【自用筆記】 AP Statistics Overview - Section 4.2 Period 1
課本: The Practice of Statistics for the AP Exam (Fifth Edition)
需要原版筆記(.markdown)的請私信,B站格式不全
4.2 Experiments
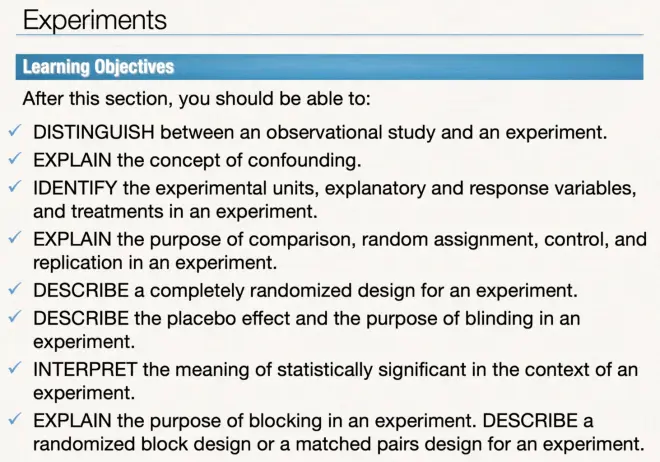
Observational Study and Experiment
Observing: looking and taking notes
Looking - An observational study observes individuals and measures variables of interest but does not attempt to influence the responses.
Imposing - An experiment deliberately imposes some treatment on individuals to measure their responses.
The goal of an observational study can be to describe some group or situation, to compare groups, or to examine relationships between variables.
The purpose of an experiment is to determine whether the treatment causes a change in the response.
When our goal is to understand cause and effect, experiments are the only source of fully convincing data.
Example on P235.
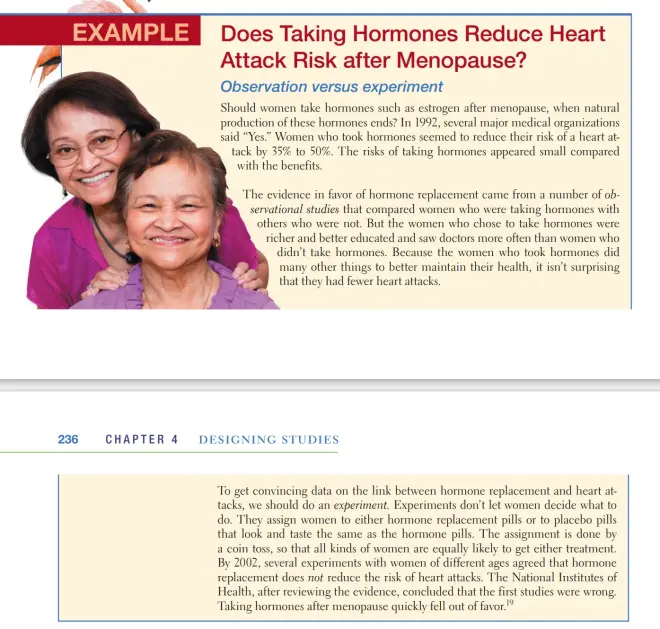
// From Chapter 3: A response variable measures an outcome of a study, while an explanatory variable may help explain or predict changes in a response variable.
Confounding
Observational studies of the effect of an explanatory variable on a response variable often fail because of confounding between the explanatory variable and one or more other variables.
Well-designed experiments take steps to prevent confounding.
Confounding occurs when two variables are associated in such a way that their effects on a response variable cannot be distinguished from each other.
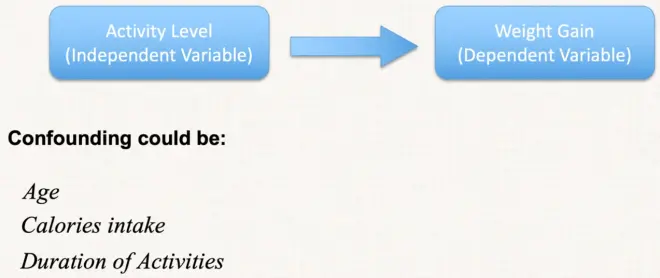
To explain confounding you should explain how the variable you chose is associated with the explanatory variable and also how it affects the response variable.
Example: the water you drink -- the number of time you playing football
Confounding could be: seasons, indoor / outdoor, your position on stage, etc..
*更多參考:偏差(bias)與混淆(confounding) - Anticoder的文章 - 知乎 https://zhuanlan.zhihu.com/p/347813287
AP Exam Tip on P236.
AP Common Error on P236.
Check Your Understanding on P237.
The Language of Experiments
An experiment is a statistical study in which we actually do something (a treatment) to people, animals, or objects (the experimental units) to observe the response. Here is the basic vocabulary of experiments.
Treatment, experimental units, subjects
A specific condition applied to the individuals in an experiment is called a treatment. ?If an experiment has several explanatory variables, a treatment is a combination of ?specific values of these variables.
