基于R統(tǒng)計軟件的三次樣條和光滑樣條模型數(shù)據(jù)擬合及預(yù)測
原文鏈接:http://tecdat.cn/?p=9670
?
樣條線是擬合非線性模型并從數(shù)據(jù)中學(xué)習(xí)非線性相互作用的一種方法。?
三次樣條
?三次樣條 具有連續(xù)的一階和二階導(dǎo)數(shù)。 我們通過應(yīng)用基礎(chǔ)函數(shù)來變換變量? 并使用這些變換后的變量擬合模型, 向模型添加非線性, 使樣條曲線能夠擬合更光滑 。
?
require(splines)
#ISLR包含數(shù)據(jù)集
require(ISLR)
attach(Wage) #綁定工資數(shù)據(jù)集
agelims<-range(age)
#生成測試數(shù)據(jù)
age.grid<-seq(from=agelims[1], to = agelims[2])
?三次樣條?
? R中使用函數(shù)擬合三次樣條。
#在年齡 25 ,50 ,60設(shè)置3個分割點
fit<-lm(wage ~ bs(age,knots = c(25,40,60)),data = Wage )
summary(fit)
##
## Call:
## lm(formula = wage ~ bs(age, knots = c(25, 40, 60)), data = Wage)
##
## Residuals:
## ? ? Min ? ? ?1Q ?Median ? ? ?3Q ? ? Max
## -98.832 -24.537 ?-5.049 ?15.209 203.207
##
## Coefficients:
## ? ? ? ? ? ? ? ? ? ? ? ? ? ? ? ? Estimate Std. Error t value Pr(>|t|)
## (Intercept) ? ? ? ? ? ? ? ? ? ? ? 60.494 ? ? ?9.460 ? 6.394 1.86e-10 ***
## bs(age, knots = c(25, 40, 60))1 ? ?3.980 ? ? 12.538 ? 0.317 0.750899
## bs(age, knots = c(25, 40, 60))2 ? 44.631 ? ? ?9.626 ? 4.636 3.70e-06 ***
## bs(age, knots = c(25, 40, 60))3 ? 62.839 ? ? 10.755 ? 5.843 5.69e-09 ***
## bs(age, knots = c(25, 40, 60))4 ? 55.991 ? ? 10.706 ? 5.230 1.81e-07 ***
## bs(age, knots = c(25, 40, 60))5 ? 50.688 ? ? 14.402 ? 3.520 0.000439 ***
## bs(age, knots = c(25, 40, 60))6 ? 16.606 ? ? 19.126 ? 0.868 0.385338
## ---
## Signif. codes: ?0 '***' 0.001 '**' 0.01 '*' 0.05 '.' 0.1 ' ' 1
##
## Residual standard error: 39.92 on 2993 degrees of freedom
## Multiple R-squared: ?0.08642, ? ?Adjusted R-squared: ?0.08459
## F-statistic: 47.19 on 6 and 2993 DF, ?p-value: < 2.2e-16
?繪制回歸線
?
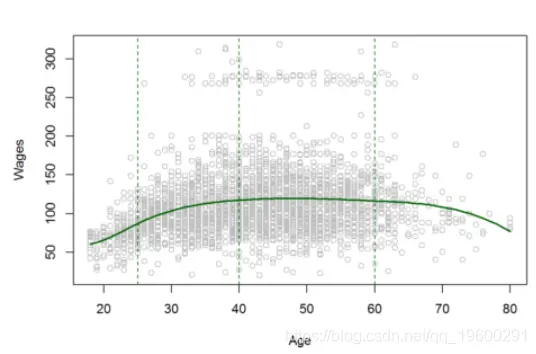
上圖顯示了三次樣條曲線的光滑和局部效果。
光滑樣條線
?我們在光滑樣條曲線中的目的是通過添加粗糙度最小化誤差函數(shù) 。
?
?
