基于RMT的思路,用GPT-3.5-turbo總結(jié)長文本(如綜述論文、長時會議轉(zhuǎn)錄、長報告等)
使用gpt-3.5-turbo總結(jié)了一篇綜述論文:

原論文摘要:
ChatGPT, an artificial intelligence generated content (AIGC) model developed by OpenAI, has attracted worldwide attention for its capability of dealing with challenging language understanding and generation tasks in the form of conversations. This paper briefly provides an overview on the history, status quo and potential future development of ChatGPT, helping to provide an entry point to think about ChatGPT. Specifically, from the limited open-accessed resources, we conclude the core techniques of ChatGPT, mainly including large-scale language models, in-context learning, reinforcement learning from human feedback and the key technical steps for developing ChatGPT. We further analyze the pros and cons of ChatGPT and we rethink the duality of ChatGPT in various fields. Although it has been widely acknowledged that ChatGPT brings plenty of opportunities for various fields, mankind should still treat and use ChatGPT properly to avoid the potential threat, e.g., academic integrity and safety challenge. Finally, we discuss several open problems as the potential development of ChatGPT.
ChatGPT是由OpenAI開發(fā)的一種人工智能生成內(nèi)容(AIGC)模型,因其在處理對話形式的具有挑戰(zhàn)性的語言理解和生成任務(wù)方面的能力而引起全球關(guān)注。本文簡要概述了ChatGPT的歷史、現(xiàn)狀和潛在未來發(fā)展,旨在為思考ChatGPT提供一個切入點。具體來說,通過有限的開放資源,我們總結(jié)了ChatGPT的核心技術(shù),主要包括大規(guī)模語言模型、上下文學(xué)習(xí)、通過人類反饋進行強化學(xué)習(xí)以及開發(fā)ChatGPT的關(guān)鍵技術(shù)步驟。我們進一步分析了ChatGPT的優(yōu)勢和劣勢,并重新思考了ChatGPT在各個領(lǐng)域中的二元性。盡管廣泛認為ChatGPT為各個領(lǐng)域帶來了許多機遇,但人類仍應(yīng)正確對待和使用ChatGPT,以避免潛在的威脅,例如學(xué)術(shù)誠信和安全挑戰(zhàn)。最后,我們討論了ChatGPT的潛在發(fā)展方向中的幾個開放問題。
由GPT-3.5-turbo總結(jié)的摘要:
Understanding ChatGPT: Pros, Cons, and Future Developments
ChatGPT, an artificial intelligence-generated content model developed by OpenAI for language understanding and generation tasks, has been making waves in the technology industry. With over 100 million monthly active users as of January 2023, ChatGPT showcases the potential for achieving artificial general intelligence and powering a myriad of applications. However, as new technological advancements often come with both opportunities and risks, it is essential to understand the pros, cons, and future developments of ChatGPT.
Core Techniques of ChatGPT
The core techniques of ChatGPT include large-scale language models, in-context learning, reinforcement learning from human feedback, and key technical steps for development. ChatGPT is built upon the GPT model and leverages various technologies like deep learning and reinforcement learning. The GPT model has undergone multiple updates from GPT-1 to GPT-4, with each update introducing new features and parameter scales, ranging from multi-task learning to in-context learning. ChatGPT uses reinforcement learning with human feedback to incrementally train the GPT-3 model for better alignment with user intent. GPT-4 exhibits human-level performance on various benchmarks and is expected to have even larger parameters and data.
Pros and Cons of ChatGPT
ChatGPT exhibits outstanding performance in various language tasks, including multilingual translation, code debugging, and multimodal generation. It also showcases strong reasoning ability and creativity, able to answer complex questions and analyze pictures, and complete tasks specified by users with logical thinking. Its knowledge modeling ability and large pre-trained model enable ChatGPT to have good results in specialized areas like the medical and financial fields.
Despite its prowess, ChatGPT poses potential threats to academic integrity and safety, including generating factual errors and hallucination results. ChatGPT's randomness can lead to serious consequences and lack the ability to clarify and confirm fuzzy queries, raising ethical and legal concerns. Additionally, the costs of research and development are high to achieve stable training and excellent performance.
