融合物理信息的神經(jīng)網(wǎng)絡(luò)(PINN)代碼解析(一)
寫在前面:非權(quán)威解析,歡迎各位大佬提出批評指正。? ?
本次解析代碼:
1.?https://github.com/okada39/pinn_cavity(定常蓋板驅(qū)動方腔內(nèi)流場數(shù)值模擬)
2.?https://github.com/yunbattle1994/PINN-incompressive-laminar-cylinder(定常圓柱繞流問題)
關(guān)于非定常問題其基本原理基本一致,需要在增加時間項,具體可參考:
Raocp的github:https://github.com/Raocp/PINN-laminar-flow(定常及非定常圓柱繞流問題)
融合物理信息的神經(jīng)網(wǎng)絡(luò)(Physics Informed Neural Network, PINN)由布朗大學(xué)Raissi M等人提出,相關(guān)的研究成果發(fā)表于JCP(截止目前引用次數(shù)超過5000次):
Raissi M, Perdikaris P, Karniadakis G E. Physics-informed neural networks: A deep learning framework for solving forward and inverse problems involving nonlinear partial differential equations[J]. Journal of Computational physics, 2019, 378: 686-707.
論文地址:https://www.sciencedirect.com/science/article/abs/pii/S0021999118307125
PINN將機(jī)器學(xué)習(xí)應(yīng)用于非線性偏微分方程的求解,其原理是通過訓(xùn)練最小化殘差以近似偏微分方程解。其中殘差綜合計算域內(nèi)離散點的偏微分方程、邊界條件和初始條件(非定常問題),如圖1所示。
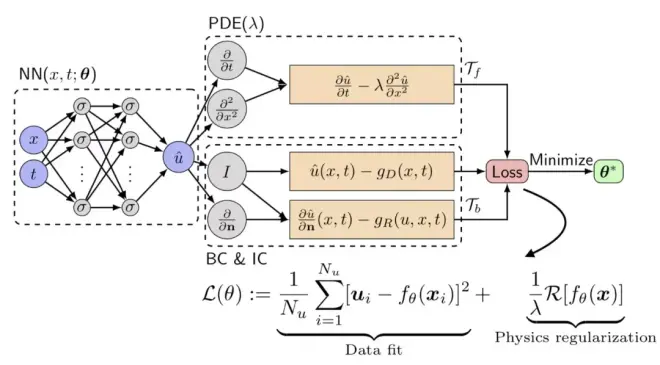
未完待續(xù)。