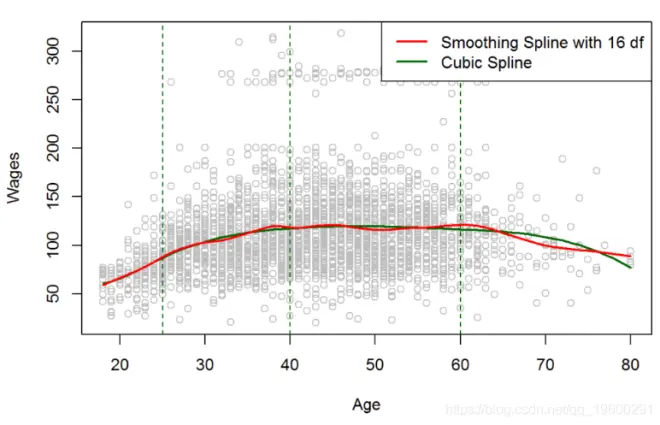
現(xiàn)在我們可以注意到,紅線(即“光滑樣條線”)更加波動,并且更靈活地擬合數(shù)據(jù)。這可能是由于高度的自由度所致。選擇參數(shù)?λ 和DF最好的辦法?是交叉驗證。?
實施交叉驗證以選擇λ值并繪制光滑樣條線:
fit2
## Call:
## smooth.spline(x = age, y = wage, cv = TRUE)
##
## Smoothing Parameter ?spar= 0.6988943 ?lambda= 0.02792303 (12 iterations)
## Equivalent Degrees of Freedom (Df): 6.794596
## Penalized Criterion: 75215.9
## PRESS: 1593.383
結(jié)果選擇了lambda=0.0279和df = 6.794596?
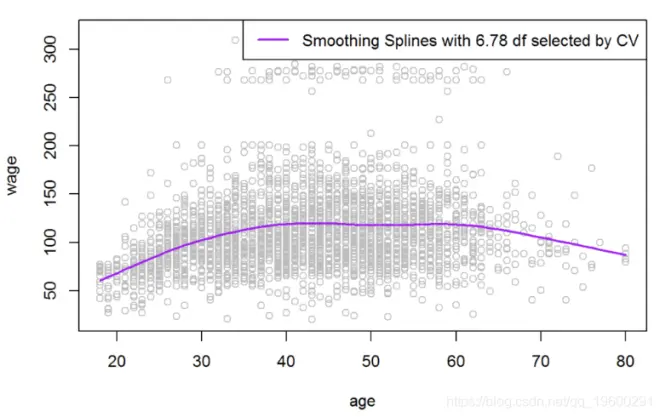
該模型也非常光滑,可以很好地擬合數(shù)據(jù)。
結(jié)論
因此, 我們需要對數(shù)據(jù)或變量進行一些轉(zhuǎn)換,以使模型在學(xué)習(xí)輸入X?i和輸出??Y之間的非線性相互作用時更靈活,更強大。?
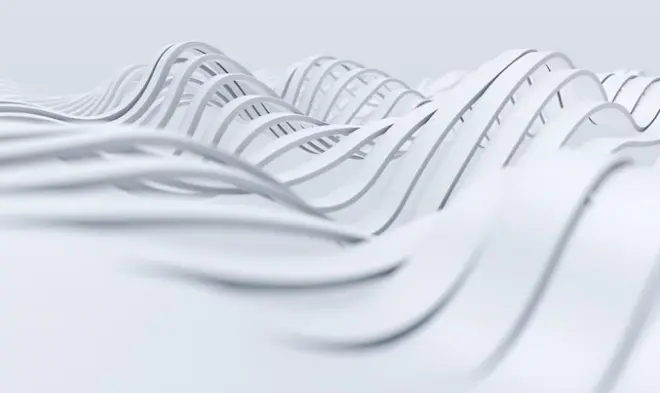
最受歡迎的見解
1.R語言多元Logistic邏輯回歸 應(yīng)用案例
2.面板平滑轉(zhuǎn)移回歸(PSTR)分析案例實現(xiàn)
3.matlab中的偏最小二乘回歸(PLSR)和主成分回歸(PCR)
4.R語言泊松Poisson回歸模型分析案例
5.R語言回歸中的Hosmer-Lemeshow擬合優(yōu)度檢驗
6.r語言中對LASSO回歸,Ridge嶺回歸和Elastic Net模型實現(xiàn)
7.在R語言中實現(xiàn)Logistic邏輯回歸
8.python用線性回歸預(yù)測股票價格
9.R語言如何在生存分析與Cox回歸中計算IDI,NRI指標(biāo)