Future Developments of ChatGPT
Future developments for ChatGPT should focus on advancing knowledge representation and reasoning techniques, model compression, and incorporation of external knowledge. Enhancing ChatGPT's reasoning abilities through knowledge representation and logical reasoning techniques can make the model more versatile and powerful. Model compression is also crucial, as transformer-based language models grow log-linearly with the number of parameters and come with significant costs.
Regarding incorporating external knowledge sources, research has shown that this can improve accuracy and reduce the potential for generating incorrect information. However, effective retrieval methods are necessary to achieve these benefits. The retrieval-augmented language model has emerged as a popular approach to mitigate "hallucination" in language models. Different research perspectives have also explored CoT prompting, generating prompts and sub-tasks, and Instruction fine-tuning (IFT) methods to improve the general ability of language models to understand and process natural language instructions.
Conclusion
ChatGPT's development and benefits in natural language processing are significant, as it enables humans to accomplish tasks quickly and accurately in various industries like finance and media. However, ethical and legal considerations must be carefully considered to ensure sustainability and avoid violating regulations. Future research on ChatGPT should focus on improving its robustness, transparency, and long-term memory, expanding its application, and addressing potential ethical concerns. With more advancements, AI models like ChatGPT will have higher capacities to solve tasks and more extensive implications in various fields.
--------------------------
了解ChatGPT:優(yōu)勢、劣勢和未來發(fā)展
ChatGPT是由OpenAI開發(fā)的用于語言理解和生成任務(wù)的人工智能生成內(nèi)容模型,在科技行業(yè)引起了轟動。截至2023年1月,ChatGPT每月活躍用戶已超過1億,展示了實現(xiàn)人工通用智能和驅(qū)動各種應(yīng)用的潛力。然而,由于新的技術(shù)進步通常帶來機遇和風(fēng)險,了解ChatGPT的優(yōu)勢、劣勢和未來發(fā)展是至關(guān)重要的。
ChatGPT的核心技術(shù)
ChatGPT的核心技術(shù)包括大規(guī)模語言模型、上下文學(xué)習(xí)、通過人類反饋進行強化學(xué)習(xí)以及開發(fā)的關(guān)鍵技術(shù)步驟。ChatGPT建立在GPT模型之上,并利用了深度學(xué)習(xí)和強化學(xué)習(xí)等各種技術(shù)。GPT模型經(jīng)歷了從GPT-1到GPT-4的多次更新,每次更新都引入了新的功能和參數(shù)規(guī)模,從多任務(wù)學(xué)習(xí)到上下文學(xué)習(xí)。ChatGPT使用強化學(xué)習(xí)和人類反饋逐步訓(xùn)練GPT-3模型,以更好地與用戶意圖對齊。GPT-4在各種基準測試中展現(xiàn)出人類水平的表現(xiàn),并預(yù)計具有更大的參數(shù)和數(shù)據(jù)。
