PoolFormer實(shí)戰(zhàn):使用PoolFormer實(shí)現(xiàn)圖像分類任務(wù)(一)
摘要
論文:https://arxiv.org/abs/2111.11418
論文翻譯:https://blog.csdn.net/hhhhhhhhhhwwwwwwwwww/article/details/128281326
官方源碼:https://github.com/sail-sg/poolformer
模型代碼解析:https://blog.csdn.net/hhhhhhhhhhwwwwwwwwww/article/details/128475827
完整的代碼:https://download.csdn.net/download/hhhhhhhhhhwwwwwwwwww/87357450
MetaFormer是顏水成大佬的一篇Transformer的論文,該篇論文的貢獻(xiàn)主要有兩點(diǎn):第一、將Transformer抽象為一個(gè)通用架構(gòu)的MetaFormer,并通過(guò)經(jīng)驗(yàn)證明MetaFormer架構(gòu)在Transformer/ mlp類模型取得了極大的成功。
第二、通過(guò)僅采用簡(jiǎn)單的非參數(shù)算子pooling作為MetaFormer的極弱token混合器,構(gòu)建了一個(gè)名為PoolFormer。
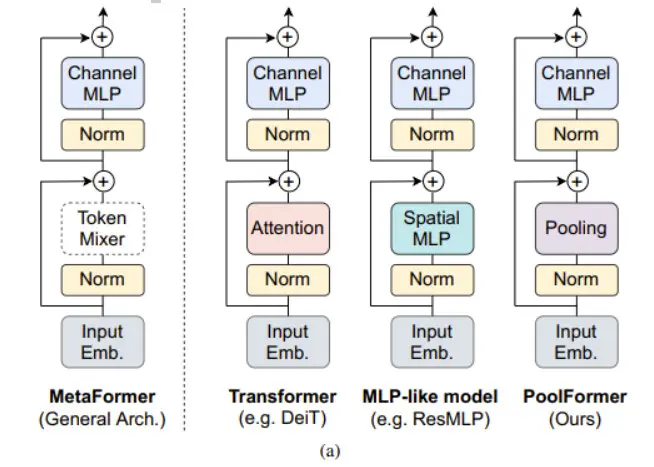
這篇文章主要講解如何使用PoolFormer完成圖像分類任務(wù),接下來(lái)我們一起完成項(xiàng)目的實(shí)戰(zhàn)。本例選用的模型是poolformer_s24,在植物幼苗數(shù)據(jù)集上實(shí)現(xiàn)了97%的準(zhǔn)確率。
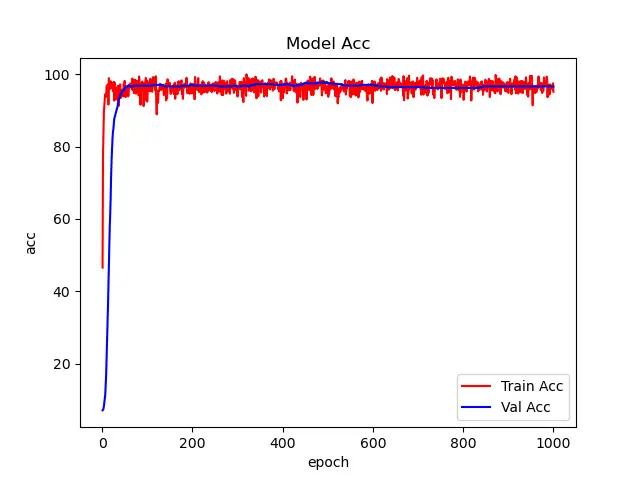
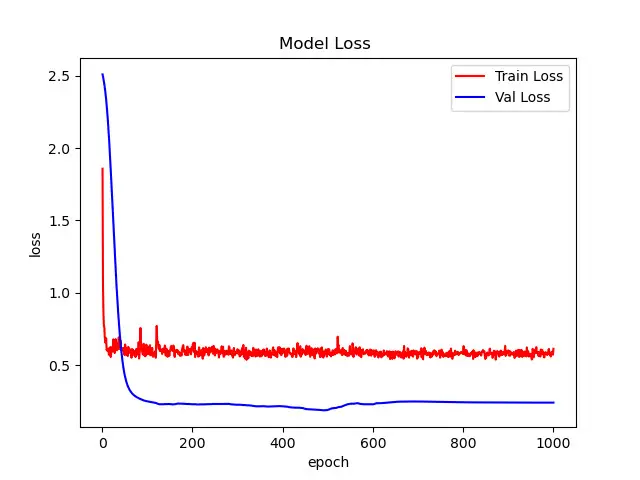
通過(guò)這篇文章能讓你學(xué)到:
如何使用數(shù)據(jù)增強(qiáng),包括transforms的增強(qiáng)、CutOut、MixUp、CutMix等增強(qiáng)手段?