The experimental units are the smallest collection of individuals to which treatments are applied. When the units are human beings, they often are called subjects.
// The doctor did to you is the treatment; You are the subject (experimental unit) if you go to the doctor.
Often times the explanatory variables in an experiment are called factors.
If an experiment has several factors, the combinations of each level of each factor from the treatments.
Example on P239.
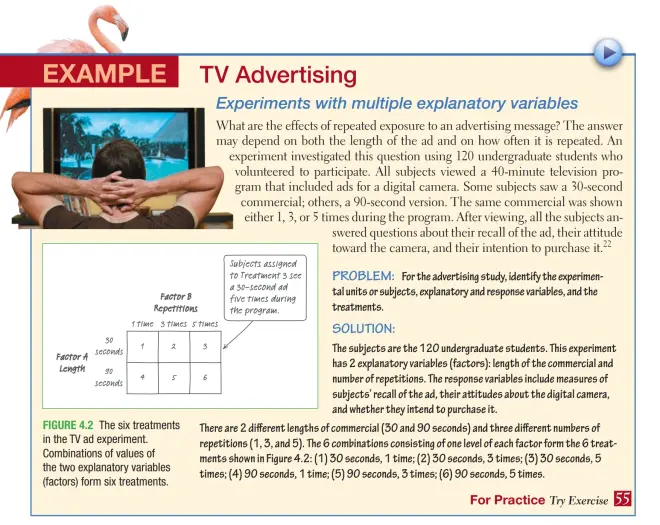
Another example on http://www.ted.com/talks/michael_norton_how_to_buy_happiness#t-107021
Describes a multifactor experiment to determine if money can buy happiness.
There are 2 factors, amount of money & how it is spent.
How to Experiment Badly
Many laboratory experiments use a design like the one in the online SAT course example on P240.
In the lab environment, simple designs often work well.
Field experiments and experiments with animals or people deal with more variable conditions.
Outside the lab, badly designed experiments often yield worthless results because of confounding.
Example on P240.
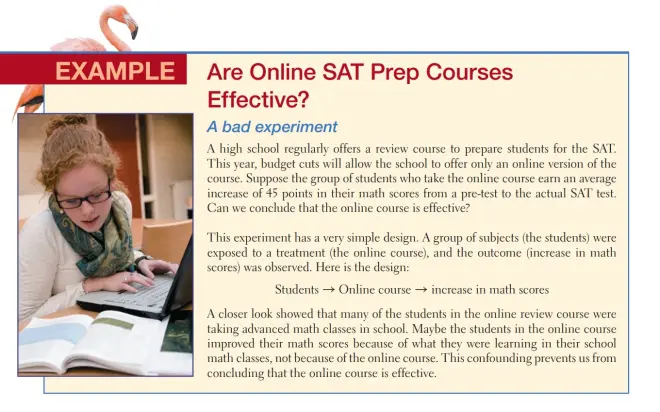
How to Experiment Well
The remedy for confounding is to perform a comparative experiment in which some units receive one treatment and similar units receive another. Most well designed experiments compare two or more treatments.
Comparison alone isn’t enough, if the treatments are given to groups that differ greatly, bias will result. The solution to the problem of bias is random assignment.
Random Assignment
In an experiment, random assignment means that experimental units are assigned to treatments using a chance process
Example on P241.
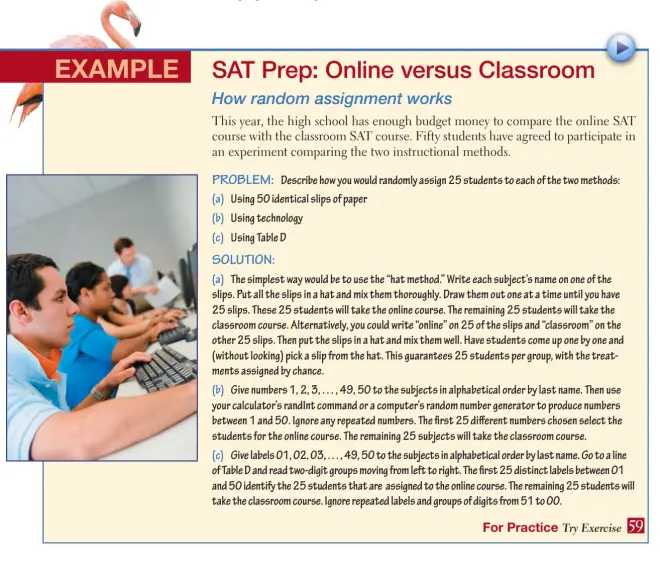
Principles of Experimental Design
The basic principles for designing experiments are as follows:
Comparison. Use a design that compares two or more treatments.
Random Assignment. Use chance to assign experimental units to treatments. Doing so helps create roughly equivalent groups of experimental units by balancing the effects of other variables among the treatment groups.
Control. Keep other variables that might affect the response the same for all groups.
Replication. Use enough experimental units in each group so that any differences in the effects of the treatments can be distinguished from chance differences between the groups
(tbc)