ChatGPT的優(yōu)勢和劣勢
ChatGPT在各種語言任務(wù)中展現(xiàn)出出色的性能,包括多語言翻譯、代碼調(diào)試和多模態(tài)生成。它還展示了強大的推理能力和創(chuàng)造力,能夠回答復(fù)雜問題、分析圖片,并通過邏輯思維完成用戶指定的任務(wù)。其知識建模能力和大規(guī)模預(yù)訓(xùn)練模型使得ChatGPT在醫(yī)療和金融等專業(yè)領(lǐng)域取得良好的結(jié)果。
盡管ChatGPT表現(xiàn)出色,但它也帶來了對學(xué)術(shù)誠信和安全的潛在威脅,包括生成事實錯誤和幻覺結(jié)果。ChatGPT的隨機性可能導(dǎo)致嚴重后果,并且缺乏澄清和確認模糊查詢的能力,引發(fā)了道德和法律方面的擔(dān)憂。此外,為了實現(xiàn)穩(wěn)定的訓(xùn)練和優(yōu)秀的性能,研發(fā)成本也很高。
ChatGPT的未來發(fā)展
ChatGPT的未來發(fā)展應(yīng)重點關(guān)注知識表示和推理技術(shù)、模型壓縮和外部知識的整合。通過知識表示和邏輯推理技術(shù)增強ChatGPT的推理能力可以使模型更加多功能和強大。模型壓縮也至關(guān)重要,因為基于Transformer的語言模型的參數(shù)數(shù)量與其增長是對數(shù)線性的,并伴隨著巨大的成本。
關(guān)于整合外部知識源,研究表明這可以提高準確性并減少生成不正確信息的可能性。然而,需要有效的檢索方法來實現(xiàn)這些好處。檢索增強語言模型已經(jīng)成為減輕語言模型中的“幻覺”的一種流行方法。不同的研究觀點還探索了CoT提示、生成提示和子任務(wù)以及指令微調(diào)(IFT)方法,以提高語言模型理解和處理自然語言指令的通用能力。
結(jié)論
ChatGPT在自然語言處理方面的發(fā)展和好處是顯著的,它使人類能夠在金融和媒體等各個行業(yè)中快速準確地完成任務(wù)。然而,必須仔細考慮道德和法律問題,以確??沙掷m(xù)性并避免違反規(guī)定。對ChatGPT的未來研究應(yīng)重點關(guān)注提高其穩(wěn)健性、透明度和長期記憶,擴展其應(yīng)用,并解決潛在的倫理問題。隨著更多的進展,像ChatGPT這樣的AI模型將在各個領(lǐng)域具有更高的任務(wù)解決能力和更廣泛的影響。
論文名稱:
A Brief Overview of ChatGPT: The History, Status Quo and Potential Future Development
論文鏈接:
https://www.sciengine.com/JAS/doi/10.1109/JAS.2023.123618

思路:
RMT(Recurrent Memory Transformer)在加入記憶塊Memory以后效果較好[1],原理是在每次生成輸出時,一部分的輸出內(nèi)容作為記憶塊Memory,記憶上文內(nèi)容。這使得可以用Transformer進行段落級別的循環(huán),處理超長文本。這種循環(huán)與RNNs的區(qū)別在于,RNNs只在token層次上進行循環(huán),效率低下;而RMT則在段落層次上進行循環(huán),擁有更高的效率。
對于長文本,由于Transformer模型寬度有限,只能分割處理,如下:
現(xiàn)在思考一個問題:
對于可以進行Prompt Engineering的預(yù)訓(xùn)練Transformer,如GPT-3.5,可否通過prompt控制模型的輸出格式,對于分割成多個segment的原文進行循環(huán)處理,按照【記憶塊mem?+?內(nèi)容塊segment】的格式進行response?
即:當(dāng)?shù)?img type="latex" class="latex" src="http://api.bilibili.com/x/web-frontend/mathjax/tex?formula=n" alt="n">段輸入提交給模型時,結(jié)構(gòu)應(yīng)為:
此時,第段的輸出為:
這樣,每一段輸入都可以“看到”上文內(nèi)容,保證模型對內(nèi)容的理解不跑偏,在Transformer模型寬度有限的情況下,可以按順序循環(huán)處理所有段落。
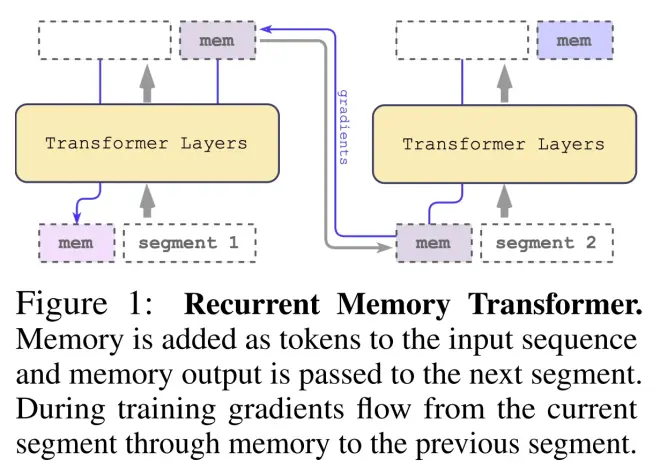
上文論文的分點摘要:
1. ChatGPT is an AI-generated content model developed by OpenAI for language understanding and generation tasks.
2. The core techniques of ChatGPT include large-scale language models, in-context learning, reinforcement learning from human feedback, and key technical steps for development.