如何實(shí)現(xiàn)PoolFormer模型實(shí)現(xiàn)訓(xùn)練?
如何使用pytorch自帶混合精度?
如何使用梯度裁剪防止梯度爆炸?
如何使用DP多顯卡訓(xùn)練?
如何繪制loss和acc曲線?
如何生成val的測(cè)評(píng)報(bào)告?
如何編寫測(cè)試腳本測(cè)試測(cè)試集?
如何使用余弦退火策略調(diào)整學(xué)習(xí)率?
如何使用AverageMeter類統(tǒng)計(jì)ACC和loss等自定義變量?
如何理解和統(tǒng)計(jì)ACC1和ACC5?
如何使用EMA?
如果使用Grad-CAM 實(shí)現(xiàn)熱力圖可視化?
安裝包
安裝timm
使用pip就行,命令:
pip?install?timm
本文實(shí)戰(zhàn)用的timm里面的模型。
安裝 grad-cam
pip?install?grad-cam
數(shù)據(jù)增強(qiáng)Cutout和Mixup
為了提高成績(jī)我在代碼中加入Cutout和Mixup這兩種增強(qiáng)方式。實(shí)現(xiàn)這兩種增強(qiáng)需要安裝torchtoolbox。安裝命令:
pip?install?torchtoolbox
Cutout實(shí)現(xiàn),在transforms中。
from?torchtoolbox.transform?import?Cutout
#?數(shù)據(jù)預(yù)處理
transform?=?transforms.Compose([
????transforms.Resize((224,?224)),
????Cutout(),
????transforms.ToTensor(),
????transforms.Normalize([0.5,?0.5,?0.5],?[0.5,?0.5,?0.5])
])
需要導(dǎo)入包:from timm.data.mixup import Mixup,
定義Mixup,和SoftTargetCrossEntropy
??mixup_fn?=?Mixup(
????mixup_alpha=0.8,?cutmix_alpha=1.0,?cutmix_minmax=None,
????prob=0.1,?switch_prob=0.5,?mode='batch',
????label_smoothing=0.1,?num_classes=12)
?criterion_train?=?SoftTargetCrossEntropy()
參數(shù)詳解:
★mixup_alpha (float): mixup alpha 值,如果 > 0,則 mixup 處于活動(dòng)狀態(tài)。
”
★ ”
★cutmix_alpha (float):cutmix alpha 值,如果 > 0,cutmix 處于活動(dòng)狀態(tài)。
”
★ ”
★cutmix_minmax (List[float]):cutmix 最小/最大圖像比率,cutmix 處于活動(dòng)狀態(tài),如果不是 None,則使用這個(gè) vs alpha。
”
★ ”
★如果設(shè)置了 cutmix_minmax 則cutmix_alpha 默認(rèn)為1.0
”
★ ”
★prob (float): 每批次或元素應(yīng)用 mixup 或 cutmix 的概率。
”
★ ”
★switch_prob (float): 當(dāng)兩者都處于活動(dòng)狀態(tài)時(shí)切換cutmix 和mixup 的概率 。
”
★ ”
★mode (str): 如何應(yīng)用 mixup/cutmix 參數(shù)(每個(gè)'batch','pair'(元素對(duì)),'elem'(元素)。
”
★ ”
★correct_lam (bool): 當(dāng) cutmix bbox 被圖像邊框剪裁時(shí)應(yīng)用。 lambda 校正
”
★ ”
★label_smoothing (float):將標(biāo)簽平滑應(yīng)用于混合目標(biāo)張量。
”
★ ”
★num_classes (int): 目標(biāo)的類數(shù)。
”
EMA
EMA(Exponential Moving Average)是指數(shù)移動(dòng)平均值。在深度學(xué)習(xí)中的做法是保存歷史的一份參數(shù),在一定訓(xùn)練階段后,拿歷史的參數(shù)給目前學(xué)習(xí)的參數(shù)做一次平滑。具體實(shí)現(xiàn)如下:
import?logging
from?collections?import?OrderedDict
from?copy?import?deepcopy
import?torch
import?torch.nn?as?nn
_logger?=?logging.getLogger(__name__)
class?ModelEma:
????def?__init__(self,?model,?decay=0.9999,?device='',?resume=''):
????????#?make?a?copy?of?the?model?for?accumulating?moving?average?of?weights
????????self.ema?=?deepcopy(model)
????????self.ema.eval()
????????self.decay?=?decay
????????self.device?=?device??#?perform?ema?on?different?device?from?model?if?set
????????if?device:
????????????self.ema.to(device=device)
????????self.ema_has_module?=?hasattr(self.ema,?'module')
????????if?resume:
????????????self._load_checkpoint(resume)
????????for?p?in?self.ema.parameters():
????????????p.requires_grad_(False)
