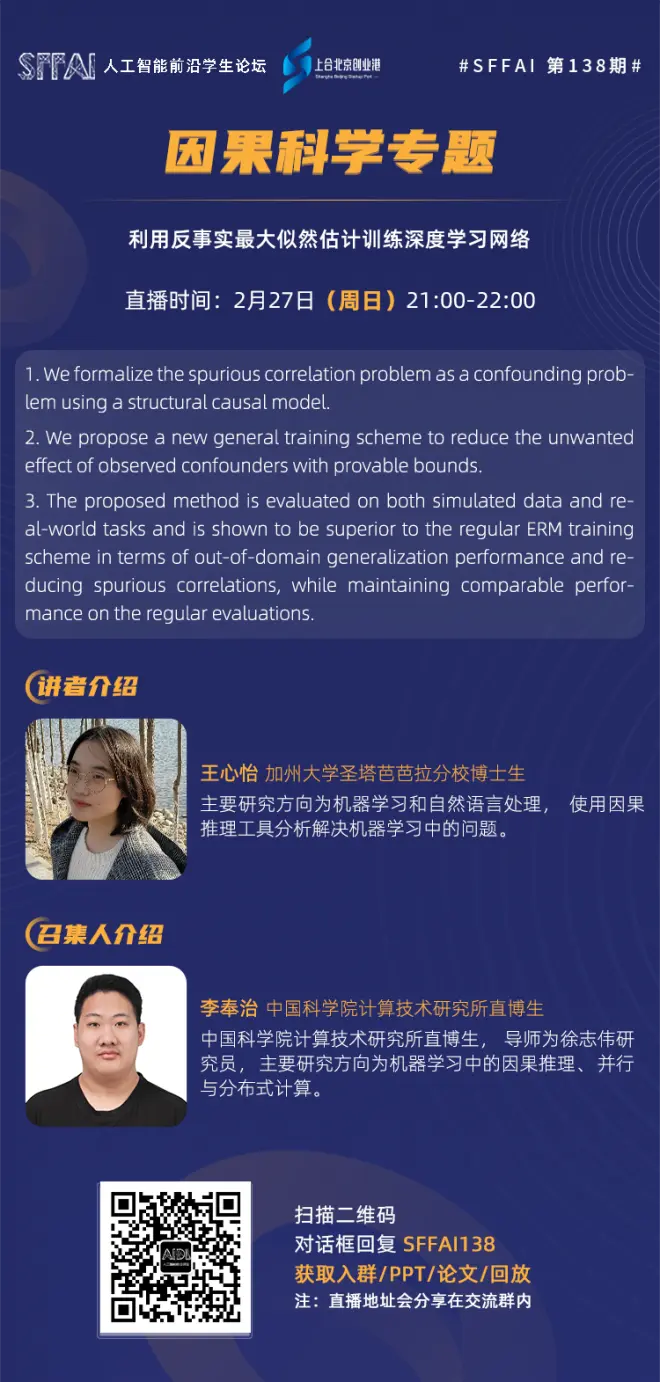
講者介紹
王心怡,加州大學(xué)圣塔芭芭拉分校博士生,主要研究方向?yàn)闄C(jī)器學(xué)習(xí)和自然語言處理,使用因果推理工具分析解決機(jī)器學(xué)習(xí)中的問題。
分享題目
利用反事實(shí)最大似然估計(jì)訓(xùn)練深度學(xué)習(xí)網(wǎng)絡(luò)
分享摘要
We propose a causality-based training framework to reduce the spurious correlations caused by observed confounders. We give theoretical analysis on the underlying general Structural Causal Model (SCM) and propose to perform Maximum Likelihood Estimation (MLE) on the interventional distribution instead of the observational distribution, namely Counterfactual Maximum Likelihood Estimation (CMLE). As the interventional distribution, in general, is hidden from the observational data, we then derive two different upper bounds of the expected negative log-likelihood and propose two general algorithms, Implicit CMLE and Explicit CMLE, for causal predictions of deep learning models using observational data. We conduct experiments on both simulated data and two real-world tasks: Natural Language Inference (NLI) and Image Captioning. The results show that CMLE methods outperform the regular MLE method in terms of out-of-domain generalization performance and reducing spurious correlations, while maintaining comparable performance on the regular evaluations.
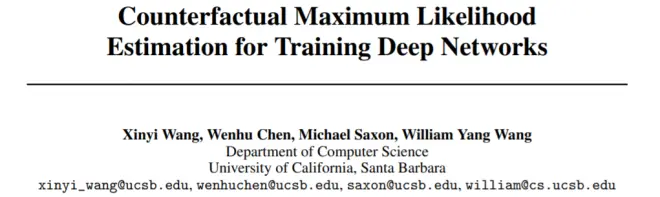
論文題目:Counterfactual Maximum Likelihood Estimation for Training Deep Networks
分享亮點(diǎn)
1.?We formalize the spurious correlation problem as a confounding problem using a structural causal model.
2.?We propose a new general training scheme to reduce the unwanted effect of observed confounders with provable bounds.
3.?The proposed method is evaluated on both simulated data and real-world tasks and is shown to be superior to the regular ERM training scheme in terms of out-of-domain generalization performance and reducing spurious correlations, while maintaining comparable performance on the regular evaluations.
直播時(shí)間
2022年2月27日(周日)21:00—22:00 線上直播
關(guān)注微信公眾號:人工智能前沿講習(xí),對話框回復(fù)“SFFAI138”,獲取入群二維碼

人工智能前沿學(xué)生論壇 SFFAI(Student Forums on Frontiers of Artificial Intelligence)每周日舉行一期, 邀請一線科研人員分享、討論人工智能各個(gè)領(lǐng)域的前沿思想和最新成果,使專注于各個(gè)細(xì)分領(lǐng)域的研究者開拓視野、觸類旁通。SFFAI 目前主要關(guān)注計(jì)算機(jī)視覺、自然語言處理、數(shù)據(jù)挖掘、機(jī)器學(xué)習(xí)理論等各個(gè)人工智能垂直領(lǐng)域及交叉領(lǐng)域的前沿進(jìn)展,進(jìn)行學(xué)術(shù)傳播,為初學(xué)者指明方向,也為講者塑造個(gè)人影響力。
歡迎大家關(guān)注SFFAI論壇:https://bbs.sffai.com/。