3. ChatGPT has both pros and cons, bringing opportunities for various fields but also posing potential threats to academic integrity and safety.
4. Open problems for potential ChatGPT development remain to be explored.
5. AI development has progressed significantly, with the development of GAN, CLIP, diffusion models, and multimodal generation.
6. Various AIGC products were developed and iterated by leading technology companies in 2022.
7. OpenAI released ChatGPT, an intelligent chatting robot which surpassed 100 million monthly active users by the end of January 2023.
8. ChatGPT performs various language tasks, including multilingual translation and code debugging.
9. ChatGPT can remember previous conversations for continuous dialogue and has been updated with more functions.
10. Users can input texts and visual images in parallel for multimodal tasks.
11. The authors of ChatGPT are from various institutions in China and Australia.
12. ChatGPT is built upon the GPT model and uses multiple technologies like deep learning and reinforcement learning.
13. The GPT model has been iteratively updated from GPT-1 to GPT-4.
14. GPT model has gone through multiple updates and uses various technologies.
15. GPT-2 introduces multi-task learning with more parameters and data than GPT.
16. GPT-3 combines meta-learning with in-context learning and has a parameter scale 100 times than GPT-2.
17. ChatGPT uses reinforcement learning with human feedback to incrementally train the GPT-3 model for better alignment with user intent, and GPT-4 exhibits human-level performance on various benchmarks.
18. GPT-4 is expected to have even larger parameters and data.
19. ChatGPT/GPT-4 shows promising performance as a general purpose task-solver.
20. Strong creativity of ChatGPT is a double-edged sword, requiring discussion on prevention of cheating and abuse.
21. Comparison of GPT models in terms of parameters, context window, and pre-training data size.
22. Core techniques of ChatGPT, including pre-trained large-scale language models and reinforcement learning from human feedback.
23. Pros and cons of ChatGPT and potential impact on various social fields discussed.
24. The core technologies behind ChatGPT include large-scale language models and reinforcement learning.
25. Different modeling methods for statistical language models represent the technical level of natural language processing.
26. ChatGPT/InstructGPT implementation involves three main steps: supervised fine-tuning, reward modeling, and dialogue-oriented reinforcement learning from human feedback.
27. Language models face limitations due to symbol combinations.
28. Pre-trained language models have become popular with self-supervised learning on large-scale texts.
29. PLMs improve semantic description of words and provide a unified modeling framework for NLP tasks.
30. This paragraph discusses the development and benefits of pre-trained language models in natural language processing.
31. There are three typical model structures of PLMs: autoregressive LM, autoencoding LM, and hybrid LM.
32. BERT and its autoencoding pre-training methods are widely followed by academia and industry.
33. OpenAI still adheres to the autoregressive method with the best neural network structure Transformer.
34. GPT-2 and GPT-3 have larger network parameters and can perform text generation tasks like machine translation and story generation.
35. GPT-3 has excellent performance and supports zero-shot and few-shot learning scenarios.
36. OpenAI has continued to develop new GPT models, such as GPT-3.5 and GPT-4, with technical improvements and scaling up deep learning.
37. Large language models learn abstract knowledge from raw data, improving their generality and generalization.
38. GPT-3's autoregressive language model directly utilizes natural language to describe tasks in different fields.
39. In-context learning (ICL) introduced by GPT-3 models plays a crucial role in improving task performance and handling zero-shot and few-shot learning scenarios.