????def?_load_checkpoint(self,?checkpoint_path):
????????checkpoint?=?torch.load(checkpoint_path,?map_location='cpu')
????????assert?isinstance(checkpoint,?dict)
????????if?'state_dict_ema'?in?checkpoint:
????????????new_state_dict?=?OrderedDict()
????????????for?k,?v?in?checkpoint['state_dict_ema'].items():
????????????????#?ema?model?may?have?been?wrapped?by?DataParallel,?and?need?module?prefix
????????????????if?self.ema_has_module:
????????????????????name?=?'module.'?+?k?if?not?k.startswith('module')?else?k
????????????????else:
????????????????????name?=?k
????????????????new_state_dict[name]?=?v
????????????self.ema.load_state_dict(new_state_dict)
????????????_logger.info("Loaded?state_dict_ema")
????????else:
????????????_logger.warning("Failed?to?find?state_dict_ema,?starting?from?loaded?model?weights")
????def?update(self,?model):
????????#?correct?a?mismatch?in?state?dict?keys
????????needs_module?=?hasattr(model,?'module')?and?not?self.ema_has_module
????????with?torch.no_grad():
????????????msd?=?model.state_dict()
????????????for?k,?ema_v?in?self.ema.state_dict().items():
????????????????if?needs_module:
????????????????????k?=?'module.'?+?k
????????????????model_v?=?msd[k].detach()
????????????????if?self.device:
????????????????????model_v?=?model_v.to(device=self.device)
????????????????ema_v.copy_(ema_v?*?self.decay?+?(1.?-?self.decay)?*?model_v)
加入到模型中。
#初始化
if?use_ema:
?????model_ema?=?ModelEma(
????????????model_ft,
????????????decay=model_ema_decay,
????????????device='cpu',
????????????resume=resume)
#?訓(xùn)練過(guò)程中,更新完參數(shù)后,同步update?shadow?weights
def?train():
????optimizer.step()
????if?model_ema?is?not?None:
????????model_ema.update(model)
#?將model_ema傳入驗(yàn)證函數(shù)中
val(model_ema.ema,?DEVICE,?test_loader)
針對(duì)沒(méi)有預(yù)訓(xùn)練的模型,容易出現(xiàn)EMA不上分的情況,這點(diǎn)大家要注意??!
項(xiàng)目結(jié)構(gòu)
PoolFormer_Demo
├─data1
│??├─Black-grass
│??├─Charlock
│??├─Cleavers
│??├─Common?Chickweed
│??├─Common?wheat
│??├─Fat?Hen
│??├─Loose?Silky-bent
│??├─Maize
│??├─Scentless?Mayweed
│??├─Shepherds?Purse
│??├─Small-flowered?Cranesbill
│??└─Sugar?beet
├─mean_std.py
├─makedata.py
├─train.py
├─cam_image.py
└─test.py
mean_std.py:計(jì)算mean和std的值。
makedata.py:生成數(shù)據(jù)集。
ema.py:EMA腳本
train.py:訓(xùn)練PoolFormer模型
cam_image.py:熱力圖可視化
為了能在DP方式中使用混合精度,還需要在模型的forward函數(shù)前增加@autocast(),如果使用GPU訓(xùn)練導(dǎo)入包from torch.cuda.amp import autocast,如果使用CPU,則導(dǎo)入from torch.cpu.amp import autocast。
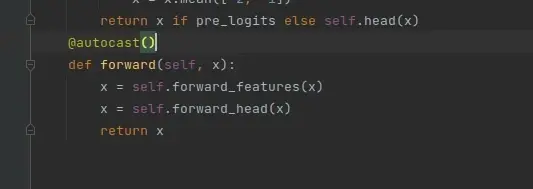
計(jì)算mean和std
為了使模型更加快速的收斂,我們需要計(jì)算出mean和std的值,新建mean_std.py,插入代碼:
from?torchvision.datasets?import?ImageFolder
import?torch
from?torchvision?import?transforms
def?get_mean_and_std(train_data):
????train_loader?=?torch.utils.data.DataLoader(
????????train_data,?batch_size=1,?shuffle=False,?num_workers=0,