40. In-context learning (ICL) is a new paradigm for meta-learning in NLP.
41. ICL allows models to learn and complete tasks through imitation and analogical learning, without parameter updates.
42. ICL can improve zero-shot and few-shot learning by appending exemplars into the context as prompts.
43. CoT prompting helps improve task-solving abilities using ICL without fine-tuning.
44. Hybrid LM models and bidirectional encoders with masked based decoding have been explored.
45. PLMs can be used for complex tasks such as answering arithmetic, commonsense, and logical reasoning questions using CoT prompting.
46. Different research perspectives have been explored for CoT, such as generating prompts and sub-tasks.
47. Instruction fine-tuning (IFT) is a method used to improve the general ability of language models to understand and process natural language instructions.
48. FLAN is an IFT model that uses natural language instructions to adjust model parameters and improve performance on specific NLP tasks.
49. Reinforcement learning (RL) focuses on learning an optimal policy to maximize rewards or reach specific targets.
50. Reinforcement learning can learn optimal policies for maximizing rewards or reaching specific targets.
51. The general paradigm of reinforcement learning is a Markov decision process (MDP) that learns an optimal policy and maximizes reward.
52. Reinforcement learning has shown strong capacities on tasks with large action spaces like gaming, robotics control, and molecular optimization.
53. The goal of MDP is to learn an optimal policy and maximize cumulative reward within an episode.
54. Reinforcement learning is a developed tool for maximizing reward in tasks with large action spaces.
55. The general paradigm of RL is a Markov decision process (MDP) for learning optimal policies.
56. Language models can be finetuned on various tasks, with examples used to address tasks.
57. RL algorithms like TRPO, SAC, PPO, TD3, and REDQ have been developed for different situations.
58. Constructing a precise reward function for complex and poorly-defined tasks is difficult, so human intuition or expertise is considered for knowledge transfer.
59. Using human feedback directly for training the agent is expensive, so a reward model is trained to replace this work.
60. The process of training the reward model involves interaction with the environment, selecting pairs of segments, and training the model.
61. RLHF can be used to fine-tune language models by aligning them with human preferences.
62. InstructGPT uses supervised fine-tuning and reward modeling.
63. Incorporating a safety reward signal can improve the safety of language models.
64. InstructGPT uses supervised fine-tuning and reward modeling techniques.
65. Labeled data is gathered from the OpenAI API and manual annotation.
66. SFT requires about 13k training prompts.
67. InstructGPT generates multiple responses for each prompt using supervised models and manual annotation.
68. The rankings of the responses are converted to scalar rewards for the Reward Model (RM) dataset.
69. The RM model is trained using the scalar rewards and a loss function.
70. The RL model in InstructGPT maximizes a function that includes a scoring function, KL divergence, and pre-training target.
71. KL divergence measures the distance between distributions generated by PPO and SFT models.
72. The pre-training target is based on GPT-3 and contains 31k training prompts.
73. ChatGPT is a powerful general-purpose conversation system based on GPT-4.
74. InstructGPT combines human-labeled data and reinforcement learning for dialogue tasks.
75. ChatGPT has strong multimodal understanding abilities for text and image generation.
76. ChatGPT exceeds human-level performance in several tasks, including text generation.
77. ChatGPT surpasses human-level performance in various tasks, including text generation.
78. ChatGPT has strong reasoning ability and creativity, able to answer complex questions and analyze pictures.
79. ChatGPT can complete tasks specified by users in a logical chain.
80. ChatGPT can collaborate with users on writing tasks and perform logical thinking due to its training on code data.
81. ChatGPT has good results in specialized areas like medical and financial fields due to its knowledge modeling ability and large pre-trained model.
82. ChatGPT has preliminary intelligent planning capabilities for complex questions, writing, and task execution order.
83. ChatGPT's large language model has powerful knowledge modeling abilities.
84. ChatGPT has general task processing, machine translation, and embodied interaction capabilities.
85. ChatGPT can generate factual errors and hallucination results due to errors in training data and the black box nature of deep learning models.
86. ChatGPT's limitations should be considered in high accuracy application scenarios such as medical consultation.
87. ChatGPT's randomness can lead to serious consequences and it lacks the ability to clarify and confirm fuzzy queries.
88. ChatGPT has insufficient modeling of explicit knowledge and is limited in producing and modeling accurate information.
89. The high costs of research and development are necessary to achieve stable training and excellent performance.
90. OpenAI requires high computing costs and engineering experience for stable and persistent model training, such as the basic large model GPT-3.
91. ChatGPT has limitations in reliability, explicit knowledge modeling, and high research and development costs that need further improvement.
92. ChatGPT has become a popular topic of discussion in various industries, with over 100 million monthly active users as of January 2023.
93. ChatGPT is a large-scale generative language model with the potential for achieving artificial general intelligence.
94. ChatGPT assists humans in a wide range of industries by generating content in a conversational manner, overcoming data limitations, and reducing costs.
95. The development of generative AI technology may assist or even replace humans in content creation work in the future.
96. ChatGPT can enhance work efficiency and quality in various industries, such as finance and media.
97. ChatGPT may bring challenges and risks, such as intellectual property protection and cyber safety.
98. ChatGPT's automatic generation of data makes it difficult to verify the source and may lead to copyright infringement and cyber attacks.
99. ChatGPT poses cyber threats and intellectual property disputes.
100. Ethical concerns arise as its responses make it difficult to detect plagiarism and may encourage cheating.
101. The environmental impact of ChatGPT is significant due to the resources required for its training and usage.
102. ChatGPT technology must abide by ethical and legal principles to ensure sustainability and avoid violating regulations.
103. Environmental protection and sustainability should be prioritized when using ChatGPT due to its potential impact on computing resources and carbon emissions.
104. Future development of ChatGPT must address the hallucination problem and find ways to reduce the size of language models for model compression.
105. Additional trends for future development include lifelong learning, complex reasoning, cross-disciplinary approaches, and incorporation of external knowledge.
106. Future developments for ChatGPT should focus on advancing knowledge representation and reasoning techniques.
107. Incorporating external knowledge sources can improve accuracy and reduce the potential for generating incorrect information, but poses challenges requiring effective retrieval methods.
108. The retrieval-augmented language model has emerged as a popular approach to mitigate "hallucination" in language models.
109. Transformer-based language models grow log-linearly with the number of parameters.
110. Large language models come with significant costs and need compression and optimization for practical usage.
111. Multiple approaches have been proposed for model compression, such as distillation-based approaches.
112. Pruning-based approaches aim to reduce model size by removing unimportant weights while maintaining performance.
113. Quantization-based approaches use 8-bit or binary numbers to store model weights, reducing size, but special hardware design may be necessary.
114. Lifelong learning incorporation could allow ChatGPT to seamlessly incorporate new data without requiring retraining from scratch.
115. Lifelong learning can improve the performance of language models like ChatGPT by adapting to real-time data and providing more personalized results.
116. Enhancing ChatGPT's reasoning abilities through knowledge representation and logical reasoning techniques can make the model more versatile and powerful.
117. ChatGPT's language processing performance has the potential for cross-disciplinary integration with chemical systems like SMILES.
118. ChatGPT can be integrated with chemical systems to enhance its versatility.
119. Future research on ChatGPT should focus on improving long-term memory, robustness and transparency, and considering external calls for application expansion.
120. ChatGPT is a powerful technology, but it is unclear how it achieves its functions.
121. The paragraph discusses potential future trends and directions for research on ChatGPT.
122. Research on emergence in language models is important for creating stronger AI models.
123. Many companies are developing ChatGPT-like products for various applications.
124. Side-effects and ethical considerations of AI must be carefully considered as technology advances.
可以看到確實可以處理長文本,并且效果不錯。
References
[1]?Bulatov, Aydar, Yury Kuratov, and Mikhail Burtsev. "Recurrent memory transformer."?Advances in Neural Information Processing Systems?35 (2022): 11079-11091.?
https://arxiv.org/abs/2207.06881