????????pin_memory=True)
????mean?=?torch.zeros(3)
????std?=?torch.zeros(3)
????for?X,?_?in?train_loader:
????????for?d?in?range(3):
????????????mean[d]?+=?X[:,?d,?:,?:].mean()
????????????std[d]?+=?X[:,?d,?:,?:].std()
????mean.div_(len(train_data))
????std.div_(len(train_data))
????return?list(mean.numpy()),?list(std.numpy())
if?__name__?==?'__main__':
????train_dataset?=?ImageFolder(root=r'data1',?transform=transforms.ToTensor())
????print(get_mean_and_std(train_dataset))
數(shù)據(jù)集結(jié)構(gòu):
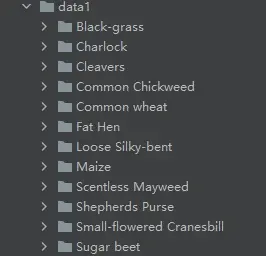
運(yùn)行結(jié)果:
([0.3281186,?0.28937867,?0.20702125],?[0.09407319,?0.09732835,?0.106712654])
把這個(gè)結(jié)果記錄下來(lái),后面要用!
生成數(shù)據(jù)集
我們整理還的圖像分類的數(shù)據(jù)集結(jié)構(gòu)是這樣的
data
├─Black-grass
├─Charlock
├─Cleavers
├─Common?Chickweed
├─Common?wheat
├─Fat?Hen
├─Loose?Silky-bent
├─Maize
├─Scentless?Mayweed
├─Shepherds?Purse
├─Small-flowered?Cranesbill
└─Sugar?beet
pytorch和keras默認(rèn)加載方式是ImageNet數(shù)據(jù)集格式,格式是
├─data
│??├─val
│??│???├─Black-grass
│??│???├─Charlock
│??│???├─Cleavers
│??│???├─Common?Chickweed
│??│???├─Common?wheat
│??│???├─Fat?Hen
│??│???├─Loose?Silky-bent
│??│???├─Maize
│??│???├─Scentless?Mayweed
│??│???├─Shepherds?Purse
│??│???├─Small-flowered?Cranesbill
│??│???└─Sugar?beet
│??└─train
│??????├─Black-grass
│??????├─Charlock
│??????├─Cleavers
│??????├─Common?Chickweed
│??????├─Common?wheat
│??????├─Fat?Hen
│??????├─Loose?Silky-bent
│??????├─Maize
│??????├─Scentless?Mayweed
│??????├─Shepherds?Purse
│??????├─Small-flowered?Cranesbill
│??????└─Sugar?beet
新增格式轉(zhuǎn)化腳本makedata.py,插入代碼:
import?glob
import?os
import?shutil
image_list=glob.glob('data1/*/*.png')
print(image_list)
file_dir='data'
if?os.path.exists(file_dir):
????print('true')
????#os.rmdir(file_dir)
????shutil.rmtree(file_dir)#刪除再建立
????os.makedirs(file_dir)
else:
????os.makedirs(file_dir)
from?sklearn.model_selection?import?train_test_split
trainval_files,?val_files?=?train_test_split(image_list,?test_size=0.3,?random_state=42)
train_dir='train'
val_dir='val'
train_root=os.path.join(file_dir,train_dir)
val_root=os.path.join(file_dir,val_dir)
for?file?in?trainval_files:
????file_class=file.replace("\\","/").split('/')[-2]
????file_name=file.replace("\\","/").split('/')[-1]
????file_class=os.path.join(train_root,file_class)
????if?not?os.path.isdir(file_class):
????????os.makedirs(file_class)
????shutil.copy(file,?file_class?+?'/'?+?file_name)
for?file?in?val_files:
????file_class=file.replace("\\","/").split('/')[-2]
????file_name=file.replace("\\","/").split('/')[-1]
????file_class=os.path.join(val_root,file_class)
????if?not?os.path.isdir(file_class):
????????os.makedirs(file_class)
????shutil.copy(file,?file_class?+?'/'?+?file_name)
完成上面的內(nèi)容就可以開(kāi)啟訓(xùn)練和測(cè)試了。